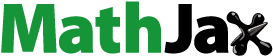
ABSTRACT
Consideration of cumulative dietary risks is a requirement in the European legislation for setting maximum residue levels for pesticides. Current cumulative exposure assessment methodologies strongly rely on representative occurrence data from food monitoring programmes. This study provides a sensitivity analysis, utilising (i) European consumption data expressed as raw agricultural commodity (RAC) equivalents from 23 different countries as published by the European Food Safety Authority and (ii) all maximum residue levels established for pesticides under European Regulation (EC) No 396/2005. Based on two different degrees of conservatism, market baskets consisting of 16 or 41 RACs, respectively, were identified, covering the majority of the total chronic and acute daily exposure. The coverage of the exposure by these market baskets was tested by comparison of cumulative probabilistic exposure assessments for the German population using all food commodities and those using the reduced sets. It was demonstrated that ≥85% of the total chronic exposure is already covered by 16 RACs, while 41 RACs are required to reach a similarly satisfying coverage of the total acute exposure. Results from this study support resource efficient modelling of complex cumulative assessment scenarios and may help to improve the design of food monitoring programmes with respect to a more efficient assessment of potential consumer risks.
Introduction
In regulatory frameworks for pesticides a shift from single substance evaluations towards the assessment of complex mixtures and multiple residues in foods takes place. In the European Union (EU), consideration of “cumulative and synergistic effects, when the methods to assess such effects are available” is a mandatory request when setting maximum residue levels (MRLs) for pesticide residues in foods and feeds under Regulation (EC) 396/2005 (EU Citation2005). However; a harmonised methodology to address the cumulative risk for consumers has not yet been implemented and is still under scientific development. To accelerate this process, large research projects like EuroMix (EuroMix Citation2018) were funded by the European Commission (EC) to improve the knowledge both on mixture toxicity and on methodologies to estimate the cumulative exposure to multiple components.
Mixture toxicity is often related to specific adverse outcome pathways (AOPs) (OECD Citation2017) and therefore strongly linked to the toxicological properties of each component in the mixture. A common approach is the grouping of potentially relevant components into cumulative assessment groups (CAGs), for example based on their capability to induce similar toxicological effects e.g. to target organs (PPR Citation2013) or by their structural relationship like sharing the organophosphorus moiety (EPA Citation2006).
From an exposure assessment point of view, the individual modes of toxicity and the grouping of components are of low relevance for the dietary risk assessment methodologies. In all cases, occurrence data for each component of interest is correlated with information on the amount of food consumed in a specific timeframe. The underlying data and the principles in the exposure models used are mostly unaffected by the size of CAGs or by the AOP they are based on. However; with increasing complexity of models, computational capacity becomes the most limiting factor, both in time and in cost. Currently, most dietary exposure models addressing multiple residues like MCRA (van der Voet et al. Citation2015) or DEEM (EPA Citation2014) are based on probabilistic approaches. The general aim is the generation of an exposure distribution for the population group of interest, from which specific percentiles are selected for decision making (e.g. percentile 99.9) (EPA Citation2000). The data sources used in such models are typically food consumption surveys and results from representative food monitoring programmes. Especially the latter provide a large potential for optimisation of the sampling design to enable a focused and efficient modelling of cumulative dietary risks. Since these programmes have not been optimised in regard to the sensitivity of analysed matrices for the consumer exposure, sampling resources and computation capacities could be invested with maximum benefit.
In Europe, the food monitoring for pesticide residues is organised both on national level (e.g. Germany (Bundesministerium für Ernährung und Landwirtschaft Citation2015)) and in an EU-wide coordinated control programme (European Parliament and the Council of the European Union Citation2018). The underlying scientific concepts for the Germany Food Monitoring (Sieke et al. Citation2008a, Citation2008b) and for the EU Annual Coordinated Control Programme (EFSA Citation2015b) focus on a food basket covering a high percentage of the average daily consumption. Although this concept ensures coverage of important foods eaten frequently, it contains no substance related information on its sensitivity for the dietary risk assessment. The contribution to the total daily dietary exposure from highly consumed food commodities like cereal grains or meat could be much lower than for less consumed foods due to different residues concentrations. Especially for cumulative risk assessments based on probabilistic modelling, the upper percentiles of exposure distributions are driven by a very limited range of food commodities. For example, the cumulative dietary risk to organophosphorus and carbamate insecticides was mainly driven by 6 different raw food commodities each, representing 73% to 89% of the total exposure (Boon et al. Citation2008). In view of the plethora of possible combinations between foods and residue components, consideration of all possible cases would require high efforts and very broad, detailed data but often with only very limited impact on the final result.
