ABSTRACT
Innovation is at the heart of dental education, dental practice, and optimal oral health care delivery. This paper explores the opportunities and challenges of incorporating artificial intelligence (AI) in dental education. Although the scientific reporting of AI-related research in dental education is limited, the application of AI in academia and dentistry has far-reaching implications in complementing human tasks such as radiological examinations, clinical diagnoses, anatomy training, orthodontics, and prosthodontics education. Moreover, AI has the potential to assess students’ comprehension based on automated facial recognition. Sooner or later AI will change most methods of communication, which will necessitate dental schools to prepare the next generation of students to learn about these powerful tools. Its adoption has great potential for enhancing educational experiences and healthcare as well as paving the way for preparing future dentists to apply AI techniques in clinical practice. The dental community needs to implement recommended guidelines to effectively digitalize dental education with AI technologies.
Continuing Education Credit Available: The practice worksheet is available online in the supplemental material tab for this article. A CDA Continuing Education quiz is online for this article: https://www.cdapresents360.com/learn/catalog/view/20.
Introduction
First coined in the 1950s, artificial intelligence (AI) is “the science and engineering of making intelligent machines.Citation1 ” AI refers to programs and machines that can create defined rules, learn from experience, and accomplish tasks often requiring human intelligence.Citation2 A new generation of algorithms that use large datasets to evaluate and predict has fostered a recent interest in AI, namely machine learning (ML), deep learning (DL), artificial neural networks (ANN), and convolutional neural networks (CNN).Citation3 ML is a branch of AI that uses algorithms to learn and improve from training data and predict outcomes.Citation4 Digital Twins also permeate the dental field, using ML algorithms to process large quantities of data and make predictions ().Citation5 DL is a subfield of ML that consists of highly interconnected structures resembling human brain cells called artificial neural networks (ANN).Citation6 It possesses many simple processing elements called neurons arranged in different layers in the web, namely an input layer, an output layer, and one or more hidden layers.Citation7 CNN is a subset of DL that is used especially for image processing and analysis of radiological datasets.Citation8 Many people are not very familiar with the concept of AI, but despite its widespread lack of familiarity, AI is revolutionizing every walk of education and healthcare. The number of studies involving AI is growing at an exponential rate, but its potential clinical uses are still being explored.Citation9
Table 1. Comparison between different AI models commonly used in dentistry and dental education. The ✓ demonstrates an identified capability for given criteria and the X demonstrates that the given technology lacks that feature. Abbreviations: ML, machine learning; DL, deep learning; ANN, artificial neural network; CNN, convolutional neural network.
The use of AI is evident in handling large volumes of data that would otherwise take a human too long or simply be impossible to process.Citation10 In healthcare, AI is being used in patient monitoring, treatment recommendations, imaging and radiology, and medical record keeping.Citation11 AI has innovated the way we practice medicine, and the COVID-19 pandemic has only accelerated this transformation in dentistry. AI is already being applied to a variety of dental activities, including orthodontics,Citation12,Citation13 dental imaging and radiology,Citation14,Citation15 periodontology,Citation16,Citation17 and cariology.Citation6,Citation18 In endodontics, AI technology is being developed to detect periapical lesions and vertical root fractures via a DL and CNN learning model, respectively.Citation19–21 AI technology is also being used to automate the process of denture design, as the existing computerized design process requires tedious manual inputs and multiple denture fitting procedures.Citation22 By automating the handling of information and data, dentists will be able to focus on other activities.
While AI frameworks in dentistry are not something unheard of, the application of AI to dental education systems remains largely unexplored. Current dental education does not prepare future dentists to incorporate AI technologies into their practice.Citation23 Curriculums for dental education need to extend beyond courses that teach basic and clinical sciences by including material to improve dental students’ ability to practice in an AI-driven environment.Citation24 Increasingly, routine cognitive tasks can be performed by machines, which will require dental schools to refine and improve their education curricula to incorporate AI into their day-to-day operations.Citation2 This paper aims to provide various applications of AI in dental education by answering the following questions: 1. what are the different AI techniques used in education? 2. How is AI currently being utilized in dental education (basic sciences, preclinical sciences, and clinical sciences)? 3. What challenges and limitations are considered with AI pedagogical tools?
