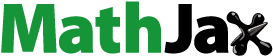
ABSTRACT
This paper provides the relationship between Economic Policy Uncertainty (EPU) and international tourism activities in the BRIC countries (i.e. Brazil, Russia, India and China). The researchers used continuous wavelets, wavelet coherence and wavelet phase-difference based on Granger causality analysis to investigate the relationship between EPU and tourism activities using the annual data. The findings show that there is a unidirectional causal influence of EPU on international tourism arrivals (ITA) in the short run and mid run for Brazil. In addition, there is a bi-directional causal influence of EPU on ITA in China and Russia in the long run.
Introduction
The coronavirus disease 2019 (COVID-19) pandemic has become a global issue. Many experts predicted that not only this pandemic but also the global economic recession would cause global economic development to decline in 2020. All the massive efforts conducted by many countries in the world to prevent the spread of COVID-19 such as social distancing, self-isolation and similar actions to lockdown may have had a major impact on tourism demand in many countries. According to UNWTO (Citation2020), the COVID-19 pandemic had caused a 22% reduction in international tourist arrivals during the first quarter of 2020 and these might decline by 60%-80% throughout 2020. Recent data from UNWTO show that tourist arrivals in March fell by 57% after lockdowns began in many countries, along with broad travel restrictions, airport closures and national borders, indicating the loss of 67 million international arrivals and around US$ 80 billion in revenues (exports from tourism). If this decline continues up to 80% compared to 2019, it is estimated that international tourist revenues will decrease by US$ 850 million to US$ 1.1 billion; export revenues from tourism will decline by US$ 910 billion to US$ 1.2 trillion; and 100–120 million direct tourism jobs are at risk. The economic and political crises, contagious diseases, financial crises, partisan disputes, social unrest in countries together with the severe acute respiratory syndrome (SARS) of 2002–2003, avian influenza (H5N1) of 2004–2006, Middle East respiratory syndrome coronavirus (MERS-CoV) of 2012 and COVID-19, raise concerns about the transmission of policy uncertainty in several key sectors of the economy including tourism activities. The tourism industry is one of the most important sectors in the world, but very vulnerable to crises, disasters and contagious diseases. The uncertainty resulting from events such as the 9/11 attacks, the Paris attacks, the Bali bombings, SARS, H5N1, MERS-CoV, COVID-19, Hurricane Katrina, the Indian Ocean tsunami and the Iran-Iraq War among others, has drastically influenced tourism demand. However, economic crises have also influenced the tourism industry through their impacts on labor markets and incomes. The crises have had global repercussions. The tourism sector does not provide products to meet basic needs. Therefore, the tourism industry should immediately reflect tourism activities with a reduction in consumption of tourism products and expenditure. In fact, tourism products are non-necessary goods and are not a priority for consumption, which enables them to be very susceptible to income variations. From a macroeconomics formula: Y = C + I+G+(X-M), all civil consumption, business investment, government expenditure and net exports may increase when the tourism industry is booming in a country. Subsequently, tourism development contributes to the national income. As a result, the economy and housing prices (HP) increase simultaneously. Therefore, there has already been interest in the relationship between tourism activities and HP development even though the theoretical ground of such relationships is not well defined. The housing economies of the world have become more interconnected than they have ever been; however, this phenomenon is no doubt a direct result of the HP development problems effect.
The relationship between economic policy uncertainty (EPU) and tourism activities has been extensively studied around several main strands. The methods used in the previous studies are diverse and the econometric models used in the existing literature include cross-sectional analysis for a group of countries versus a time-series analysis for a single country, and single equations versus simultaneous equations using large samples or breaking the sample up according to economic and non-economic structural features. The researchers have used classical analysis methods based on auto-regressive models, and linear or non-linear co-intergeneration techniques, such as the Johansen and fractional and threshold co-intergeneration tests, which have been frequently applied in the first strand of the literature to focus on whether the financial crisis or EPU and tourism activities have cyclical or anti-cyclical association among the series (e.g. Andraz & Rodrigues, Citation2016; Perles-Ribes et al., Citation2016).
This work proposes to utilize a novel wavelet analysis to explore the real relationship between EPU and tourism activities in both the time and frequency domains. Wavelet analysis has significant superiority over those conventional time-domain methods employed in the previous studies. It expands the underlying time series into a time-frequency space where both time- and-frequency varying information of the series can be visualized in a highly intuitive way. By means of wavelet coherence and wavelet phase-difference, a simultaneous assessment of how the co-movement and causalities between EPU and tourism activities vary across frequencies and change over time is further achieved in a time-frequency window. In this way, the high-frequency (short-term) and low-frequency (long-term) relationship between EPU and tourism activities, as well as possible structural changes and time variations in such relationship can be clearly observed. The applications of wavelet analysis in economics and finance were introduced by Ko and Lee (Citation2015); however, they have been performed popularly until recent years by focusing on the co-movement between stock markets as well as between energy commodities and the macro-economy (e.g. Raza et al., Citation2017).