In the current work, the sensitivity of raw food commodities for cumulative dietary risk assessments was determined considering the pesticide MRLs established in the European Union. Based on the results, a prioritisation of foods in monitoring programmes is suggested, which allows to focus on key commodities and to identify less relevant commodities which could be omitted from the programmes. Also, in view of future approaches to establish MRLs on the basis of cumulative effects, knowledge of key commodities may help to increase the practicability.
Material and methods
In the first step, a sensitivity analysis was conducted for the contribution of all raw food commodities to the long- (chronic) and short-term (acute) daily exposure. The results were expressed as percentages of the total exposure via all food items and as percentages of the compounds’ health based guidance values (HBGVs), namely the acceptable daily intake (ADI) or the acute reference dose (ARfD), as derived by EFSA in the assessment for the approval of active substance into Annexe I of the European Regulation (EC) 1109/2007 (The European Parliament and the Council of the European Union). The information was obtained from the Pesticide Database of the European Commission (European Commission (COM)). Data management was performed with Microsoft EXCEL while all model calculations were performed using R Software (R Core Team Citation2019).
Consumption data
Consumption data were obtained from the EFSA raw primary commodity (RPC) model (Dujardin and Kirwan Citation2019). The EFSA RPC model is based on 51 dietary surveys from 23 different European countries, representing a total of over 26 million consumption records covering 111 sub-populations. Within the EFSA RPC model, consumption data were transformed into their respective raw primary commodity equivalents according to Annexe I of Regulation (EC) 396/2005, based on recipe data and under consideration of processing effects by using yield factors. The complete daily consumption of a given commodity from all sources was obtained by aggregating all entries to one single raw commodity equivalent per commodity and survey. The RPC model provides statistical parameters (e.g. mean, median and selected percentiles) for the consumption of each raw food item per survey, based either on the total population (including non-consumers) or on consumers only (individuals with a daily portion size >0). In both datasets, consumption is expressed either on a “g per day” or “g per kg bodyweight and day” basis, from which the latter was used for direct comparison with HBGVs.
From the RPC model, the “total population” was considered to calculate the relative contribution of each commodity to the chronic exposure as suggested for predicting the dietary exposure of pesticide residues (Global Environment Monitoring System – Food Contamination Monitoring and Assessment Programme (GEMS/Food) Citation1997). For the acute exposure, consumption data for “consumers only” were considered. This approach reflects the procedure to derive large portions in the “International Estimate of Short-Term Intake” (IESTI) approach (FAO Citation1999; EFSA Citation2015a) which is broadly used in pesticide regulatory frameworks.
Occurrence data and toxicological information
The maximum concentration of pesticides allowed in food commodities according to Regulation (EC) 396/2005 as well as corresponding HBGVs were obtained from the European Commission’s Pesticide Database (date: 31.07.2019) (European Commission (COM) Citation2019). MRLs set at the limit of quantification (LOQ) were considered at the numeric value of the LOQ. Compounds without established MRLs have not been considered in the calculation. Also, MRLs normally address residue components according to the residue definition for enforcement purposes, primarily selected to ensure easy and cost-efficient analysis. For risk assessment purposes, it may be necessary to considered the presence of additional residue components with toxicological relevance. The ratio between these two approach is commonly described as a conversion factor (CF) (EFSA Panel on Plant Protection Products and their Residues Citation2016), which may be used to extrapolate from analytical enforcement results to the residue relevant for the exposure assessment. However, since CF are very limited in their availability, differences in the residue definitions for enforcement purposes and for risk assessment were not taken into account.
For considering the sensitivity of individual foods to the cumulative exposure, ADI and ARfD values are expected to provide a conservative estimate of the risk involved. Both HBGVs are established at the lowest “no observed adverse effect level” (NOAEL) multiplied by a safety factor (typically 100). When this lowest NOAEL is related to the toxicological effect of the CAG, the individual toxicity is directly addressed. When another toxicological effect provides the lowest NOAEL, the sensitivity is even overestimated.
