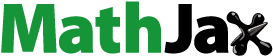
Abstract
The COVID-19 pandemic triggered an unprecedented research effort to develop vaccines and therapeutics. Urgency dictated that development and regulatory assessment were accelerated, while maintaining all standards for quality, safety and efficacy. To speed up evaluation the European Medicines Agency (EMA) implemented “rolling reviews” allowing developers to submit data for assessment as they became available. We discuss the clinical trial designs and the applied statistical approaches in vaccine efficacy trials, focusing on aspects such as multiple testing, interim and updated analyses, and reporting of results for the first four vaccines recommended for approval by the EMA. The fast accrual of COVID-19 cases in the clinical vaccine efficacy trials led to multiple data updates within a short time frame, which had consequences for the evaluation and interpretation of results. Key trial results are discussed in the light of these aspects. Notably, the aspects discussed did not affect the benefit/risk relationship in a meaningful way, which was clearly positive for all four vaccines. Assessment of the development and evaluation of the four vaccine trials during the pandemic has led to a proposal for standardized terminology for trials with multiple analyses and a recommendation to appropriately preplan the timing of primary and updated analyses. For the reporting of updated estimates of vaccine efficacy, we discuss how to best describe the uncertainty around estimates of vaccine efficacy (e.g., via confidence intervals). Finally, we briefly highlight the benefit of a comprehensive discussion on estimands for vaccine efficacy trials.
1 Introduction
Between December 2020 and April 2021, four COVID-19 vaccines were granted conditional marketing authorization by the European Commission, following evaluation by the Committee for Human Medicinal Products (CHMP) of the European Medicines Agency (EMA): two mRNA-based vaccines (Comirnaty developed by BioNTech/Pfizer and Spikevax by Moderna) and two adenovirus vector-based vaccines (Vaxzervria developed by University of Oxford/AstraZeneca and Jcovden developed as a suspension for injection by Janssen Cilag International NV). All approvals followed the provision and review of data from clinical vaccine efficacy trials.
At the design stage, it was uncertain what vaccine efficacy against confirmed COVID-19 disease could be expected. The World Health Organization’s (WHO) target product profile (World Health Organization Citation2020b) aiming at a minimum point estimate of 50% vaccine efficacy on the population level was a starting point for trial designs. Later the WHO released a draft trial synopsis (World Health Organization Citation2020a) detailing that the aim should be to exceed a lower boundary of 30% for the adjusted 95% confidence interval (CI) around the point estimate of vaccine efficacy. In November 2020 the EMA adopted a guiding document (European Medicines Agency, Committee for Human Medicinal Products Citation2020) requiring that the lower bound of the 95% CI should exceed 20% and preferably 30%, as the basis of a positive conclusion on benefit/risk (B/R) from an efficacy perspective. The sample size of the trials was determined by two components:
an overall number of subjects to be recruited, randomized, treated and followed-up during the trial;
the number of events (defined as confirmed cases of COVID-19 disease, that is, symptomatic infection with SARS-CoV-2) accrued.
As the probability to show a statistically significant result is—beside the effect size—determined by the number of events, the sample size of each trial and timing of efficacy analyses were based on the total number of events. The overall number of participants was very large to accrue the required number of events in as a short time frame as possible given the high unmet need. Unavoidably, this resulted in large sample size and short follow up at time of confirmatory analyses.
The definition of COVID-19 disease and severity of disease differed slightly between the four development programmes. The number and type of symptoms required to define a symptomatic disease was different (see Supplementary Table 1), as well as the time from when cases were counted (see ). In addition, each trial had specific assumptions about the relative risk of infection over time between vaccinated and control subjects. The assumptions for the time to maximum protection after vaccination and statistical methods used to estimate vaccine efficacy also differed between vaccines and trials (see Section 4 for details).
Table 1: Overview of the study design features of the four licensed COVID-19 vaccines as of 22/04/2021 based on the European Public assessment reports (EPAR).
At the planning stage, it was anticipated that a (conditional) approval could be granted based on a positive interim analysis of efficacy data, or in the case of Janssen a crossing of sequential boundaries for the two co-primary endpoints. All trials included one or more preplanned interim analyses under Type I error control at a pre-specified targeted number of events (and for Janssen a minimal number of required events combined with a subsequent continuous assessment of updated trial results). After the interim analyses the trials would continue to accrue events in order to increase the precision of the estimate of vaccine efficacy, to evaluate longer term safety and efficacy and to extend the information for important subgroups. Although approaches to the conduct of interim analyses and control of Type I error differed substantially between vaccines, there were, common features that in our view require further consideration with respect to statistical properties and associated regulatory decision making.
Usually, applicants submit all available data on quality, safety and efficacy at the same time when filing the marketing authorization application. To speed up the regulatory assessment during the pandemic the EMA adopted a system of “rolling reviews” (European Medicines Agency Citation2020) allowing submission of data to the EMA as they became available. The reviewers assessed parts of the dossier in a sequential manner. A formal, final submission for a (conditional) marketing authorization application was triggered once it was considered that sufficient data were available. This process allowed for an earlier start of review and interaction with the applicant, while maintaining all standards for quality, safety and efficacy to ensure a robust and scientifically sound regulatory decision.