Materials and Methods
Inclusion and Exclusion Criteria
The review included both gray literature and peer-reviewed journal articles that were published in English between January 2009 and March 2023. Due to the vast amount of research available on the application of AI in dentistry, the review focused specifically on dental education. This included the applications of AI in teaching theoretical, preclinical, and clinical dental knowledge at the undergraduate and graduate levels. Incomplete studies, letters to editors, conference readings, and posters were excluded from the review.
Search Strategy
The search strategy aimed to identify all relevant studies pertaining to AI in dental education. To achieve this, a thorough search was conducted across four databases (PubMed, Scopus, Web of Science, and Cochrane Library) and gray literature. Databases were searched on March 20, 2023 with the following search terms: “artificial intelligence” AND “dental education,” “artificial intelligence” AND “dental students,” “artificial intelligence” AND “dental educators.” Two reviewers independently assessed article eligibility based on the established inclusion and exclusion criteria, with a third reviewer available to resolve any discrepancies that arose. The references were then transferred to EndNote, where duplicate citations were eliminated.
AI in Education
Machine Learning (ML)
ML is an established concept, but its advancements in dental education curricula contain very limited information regarding its practices. ML is a branch of AI with algorithms that enable systems to learn autonomously and improve from experience without human assistance.Citation25 In this sense, virtual patients with an AI chatbot were created to help dental students develop their diagnostic skills.Citation26 Additional AI pedagogical tools are summarized in .
Deep Learning (DL)
DL is a form of ML that consists of artificial neural networks built on a deep hierarchy of layers, with each layer solving different pieces of a complex problem.Citation27 As a pedagogical tool, DL has been applied in the identification of dental caries and oral lesions from digital radiographs and other digital images.Citation28
Neural Networks
ANN is an AI modeling technique consisting of a highly interconnected structure resembling human brain cells.Citation7 Previous studies show that an ANN can be trained to interpret dental data accurately without requiring prior knowledge. Some examples of its application include: locating the minor apical foramen,Citation29 determining the working length,Citation30 and determining the effects of trace elements on the mechanical properties of dentin.Citation31 CNNs are referred to as deep learning structures and the diagnostic accuracy of these models is approaching levels of human expertise.Citation32 The integration of these models in the day-to-day operations of dental schools would bring dental education to a new era of digitalization.
Digital Twins
With the increasing ability to capture large amounts of evolving data, the concept of Digital Twins enables the dynamic convergence of the physical and digital worlds.Citation33 In simpler terms, Digital Twins technology allows for real-time monitoring and analysis of complex systems, providing valuable insights that can improve our understanding and decision-making.Citation34 In dentistry, it can help in analyzing and predicting complex dental issues, leading to better treatment and outcomes.Citation5
AI in Dental Education
Dentistry has seen major changes over the past decade, but dental education still remains largely traditional.Citation35 The process of becoming a dentist requires extensive training, which is often centered on six domains: knowledge, technical skills, critical thinking, ethics, social responsibility, and interprofessional education/practice.Citation36 Dental students are required to become competent in these areas as defined by the Commission on Dental Accreditation (CODA) and the American Dental Education Association (ADEA).Citation37 A large part of the dental school curriculum involves absorbing a great deal of information and applying it to patient care. It was noted that dental schools face difficulty making curriculum changes that adopt new technologies, which could explain the small amount of time dedicated to familiarizing dental students with novel technologies such as AI. Survey studies clearly demonstrate that dental students currently lack education and knowledge of AI, which is essential to practice in the 21st century.Citation23 However, the implementation of AI seems inevitable since increasingly more papers are being published on how AI should be adopted in dental education.Citation2,Citation9 With the growing use of AI in dentistry, the American Dental Association Standards Committee on Dental Informatics recently released a white paper on the use of artificial and augmented intelligence in dentistry.Citation37 Several successful initiatives for integrating AI in dental training are presented in .
Table 2. Initiatives for AI in dental education.