This study contributes to the existing literature in several important ways. First of all, in an attempt to capture the variations in the relationship at different time dimensions the researchers apply wavelet-based techniques. The wavelet transformation allows localized time–frequency decomposition, which enables gauge time–frequency domain based interdependencies. Wavelet-based techniques are superior as they are immune to the limitations of many standard econometric techniques and data analysis on a time–frequency domain (Raza et al., Citation2017). This method is able to discern the relationship between a pair of time-series. Secondly, the researchers used the distinctive data of EPU and international tourist arrivals (ITA) of Baker et al. (Citation2016) over a long period from 1995 to 2015, implying that our findings would be more suggestive for practitioners and policy-makers in EPU and tourism activities. Accordingly, policy-makers can use tourism activities as a policy tool for falling regional welfare inequalities (e.g. Aprilani, Citation2021; Calero & Turner, Citation2020; de Souza Neto & Marques, Citation2021; Lanzara & Minerva, Citation2019; Zaroki et al., Citation2018). Thirdly, this study applies the real gross domestic product (RGDP) and international tourism receipts (ITR) as two control variables to reveal the true relationship between EPU and tourism activities by removing the effect of economic growth on both EPU and tourism performance. Subsequently, the partial wavelet coherence and wavelet phase-difference are also estimated in this paper as necessary complements to the commonly used wavelet coherence and wavelet phase-difference. Lastly, this paper performs a simultaneous assessment of tourism co-movement and causal relations in both the time and frequency domains. The empirical results of this study show very substantial time- and-frequency varying features in the co-movement and causality between EPU and tourism activities. This provides additional and useful implications of investment strategies and forecasting performance of tourism activities for policy-makers in EPU and tourism activities. Besides, the co-movement during the recent financial crisis or EPU has been particularly identified, with the results demonstrating that EPU and tourism activities are actually responding significantly more to economic growth fundamentals than to each other. In the light of worldwide attention to economic activities in the BRIC countries, tourism investigation into the connection among the sequence is not only appropriate but possibly long overdue. All these notable differences play a crucial role in improving the quality of conclusions.
The rest of this paper is organized as follows. Section 2 briefly reviews the related literature. Section 3 provides an overview of data collection and wavelet theory. Section 4 presents the empirical results while the conclusions are given in Section 5.
Literature review
Literature which is particularly concerned with the causal relationship between EPU and tourism activities has been growing. It is important for policy-makers to identify the nature of causal relationships between EPU and tourism activities. Tourism is the foremost sector helping government policy-makers cater for these issues by providing foreign exchange that is beneficial for creating the provincial employment opportunities that are essential in managing unemployment and encouraging construction, accommodation, transportation and beverage or food sectors that can bring a rise in tourism activities by providing added value. Moreover, this sector also develops in conjunction with countries by transferring income to developing countries from developed ones. Accordingly, policy-makers can use tourism as a policy tool for falling regional welfare inequalities. Previous studies (e.g. Demir et al., Citation2015; Perles-Ribes et al., Citation2016; Raza et al., Citation2017; Wu et al., Citation2020, Citation2021c, Citation2021d) have found that the relationship between global financial crises or EPU and tourism activities has generally been addressed by two different components in the tourism economic literature.
However, other sources, such as ecological, financial, regional and global crises, have undoubtedly attracted a lot of attention to the most recent global financial crisis or EPU (e.g. Aye et al., Citation2015; Bekiros et al., Citation2015; Chang et al., Citation2015; Demir et al., Citation2015; Ko & Lee, Citation2015; Wu et al., Citation2020, Citation2021b, Citation2021c; Wu & Wu, Citation2021; Zhang et al., Citation2015). These studies reveal the geographic and time asymmetries of the consequences of the crisis. As mentioned above, in the tourism literature it may be seen that efforts have been undertaken over recent decades to identify whether EPU and tourism activities are direct or indirect by employing various linear or non-linear co-intergeneration techniques. Nevertheless, the findings differ widely based on the dataset examined and the method used in this work.
