Abstract
Chinese cities are undergoing rapid urban sprawl, dramatic landscape change, industrialisation, and ecological damage, which threaten urban sustainable development. The aim of our research was to answer the following question: is it possible to achieve sustainable development through rational ecological protection policies that harmonise future urbanisation, re‐industrialisation, economic development, and sustainable urban land use in these cities? To answer the question, we conducted a simulation of policy impacts on future urban growth and landscape change. We applied a widely used regional assessment and predictive model, SLEUTH, to explore the potential impacts of different ecological protection policy scenarios in the Shenyang-Fushun metropolitan region of China. Future urban growth and landscape changes were projected out to 2050, assuming three different ecological protection policy scenarios: (1) accelerated urban development (AUD), (2) managed urban development (MUD), and (3) limited urban development (LUD). Landscape metrics were used to compare and analyse the impacts of the future scenarios on urban growth and landscape change. Analysis reveals that urban growth and landscape fragmentation increase in all scenarios, but future urban growth area and landscape pattern differ among the scenarios. The AUD scenario will cause excessive urban growth, major land loss, and serious landscape fragmentation. The LUD scenario shows good land resource protection and low landscape fragmentation, but the urban growth rate is unrealistically low, reducing the extent of likely re-industrialisation and economic development. The MUD scenario shows moderate urban growth and landscape fragmentation. It would be the most suitable scenario for the industrial metropolitan area under study if the urban sprawl can be controlled, and it would better harmonise re-industrialisation, ecological health, and land preservation and achieve sustainable development in the area.
1. Introduction
Urbanisation and industrialisation are in conflict with environmental and ecological protection in the metropolitan areas of developing countries. Chinese urbanisation has attracted much attention in recent decades (Ma and Hanten Citation1981, Yeh and Wu Citation1999, Liu et al. Citation2005a, Xu et al. Citation2007, Zhao et al. Citation2008). Rapid urban growth has led to serious environmental and ecological problems such as surface water pollution, biodiversity decrease (Zhao et al. Citation2006), forest decline, and cropland loss (Lin and Ho Citation2003, Liu et al. Citation2005b). Industrialisation in China also resulted in severe environmental and ecological problems (Cole et al. Citation2007, Fang et al. Citation2007, Deng et al. Citation2008). Water quality decline, soil pollution, and air pollution appeared in many Chinese industrial cities (Chan and Yao Citation2008, Zhang et al. Citation2008a). The demand for land resources for rapid urbanisation and industrialisation and their ecological consequences had threatened sustainable metropolitan development (Jantz et al. Citation2003, Deng et al. Citation2008). Environmental and ecological protection is especially important in the industrial metropolitan areas of developing countries, which are undergoing rapid urban growth and industrialisation (or re-industrialisation). So it is critical to make rational ecological protection policies that harmonise urban growth, industrialisation, and urban sustainable development.
Governments and citizens had paid more attention to the ecological impacts of urban growth and re-industrialisation in the industrial cities because of the increased awareness of the need for nature protection. The central government put forward the “Co-ordination of Harmonious Development of Man and Nature” policy to protect the environmental and ecological systems (CPCCC Citation2003). Ecological protection of industrial metropolitan areas is important because China's eco-industrial development is still in its infancy (Fang et al. Citation2007). Improving ecological conditions may be the dominant factor that affects sustainable urban development in China (Wen and Chen Citation2008). At the same time, further industrial development and urban growth seem inevitable in the industrial cities. The State Council of the People's Republic of China put forward the “Northeastern Industry Base Redevelopment” policy to promote industrial innovation and urban economic development in Northeastern industrial cities in 2003 (SCLONIBR Citation2004). National, regional, and local policies were proposed to plan and construct urban and industrial development zones to attract state, domestic, and foreign capital, which continue to influence urban spatial patterns and landscape change (Wu and Barnes Citation2008). In addition to these are regional or local urban planning policies, urban development policies, and environmental protection policies. All these policies will influence urban growth and landscape patterns, which will greatly influence future environmental outcomes (Yang and Lo Citation2003, Civerolo et al. Citation2007). It is important to assess the aggregate influence of multiple policies and choose sound ecological protection polices, which will influence future urban sustainable development.
This research simulated the aggregate impacts of the Co-ordination of Harmonious Development of Man and Nature policy, the Northeastern Industry Base Redevelopment policy, urban planning policies, urban development policies, and other environmental policies on urban growth patterns and landscape changes in the Shenyang-Fushun metropolitan area of China using urban modelling, scenario-based planning, and landscape metrics. The primary objective of this work was to explore rational ecological protection policies that harmonise urban growth, industrialisation, and urban sustainable development in the industrial metropolitan areas of developing countries. Exploration of the outcomes of these policies using computer simulation modelling informed by digital mapping allowed the generation of future scenarios that are of great interest to planners, decision makers, and people who are responsible for urban land use sustainability.
2. Methods
2.1. Study area
The Shenyang-Fushun metropolitan area is located on the transition zone from the branch range of Changbai Mountain to the flood plain of Liao River in Northeast China (), which is one of the fastest urbanising and the most typical and earliest heavy industrial metropolitan areas in China. Fushun is located at the upper reach of the Hunhe River and Shenyang is located at the middle reach of the Hunhe River. Most of the area of Fushun is mountainous, with a large reservoir in the upper reach of the Hunhe River. It is a city famous for its coalfield and petrochemistry. Fushun is the fourth largest city in the Liaoning Province. Shenyang is the capital and the largest city in the Liaoning Province and is the communications, commerce, science, and cultural centre of Northeast China. Most of the area of Shenyang is plain. Shenyang has a long industrial history: it has been a heavy-industry city since the 1910s, based on manufacturing (Chen et al. Citation2006). The development of heavy industry in Shenyang and Fushun caused serious environmental pollution and ecological damage (Sun et al. Citation2006). Shenyang had been the world's top 10 polluted cities until 1999.