Traditional face-to-face learning allows teachers to respond more effectively to students since they can observe their facial expressions and immediately modify their teaching methods to meet their needs. Automatic feedback plays a critical role in improving the students’ performance, suggesting that AI technologies can be used as supplemental pedagogical tools to support students’ learning and may also be used as an indicator of students’ interactivity in the classroom. With the overflow of information, it has become a challenge for dental students to gain a comprehensive understanding of the content. Facial expression analysis may be integrated in dental education systems to better assess the students’ comprehension and adjust the course content accordingly, contributing to the widespread introduction of AI tools to assist with enhancing dental pedagogy (). Ekman and Friesen proposed that facial expression may be divided into seven different states: happiness, surprise, sadness, fear, anger, disgust, and natural.Citation38 Similarly, Maqableh et al. used facial expressions to measure students’ interaction with distance learning environments during the COVID-19 pandemic.Citation39 As an example, happiness may be matched with a high level of understanding. DL may be used to enhance the engagement of students in digital learning along with a neural network model to further analyze the students’ facial features and automatically assess their understanding. Despite the adoption of these technologies in medical education, dental education is still in its infancy and could use improvements to its learning curriculum.
Figure 1. (a) Facial landmarks that may be used for image processing. (b). Isolating the eyes from the rest of the face. (c). Database of eyes. (d). Variations of facial expressions. (e). AI workflow in assessing the students’ comprehension with facial expression analysis.
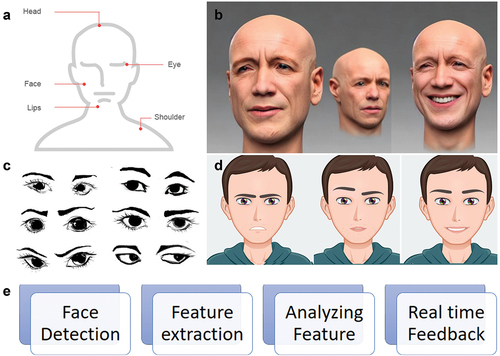
Basic Dental Sciences
During the COVID-19 pandemic, anatomy education experienced a disruption, with remote teaching becoming widely adopted among anatomy disciplines.Citation40,Citation41 Students and educators alike have called for the continued integration of AI into anatomy education as a standard teaching tool, valuing these advanced technologies over the traditional approach.Citation42 AI capabilities are currently integrated into anatomy online learning systems for student monitoring, deployed through three-dimensional anatomical model development and anatomy gamification.Citation42 Using AI, anatomy education and assessments could be delivered more consistently and efficiently, and errors and patterns may be identified that humans might otherwise overlook. Recently, chatbots and conversational agents have been developed to provide “Googleable” answers to anatomy questions.Citation43 Automation may relieve educators from traditional teaching tasks, allowing them to focus on more complex pedagogical tasks such as curriculum design. These recent advancements serve as a guide for how dental educators can integrate AI in clinical anatomy disciplines.
Preclinical Dental Sciences
Diagnosis is the foundation for all dental treatments. Effective and efficient diagnosis is an essential skill that dental students must develop as part of their undergraduate dental education.Citation26 Diagnostic learning may be effectively reenacted through repeated practice of clinical cases followed by faculty feedback, and more recently virtual patients are able to help dental students improve their skills.Citation44,Citation45 By simulating virtual patients with AI, Suárez et al. provided dental students with multiple clinical cases to practice as well as more realistic interactions with the virtual patient, ensuring their training and adaptation to new technological developments.Citation26
Radiological examination is an integral part of patient management in dentistry, as it often assists in the diagnosis of oral pathologies.Citation46 With AI, dental students may be able to better detect pathologies and interpret images more quickly and accurately. Although teaching strategies may vary across different countries, an undergraduate dental student primarily studies radiation physics, radiographic techniques, and digital imaging interpretation in dentomaxillofacial radiology classes, as one example.Citation9 Radiographic images as an element of big data may be gradually introduced within the curriculum, along with the rationale informing AI algorithms and possible implementations in dentistry.Citation47 Students can train on far more radiographs when using an AI system, rather than training on manually selected and annotated radiographs. The successful implementation of AI into dentistry will require extensive training in dental education.