Moreover, the frequency-variation in such relationship has certainly not been identified by the time-domain approaches as well. Nevertheless, as a matter of fact, time- and-frequency varying features in such relationship have important practical implications for government policy-makers. Time-varying co-movement implies that EPU and the diversification benefits of tourism activities evolve with time and thus policy-makers should take it into account while examining the co-movement between EPU and tourism activities. Frequency-varying co-movement suggests that policy-makers with different EPU horizons pay more attention to co-movement at corresponding frequencies in order to establish tourism activities more effectively. The time- and-frequency varying features in the causality can also significantly affect the accuracy of forecasting tourism activities, and hence influence investment benefits of practitioners and regulatory benefits of policy-makers. The review of the aforementioned literature comprehends the existence of a uni-directional causal relationship between global financial crises or EPU and tourism activities in a majority of countries. However, some EPU has a direct, indirect and bi-directional causal relationship with tourism activities. This can reflect the need to further evaluate the causality and the reasons behind the existence of such difference. Accordingly, this work attempts to perform the investigation into the relationship of EPU and tourism activities using the extensive framework of continuous wavelet analysis.
Data collection and wavelet theory
The BRIC data were collected from the World Bank in the World Development Indicators (WDI) (ITR, ITA and RGDP) and the sample was restricted to these countries for which the EPU data were introduced by Baker et al. (Citation2016). The annual data used in this study included the period from 1995 to 2016 for the BRIC countries with the exception of Russia from 2003 to 2016. The sample period was decided purely by data availability on the measure of tourism activities. All variables were used in their natural log form. The data used in this study focused on ITA to evaluate the performance of tourism activities and EPU to measure the uncertainty in economic policies for each country. International tourism involves the number of tourists who travel to a country other than that in which they have their usual residence, but outside their usual environment, for a period less than 12 months and whose main purpose in visiting is other than an activity remunerated from within the country visited. When data on the number of tourists are unavailable, the number of visitors, which includes tourists, same-day visitors, cruise passengers and crew members, is shown instead. Sources and collection methods for arrivals differ across countries. In some cases, data are from border statistics (police, immigration, and the like) and supplemented by border surveys. In other cases, data are from tourism accommodation establishments. For some countries the number of arrivals is limited to arrivals by air and for others to arrivals staying in hotels. Some countries include arrivals of nationals residing abroad while others do not. Caution should thus be used in comparing arrivals across countries. The data on inbound tourists refer to the number of arrivals, not to the number of people traveling. Thus a person who makes several trips to a country during a given period is counted each time as a new arrival. For economic uncertainty, the researchers used the EPU index. This index is constructed from several components. To measure policy-related economic uncertainty, the researchers constructed an index based on newspaper articles regarding policy uncertainty. The index constructs these country-level indices in the same manner as the EPU index based on American newspapers. The researchers drew on two newspapers per country for the indices, namely, Le Monde and Le Figaro for France, Handelsblatt and Frankfurter Allgemeine Zeitung for Germany, Corriere Della Sera and La Repubblica for Italy, El Mundo and El Pais for Spain, and the Times of London and the Financial Times for the UK. In this paper, the researchers used the EPU index to analyze how uncertainty affects tourism. Previous literature (e.g. Demir et al., Citation2017; Demir & Ersan, Citation2018) has investigated the impact of a variety of macro-economic variables on hospitality stock returns. To the best of our knowledge, this is the first study to examine how EPU affects tourism activities. Besides, this work used control variables as other important variables that, if omitted, might cause the estimated coefficients to be biased in which case independence will almost certainly be violated.Footnote1 The EPU and tourism are often considered in the interactions between ITR and RGDP (Wu et al., Citation2021a). Thus, the researchers adopted ITR and RGDP as two control variables. The international tourism receipts are expenditures by international inbound visitors, including payment to national carriers for international transport. These receipts include any other prepayment made for goods or services received in the destination country. They also may include receipts from same-day visitors, except when these are important enough to justify separate classification. For some countries they do not include receipts for passenger transport items. Data are in current U.S. dollars. This study accounts for this using RGDP, which is a measure of GDP that has been adjusted for the price level. In this way, RGDP is a truer measure of output in an economy. plots the EPU index versus the ITA index across the BRIC countries. Besides, many technical advantages of approaches in this paper were discussed in the introduction and an additional feature of the study analysis was attributed to the fact that the researchers could work with the decomposition of time series at different time frequencies using causal analysis, as discussed above. reports the summary statistics for the ITA, ITR, RGDP and EPU for each country.
Table 1. A summary of statistics of EPU, ITA, ITR and RGDP in the BRIC countries.
Continuous wavelet transform
The continuous wavelet transform of a given time series can be defined as a convolution type:
(1)
(1) where the asterisk indicates the complex conjugation and
reveals the complex conjugate function of
, namely the so-called basis wavelet function. As mentioned above, the basis wavelet is derived from a given mother wavelet, which is represented by
in the sense that:
(2)
(2) where
is the wavelet scale that controls how the mother wavelet is stretched, while
is the location parameter that controls where the wavelet is centered. By changing the scale parameter
and translating along the localized time index
, one can construct a picture which shows how the amplitudes of
vary across scales and how such amplitudes change over time (Torrence & Compo, Citation1998).