Sensitivity analysis model
In the current work, a model was used to address the sensitivity of each food commodity to the total long- or short-term exposure. The sensitivity was expressed either by the relative contribution to the total daily exposure or by the utilisation of health based guidance values for each commodity.
The relative contribution to the total daily exposure was expressed in percent and calculated as the ratio between the exposure arising from each individual raw food commodity and the total mean exposure from all raw food commodities (EquationEquation 1(1)
(1) ). For each individual commodity, the mean consumption (“mean approach”) and the percentile P97.5 (or the next lower available percentile P90, P75 or mean, if the P97.5 was not available in the RPC model), were used (“high approach”). This calculation was performed for each consumption survey and each pesticidal active substance, providing a large overall distribution of relative contributions from all active substances per food commodity.
In addition, the utilisation of HBGVs by the exposure for individual food commodities (EquationEquation 2(2)
(2) ) was calculated. The mean and high approach for the “total population” was assessed against the ADI, while only the high approach based on “consumers only” was assessed against the ARfD. Compounds with a very limited range of uses could result in high relative contributions of single food commodities to the total daily exposure. However, their relevance becomes low when only a very minor percentage of the ADI value is utilised. On the other hand, single combinations of commodity and MRL may already result in acute exposures close to the ARfD although the contribution to the long-term total exposure is minor. The calculations were performed for each survey and for each pesticidal active substance.
For both approaches, summary statistics were applied to the total distributions of the relative contributions to the exposure and of the utilisations of HBGVs. Based on the reporting frequency in the RPC model, total distributions consisted of 455 up to 56000 individual results. When HBGVs have not been established or were not considered necessary, only the relative contribution to the exposure was calculated. All food commodities either posing a significant contribution to the total daily exposure or utilising a significant percentage of the HBGVs were taken into account to derive an overall market basket.
Reassessment of cumulative risks from pesticide residues in food
Based on the overall market baskets derived by the model, the impact of the reduced number of food commodities on probabilistic cumulative dietary exposure assessments was investigated. In 2018, the cumulative exposure against pesticide residues was estimated for three German sub-populations based on food monitoring data in 115 commodities (Sieke Citation2018). The cumulative assessment groups were obtained from EFSA and referred to chronic and acute effects to the nervous and thyroid system (PPR Citation2013). It should be noted that recently the CAGs for the nervous and thyroid system (Crivellente et al. Citation2019a, Citation2019b) have been updated. However; to estimate the relative coverage of the total diet under realistic conditions, the exact composition of the CAG is not expected to make much difference and therefore adaption to the new CAGs was not performed.
The datasets from the 2018 calculation were directly used for recalculations with the full range of commodities and with the reduced market basket. All scenarios were newly calculated with the MCRA 8.2 software version released in the meantime to identify differences solely based on the food selection, not on changes of the algorithm. Results for the reduced market baskets were expressed in terms of percentage of the exposure of the whole market basket for selected percentiles (percentile 50, 90, 95, 99, 99.9 and 99.99).
Due to the high percentage of non-detects in food monitoring, two different approaches were selected. For each CAG the limit of quantification (LOQ) was either used as such or substituted by zero. The calculations were based on German consumption data for children (0.5–4 years) (Heseker et al. Citation2003) and the general adult population (14–80 years) (Brombach et al. Citation2006; Krems et al. Citation2006; Max Rubner-Institut (MRI) Citation2016), however, for the latter the size of the database did not allow computation of the acute CAG for neurotoxic effects on the motor system (“motor division”).
Results
The results of the model were evaluated by calculating the mean, median, 90th, 95th and 97.5th percentile and the maximum for the contribution of each food commodity and scenario (see supplemental information). Generally, a contribution of ≥10% was considered sufficiently significant to affect the outcomes of total exposure estimations. From the calculated statistical figures, the 95th percentile (P95) was selected to provide a robust upper-bound estimate of the relevance of each food commodity. In addition, the 97.5th (P97.5) was selected to correlate to the degree of conservatism involved in the IESTI concept to address potential cases relevant for acute exposure scenarios.