Clinical Dental Sciences
The clinical aspect of dental education is still in the early stages of AI development, making it challenging to define what dental students need to learn. However, orthodontics and radiology have emerged as frontrunners in AI implementation.Citation48,Citation49 The University of Connecticut (UConn) School of Dental Medicine developed an AI based model for diagnosis and treatment planning of orthodontic patients requiring extraction/non-extraction treatment, which could assist dental students with all aspects of orthodontic patient care (e.g., measurements and data interpretation).Citation50 Additionally, the University of Florida College of Dentistry (UFCD) recently partnered with Overjet (AI-powered technology for cavity detection and bone level measurements in dental X-rays) to introduce dental students and residents to AI technology through an education program consisting of a lecture series and hands-on learning opportunities to enhance their diagnostic and case presentation skills.Citation51
Prosthodontics is another diverse and complex dental specialty and one that could benefit from the routine adoption of AI technologies.Citation52 Students at the New York University (NYU) College of Dentistry have been practicing teeth setup placement in dentures with Dentca Academy software, an AI technology for teaching artificial teeth arrangement for a removable prosthesis course.Citation53 With the help of AI, dental students can learn how to create a more accurate denture design and implement a fast and efficient workflow for the production of dentures. Similarly, the Arizona School of Dentistry and Oral Health (ASDOH) also created an AI-assisted removable partial denture design software, which uses specialized algorithms to automatically generate denture designs for any possible missing tooth configuration and clinical scenario. Additionally, the software contains a game component that provides AI-generated clinical scenarios and instant feedback to the students.Citation54
Challenges with AI
Although AI holds many promises, it also has its own set of challenges and limitations that cannot be overlooked. Insufficient time in curricular hours has led to challenges in adjusting new content areas. Studies have also concluded that the primary concern with AI diagnosis and treatment is anonymizing confidential patient information to ensure secure data sharing among different institutions.Citation7 Moreover, dental schools currently lack the faculty expertise required to teach AI in dental content. The lack of expertise in teaching AI to dental students may be resolved by promoting interfaculty collaboration between healthcare experts, engineers, and computer scientists.Citation55 There is also a need to balance the teaching and learning dental students receive from human experts and machines, as AI should be incorporated as a complement to dental educators. Moreover, teaching communication, ethics, and leadership skills in dentistry are likely to grow in importance with the adoption of AI in dental education.Citation2,Citation48,Citation56,Citation57
Consideration should also be given to assessment measures from AI.Citation2,Citation57 To determine the feasibility of integrating AI technology into a course, a pilot implementation can be conducted to determine student and faculty perceptions, as well as pilot outcome assessments. A successful pilot, along with positive perceptions and learning outcomes, could pave the way for the future expansion of an AI initiative at a dental school.Citation2,Citation57 The current curriculum needs input from experts in dentistry and education who will need to work closely with data scientists to ensure that AI tools are integrated correctly.
Conclusion
Thus far, only a limited number of studies have reported findings about AI in dental education. In this paper, we highlight the usefulness of incorporating AI into the day-to-day operations of dental schools and dental education curricula. The current educational trend specifically concentrates on using AI models to help dental students with radiology training, orthodontics, denture design, diagnostic skills, and anatomy education, with several successful initiatives at UConn School of Dental Medicine, UFCD, NYU College of Dentistry, ASDOH, and University of the Pacific Arthur A. Dugoni School of Dentistry. AI, which complements tasks that would otherwise require human intelligence, has great potential for enhancing educational experiences and healthcare delivery, as well as paving the way for preparing future dentists to apply AI techniques in clinical practice.
Supplemental Material
Download MS Word (23.4 KB)Acknowledgments
MAS is a recipient of the DenburTech, New Jersey Health Foundation, and TechAdvance Awards. This publication is dedicated to the memory of Dr. H. Afsar LajevardiCitation58, a legendary pediatrician (1953–2015) who passed. We will never forget Dr. H Afsar Lajevardi’s kindness and support. The views expressed in this paper are those of the authors and do not necessarily reflect the views or policies of the affiliated organizations. The authors hereby announce that they have active cooperation in this scientific study and preparation of the present manuscript. The authors confirm that they have no financial involvement with any commercial company or organization with direct financial interest regarding the materials used in this study.
Supplementary Data
Supplemental data for this article can be accessed online at https://doi.org/10.1080/19424396.2023.2217692.