2.2. SLEUTH urban growth and land use change model
SLEUTH is an initialism formed from the names of the input data layers: Slope, Land cover, Exclusion areas, Urban extents, Transportation, and Hill shade. It is comprised of the urban growth model (UGM) and the optional land cover deltatron model (LCDM) (Clarke et al. Citation1997, USGS Citation2001). The UGM simulates conversion of non-urban cells to urban cells using a cellular automaton. The LCDM simulates land use/cover change with a second cellular automaton. The UGM can run independently, but the LCDM must run with the UGM (Clarke and Gaydos Citation1998, Dietzel and Clarke Citation2006). SLEUTH is understandable to the average citizen and facilitates scenario design and impact assessments (Jantz et al. Citation2003). Various assumptions about future land use policies and their potential environmental effects can be made to facilitate discussion in the model.
The SLEUTH defines five growth coefficients: diffusion, breed, spread, slope resistance, and road gravity. Each growth coefficient, ranging from 0 to 100, is highly descriptive of a growth trajectory, and has been called “the DNA of a region” (Silva Citation2004, Gazulis and Clarke Citation2006, Clarke et al. Citation2007). The growth coefficients are derived during the calibration process, in which the permutation and combination of five different parameters are tested for their ability to replicate historic growth patterns. Spontaneous growth, new spreading centre growth, edge growth, and road-influenced growth are four main growth rules controlled by the five growth coefficients. The four basic growth rules were described in previous studies (Clarke et al. Citation1997, Clarke and Gaydos Citation1998, USGS Citation2001, Jantz et al. Citation2003, Dietzel and Clarke Citation2006). The self-modification rule is an optional rule, which alters the diffusion, breed, and spread coefficient values to simulate accelerated growth or depressed growth. The self-modification rule makes urban growth a non-linear process (Candau et al. Citation2000, Dietzel and Clarke Citation2006).
The LCDM simulates land use/cover changes induced by urban growth produced in the UGM via a transition matrix computed from two land use maps at different time periods (Clarke Citation2008). It uses an updatable deltatron layer to simulate land use and land cover change. Deltatrons, which are “bringers of change,” track the spatial and temporal effects of land transitions. They do not contain land class values, but act as a reference of where and when a change has occurred. Depending on the age of the deltatron, its locally associated land class may be available for propagating change or may be held in its current state. The LCDM operational procedure was recorded as follows (Candau et al. Citation2000, Solecki and Oliveri Citation2004, Clarke Citation2008):
1. | Initiate change. Newly urbanised cells created in the UGM are assumed to induce potential changes in land cover and, as a result, produce deltatrons in some cells (non-urban, non-water, and non-deltatron) at random. The probability of transition is then computed based on the weighted average slopes for each land class type, the historic land class changes, and the slope of the current cell. If a transition does occur, a new deltatron is created. | ||||
2. | Create change cluster. This is an aggregation process (growth) of newly created deltatrons and their associated land cover transitions. Neighbouring cells of the new deltatrons are randomly selected and tested by the weighted slope and past transition probabilities for transition. The cells can only change to the same land class the associated deltatron has, or should remain unchanged. The newly transitioned cell now acts as the land cover change aggregation centre again. The growth extent of each new deltatron was controlled by initial condition and cluster size in LCDM. | ||||
3. | Propagate change. All non-deltatron cells which are neighbours to at least two deltatron cells that were created in the previous year are tested against the same weighted probability of transition to either remain unchanged or change to the same land cover type as a neighbouring deltatron's land cover type. This step enforces spatial autocorrelation among the land use changes. | ||||
4. | Age of the deltatrons. All deltatrons are aged to the next time step. The number of cycles a deltatron may live is defined by the initial condition. If the deltatrons exceed a user-set age, they “die” and can potentially become new deltatrons in the next growth cycle. |
SLEUTH model implementation consists of four major stages: (1) preparation of the input data, (2) model calibration, (3) simulation of future urban growth and landscape changes, and (4) analysis and use of the model outputs. Within the basic SLEUTH programme, users can also define several additional growth parameters according to the needs of a particular study, for example, the excluded layer, roads layer, urban extent layer, critical slope, critical_high, critical_low, boom, bust, and random seeds (USGS Citation2001).
SLEUTH enables users to define future urban growth and landscape changes as a projection of the past, design future alternative urban growth and land management scenarios (Clarke et al. Citation1997, Jantz et al. Citation2003, Xi et al. Citation2008), and estimate the effects of increased urbanisation on the local environment (Civerolo et al. Citation2007).