Total population
For the total population, contributions from individual food commodities to the overall exposure for each compound ranged from close zero up to 60% for the P95 and P97.5 ( and ). In total, 12 food commodities were identified as major contributors to the exposure in the mean approach (descending order: cow milk ≤61.4%, oranges <60.1%, sugar cane ≤32.4%, apples ≤31.9%, sugar beet roots ≤30.0%, wine grapes ≤28.9%, potatoes <23.7%, wheat grain ≤23.0% head lettuce ≤15.3%, tomatoes ≤15.1% and olives for oil production ≤12.3%). Taking also into account the individual chronic HBGVs for each pesticide, only nine food commodities remained at the P95 and P97.5 which were major contributors to the exposure and utilised the ADI by 10% or more (descending order: cow milk ≤77.6%, apples ≤35.9%, sugar beet roots ≤35.6%, oranges ≤31.9%, wheat grain ≤27.2%, sugar cane ≤24.3%, potatoes ≤15.3%, wine grapes ≤14.5% and tomatoes ≤13.3%).
Table 1. Total population chronic contribution (95th percentile of the results, descending).
Table 2. Total population chronic contribution (97.5th percentile of the results, descending).
In the high approach, which calculates the percentage of a large daily portion size versus the mean daily consumption, 18 food commodities were identified with more than 10% contribution () for the P95 and 34 food commodities for the P97.5 (). The RPC model expresses all food consumptions in terms of their raw agricultural commodity equivalents. Highly processed foods or ingredients like white sugar, hard cheese or olive oil show exceptionally high sensitivity in the calculation, since yield rates are low and therefore high amounts of raw commodity are used to prepare the processed food. Of the major contributors to the exposure, 16 food commodities at the P95 and 28 food commodities at the P97.5 also resulted in utilisations of the ADI of 10% or more.
Consumers only
When only consumers of a food are considered, the contribution of portion sizes to the total daily consumption would give unrealistic results, since the frequency of consumption is not considered anymore. The sensitivity of rarely consumed food commodities would be overestimated in comparison to frequently eaten food commodities. Thus, only the sensitivity against HBGVs is calculated to identify food commodities utilising a large percentage of the compound specific ADI or ARfD value. The aim is to address consumers with high consumption frequencies and consumers of single large portions.
For the mean approach, only 9 food commodities were identified (see ) utilising the ADIs by 10% or more (in descending order: nettle ≤37.7%, oranges ≤37.4%, cow milk ≤21.7%, mandarins ≤15.9%, apples ≤14.3%, wheat grain ≤12.4%, quinces ≤11.9%, sugar beet roots ≤10.7% and lamb´s lettuce ≤10.1%). The high approach resulted in a total of 14 food commodities for the P95 and 38 food commodities for the P97.5 with at least 10% ARfD utilisation.
Table 3. Consumer only HGBV contribution (95th and 97.5th percentile of the results, descending).
Consolidated market basket
Based on the analyses for the total population and for consumers only, all food commodities with high sensitivity (≥10%) towards the total daily consumption or to the exposure were identified (). For the P95, 16 food commodities with high sensitivity were identified whereas for the more conservative P97.5 this was a total of 41 food commodities. Normally, all commodities with significant contributions to the total daily diet are also significant commodities in terms of exposure. Exceptions are tea leaves, rape seeds and coffee beans. Although these foods may add more than 10% to the total exposure, related residues are too low to represent a significant utilisation of HBGVs. Also, sugar cane and sugar beet are consumed primarily as highly processed white sugar, for which residue concentrations are normally negligible (Scholz et al. Citation2018). In view of estimating the dietary exposure, these raw commodities can be considered as irrelevant.
Table 4. Consolidated market basket of commodities contributing significantly to the total daily diet and dietary exposure (95th and 97.5th percentile of the results, alphabetic order).
For some commodities separately listed in the EFSA RPC model (e.g. bilberries and strawberries) only one common code exists in the Regulation (EC) No 396/2005 and the respective MRL applies to all sub-commodities. Aggregation to one representative commodity is therefore possible.
Recalculation of previous cumulative exposure assessments
Chronic CAGs
The coverage of the total exposure by the reduced market baskets was generally higher for the upper percentiles of the exposure distribution relevant for decision making like the P99.9. Since lower percentiles represent the background exposure consisting of a plethora of foods with multiple active substances, the outcome is strongly affected by any reduction of the number of commodities. In contrast, the upper percentiles are mainly driven by single food commodity and compound combinations, which remain unchanged as long as sensitive pairings are still considered.