Additional information
Notes on contributors
Mohammad Ali Saghiri
MA Saghiri was a visiting scholar at the University of Michigan School of Dentistry where he published more than 20 articles under the supervision of the late Prof. Kamal Asgar. He entered academia as an Assistant Professor of Dental Materials at Azad Dental School in 2012 and later was appointed as an Associate Professor and head of IT at Azad Dental School. Dr. Saghiri returned to the US in 2013 and joined Prof. Nader Sheibani’s Lab as an Assistant Scientist at the Department of Ophthalmology and Visual Sciences. After the completion of his postdoctoral studies, he joined Philips Inc. as a Clinical and Biological Scientist and mostly worked on cutting-edge technology for oral hygiene care until 2018. Presently, he is the Director of the Biomaterials Research Center and an Assistant Professor in the Department of Restorative Dentistry at Rutgers School of Dental Medicine. He is also an Adjunct Assistant Professor in the Department of Endodontics at the University of the Pacific School of Dentistry. Dr. Saghiri has authored and co-authored over 120 articles in peer-reviewed dental journals that address scientific, research, educational, and clinical topics. He was awarded three times for his outstanding contributions to research and endodontics at Azad Dental School. Additionally, he received the DenburTech Award, which is only given to clinical scientists who have published more than 100 articles under 40 years of age.
Julia Vakhnovetsky
Julia Vakhnovetsky recently graduated from Rutgers University with a major in Biological Sciences and is currently the Clinical Coordinator at the Biomaterials Research Center at Rutgers School of Dental Medicine. She has authored and co-authored over 10 articles in peer-reviewed dental journals that address scientific, research, educational, and clinical topics as an undergraduate student. She will continue her graduate studies in dental medicine at the University of Michigan School of Dentistry.
Elham Samadi
Elham Samadi is an international dentist who recently joined the Biomaterials Research Lab at Rutgers School of Dental Medicine. Her focus is on the impact of various systemic diseases on the quality of dentin and the longevity of dental treatments, with a particular interest in diabetes mellitus. Additionally, she has taken courses in digital dentistry to enhance her skills and knowledge in this area.
Mahsa Amanabi
Mahsa Amanabi is an international dentist who recently collaborated with the Biomaterials Research Lab at Rutgers School of Dental Medicine. Her current research focuses on using artificial intelligence to detect diabetes through voice analysis, and she is also developing an innovative expandable endodontic sealer.
Steven M. Morgano
Steven M. Morgano is a fellow of the American College of Prosthodontists, a past president of the Massachusetts section, and has been a member of the College since 1979. He has taught at eight different dental schools, including Boston University and Harvard School of Dental Medicine, and at universities in Kuwait and Dubai. He is currently a professor and chairman of the Department of Restorative Dentistry at Rutgers School of Dental Medicine. Dr. Morgano received his DMD degree from Tufts University School of Dental Medicine, completed his General Practice Residency at Philadelphia General Hospital, and received his specialty certificate in prosthodontics from the Hines Veterans Affairs Medical Center in 1979. He practiced as a prosthodontist in the Veterans Affairs for 15 years before devoting himself to academics full-time. Dr. Morgano is also a Diplomate of the American Board of Prosthodontics and has authored and co-authored over 90 articles in numerous dental journals.
References
- Monostori L. Artificial intelligence. In: Laperrière L, and Reinhart G, eds. CIRP Encyclopedia of Production Engineering. Berlin, Heidelberg: Springer; 2014: 47–7.
- Islam NM, Laughter L, Sadid-Zadeh R, et al. Adopting artificial intelligence in dental education: a model for academic leadership and innovation. J Dent Educ. 2022;86(11):1545–1551. doi:10.1002/jdd.13010.
- Kaul V, Enslin S, Gross SA. History of artificial intelligence in medicine. Gastrointest Endosc. 2020;92(4):807–812. doi:10.1016/j.gie.2020.06.040.
- Sadiku MN, Musa SM, Chukwu UC. Artificial intelligence in education. Int J Sci Adv. 2022;2(1). doi:10.51542/ijscia.v2i1.2.
- Saghiri MA, Vakhnovetsky J, Saghiri AM. The future of digital twins in precision dentistry. J Oral Biol Craniofac Res. 2023;13(1):19. doi:10.1016/j.jobcr.2022.10.003.