Three conditions must be fulfilled to be a mother wavelet of the continuous wavelet transform. First of all, it must have a zero mean; namely ensures that it oscillates across positives and negatives and is non-zero locally. Secondly, its square must integrate to unity, namely
, which implies a limitation to an interval of time. Lastly, it must meet admissibility conditions which can be represented as:
(3)
(3) where
is Fourier transform of the mother wavelet
. In wavelet theory and practice, various types of mother wavelets are available for various purposes, such as Daubechies, Mexican hat, Haar, Gauss, Morlet, Shannon, Symlets, Coiflets, Meyer, Spline, Gabor and so on. Among them, the most popularly applicable mother wavelet for feature extraction purpose is the Morlet wavelet introduced by Grossmann and Morlet (Citation1984) and represented as:
(4)
(4) where
ensures its unity energy and
makes it satisfy the admissibility condition of Equation (3). In particular, when the dimensionless frequency
is equal to six, the Morlet wavelet achieves optimal trade-off between time and frequency localization (Grinsted et al., Citation2004). According to Aguiar-Conraria and Soares (Citation2014), the Fourier frequency
is given by
. Therefore, for the best choice of
, the conversion from the wavelet scale
into the Fourier frequency
can be facilitated in the sense that:
(5)
(5) where the wavelet scale is considered as a reciprocal of the Fourier frequency, implying that
decomposes into a joint time-frequency plane where the shorter (longer) wavelet scale corresponds to the higher (lower) frequency. Moreover, since the Morlet wavelet is a complex wavelet, the continuous wavelet transform is able to be divided into the real and imaginary parts. As such, the amplitudes and phases of continuous wavelet transforms can be calculated for further estimations of wavelet power spectrum, wavelet coherence and wavelet phase-difference.
Wavelet coherence and wavelet phase-difference
Wavelet coherence allows a three-dimensional analysis, which simultaneously considers the time and frequency components, as well as the strength of correlation between time series (Loh, Citation2013). Following the approach of Torrence and Webster (Citation1999), wavelet coherence is estimated using the cross-wavelet and auto-wavelet power spectrums in the sense that:
(6)
(6) where wavelet coherence is represented by the above squared type and smoothed by the smoothing operator
.Footnote2 In this way, it gives a quantity between zero and one in a time-frequency window.
According to Bloomfield et al. (Citation2004), the wavelet phase-difference characterizes the phase relationship between and
as follows:
(7)
(7) where
and
are the imaginary and real parts of the smoothed cross-wavelet transform, respectively.
A wavelet phase-difference of zero indicates that the two underlying series move together, whereas a wavelet phase-difference of (
) implies that it moves in the opposite direction. If
, then the series are in phase (positively co-moved) with
leading
. If
, then the series are out of phase (negatively co-moved) with
leading
. If
, then the series are out of phase with
leading
. If
, then the series are in phase with
leading
. Note that the wavelet phase-difference can also be indicative of causality between
and
in both the time and frequency domains. As a consequence, it is significantly superior to the conventional Granger causality test which assumes that a single causal relationship holds for the whole sample period as well as at each frequency (Tiwari et al., Citation2013). For example, if
leads
, then it suggests a causal relationship running from
to
at particular time and frequency.
According to Aguiar-Conraria and Soares (Citation2014), the squared partial wavelet coherency between and
after controlling the series
can be defined as:
(8)
(8) where
and
indicate the wavelet coherencies between
and
as well as
and
, respectively. Accordingly, we can also represent the partial wavelet phase-difference such as:
(9)
(9) where
and
indicate the imaginary and real parts of the complex partial wavelet coherency
, respectively. The complex partial wavelet coherency, as its name implies, is the complex type of
before taking the absolute value.
Empirical results and discussion
As mentioned in the data collection section, partial wavelet coherence together with partial wavelet phase-difference provides reliable indications of correlation and lead-lag relationships between EPU and ITA. As indicated in , this work reclassifies the frequency on the y-axis into one band: 3–8 year frequency bands, corresponding to the short-run, mid-run and long-run relationships between EPU and ITA, respectively. Also, the left sides in display the wavelet coherence and wavelet phase-difference between EPU and ITA. In contrast, on the right side, the researchers have the partial wavelet coherence and wavelet phase-difference after controlling the other two variables (i.e. RGDP and ITR) for the BRIC countries. It is difficult to make sense of the figures on the left side. The regions of high wavelet coherence remain scarce and the wavelet phase-difference is meaningless in these situations. If anything occurs, one can conclude that there is no relationship between EPU and ITA. However, once other variables are controlled, the figures become clear.