2.3. Model input data
We derived five periods of urban extent and two periods of land use/cover from Landsat TM images. Land use/cover is classified into seven types: urban, agricultural, forest, water (including wetland), rural (settlement), mine, and barren. The road layers, including highways, railways, national roads, provincial roads, and county roads, were digitised from the transportation maps of Liaoning Province (1:550,000) and modified with Landsat TM images of the corresponding year. The slope and hill shade layers were created from a digitised topographical map (1:100,000). The excluded layer consisted of natural water bodies, nature reserves, national parks, and scenic areas, and areas that are not allowed for urban development (). The excluded layer defines the probability of protecting different land use types and is a primary tool for policy simulation, planning, and decision making; it can be called a policy layer to some extent. For the calibration phase, the excluded layer consisted of natural water, which was 100% excluded from urbanisation, as well as national parks and scenic areas, which were 60% excluded from urbanisation. For the prediction phase, all excluded areas in the calibration phase were preserved and assigned a value of 100. Policy, politics, environment, planning, and management factors were spatialised and parameterised into the excluded layers, which come with a range of values (0–100) indicating the probabilities of exclusion. In addition, we set buffer zones along rivers and around drinking water lakes, and gave 20–80% probabilities of exclusion (Jantz et al. Citation2003). We gave the urban, industry, and dense development zones lower probabilities of exclusion. All input layers were rasterised at a 60 m resolution using the nearest-neighbour sampling method to the spatial extent of the study area except the roads layers, which were rasterised directly from the vector roads data.
Table 1. Six themes of input data layer and accuracy in the SLEUTH model
2.4. SLEUTH model calibration and validation
The calibration of SLEUTH is the most important phase for the capture of urban growth characteristics and for the success of model forecasting (Xi Citation2009). The “brute force” calibration method was used in the study (Silva and Clarke Citation2002). The calibration approach explores the local urban growth and landscape changes through synthesising physical, economic, political, policy, planning, and management factors that influence the spatiotemporal dynamics of urban land use. SLEUTH uses historical data sources for calibration. It needs at least four different periods of urban extents, two periods of transportation, a slope layer, an excluded layer, a hill shade, and two different periods of land cover if land use change is to be simulated. In this analysis, five urban extents, five transportation extents, and two land cover maps were used. Other single layers included slope, hill shade, and exclusion areas, as discussed above.
Kappa index is an effective tool for map comparison and consists of standard Kappa, location Kappa, and quantity Kappa (Stehman Citation1999, Pontius Citation2002). It can reveal the accuracy of the simulated map with respect to the observed map, from quantity and location (of urban grid) when they were used in model validation. If Kappa = 1, the simulated map has perfect coherence to the observed map; if Kappa > 0.75, the simulated map has high coherence to the observed map; if 0.40 ≤ Kappa ≤ 0.75, the simulated map has low coherence to the observed map; and if Kappa < 0.40, the simulated map has poor coherence to the observed map (Xi Citation2009). The urban extent and the land use map in 1997 were used as input seed layers. The simulated urban extent and land use maps of 2004 were obtained from running the SLEUTH model using growth coefficients derived from calibration. Kappa index is calculated using the simulated map and the observed map of 2004.
2.5. Landscape metrics
To quantify and examine spatiotemporal urban growth and landscape changes, five landscape metrics () were chosen on the basis of published research (Sui and Zeng Citation2001, Herold et al. Citation2003, Dietzel et al. Citation2005, Kong and Nakagoshi Citation2006, Xie et al. Citation2006, Lin et al. Citation2007). These were number of patches (NP), class area (CA), percentage of landscape (PLAND), largest patch indexes (LPI), and landscape shape index (LSI). These metrics describe urban growth area and pattern, landscape change, and ecological impacts. The SLEUTH simulation maps were input to the Fragstats software (McGarigal and Holmes Citation2002) to calculate the landscape metrics.
Table 2. Landscape metrics used in the study
2.6. Scenario design and simulation
Scenarios have been in the planner's and manager's toolkit as a means of representing the future for several decades. The dual functions of scenarios are bridging, which involves exchange of information between modellers and scenario users, and stretching, which involves broadening people's perspectives, contributing to a more effective decision-making process (Xiang and Clarke Citation2003, Nassauer and Corry Citation2004). The design of scenarios accounts for the following aspects: (1) protective level, location, and size of different land use types (e.g. water, reserves, forest, and wetlands are given different exclusion probabilities at certain locations under different scenarios); (2) whether there are urban development zones defined by urban planning projects, industrial development zones, and policy zones that are influenced by special national, regional, and local policies (Li et al. Citation2007). Three scenarios are designed: (1) accelerated urban development (AUD), (2) managed urban development (MUD), and (3) limited urban development (LUD).
The AUD scenario reflects the common rapid urban growth phenomenon in the industrial cities of Northeastern China. There is little or no ecological protection in the urban planning zones, urban development zones (), and industrial development zones such as Shenbei, Shenxi, and Hunnan (). The zones influenced by both urban planning projects and industrial development policies were given 0–5% probability of exclusion. The zones influenced by either urban planning projects or industrial development policies were given 10–20% probability of exclusion. Easements were assigned 10–20% probability of exclusion in development policy zones and 70% probability of exclusion outside of the zones. Forest was given 0% probability of exclusion in development policy zones and 60–70% probability of exclusion outside of development policy zones. Agriculture was assigned a 0% probability of exclusion in development policy zones and 50–60% probability of exclusion otherwise. Streams, lakes, and reserves were given 100% probability of exclusion within their buffer zones throughout the study area. Potential geological disaster zones were ignored. The detailed settings of the excluded layer are shown in . The planned roads and random seeds were added in the respective layers. The critical slope was 21%, critical_high was 1.4, boom was 1.02, critical_low 0.96, and bust was 0.8 (Jantz et al. Citation2003).
Figure 2. Urban development zones and urban planning zones (a) and industrial development zones (b) in Shenyang-Fushun, China.