- Khanagar SB, Al-Ehaideb A, Maganur PC, et al. Developments, application, and performance of artificial intelligence in dentistry–A systematic review. J Dent Sci. 2021;16(1):508–522. doi:10.1016/j.jds.2020.06.019.
- Nguyen TT, Larrivée N, Lee A, Bilaniuk O, Durand R. Use of artificial intelligence in dentistry. Current clinical trends and research advances. J Can Dent Assoc. 2021;7:1488–2159.
- Hwang J-J, Jung Y-H, Cho B-H, Heo M-S. An overview of deep learning in the field of dentistry. Imaging Sci Dent. 2019;49(1):1–7. doi:10.5624/isd.2019.49.1.1.
- Saghiri MA, Vakhnovetsky J, Nadershahi N. Scoping review of artificial intelligence and immersive digital tools in dental education. J Dent Educ. 2022;86(6):736–750. doi:10.1002/jdd.12856.
- El-Hassoun O, Maruscakova L, Valaskova Z, Bucova M, Polak S, Hulin I. Artificial intelligence in service of medicine. Bratislava Med J. 2019;120(3):218–222. doi:10.4149/BLL_2019_028.
- Kundu S. How will artificial intelligence change medical training? Commun Med. 2021;1(1):8. doi:10.1038/s43856-021-00003-5.
- Bichu YM, Hansa I, Bichu AY, Premjani P, Flores-Mir C, Vaid NR. Applications of artificial intelligence and machine learning in orthodontics: a scoping review. Prog Orthod. 2021;22(1):18. doi:10.1186/s40510-021-00361-9.
- Khanagar SB, Al-Ehaideb A, Vishwanathaiah S, et al. Scope and performance of artificial intelligence technology in orthodontic diagnosis, treatment planning, and clinical decision-making-a systematic review. J Dent Sci. 2021;16(1):482–492. doi:10.1016/j.jds.2020.05.022.
- Putra RH, Doi C, Yoda N, Astuti ER, Sasaki K. Current applications and development of artificial intelligence for digital dental radiography. Dentomaxillofac Radiol. 2022;51(1):20210197. doi:10.1259/dmfr.20210197.
- Hung K, Montalvao C, Tanaka R, Kawai T, Bornstein MM. The use and performance of artificial intelligence applications in dental and maxillofacial radiology: a systematic review. Dentomaxillofac Radiol. 2020;49(1):20190107. doi:10.1259/dmfr.20190107.
- Feres M, Louzoun Y, Haber S, Faveri M, Figueiredo LC, Levin L. Support vector machine-based differentiation between aggressive and chronic periodontitis using microbial profiles. Int Dent J. 2018;68(1):39–46. doi:10.1111/idj.12326.
- Carrillo-Perez F, Pecho OE, Morales JC, et al. Applications of artificial intelligence in dentistry: a comprehensive review. J Esthet Restor Dent. 2022;34(1):259–280. doi:10.1111/jerd.12844.
- Prados-Privado M, García Villalón J, Martínez-Martínez CH, Ivorra C, Prados-Frutos JC. Dental caries diagnosis and detection using neural networks: a systematic review. J Clin Med. 2020;9(11):3579. doi:10.3390/jcm9113579.
- Setzer FC, Shi KJ, Zhang Z, et al. Artificial intelligence for the computer-aided detection of periapical lesions in cone-beam computed tomographic images. J Endod. 2020;46(7):987–993. doi:10.1016/j.joen.2020.03.025.
- Kositbowornchai S, Plermkamon S, Tangkosol T. Performance of an artificial neural network for vertical root fracture detection: an ex vivo study. Dent Traumatol. 2013;29(2):151–155. doi:10.1111/j.1600-9657.2012.01148.x.
- Umer F, Habib S. Critical analysis of artificial intelligence in endodontics: a scoping review. J Endod. 2022;48(2):152–160. doi:10.1016/j.joen.2021.11.007.
- Takahashi T, Nozaki K, Gonda T, Ikebe K. A system for designing removable partial dentures using artificial intelligence. Part 1. Classification of partially edentulous arches using a convolutional neural network. J Prosthodont Res. 2021;65(1):115–118. doi:10.2186/jpr.JPOR_2019_354.