Figure 2. The wavelet and partial wavelet coherence (a.1 and b.1) and wavelet phase-difference (a.2, b.2, a.3 and b.3) between EPU and ITA, with ITR and RGDP as control variables. The y-axis refers to the frequencies (measured in years), while the x-axis is referred to as the time period over the period 1995–2016. The black (grey) contour designates 5 (10%) significance level based on the bootstrap method with 5000 replications.
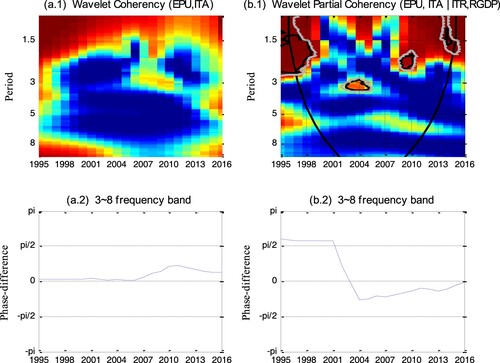
Figure 3. The wavelet and partial wavelet coherence (a.1 and b.1) and wavelet phase-difference (a.2, b.2, a.3 and b.3) between EPU and ITA, with ITR and RGDP as control variables. The y-axis refers to the frequencies (measured in years), while the x-axis is referred to as the time period over the period 1995–2016. The black (grey) contour designates 5 (10%) significance level based on the bootstrap method with 5000 replications.
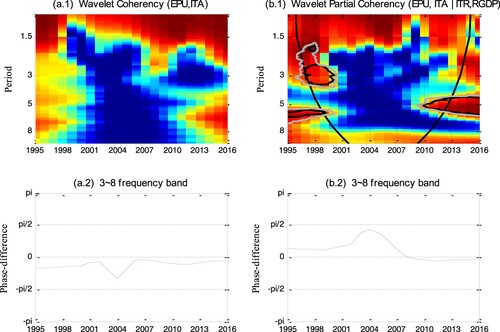
Figure 4. The wavelet and partial wavelet coherence (a.1 and b.1) and wavelet phase-difference (a.2, b.2, a.3 and b.3) between EPU and ITA, with ITR and RGDP as control variables. The y-axis refers to the frequencies (measured in years), while the x-axis is referred to as the time period over the period 1995–2016. The black (grey) contour designates 5 (10%) significance level based on the bootstrap method with 5000 replications.
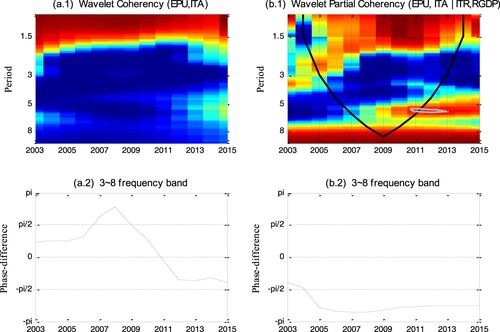
Figure 5. The wavelet and partial wavelet coherence (a.1 and b.1) and wavelet phase-difference (a.2, b.2, a.3 and b.3) between EPU and ITA, with ITR and RGDP as control variables. The y-axis refers to the frequencies (measured in years), while the x-axis is referred to as the time period over the period 1995–2016. The black (grey) contour designates 5 (10%) significance level based on the bootstrap method with 5000 replications.
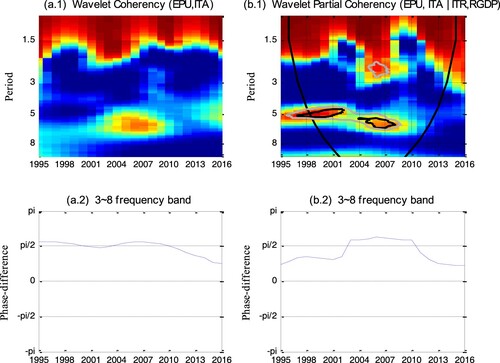
On the right side, as indicated in , significant correlations of partial wavelet coherence between EPU and ITA (at 5% significance level) were found in the short-run period band (1–3 years) during the period 2008–2010 (a period close to the 2008 financial crisis), and from 2013 to 2014 (a period close to the Eurozone budget deficit crisis). The results were found in the mid-run period band (3–5 years) during the period 1997–1999 and 2002–2005 (a period close to the Asian crisis and Iraq War); however, for the time scale of the long-run period band (5–8 years), the researchers failed to find significant correlations between EPU and ITA (at 5% significance level) for Brazil. Also, the study finding indicated that there were significant correlations of partial wavelet coherence between EPU and ITA (at 5% significance level), which were found in the mid-run period band (3–5 years) during the period 1998–2000 (a period close to the Asian crisis) for Russia. For the time scale of the long-run period band (5–8 years) during the period 2010–2013 (a period close to the Euro crisis), significant correlations were found between EPU and ITA (at 5% significance level) for Russia. The different results of partial wavelet coherence between EPU and ITA of the sample are shown in . The researchers failed to find significant correlations between EPU and ITA (at 5% significance level) for India in the short-run, mid-run and long-run period bands. shows the time scale of the long-run period band (5–8 years) during the periods 1998–2002 and 2004–2008 (periods close to the Asian crisis, the Iraq War and the Euro crisis), and the researchers can find significant correlations between EPU and ITA (at 5% significance level) for China.