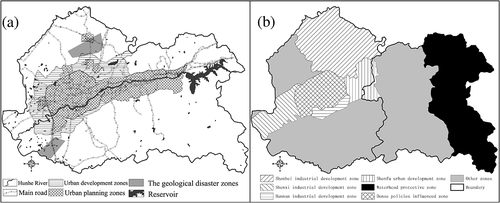
Figure 3. Excluded layers for (a) accelerated urban development (AUD), (b) managed urban development (MUD), and (c) limited urban development (LUD).
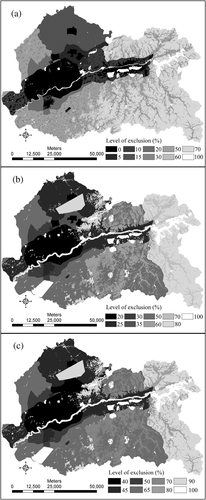
The MUD scenario reflects a stronger commitment towards harmonising re-industrialisation, environment, and resource protection (). In the excluded layer, the zones influenced by both urban planning projects and industrial development policies were given 10–20% probability of exclusion. The zones influenced only by urban planning projects or industrial development policies were given 20–30% probability of exclusion. Easements were assigned 80% probability of exclusion in the development policy areas, and 100% probability of exclusion outside. Forest and agriculture were given 20–35% probability of exclusion in development policy zones, and 50–80% probability of exclusion outside. Streams, lakes, and reserves were given 100% probability of exclusion, and the areas within 120 m buffer zones were given 80% probability of exclusion in the whole study area. The potential geological disaster zones were taken into account and given 80–100% probability of exclusion. There were no new roads and random urban seeds. The critical slope was 21%. The critical_high was 1.3, the boom 1.01, the critical_low 0.97, and the bust 0.9.
The LUD scenario reflects a more strict set of environmental protection policies aimed towards limited industrial development and more natural resource protection. The data settings in the excluded layer were similar to those in the MUD scenario, but protective levels were higher (). The critical slope was 15%, entirely preventing development on steeper slopes. In addition, streams, wetlands, and lakes included larger buffer zones.
3. Results
3.1. Trends in urban growth and landscape changes, 1988–2004
The average urban growth rate was 4.7% from 1988 to 2004. The urban area proportions (PLAND) were 5.45, 5.85, 6.42, 7.82, and 9.55%, respectively, in 1988, 1992, 1997, 2000, and 2004 in the study area. The urban area increased by 20,017.8 ha, rural area increased by 4654.44 ha, water area increased by 2597.04 ha, agriculture area decreased by 22,540.32 ha, forest area decreased by 3827.16 ha, and mines and barren land decreased by 144.72 and 757.08 ha, respectively, from 1997 to 2004. The greatest increase was in the urban class, which converted mainly from agricultural and rural land.
3.2. SLEUTH model calibration and validation
We chose four statistics (compare, pop, lee-sallee, and f‐match) for selecting the “best fit” calibration coefficients (Dietzel and Clarke Citation2006). shows the calibration process and results. The calibration coefficients (“DNA”) of the Shenyang-Fushun metropolitan area are (diffusion = 24, breed = 58, spread = 45, slope resistance = 36, road gravity = 5). All the Kappa indices were more than 0.75 (), which revealed that the simulated map had high coherence to the observed map in year 2004. The SLEUTH model had higher quantity simulation accuracy than location simulation accuracy (Wu et al. Citation2009). It had high simulation accuracy in both the class level and the landscape level in our study area.
Table 3. Calibration routines and results for SLEUTH model of the Shenyang-Fushun metropolitan area with land use data (Jantz et al. 2003)
Table 4. The Kappa index of the simulated map to the observed map in 2004
3.3. Urban growth area and pattern prediction under each scenario, 2005–2050
The three scenarios were forecast with the model from 2005 to 2050, with urbanisation threshold probability more than 60%, using 100 Monte Carlo simulations for each year. illustrates the significant change in the number of urban patches (NP) under the three different scenarios from 2005 to 2050. The simulation NP value was highest under the AUD scenario from 2005 to 2040, and then decreased from 2041 to 2050. A coalescence of urban class patches took place from 2041 to 2050 (Dietzel et al. Citation2005). The NP value increased more slowly from 2005 to 2050 under the LUD scenario. From 2005 to 2050, the urban area increased dramatically under the AUD scenario, increased moderately under the MUD scenario, and increased slowly under the LUD scenario (). The increased urban area under the AUD scenario was 2.1 times that under the MUD scenario, and 8.1 times that under the LUD scenario in 2050. The urban PLAND shows similar tendency with the NP value (). The urban PLAND was 35.1, 22.0, and 12.8%, respectively, in the AUD, MUD, and LUD scenarios in 2050. The LPI of urban class increased slowly under each scenario except for a quick increase appearing in the AUD scenario from 2040 to 2050 () because of urban patch coalescence.
Figure 4. Landscape metrics of policy planning scenarios at urban class level (a) number of patches, (b) class area, (c) percentage of landscape, and (d) largest patch index. Note: *The grey segments show the observed results and the black segments the predictive results. **The same legend as follows.