- Yüzbaşıoğlu E. Attitudes and perceptions of dental students towards artificial intelligence. J Dent Educ. 2021;85(1):60–68. doi:10.1002/jdd.12385.
- Thulasi MS, Sowjanya B, Sreenivasulu K, Kumar MR. Knowledge attitude and practices of dental students and dental practitioners towards artificial intelligence. Int J Intell Syst Appl Eng. 2022;10:248–253.
- Hastie T, Tibshirani R, Friedman JH, Friedman JH. The Elements of Statistical Learning: Data Mining, Inference, and Prediction. New York: Springer; 2009.
- Suárez A, Adanero A, Díaz-Flores García V, Freire Y, Algar J. Using a virtual patient via an artificial intelligence chatbot to develop dental students’ diagnostic skills. Int J Environ Health Res. 2022;19(14):8735. doi:10.3390/ijerph19148735.
- Jiang F, Jiang Y, Zhi H, et al. Artificial intelligence in healthcare: past, present and future. Stroke Vasc Neurol. 2017;2(4):230–243. doi:10.1136/svn-2017-000101.
- Lee S, Oh SI, Jo J, Kang S, Shin Y, Park J-W. Deep learning for early dental caries detection in bitewing radiographs. Sci Rep. 2021;11(1):16807. doi:10.1038/s41598-021-96368-7.
- Saghiri MA, Asgar K, Boukani KK, et al. A new approach for locating the minor apical foramen using an artificial neural network. Int Endod J. 2012;45(3):257–265. doi:10.1111/j.1365-2591.2011.01970.x.
- Saghiri MA, Garcia-Godoy F, Gutmann JL, Lotfi M, Asgar K. The reliability of artificial neural network in locating minor apical foramen: a cadaver study. J Endod. 2012;38(8):1130–1134. doi:10.1016/j.joen.2012.05.004.
- Saghiri MA, Saghiri AM, Samadi E, Nath D, Vakhnovetsky J, Morgano SM. Neural network approach to evaluate the physical properties of dentin. Odontology. 2022;111(1):68–77. doi:10.1007/s10266-022-00726-4.
- Schwendicke F, Golla T, Dreher M, Krois J. Convolutional neural networks for dental image diagnostics: a scoping review. J Dent. 2019;91:103226. doi:10.1016/j.jdent.2019.103226.
- Wright L, Davidson S. How to tell the difference between a model and a digital twin. Adv Model Simul Eng Sci. 2020;7(1):1–13. doi:10.1186/s40323-020-00147-4.
- Croatti A, Gabellini M, Montagna S, Ricci A. On the integration of agents and digital twins in healthcare. J Med Syst. 2020;44(9):1–8. doi:10.1007/s10916-020-01623-5.
- Breitman LS, Van Doren EJ, and Lee JE. A call-to-action: addressing the technological gap in predoctoral dental education. Harvard Medical Student Review. https://www.hmsreview.org/covid/addressing-the-technological-gap-in-predoctoral-dental-education. 2022. Accessed November 21, 2022.
- Hoskin ER, Johnsen DC, Saksena Y, et al. Dental educators’ perceptions of educational learning domains. J Dent Educ. 2019;83(1):79–87. doi:10.21815/JDE.019.010.
- Faiella RA, Connelly S. Overview of artificial and augmented intelligence uses in dentistry. ADA Standards Committee on Dental Informatics (SCDI) - Artificial Intelligence in Dentistry. https://www.ada.org/resources/practice/dental-standards/standards-committee-on-dental-informatics. 2022. Accessed November 21, 2022.
- Ekman P, Friesen WV. Facial action coding system. Environ Psychol Nonverbal Behav. 1978:1;56–75.
- Maqableh W, Alzyoud FY, Zraqou J. The use of facial expressions in measuring students’ interaction with distance learning environments during the COVID-19 crisis. Visual Inf. 2022;7(1):1–17. doi:10.1016/j.visinf.2022.10.001.
- Pather N, Blyth P, Chapman JA, et al. Forced disruption of anatomy education in Australia and New Zealand: an acute response to the Covid‐19 pandemic. Anat Sci Educ. 2020;13(3):284–300. doi:10.1002/ase.1968.