As shown in , a strong and significant correlation (at 5% significance level) was identified in the 3–8 year frequency band. On the right side the correlation was restricted within a range between zero and ((i.e. positive (in-phase) relationship between EPU and ITA)) during the periods of 2002–2005, 2008–2010 and 2013–2014, implying that ITA was leading correlated with EPU for Brazil. In addition, the partial wavelet phase-differences in for the time scale of the 3–8 year frequency band on the right side was restricted within a range between zero and
((i.e. positive (in-phase) relationships between EPU and ITA)) from 1998 to 2000, implying that EPU was leading correlated with ITA, and that ITA led EPU during 2010–2013 for Russia. However, the partial wavelet phase-difference in showed the restriction within a range between
and
((i.e. negative (anti-phase) relationships between EPU and ITA)) during the period 2010–2011 but did not have significant correlations (at 5% significance level) in India. On the right side, restricted within a range between
and
((i.e. negative (anti-phase) corrections between EPU and ITA)) from the period 2004–2008 implied that ITA was negatively correlated with EPU, and that ITA led EPU in the long-run over the period of the Iraq War and the Eurozone budget deficit crisis.
Conclusions and implications
In this section, the researchers discuss the implications of this empirical work. Before presenting the results of wavelet analysis in this paper, they examine the research question of interest using three-dimensional partial wavelet coherence (Wu & Wu, Citation2019) and time and frequency based Granger-casualty (Breitung & Candelon, Citation2006). Several conclusions can be drawn from this work based on the interpretation of official statistics. The researchers find that EPU plays an important role in explaining the changes in tourism activities of the BRIC countries while controlling the ITR and RGDP indices. As reflected in the use of wavelet models, the effects of EPU macroeconomic variables on tourism activities in tourism markets are mostly significant. This is in line with relevant literature, suggesting explanatory power for these variables (e.g. Chen et al., Citation2010). Wavelet analysis allows the researchers to make a simultaneous assessment of the co-movement and causality between EPU and ITA in both the time and frequency domains. The study results provide robust evidence that the co-movement and causality vary across frequencies and evolve with time. In terms of co-movement from the time domain, EPU and ITA have shown commonly positive and negative co-movement over the past decades. Also, from the frequency domain, EPU and ITA correlate with each other mainly across lower frequency when they are correlated with each other over the long term. Besides, with respect to the causal relationship between EPU and ITA, the researchers find that the causal effects are generally included at lower frequency from a frequency-domain view. In contrast, from a time-domain view, the time-varying features in the long-run causalities imply structural changes in EPU and ITA.
In short, a main limitation of this study is that related variables such as comtrade or economic freedom indices, governance indicators or institutional quality indices as a control variable are not considered and this could be also implemented for further work. Consequently, future studies should consider these variables or imports among others as a control variable while analyzing the effect of EPU on tourism activities. In future research, employing different economic methods such as time-frequency domain, bootstrap rolling-window, multiple wavelet coherence or novel Fourier wavelet (three-dimensional surface) would re-examine the causal relationship between EPU and tourism activities in the BRIC countries.
Disclosure statement
No potential conflict of interest was reported by the author(s).
Notes
1 Given the observational context in which the researchers expect their estimators to prove most valuable, the researchers emphasize that estimated coefficients of control variables, including covariate-moderator interactions, will generally not be interpreted as causal effects absent a strong theoretical justification or causal identification strategy. See Blackwell and Olson (Citation2021) and Keele et al. (Citation2020) for a full discussion of when control variables can be interpreted causally.
2 Without smoothing, the squared wavelet coherence would be always one at any frequency and time. Smoothing is achieved by convolution in time and frequency; see Torrence and Compo (Citation1998) for details.