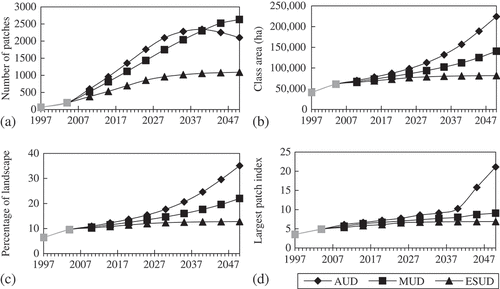
The fastest edge growth and dispersed growth appeared under the AUD scenario, with the main growth areas in industrial development zones, urban planning zones, and policy influenced zones. In the AUD scenario, urban growth occupied easement, riparian zones, and even potential geological disaster zones. Some dispersed urban growth appeared in the headwater protection zone. The urban land of Shenyang and Fushun joined together in 2045 under the AUD scenario. The MUD scenario shows lower edge growth, less dispersed growth patterns, and less urban growth area than the AUD scenario. The MUD scenario better protects the easements, riparian zones, and headwater protective zones and limits urban growth in the potential geological disaster zones. Some dispersed urban growth appeared in the Shenxi and Shenbei industrial development zones. The urban land of Shenyang and Fushun do not join together until 2050 under the MUD scenario. The LUD scenario shows the least urban growth area and the most clustered urban growth. It shows the least urban growth at Shenxi and Shenbei industrial development zones and the Shenfu urban development zone.
3.4. Agricultural land change
In the simulations, other landscape types changed greatly besides the urban land class. The total PLAND of water, mine, and barren land was less than 3.27% from 2004 to 2050, so we will discuss the changes in agriculture, forest, and rural land within the study area.
The NP of agricultural land increased, but the agricultural CA, agricultural PLAND, and the largest patch index (LPI) decreased in all three scenarios. The AUD scenario showed highest NP and agricultural land loss, and the LUD scenario showed lowest NP and agricultural land loss from 2005 to 2050. The NP of agricultural land increased quickly from 2030 to 2050, and agricultural CA, LPI, and PLAND decreased quickly at the same time under the AUD scenario ().
3.5. Forest change
The NP of forest increased but forest CA, forest PLAND, and the LPI decreased in each scenario from 2005 to 2050. The AUD scenario showed higher NP and the largest forest land loss, and the LUD scenario showed lowest NP and forest land loss from 2005 to 2050. The LPI decreased slowly in each scenario from 2005 to 2030 and decreased dramatically from 2031 to 2050 in the AUD and MUD scenarios ().
3.6. Rural change
The NP of rural land increased, but the rural CA and rural PLAND decreased in each scenario from 2005 to 2050. The AUD scenario showed the highest NP and rural land loss, and the LUD scenario showed the lowest NP and rural land loss from 2005 to 2050. The NP increased dramatically and the CA and PLAND decreased dramatically in the AUD scenario from 2015 to 2050. The LPI showed less change only in the LUD scenario ().
3.7. Landscape change
The NP and LSI of landscape increased in each scenario from 2005 to 2050. The AUD scenario showed the highest NP and LSI, the LUD scenario showed the lowest NP and LSI, and the MUD scenario showed in-between from 2005 to 2050 ().
Figure 8. Landscape metrics of policy planning scenarios at landscape level (a) number of patches and (b) landscape shape index.
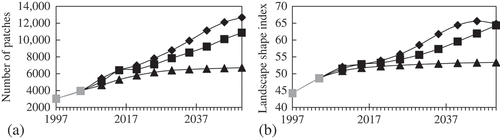
shows the increased urban area and decreased agriculture, forest, rural land, and other land types. The AUD scenario has the largest urban area growth and loss of land resources and the LUD has the lowest urban area growth and loss of land resources, with the MUD scenario in-between.
4. Discussion
Landscape metrics are an effective means of evaluating and comparing simulation results for a particular landscape (Botequilha and Ahern Citation2002) at both the class level and the landscape level. The changes in NP, CA, LPI, LSI, and PLAND show pattern changes and fragmentation at the class level or the landscape level under each scenario (). The decrease of CA, LPI, and PLAND and the increase of NP in agriculture, forest, and rural land show the fragmentation at the class level. The NP and LSI increase in landscape show the fragmentation at the landscape level (Jantz and Goetz Citation2005). The value changes of landscape metrics also show the different environmental impacts of alternative scenario simulation outcomes (Lin et al. Citation2007).
The AUD scenario will be met by extreme urban area growth and rapid loss of other land types. Urban growth appears in reserves, easements, headwater zones, and riparian areas. The largest NP at each class level and landscape level indicates landscape fragmentation at both the class level and the landscape level. The most urban growth appears in the industrial development zones, urban development zones, and density policy zones, where the lowest ecological protections prevail. The industrial development zones were not covered completely by urban land, so the extent of industrial development zones is probably too large, which will result in waste of land resources or greater urban sprawl in those zones. Rapid loss and fragmentation of agricultural land and forest will threaten Chinese food security (Chen Citation2007) and biodiversity (Zhang et al. Citation2008b). The AUD scenario admonishes both governments and businesses.
The MUD scenario reflects about a half of the urban growth area and loss of other land types compared to the AUD scenario. Urban growth is less in the industrial development zones, urban development zones, and density policy zones. Diffused urban growth decreases, and fewer urban patches appear in reserves, easements, headwater zones, and riparian areas. Landscape fragmentation is less than in the AUD scenario at both the class level and the landscape level. Stronger ecological protection can decrease the urban growth rate and protect agriculture, forests, conserves, easements, headwater zones, and riparian areas. The high probability of exclusion in the potential geological disaster zones successfully prevents urban growth in these areas, which is important to avoid damage caused by landslide or earthquake (Li et al. Citation2007).
The LUD scenario shows good protection of the landscape and the lowest amount of urban growth among the three scenarios. The simulated average urban growth rate was 0.7% from 2005 to 2050. Such a low growth rate is unlikely given the population pressure and industrial/economic development (Deng et al. Citation2008). Strict ecological protection policies will significantly decrease the projected urban growth rate. Nowadays, industry redevelopment, urban construction, and economic development are of great importance in the industrial cities of Northeastern China, so this low urban growth rate is unlikely.