For all vaccines except for the Janssen vaccine, the initial clinical efficacy data presented in the rolling review were based on positive interim analyses of the pivotal vaccine efficacy trial or trials. During the rolling review, additional safety and efficacy data were submitted to the EMA, leading to updated vaccine efficacy estimates. In line with EMA guidance (European Medicines Agency, Committee for Medicinal Products for Human Use 2007), the additional information was taken into consideration for the final benefit/risk assessment.
The pandemic setting has raised relevant issues in vaccine efficacy trial designs, trial execution, methodology and decision-making which are important to address from a statistical perspective. Due to large observed protective effect sizes, efficacy was clearly demonstrated by all four vaccines compared to the WHO target profile and EMA guidance (European Medicines Agency, Committee for Medicinal Products for Human Use 2019; World Health Organization Citation2020b), and this surpassed potential increased uncertainty or potential bias resulting from the statistical properties of the analyses. However, in similar pandemic situations, where vaccines may not be so overwhelmingly efficacious, these methodologic considerations may severely affect the correctness of regulatory decisions. In addition, this information is also of relevance for stakeholders such as organizations responsible for vaccination strategies, as well as for the general public.
This article is not intended as a guidance document. It aims to address the most important statistical issues identified during regulatory assessment of these four vaccines and consequences for decision making. The article is intended to advance the discussion with all parties involved in drug development and to trigger methodological research to address some of these challenges.
In Section 2, common statistical models and analysis methods for vaccine efficacy are summarized. In Section 3, the separation of confirmatory analyses and benefit/risk assessment is discussed. Terminology for studies with interim analyses is refined to describe and discuss the confirmatory value of the various analyses that were presented and assessed as part of the rolling review. In Section 4, these issues are illustrated using the four recently licensed COVID-19 vaccines as case studies, followed by reflections on the proof of efficacy for the case studies in Section 5. Section 6 discusses issues that arose in the benefit/risk assessment including the consistency of estimates between analyses, the value and reporting of updated analyses, and the consequences of emerging variants. The article concludes with a discussion that seeks to initiate a follow-up exchange on study designs and standards for the future.
2 Statistical Models and Analysis Populations to Test and Estimate Vaccine Efficacy
In vaccine development, the primary endpoint for clinical efficacy studies is usually the prevention of (symptomatic) disease (European Medicines Agency, Committee for Medicinal Products for Human Use 2006). The preventive effect is typically measured by the so-called vaccine efficacy (VE) which is defined as
(1)
(1) where
and
are the incidence rates of unvaccinated and vaccinated subjects. Usually, VE is reported as a percentage. Assuming constant incidence rates over time, simple algebra yields VE
1 - I
and the latter term
, is equivalent to the relative risk (RR) of developing the disease for vaccinated compared to unvaccinated subjects. Consequently, the vaccine efficacy is often estimated using methods to estimate (a) the ratio of probabilities to incur disease during a fixed follow-up period, (b) the ratio of incidences of disease per given time-unit, (c) the hazard ratio (i.e., the instantaneous risk) of incurring the disease. Less common are approaches that estimate the Vaccine Efficacy as one minus the odds ratio of incurring disease, which closely approximates vaccine efficacy as defined above in case of incidence rates close to zero.
For COVID-19 vaccines the generally used primary endpoint is laboratory confirmed COVID-19 disease of any severity (European Medicines Agency, Committee for Human Medicinal Products Citation2020). The following models are described in more detail in sec. 8.2 of Nauta (Citation2020): The simplest statistical model assumes that the number of events accrued within each treatment arm follows a Poisson distribution with fixed rates. With that, the number of events in a particular arm, conditional on the overall number of events follows a binomial distribution, such that statistical hypothesis tests and point estimates of the vaccine efficacy can be derived from standard methods for binomially distributed quantities. Alternatively, vaccine efficacy may be modeled using a number of regression approaches, allowing adjustment for covariates such as randomization stratification factors and relaxation of certain assumptions (e.g., on the constancy of incidence rates). For example, incidence rate ratios can be derived from regression models for count processes (such as negative binomial regression), hazard ratios from time to event models (such as Cox proportional hazards models), and odds ratios from binomial regression models (such as logistic regression). An important aspect of vaccine efficacy trials is that follow-up times usually differ across subjects due to successive enrollment, intercurrent events and the different rate or timing of infections. Whereas time to event models automatically account for variable follow-up times, count models require explicit modeling of the observation time. Consequently, the simple binomial model is typically adjusted for the overall observation time per treatment group. Regression models for count processes include time dependent offsets per subject in the model definition. The resulting estimates can then be interpreted as incidences (events per person time) rather than pure counts.
provides an overview of the most important trial design characteristics together with the applied primary analysis models. As one can see, all three types of modeling approaches were used over the four trials. A more detailed description of the four case studies can be found in the supplementary materials.