References
- Aguiar-Conraria, L., & Soares, M. J. (2014). The continuous wavelet transform: Moving beyond uni-and bivariate analysis. Journal of Economic Surveys, 28(2), 344–375. https://doi.org/10.1111/joes.12012
- Andraz, J. M., & Rodrigues, P. M. (2016). Monitoring tourism flows and destination management: Empirical evidence for Portugal. Tourism Management, 56, 1–7. https://doi.org/10.1016/j.tourman.2016.03.019
- Aprilani, T. L. (2021). Community perception on the development of the tourism industry in improving the welfare of the community in Mandalika KEK. Journal of Research in Business, Economics, and Education, 3(3), 1843–1856.
- Aye, G., Gupta, R., Hammoudeh, S., & Kim, W. J. (2015). Forecasting the price of gold using dynamic model averaging. International Review of Financial Analysis, 41, 257–266. https://doi.org/10.1016/j.irfa.2015.03.010
- Baker, S. R., Bloom, N., & Davis, S. J. (2016). Measuring economic policy uncertainty. The Quarterly Journal of Economics, 131(4), 1593–1636. https://doi.org/10.1093/qje/qjw024
- Bekiros, S., Gupta, R., & Paccagnini, A. (2015). Oil price forecastability and economic uncertainty. Economics Letters, 132, 125–128. https://doi.org/10.1016/j.econlet.2015.04.023
- Blackwell, M., & Olson, M. P. (2021). Reducing model misspecification and bias in the estimation of interactions. Political Analysis, 1–20. https://doi.org/10.1017/pan.2021.19
- Bloomfield, D. S., McAteer, R. J., Lites, B. W., Judge, P. G., Mathioudakis, M., & Keenan, F. P. (2004). Wavelet phase coherence analysis: Application to a quiet-sun magnetic element. The Astrophysical Journal, 617(1), 623–632. https://doi.org/10.1086/425300
- Breitung, J., & Candelon, B. (2006). Testing for short- and long-run causality: A frequency-domain approach. Journal of Econometrics, 132(2), 363–378. https://doi.org/10.1016/j.jeconom.2005.02.004
- Calero, C., & Turner, L. W. (2020). Regional economic development and tourism: A literature review to highlight future directions for regional tourism research. Tourism Economics, 26(1), 3–26. https://doi.org/10.1177/1354816619881244
- Chang, T., Chen, W. Y., Gupta, R., & Nguyen, D. K. (2015). Are stock prices related to the political uncertainty index in OECD countries? Evidence from the bootstrap panel causality test. Economic Systems, 39(2), 288–300. https://doi.org/10.1016/j.ecosys.2014.10.005
- Chen, M. H., Liao, C. N., & Huang, S. S. (2010). Effects of shifts in monetary policy on hospitality stock performance. The Service Industries Journal, 30(2), 171–184. https://doi.org/10.1080/02642060802126684
- Demir, E., Alıcı, Z. A., & Chi Keung Lau, M. (2017). Macro explanatory factors of Turkish tourism companies’ stock returns. Asia Pacific Journal of Tourism Research, 22(4), 370–380. https://doi.org/10.1080/10941665.2016.1271814
- Demir, E., & Ersan, O. (2018). The impact of economic policy uncertainty on stock returns of Turkish tourism companies. Current Issues in Tourism, 21(8), 847–855. https://doi.org/10.1080/13683500.2016.1217195
- Demir, E., Lau, C. K. M., & Alıcı, Z. A. (2015). Macro explanatory factors of Borsa Istanbul tourism index. Paper presented at World Finance and Banking Symposium 2015, Hanoi, Vietnam.
- de Souza Neto, V. R., & Marques, O. (2021). Rural tourism fostering welfare through sustainable development: A conceptual approach. In V. R. de Souza Neto (Ed.), Rebuilding and restructuring the tourism industry: Infusion of happiness and quality of life (pp. 38–57). IGI Global.