Simulation accuracy is important for modelling. The historical urban extent and land use data were used in model validation (). Although we got high Kappa indices for the study area, the urban land Kappa indices were the lowest. SLEUTH showed high precision in predicting non-urban land use change. It performs better in quantity simulation for each land type compared to location simulation (Wu et al. Citation2009). So SLEUTH can be used as a tool in large-scale planning and environmental impact assessment (Jantz et al. Citation2003).
There were some issues in this application. First, we had not considered all the policies of the study area, which may be done through detailed scenario design in subsequent studies. Whether the ecological corridors/network policy scenarios could reduce fragmentation of habitats can be analysed in a follow-up paper. Second, SLEUTH takes all the urban land as one type, but there are different growth rates among industrial land, residential land, and urban land used for other purposes, which were not considered in our study.
5. Conclusion
The study simulated the impacts of different ecological protection policies on future urban growth and landscape change in the industrial metropolitan areas of Northeastern China using the SLEUTH model. Ecological protection policy scenarios show three possible future urban growth patterns and landscape structures. Excessively strict ecological protection is not realistic for the re-industrialisation of cities in Northeastern China because it would not reflect the likely population and economic development pressure. Weak ecological protection will cause extreme urban growth and loss of land resources and other land types. The ecological protection level in the intermediate MUD scenario is suitable for the study area, but only if the diffused urban growth pattern (sprawl) can be effectively controlled to balance re-industrialisation, economic development, and ecological protection. Multilevel governments in China should create discreet development policies and development zones for industry development and urban construction. Some of the strategies for eco-sensitive planning may be useful for wise policy making and management in Chinese heavy-industry cities. Urban density planning is a good strategy for comparing urban growth patterns and identifying factors influencing processes associated with urbanisation (Seik Citation2001). High-density urban development can significantly reduce encroachment on agricultural land and other important environmentally sensitive areas (Yeh and Li Citation2002). Smart growth (Moglen et al. Citation2003, Scott Citation2007) and urban growth boundary (Ding et al. Citation1999, Mubarak Citation2004) strategies are effective in controlling urban growth and reducing environmental pollution. Chinese industrial metropolitan areas need to select and integrate more strategies for eco-sensitive planning based on their success in other countries and establish relatively detailed ecological protection policies for harmonising re-industrialisation, environment, and resource protection in heavy-industry cities.
Notes on contributors
Dr. Fengming Xi is an assistant professor at the Institute of Applied Ecology, Chinese Academy of Sciences. Dr. Xi's research interests include urban sustainable development, landscape ecology, industrial ecology, circular economy simulation system, and low carbon city.
Dr. Hong S. He is a landscape ecologist and a professor at the University of Missouri and a research professor at the Institute of Applied Ecology, Chinese Academy of Sciences. Dr. He's research interests include effects of climate warming, natural and anthropogenic disturbances on forest landscapes, forest landscape modelling, quantifying and predicting urban and rural sprawl.
Dr. Yuanman Hu is a landscape ecologist and research professor at the Institute of Applied Ecology, Chinese Academy of Sciences. His research interests focus on landscape ecology, urban landscape modelling, and forest landscape dynamics.
Dr. Rencang Bu is a research professor at the Institute of Applied Ecology, Chinese Academy of Sciences. Dr. Bu's research interests include effects of climate warming, forest landscape modelling, quantifying and predicting urban sprawl.
Dr. Yu Chang is a landscape ecologist and a professor at the Institute of Applied Ecology, Chinese Academy of Sciences. Dr. Chang's research interests include landscape ecology, disturbances ecology, and quantifying and predicting landscape change.
Dr. Xiaoqing Wu is an assistant professor at the Yantai Institute of Coastal Zone Research, Chinese Academy of Sciences. Dr. Wu's research interests include simulating and assessing urban growth and its environmental impacts, and integrated coastal zone management.
Dr. Miao Liu is an assistant professor at the Institute of Applied Ecology, Chinese Academy of Sciences. Dr. Liu's research interests focus on landscape ecology, 3S applications, ecological planning and watershed ecology.
Dr. Tiemao Shi is a professor at Shenyang Jianzhu University. Dr Shi's research interests include urban and rural planning, landscape ecology, and urban green space design.
Acknowledgements
This research was supported by the National Natural Sciences Foundation of China (grant no. 40801069), the National Science and Technology Supporting Programme of China (2006BAJ10B05-3, 2006BAJ11B04-2), and Knowledge Innovation Programme of the Institute of Applied Ecology, Chinese Academy of Sciences (O6LYQY1001).
References
- Botequilha, L.A., and Ahern, J., 2002. Applying landscape ecological concepts and metrics in sustainable landscape planning, Landscape and urban planning 59 (2002), pp. 65–93.
- http://www.colorado.edu/research/cires/banff/pubpapers/94/ Last accessed 23May2005. , Candau, J., Rasmussen, S., and Clarke, K.C., 2000. A coupled cellular automaton model for land use/land cover dynamics. In: 4th international conference on integrating GIS and environmental modeling (GIS/EM4): problems, prospects and research needs, Banff, Alberta, Canada, 2–8 September..
- Chan, C., and Yao, X., 2008. Air pollution in mega cities in China, Atmospheric environment 42 (2008), pp. 1–42.