Based on the strongly reduced P95 market basket, the median coverage of the exposure calculated for the total market basket was less than 70% for the 50th percentile, but increased to 85–89% for the 99.9th percentile (). The results were in the same order of magnitude for all 11 CAGs considered for the chronic cumulative exposure. The impact of the LOQ scenario was low. Although absolute exposures differed strongly due to the high amount of “<LOQ” values substituted by zero in the “LOQx0” scenario, the relative change by the reduction of food items was identical for both approaches.
As expected, the percent coverage for the larger P97.5 market basket was higher, ranging from 82–89% for the 50th percentile up to 89–94% for the 99.9th percentile (). The LOQx1 scenario generally resulted in a higher coverage of the total exposure than the LOQx0 scenario. However, the differences were still small with less than 10% deviation between both scenarios.
Acute CAGs
The recalculation of acute scenarios for the CAGs showed a much lower coverage of the total exposure for the reduced P95 market basket (). For the LOQ×0 scenario, the 50th and 90th percentile of the exposure distribution represented less than 60% of the total exposure. Median levels for higher percentiles like the 99.9th percentile were below 70%, suggesting a significant underestimation of the total acute exposure based on the 16 food commodities selected. The LOQ×1 scenario gave more robust results, showing coverages of the total exposure above 80% for all scenarios.
The larger P97.5 market basket also takes into account food commodities, which potentially utilise a significant part of the ARfD (). Consequently, the coverage of the total acute exposure was much higher with >90% for the 90th to 99.9th percentiles. The 50th percentile again resulted in a low coverage of <70% of the total acute exposure.
One deviation was observed for the CAG on acute effects on the autonomic system, which gave less than 60% coverage of the total exposure at higher percentiles like the 99.9th. Detailed investigation of the risk drivers in this group revealed, that the combination of oxamyl in peppers represents a main contributor to the exposure, which is not covered by the P97.5 market basket. In the underlying German monitoring data used for the probabilistic modelling, three samples with oxamyl residues above the LOQ were reported, two of them exceeding the established MRLs by a factor of up to 25. The sample with the highest residue already resulted in an exposure above the ARfD for oxamyl per se (BVL Citation2011). An additional calculation of the P97.5 market basket plus peppers resulted in an increased coverage of the total exposure of 73.7% at the 99.9th percentile (), demonstrating the high sensitivity of the probabilistic cumulative exposure calculation for these two non-MRL compliant samples.
Discussion
The current assessment has demonstrated that for probabilistic cumulative exposure calculations a strongly reduced number of raw food commodities still covers the majority of the total exposure. For chronic scenarios, where single high findings level out for the long-term exposure, already the small P95 market basket consisting of 16 raw food commodities gave a coverage of ≥85%. However, also short-term cumulative effects are relevant and may already be induced by single compound-commodity combinations. Only the larger P97.5 market basket provides a sufficiently high coverage of the total short-term exposure (≥90%) to address this scenario. Under practical conditions, the coverage is likely to be even higher than calculated, since possible extrapolations between closely related commodities, as they are currently accepted in the EU (e.g. wheat to rye grain or tomatoes to aubergines) (EC Citation2017), have not been considered yet.
Consequently, when using exposure based market baskets, resources can be focused to improve the robustness and the sample size of residue data for the key commodities identified. Additional information like processing factors or information on the agricultural use can be implemented in the modelling to improve the outcome. Finally, technical resources for the modelling itself in terms of computing power and time could be redirected to put more emphasis on a broader range of sub-populations or add factors like non-dietary exposure pathways or benchmark-dose modelling of HBGVs.
In the European multi-annual control programme for pesticides (EC Citation2019), currently 30 plant and 6 animal food commodities are considered for the years 2020 to 2022. From the P95 market basket, all commodities except mandarins are already scheduled for analysis in the EU. In comparison to the P97.5 market basket, significant differences exist. Beans with pods, cherries, goat meat, lemons, maize grain, mandarins, pineapples, plums and sunflower seeds gave a significant contribution to the total exposure, but are currently not part of the monitoring programme. Also lamb´s lettuce, quinces and watermelons were identified as relevant, but residues in these commodities can be extrapolated from lettuce, apples/pears or melons, respectively, and do not necessarily require specific sampling. Additionally, bilberries, cranberries, nettle and sorrel, which were identified as relevant contributors to the exposure for single populations reported in the EFSA RPC model, are not covered by the EU coordinated control programme. In contrast, kiwi fruits, dry beans, aubergines, broccoli, sweet peppers, cultivated fungi, head cabbage, bovine liver and chicken eggs are included in the current monitoring programme, although the contribution of these commodities is too low to influence the total long- or short-term cumulative exposure significantly at the upper percentiles.