- Sun L, Tang Y, Zuo W. Coronavirus pushes education online. Nat Mater. 2020;19(6):687. doi:10.1038/s41563-020-0678-8.
- Lazarus MD, Truong M, Douglas P, Selwyn N. Artificial intelligence and clinical anatomical education: promises and perils. Anat Sci Educ. 2022. doi:10.1002/ase.2221.
- Li YS, Lam CSN, See C. Using a machine learning architecture to create an AI-Powered chatbot for anatomy education. Med Sci Educ. 2021;31(6):1729–1730. doi:10.1007/s40670-021-01405-9.
- Schubach F, Goos M, Fabry G, Vach W, Boeker M. Virtual patients in the acquisition of clinical reasoning skills: does presentation mode matter? A quasi-randomized controlled trial. BMC Med Educ. 2017;17(1):1–13. doi:10.1186/s12909-017-1004-2.
- Kononowicz AA, Woodham LA, Edelbring S, et al. Virtual patient simulations in health professions education: systematic review and meta-analysis by the digital health education collaboration. J Med Internet Res. 2019;21(7):e14676. doi:10.2196/14676.
- Ezhov M, Gusarev M, Golitsyna M, et al. Clinically applicable artificial intelligence system for dental diagnosis with CBCT. Sci Rep. 2021;11(1):15006. doi:10.1038/s41598-021-94093-9.
- Buyuk C. Should artificial intelligence integrate with dental education? An assessment through the dentomaxillofacial radiology perspective. J Res Dent. 2021;9(1):6–13. doi:10.19177/jrd.v9e120216-13.
- Thurzo A, Strunga M, Urban R, Surovková J, Afrashtehfar KI. Impact of artificial intelligence on dental education: a review and guide for curriculum update. Educ Sci. 2023;13(2):150. doi:10.3390/educsci13020150.
- Strunga M, Urban R, Surovková J, Thurzo A. Artificial intelligence systems assisting in the assessment of the course and retention of orthodontic treatment. Healthcare. 2023;11(5):683. doi:10.3390/healthcare11050683.
- Murray M Using AI Algorithms to Streamline Orthodontic Care. https://today.uconn.edu/2023/03/using-ai-algorithms-to-streamline-orthodontic-care/. 2023. Accessed March 26, 2023.
- UFCD & Overjet Partner to Develop Dental AI Education Program. https://dental.ufl.edu/2022/12/06/ufcd-and-overjet-partner-to-develop-dental-ai-education-program/. 2022. Accessed March 26, 2023.
- Bernauer SA, Zitzmann NU, Joda T. The use and performance of artificial intelligence in prosthodontics: a systematic review. Sensors (Basel). 2021;21(19):6628. doi:10.3390/s21196628.
- Giugliano T, Chikunov I, Zhivago P, Jahangiri L. A digital method of teaching artificial teeth arrangement. J Dent Educ. 2021;85(S1):904–906. doi:10.1002/jdd.12325.
- Ghorbanifarajzadeh MM, Shazib MA, Riggs S, Dogan J, Dolan TA. Preparing the next generation of clinicians for practice using augmented and artificial intelligence. https://www.aegisdentalnetwork.com/cced/2022/12/preparing-the-next-generation-of-clinicians-for-practice-using-augmented-and-artificial-intelligence. 2022. Accessed November 21, 2022.
- Imran N, Jawaid M. Artificial intelligence in medical education: are we ready for it? Pak J Med Sci. 2020;36(5). doi:10.12669/pjms.36.5.3042.
- Masters K. Artificial intelligence in medical education. Med Teach. 2019;41(9):976–980. doi:10.1080/0142159X.2019.1595557.
- Schwendicke F, Chaurasia A, Wiegand T, et al. Artificial intelligence for oral and dental healthcare: core education curriculum. J Dent. 2023;128:104363. doi:10.1016/j.jdent.2022.104363.
- Saghiri MA, Saghiri AM. In Memoriam: Dr. Hajar Afsar Lajevardi MD, MSc, MS (1955–2015). Iran J Pediatr. 2017;27(1). doi:10.5812/ijp.8093.