- Chen, J., 2007. Rapid urbanization in China: a real challenge to soil protection and food security, Catena 69 (2007), pp. 1–15.
- Chen, Z., et al., 2006. Solar activity, global surface air temperature anomaly and Pacific Decadal Oscillation signals observed in urban outskirts tree ring records from Shenyang, China, Advances in space research 38 (2006), pp. 2272–2284.
- Civerolo, K., et al., 2007. Estimating the effects of increased urbanization on surface meteorology and ozone concentrations in the New York City metropolitan region, Atmospheric environment 41 (2007), pp. 1803–1818.
- Clarke, K.C., 2008. "Mapping and modelling land use change: an application of the SLEUTH model". In: Pettit, C., et al., eds. Landscape analysis and visualisation: spatial models for natural resource management and planning. Berlin: Springer; 2008. pp. 353–366.
- Clarke, K.C., and Gaydos, L.J., 1998. Loose-coupling a cellular automaton model and GIS: long-term urban growth prediction for San Francisco and Washington/Baltimore, International journal of geographical information science 12 (1998), pp. 699–714.
- Clarke, K.C., Hoppen, S., and Gaydos, L., 1997. A self-modifying cellular automaton model of historical urbanization in the San Francisco Bay area, Environment and planning B: planning and design 24 (1997), pp. 247–261.
- Clarke, K.C., et al., 2007. "A decade of SLEUTHing: lessons learned from applications of a cellular automaton land use change model". In: Fisher, P., ed. Classics from IJGIS. Twenty years of the international journal of geographical information systems and science. Boca Raton, FL: Taylor and Francis, CRC; 2007. pp. 413–425, Chap. 16.
- Cole, M.A., Elliott, R.J.R., and Wu, S., 2007. Industrial activity and the environment in China: an industry-level analysis, China economic review 19 (3) (2007), pp. 393–408.
- http://www.china.com.cn/chinese/zhuanti/sljszqh/426675.htm, CPCCC (Communist Party of China Central Committee), 2003. The decisions on improving issues of the socialist market economic system. Communist Party of China Central Committee.In Chinese.
- Deng, X., et al., 2008. Growth, population and industrialization, and urban land expansion of China, Journal of urban economics 63 (2008), pp. 96–115.
- Dietzel, C., and Clarke, K.C., 2006. The effect of disaggregating land use categories in cellular automata during model calibration and forecasting, Computers, environment and urban systems 30 (2006), pp. 78–101.
- Dietzel, C., et al., 2005. Spatio-temporal dynamics in California's Central Valley: empirical links to urban theory, International journal of geographical information science 19 (2005), pp. 175–195.
- Ding, C., Knaap, G.J., and Hopkins, L.D., 1999. Managing urban growth with urban growth boundaries: a theoretical analysis, Journal of urban economics 46 (1999), pp. 53–68.
- Fang, Y., Côté, R.P., and Qin, R., 2007. Industrial sustainability in China: practice and prospects for eco-industrial development, Journal of environmental management 83 (2007), pp. 315–328.
- Gazulis, N., and Clarke, K.C., 2006. Exploring the DNA of our regions: classification of outputs from the SLEUTH model. Lecture notes in computer science: cellular automata. Berlin, Heidelberg: Springer; 2006. pp. 462–471.
- Herold, M., Goldstein, N.C., and Clarke, K.C., 2003. The spatiotemporal form of urban growth: measurement, analysis and modeling, Remote sensing of environment 86 (2003), pp. 286–302.
- Jantz, C.A., Goetz, S.J., and Shelley, M.K., 2003. Using the SLEUTH urban growth model to simulate the impacts of future policy scenarios on urban land use in the Baltimore–Washington metropolitan area, Environment and planning B: planning and design 30 (2003), pp. 251–271.
- Jantz, C.A., and Goetz, S.J., 2005. Analysis of scale dependencies in an urban land-use-change model, International journal of geographical information science 19 (2005), pp. 217–241.
- Kong, F., and Nakagoshi, N., 2006. Spatial-temporal gradient analysis of urban green spaces in Jinan, China, Landscape and urban planning 78 (2006), pp. 147–164.
- Li, Y., et al., 2007. Ecological safety pattern of spatial extension in Shenyang China, Chinese journal of ecology 26 (2007), pp. 875–881, (In Chinese).
- Lin, G.C., and Ho, S.P., 2003. China's land resources and land use changes: insights from the 1996 land survey, Land use policy 20 (2003), pp. 87–107.
- Lin, Y., et al., 2007. Impacts of land use change scenarios on hydrology and land use patterns in the Wu-Tu watershed in Northern Taiwan, Landscape and urban planning 80 (2007), pp. 111–126.
- Liu, J., Zhan, J., and Deng, X., 2005a. The spatio-temporal patterns and driving forces of urban land expansion in china during the economic reform era, AMBIO: a journal of the human environment 34 (6) (2005a), pp. 450–455.
- Liu, X., et al., 2005b. Spatial heterogeneity of the driving forces of cropland change in China, Science in China series D earth sciences 48 (2005b), pp. 2231–2240.
- Ma, L.J., and Hanten, E.W., 1981. Urban development in modern China. Boulder, CO: Westview Press; 1981.
- http://www.umass.edu/landeco/research/fragstats/documents/fragstatsdocuments.html Last accessed 12Sept2008. , McGarigal, K. and Holmes, E.C., 2002. FRAGSTATS (Version 3): FRAGSTATSMetrics. University of Massachusetts-produced program. Available from.