The European multi-annual control programme for pesticides primarily relies on the Pesticide Monitoring Programme designed by EFSA (EFSA Citation2015b), which ranked food commodities from multiple European Member States according to their proportions in terms of the total daily consumption. The 30 food items identified by this approach represented up to 74% of the daily consumption. However; the contribution of food items to the daily consumption is not necessarily related to their total exposure. Food commodities with large portion sizes but diminutive residues might falsely be identified as relevant while food commodities with small portion sizes but high residues might be omitted. In terms of exposure, the P95 market basket based on 16 raw food commodities already covers a higher percentage of the total exposure (≥85%) than the 30 food commodities suggested by EFSA.
Although the proposed market baskets already represent a refinement in view of consumer exposure assessment, it became obvious that the rate of non-MRL compliant samples may have a high sensitivity to the upper percentiles of the exposure distribution. Frequent findings of residues resulting in potential exceedances of the ARfD per se, like oxamyl in peppers, require consideration both in cumulative exposure modelling and in establishing monitoring programmes.
It needs to be mentioned that the current sampling approaches for pesticide residues in monitoring programmes are a hybrid between the purposes of estimating the consumer exposure and identifying potential MRL violations. For example, the Pesticide Monitoring Programme designed by EFSA or the Pesticide data programme in the USA (USDA Citation2019) both rely on foods which are highly consumed, especially by vulnerable sub-populations like infants and children. The focus is normally based on the raw/fresh commodity, while processed foods are only considered following simple transformations like cooking, freezing or juicing. The selection criteria are solely the contribution to the diet, not the sensitivity towards the exposure of pesticide residues. Another approach is followed for market baskets used in total diet studies (TDS). These kind of studies allow measurement of the average exposure by analysis of representative samples for the majority of foods consumed. Again, the selection of food items follows the principle to cover a high percentage of the daily consumption, but in contrast to food monitoring programmes the food list is amended by specific food items with high contribution to the exposure. For example, in the second Fresh TDS (Sirot et al. Citation2009) 51 additional foods have been identified adding significantly to the exposure. The main advantage of a TDS is the good approximation to the true average dietary exposure, since effects like industrial processing, blending or the composition of complex foods is already taken into account. However, total diet studies also have the drawback that their design is limited to measure mean residues and to estimate the chronic dietary exposure. It does not allow assessments on the acute dietary exposure or on the MRL compliance of individual samples (European Food Safety Authority/FAO/WHO Citation2011).
The newly proposed exposure based market basket keeps the principles of the established monitoring programmes to assess both the dietary exposure and the MRL compliance, but was amended with the relevance of each food towards the exposure. Exposure based market baskets might be helpful when in future cumulative and synergistic effects have to be taken into account for establishing legal limits for pesticide residues as demanded by EU legislation (EU Citation2005). Since consumer protection is the key intention for such a requirement, the range of commodities for which interaction between active substances needs to be regulated could be drastically reduced.
In summary, the exposure based market baskets identified in the current work allow better priorisation of raw food commodities for food monitoring programmes. While the current design of the European food monitoring relies on the contribution of each food to the daily consumption only, a more focused layout becomes possible when potential residue concentrations and HBGVs are taken into account additionally. In parallel, the proposals allow more resource efficient approaches both for cumulative and single compound based exposure assessments and provide a quantitative estimate of the uncertainties arising from a limited set of foods.
Disclaimer
This Paper presents the opinion of the author and not necessarily the regulatory views of the BfR.
Supplemental Material
Download MS Excel (192.7 KB)Acknowledgments
The author would like to thank the MCRA team at the RIVM, especially Dr. J. van Klaveren and G. van Donkersgoed for their support.
Supplementary material
Supplemental data for this article can be on the publisher’s website.
References
- Boon PE, Van der Voet H, Van Raaij MTM, Van Klaveren JD. 2008. Cumulative risk assessment of the exposure to organophosphorus and carbamate insecticides in the Dutch diet. Food Chem Toxicol. 46(9):3090–3098.
- [BVL] Bundesamt für Verbraucherschutz und Lebensmittelsicherheit. 2011. Dombrowski S, editor. Berichte zur Lebensmittelsicherheit 2009. Basel (Germany): Springer. BVL-Reporte; p. 5.5.