- Grinsted, A., Moore, J. C., & Jevrejeva, S. (2004). Application of the cross wavelet transform and wavelet coherence to geophysical time series. Nonlinear Processes in Geophysics, 11(5/6), 561–566. https://doi.org/10.5194/npg-11-561-2004
- Grossmann, A., & Morlet, J. (1984). Decomposition of hardy functions into square integral wavelets of constant shape. SIAM Journal on Mathematical Analysis, 15(4), 723–736. https://doi.org/10.1137/0515056
- Keele, L., Stevenson, R. T., & Elwert, F. (2020). The causal interpretation of estimated associations in regression models. Political Science Research and Methods, 8(1), 1–13. https://doi.org/10.1017/psrm.2019.31
- Ko, J. H., & Lee, C. M. (2015). International economic policy uncertainty and stock prices: Wavelet approach. Economics Letters, 134, 118–122. https://doi.org/10.1016/j.econlet.2015.07.012
- Lanzara, G., & Minerva, G. A. (2019). Tourism, amenities, and welfare in an urban setting. Journal of Regional Science, 59(3), 452–479. https://doi.org/10.1111/jors.12440
- Loh, L. (2013). Co-movement of Asia-Pacific with European and US stock market returns: A cross-time-frequency analysis. Research in International Business and Finance, 29, 1–13. https://doi.org/10.1016/j.ribaf.2013.01.001
- Perles-Ribes, J. F., Ramón-Rodríguez, A. B., Rubia-Serrano, A., & Moreno-Izquierdo, L. (2016). Economic crisis and tourism competitiveness in Spain: Permanent effects or transitory shocks? Current Issues in Tourism, 19(12), 1210–1234. https://doi.org/10.1080/13683500.2013.849666
- Raza, S. A., Sharif, A., Wong, W. K., & Karim, M. Z. A. (2017). Tourism development and environmental degradation in the United States: Evidence from wavelet-based analysis. Current Issues in Tourism, 20(16), 1768–1790. https://doi.org/10.1080/13683500.2016.1192587
- Tiwari, A. K., Mutascu, M., & Andries, A. M. (2013). Decomposing time-frequency relationship between producer price and consumer price indices in Romania through wavelet analysis. Economic Modelling, 31, 151–159. https://doi.org/10.1016/j.econmod.2012.11.057
- Torrence, C., & Compo, G. P. (1998). A practical guide to wavelet analysis. Bulletin of the American Meteorological Society, 79(1), 61–78. https://doi.org/10.1175/1520-0477(1998)079<0061:APGTWA>2.0.CO;2
- Torrence, C., & Webster, P. J. (1999). Interdecadal changes in the ENSO–monsoon system. Journal of Climate, 12(8), 2679–2690. https://doi.org/10.1175/1520-0442(1999)012<2679:ICITEM>2.0.CO;2
- UNWTO. (2020). Impact assessment of the COVID 19 outbreak on international tourism. https://www.unwto.org/impact-assessment-of-the-covid-19-outbreak-on-international-tourism
- Wu, T. P., & Wu, H. C. (2019). Causality between European economic policy uncertainty and tourism using wavelet-based approaches. Journal of Travel Research, 58(8), 1347–1356. https://doi.org/10.1177/0047287518803204
- Wu, T. P., & Wu, H. C. (2021). Global economic policy uncertainty and tourism of fragile five countries: Evidence from time and frequency approaches. Journal of Travel Research, 60(5), 1061–1073. https://doi.org/10.1177/0047287520921245
- Wu, T. P., Wu, H. C., Liu, S. B., Wu, C. F., & Wu, Y. Y. (2021a). Causality between global economic policy uncertainty and tourism in fragile five countries: A three-dimensional wavelet approach. Tourism Recreation Research, 1–15. https://doi.org/10.1080/02508281.2020.1870072
- Wu, T. P., & Wu, H. C., Wang, C. M., Chen, F., Wu, S. T., & Liao, S. Y. (2021b). Decomposing a time-frequency relationship between economic policy uncertainty and tourism in the G8 countries through wavelet analysis. Journal of Policy Research in Tourism, Leisure and Events, 13(2), 266–284. https://doi.org/10.1080/19407963.2020.1793767
- Wu, T. P., Wu, H. C., Wang, C. M., & Wu. Y. Y. (2021c). Causality between tourism and housing prices: A wavelet-based approach. Journal of Policy Research in Tourism, Leisure and Events. https://doi.org/10.1080/19407963.2021.1959339
- Wu, T. P., Wu, H. C., Wu, C. F., Liu, C. Y., & Wu, H. (2021d). The influence of economic policy uncertainty on PIIGS tourism: Evidence from three-dimensional wavelet analyses. Tourism Analysis. https://doi.org/10.3727/108354220X15959905699422
- Wu, T. P., & Wu, H. C., Wu, Y. Y., Wu, S. T., & Liao, S. Y. (2020). Causality between economic policy uncertainty and tourism using three-dimensional wavelet-based approaches. Journal of China Tourism Research. https://doi.org/10.1080/19388160.2020.1834482
- Zaroki, S., Ezoji, H., & Sadati Amiri, S. R. (2018). Analysis of the role of tourism on economic welfare using panel data. Journal of Tourism Planning and Development, 7(26), 96–121. https://doi.org/10.22080/JTPD.2018.2043
- Zhang, G., Han, J., Pan, Z., & Huang, H. (2015). Economic policy uncertainty and capital structure choice: Evidence from China. Economic Systems, 39(3), 439–457. https://doi.org/10.1016/j.ecosys.2015.06.003