- Moglen, G.E., Gabriel, S.A., and Faria, J.A., 2003. A framework for quantitative smart growth in land development, Journal of the American water resources association (JAWRA) 39 (2003), pp. 947–959.
- Mubarak, F.A., 2004. Urban growth boundary policy and residential suburbanization: Riyadh, Saudi Arabia, Habitat international 28 (2004), pp. 567–591.
- Nassauer, J.I., and Corry, R.C., 2004. Using normative scenarios in landscape ecology, Landscape ecology 19 (2004), pp. 343–356.
- Pontius, R., 2002. Statistical methods to partition effects of quantity and location during comparison of categorical maps at multiple resolutions, Photogrammetric engineering and remote sensing 68 (10) (2002), pp. 1041–1050.
- http://chinaneast.xinhuanet.com/2004-10/28/content_3115778.htm Last accessed 13Mar2008. , SCLONIBR, 2004. Stratagem of northeastern industry base redevelopment. State Council Leading Office of Northeastern Industry Base Redevelopment. (In Chinese)..
- Scott, J., 2007. Smart growth as urban reform: a pragmatic ‘Recoding’ of the new regionalism, Urban studies 44 (1) (2007), pp. 15–35.
- Seik, F.T., 2001. Planning and design of Tampines, an award-winning high-rise, high density township in Singapore, Cities 18 (1) (2001), pp. 33–42.
- Silva, E.A., 2004. The DNA of our regions: artificial intelligence in regional planning, Futures 36 (2004), pp. 1077–1094.
- Silva, E.A., and Clarke, K.C., 2002. Calibration of the SLEUTH urban growth model for Lisbon and Porto, Spain, Computers, environment and urban systems 26 (2002), pp. 525–552.
- Solecki, W., and Oliveri, C., 2004. Downscaling climate change scenarios in an urban land use change model, Journal of environmental management 72 (2004), pp. 105–115.
- Stehman, S.V., 1999. Comparing thematic maps based on map value, International journal of remote sensing 20 (12) (1999), pp. 2347–2366.
- Sui, D.Z., and Zeng, H., 2001. Modeling the dynamics of landscape structure in Asia's emerging desakota regions: a case study of Shenzen, Landscape and urban planning 53 (2001), pp. 37–52.
- Sun, L., Zhang, Y., and Sun, T., 2006. Temporal-spatial distribution and variability of cadmium contamination in soils in Shenyang Zhangshi irrigation area, China, Journal of environmental sciences 18 (6) (2006), pp. 1241–1246.
- http://www.ncgia.ucsb.edu/projects/gig/ Last accessed 28Jul2005. , USGS, 2001. Project gigalopolis: urban and land cover modeling..
- Wen, Z., and Chen, J., 2008. A cost-benefit analysis for the economic growth in China, Ecological economics 65 (2008), pp. 356–366.
- Wu, J., and Barnes, T., 2008. Local planning and global implementation: foreign investment and urban development of Pudong, Shanghai, Habitat international 32 (2008), pp. 364–374.
- Wu, X., et al., 2009. Performance evaluation of the SLEUTH model in the Shenyang metropolitan area of Northeastern China, Environmental modeling & assessment 14 (2009), pp. 221–230.
- Xi, F., 2009. Simulation and prediction of landscape dynamics in the urban agglomeration of Central Liaoning Province, China (2009), pp. 103–111, PhD dissertation. Institute of Applied Ecology, Chinese Academy of Sciences, Shenyang, P.R. China (In Chinese).
- Xi, F., et al., 2008. Simulation and prediction of urban and rural settlement growth and land use change in Yingkou City, Chinese journal of applied ecology 19 (2008), pp. 1529–1536, In Chinese.
- Xiang, W., and Clarke, K.C., 2003. The use of scenarios in land-use planning, Environment and planning B: planning and design 30 (2003), pp. 885–909.
- Xie, Y., et al., 2006. Ecological analysis of an emerging urban landscape pattern-desakota: a case study in Suzhou, China, Landscape ecology 21 (2006), pp. 1297–1309.
- Xu, C., et al., 2007. The spatiotemporal dynamics of rapid urban growth in the Nanjing metropolitan region of China, Landscape ecology 22 (2007), pp. 925–937.
- Yang, X., and Lo, C.P., 2003. Modelling urban growth and landscape changes in the Atlanta metropolitan area, International journal of geographical information science 17 (2003), pp. 463–488.
- Yeh, A.G.O., and Li, X., 2002. A cellular automata model to simulate development density for urban planning, Environment and planning B: planning and design 29 (2002), pp. 431–450.
- Yeh, A.G.O., and Wu, F., 1999. The transformation of the urban planning system in China from a centrally-planned to transitional economy, Progress in planning 51 (1999), pp. 167–252.
- Zhang, H., et al., 2008a. Eco-health evaluation for the Shanghai metropolitan area during the recent industrial transformation (1990–2003), Journal of environmental management 88 (4) (2008a), pp. 1047–1055.
- Zhang, W., et al., 2008b. Impacts of land-use change on mammal diversity in the upper reaches of Minjiang River, China: implications for biodiversity conservation planning, Landscape and urban planning 85 (2008b), pp. 195–204.
- Zhao, S., et al., 2006. Ecological consequences of rapid urban expansion: Shanghai, China, Frontiers in ecology and the environment 4 (7) (2006), pp. 341–346.
- Zhao, J., et al., 2008. Coping with an urbanising world: interdisciplinary research towards sustainability, International journal of sustainable development & world ecology 15 (2008), pp. 284–287.