- Brombach C, Wagner U, Eisinger-Watzl M, Heyer A. 2006. Die Nationale Verzehrsstudie II - Ziel: aktuelle und belastbare Primärdaten für die Ernährungsberichterstattung des Bundes generieren. Ernährungs-Umschau. 53(1):5.
- [EFSA] European Food Safety Authority: Panel on Plant Protection Products their Residues (PPR). 2013. Scientific opinion on the identification of pesticides to be included in cumulative assessment groups on the basis of their toxicological profile. EFSA J. 11(7):n/a-n/a.
- Bundesministerium für Ernährung und Landwirtschaft. 2015. Allgemeine Verwaltungsvorschrift zur Durchführung des Monitorings von Lebensmitteln, kosmetischen Mitteln und Bedarfsgegenständen für die Jahre 2016 bis 2020. GMBl 2015 Nr. 68:1341.
- [EFSA] European Food Safety Authority: Panel on Plant Protection Products and their Residues. 2016. Guidance on the establishment of the residue definition for dietary risk assessment. EFSA J. 14(12):e04549.
- [EFSA] European Food Safety Authority, Dujardin B, Kirwan L. 2019. The raw primary commodity (RPC) model: strengthening EFSA’s capacity to assess dietary exposure at different levels of the food chain, from raw primary commodities to foods as consumed. EFSA Supporting Publ. 16(1): 1532E.
- [EFSA] European Food Safety Authority, Crivellente F, Hart A, Hernandez-Jerez AF, Hougaard BS, Pedersen R, Terron A, Wolterink G, Mohimont L. 2019a. Establishment of cumulative assessment groups of pesticides for their effects on the nervous system. Efsa J. 17(9): e05800.
- [EFSA] European Food Safety Authority, Crivellente F, Hart A, Hernandez-Jerez AF, Hougaard BS, Pedersen R, Terron A, Wolterink G, Mohimont L. 2019b. Establishment of cumulative assessment groups of pesticides for their effects on the thyroid. Efsa J. 17(9): e05801.
- [EP] European Parliament and the Council of the European Union. Regulation (EC) No 1107/2009 of the European Parliament and of the Council of 21 October 2009 concerning the placing of plant protection products on the market and repealing Council Directives 79/117/EEC and 91/414/EEC, Official Journal of the European Union, L309/1, 14.11.2009, Brussels(Belgium).
- [FAO] Food and Agriculture Organization of the United Nations. 1999. Progress on acute dietary intake estimation – International Estimate of Short Term Intake (IESTI). FAO Plant Protection and Protection Paper. 153(Pesticide residues in food 1999. Report of the Joint Meeting of the FAO Panel of Experts on Pesticide Residues in Food and the Environment and the WHO Core Assessment Group on Pesticide Residues; Rome, Italy.
- [EU] European Union. 2005. Regulation (EC) No 396/2005 of the european parliament and of the council of 23 February 2005 on maximum residue levels of pesticides in or on food and feed of plant and animal origin and amending council directive 91/414/EEC, OJ L 70, 16.3.2005. Brussels (Belgium): European Union (EU); p. 1–16. editor.
- [EFSA] European Food Safety Authority/FAO/WHO. 2011. Towards a harmonised total diet study approach: a guidance document. EFSA Journal. 9:2450. 71.
- [EFSA] European Food Safety Authority. 2015a Sept. EFSA scientific workshop, co-sponsored by FAO and WHO, revisiting the international estimate of short-term Intake (IESTI equations) used to estimate the acute exposure to pesticide residues via food, 8/9. Switzerland: Geneva.
- [EFSA] European Food Safety Authority. 2015b. Pesticide monitoring program: design assessment. EFSA J. 13(2):4005.
- [EC] European Commission. 2017. Guidance document - guidelines on comparability, extrapolation, group, tolerances and data, requirements for setting MRLs. SANCO 7525/VI/95, Rev. 10.3. Brussels (Belgium): European Commission (COM).
- [EP] European Parliament and the Council of the European Union. 2018. Commission Implementing Regulation (EU) 2018/555 of 9 April 2018 concerning a coordinated multiannual control programme of the Union for 2019, 2020 and 2021 to ensure compliance with maximum residue levels of pesticides and to assess the consumer exposure to pesticide residues in and on food of plant and animal origin. Brussels (Belgium): Official Journal of the European Union L 92/6, 10.04.2018.
- EuroMix. 2018. EuroMix; [accessed 2018 Apr 01]. https://www.euromixproject.eu/.
- [EC] European Commission. 2019. Commission Implenting Regulation (EU) 2019/533 of 28 March 2019 concerning a coordinated multiannual control programme of the Union for 2020, 2021 and 2022 to ensure compliance with maximum residue levels of pesticides and to assess the consumer exposure to pesticide residues in and on food of plant and animal origin. Brussels (Belgium): L 88/28 Official Journal of the European Union European Commission (COM).
- [COM] European Commission. EU - pesticides database. European Commission (COM); [ accessed 2019 Aug 01]. http://ec.europa.eu/food/plant/pesticides/eu-pesticides-database.
- Global Environment Monitoring System – Food Contamination Monitoring and Assessment Programme (GEMS/Food). 1997. Guidelines for predicting dietary intake of pesticide residues (revised). Geneva (Switzerland): World Health Organisation. WHO/FSF/FOS/97.7:41.
- Heseker H, Oepping A, Vohmann C. Forthcoming 2003. Nutrition survey to determine the food intake of babies and infants for the estimation of the exposure with pesticide residues - VELS. University Paderborn.
- [MRI] Max Rubner-Institut. German nutrient data base; [ accessed 2016 Mar 01]. http://www.bls.nvs2.de/index.php?id=39&L=1.
- [OECD] Organisation for Economic Co-operation and Development. 2017. Revised guidance document on developing and assessing adverse outcome pathways, series on testing & assessment. Paris. No. 184.
- Krems C, Bauch A, Götz A, Heuer T, Hild A, Möseneder J, Brombach C. 2006. Methoden der Nationalen Verzehrsstudie II. Ernährungs-Umschau. 53(2):6.
- R Core Team. 2019. R: A language and environment for statistical computing. Vienna (Austria): R Foundation for Statistical Computing. http://www.R-project.org/.
- Scholz R, Herrmann M, Kittelmann A, Schledorn M, Donkersgoed G, Graven C, Velde‐Koerts T, Anagnostopoulos C, Bempelou E, Michalski B. 2018. Database of processing techniques and processing factors compatible with the EFSA food classification and description system FoodEx 2 Objective 1: compendium of representative processing techniques investigated in regulatory studies for pesticides. EFSA Supporting Publ. 15(11).
- Sieke C. 2018. Probabilistic cumulative dietary risk assessment of pesticide residues in foods for the German population based on food monitoring data from 2009 to 2014. Food Chem Toxicol. 121:396–403.
- Sieke C, Lindtner O, Banasiak U. 2008a. Pflanzenschutzmittelrückstände, Nationales Monitoring, Abschätzung der Verbraucherexposition: teil 1. Deutsche Lebensmittel-Rundschau. 104(6):271–279. (2008).
- Sieke C, Lindtner O, Banasiak U. 2008b. Pflanzenschutzmittelrückstände, Nationales Monitoring, Abschätzung der Verbraucherexposition: teil 2. Deutsche Lebensmittel-Rundschau. 104(7):336–342. (2008).
- [EPA] U.S. Environmental Protection Agency. 2000. Choosing a percentile of acute dietary exposure as a threshold of regulatory concern. Washington (D.C. (USA)):[EPA] U.S. Environmental Protection Agency.
- [EPA] U.S. Environmental Protection Agency. 2006. Organophosphorus cumulative risk assessment 2006 update.
- [EPA] U.S. Environmental Protection Agency. 2014. Dietary exposure evaluation model user’s guide.
- [USDA] U.S. Department of Agriculture. 2019. Pesticide Data Program Annual Summary Calendar Year 2018. Washington (DC): U.S. Department for Agriculture; p. 214.
- Sirot V, Volatier JL, Calamassi-Tran G, Dubuisson C, Ménard C, Dufour A, Leblanc JC. 2009. Core food of the French food supply: second total diet study. Food Addit Contam. 26(5):623–639.
- van der Voet H, de Boer WJ, Kruisselbrink JW, Goedhart PW, van der Heijden GWAM, Kennedy MC, Boon PE, van Klaveren JD. 2015. The MCRA model for probabilistic single-compound and cumulative risk assessment of pesticides. Food Chem Toxicol. 79:5–12.