In line with current guidance (European Medicines Agency, Committee for Medicinal Products for Human Use 2006; European Medicines Agency, Committee for Human Medicinal Products Citation2018), most vaccine clinical efficacy trials aim to estimate vaccine efficacy in all randomized participants who are seronegative at baseline and who received all vaccinations, and thus use a specific kind of “per protocol” analysis set as the main analysis set (see ). Although this is not in line with the intention-to-treat principle, in this preventive setting, protective effects in the fully vaccinated subjects who were not previously exposed are of primary importance. Moreover, the usual expectation is that only a very small proportion of subjects has to be excluded for other protocol deviations and that the characteristics of such patients should not differ systematically from patients completing the vaccine schedule. Additional criteria on exclusion of subjects with important protocol deviations are usually defined. Endpoints are typically counted only after an initial period after full vaccination, as vaccines cannot be assumed to be fully effective immediately. This definition differed between the trials (see ). In the COVID-19 vaccine trials, additionally, not all patients had reached the status of full vaccination at the time of the positive confirmatory analysis due to the fast accrual of events. These patients were excluded from the analysis set and, hence, the number of patients was higher in the updated analyses.
3 Statistical Questions and Terminology for Regulatory Decision Making
After completion of the rolling review, all four vaccines were granted a conditional marketing authorization. In the European regulatory system, a conditional approval can be issued if (i) the benefit/risk balance of the medicine is positive; (ii) it is likely that the applicant will be able to provide comprehensive data post-authorization; (iii) the medicine fulfills an unmet medical need; and (iv) the benefit of the medicine’s immediate availability to patients is greater than the risk inherent in the fact that additional data are still required (European Commission Citation2006). As for all marketing authorization applications, it thus needs to be assessed whether the benefit/risk balance is positive, considering quality, safety, and efficacy of the product under review.
With respect to assessment of efficacy, two statistical concepts are of specific importance: The first concerns assessment of whether efficacy has been demonstrated. This inferential evaluation is based on confirmatory statistical hypothesis testing. Therefore, the Type I error rate must be controlled at the trial level, (European Medicines Agency, Committee for Proprietary Medicinal Products Citation2002; European Medicines Agency, Committee for Human Medicinal Products Citation2017), that is, the probability of an erroneous conclusion of efficacy needs to be smaller or equal to a predefined constant—typically one-sided 2.5%—for a relevant primary endpoint. The second statistical concept is the appropriate estimation of the magnitude of the treatment or protective effect of interest and associated uncertainty through confidence intervals or other probability measures.
For decision making in the regulatory context, these two statistical concepts are preferably addressed simultaneously and based on the same methods and the same body of data. However, in the present vaccine development setting, this was not the case: confirming efficacy through hypothesis testing and estimating the magnitude of the protective effect (and adverse drug reactions) for benefit/risk assessment and labeling information in the Summary of Product Characteristics (SmPC) were based on different data cutoffs, except for the Janssen case. Vaccine efficacy was confirmed already at an interim analysis in all other cases. Thereafter, vaccine efficacy estimates were regularly updated once additional data accrued, even during the rolling review and the conditional marketing authorization procedure. In each case, the additional data did not raise concerns to revise the efficacy conclusion and were considered of importance to stakeholders (e.g., prescribers, vaccination campaigns, general public). Nevertheless, as these additional analyses were no longer obtained under the preplanned trial design and statistical analysis plan, the statistical properties of these estimates are unclear, for example, potential bias, coverage probability of confidence intervals. To articulate and discuss the statistical issues more precisely, it is helpful to introduce more refined terminology to describe the inferential value of the different analyses.
3.1 Terminology for Trials with Interim or Updated Analyses
The terminology used to describe individual analyses reported in trials with multiple analyses conducted at different timepoints often differs between studies. ICH E9 states that the aim of a confirmatory trial is “to provide firm evidence on efficacy and safety” and that the key hypothesis of interest is “tested when the trial is complete” (ICH Citation1998). Nevertheless, this is not easily generalizable to all study designs. Many examples exist where the study design did not require for completion of long-term follow-up before submitting a marketing authorization application. In studies with time to event endpoints, the concept of “final results” is challenging because not all study participants will experience the event of interest before the end of the study and may not experience the event of interest throughout their lifetime.
For the purpose of benefit/risk assessment, it is typically preferred to infer conclusions on efficacy and estimate the treatment or protective effect with corresponding uncertainties based on the same body of data. However, in group sequential and adaptive clinical trials rejection of the null hypothesis (i.e., statistical conclusion of efficacy) may occur already at an earlier point in time, whereas more precise estimates of the treatment or protective effect are obtained later if the study is continued. In order, for the statistical properties of the resulting decisions (Type I error rate) and estimates (bias, confidence intervals) to hold, it is crucial that the different analyses and timepoints are conducted according to a prospectively defined plan.
At the planning stage this confirmatory strategy is specified and may consist of one or more statistical hypotheses relating to one or more endpoints, sub-groups, or study arms. In addition, the confirmatory strategy defines options for analyses (e.g., at interim analyses) of the trial data that permit a confirmatory conclusion on one or more of the pre-specified hypotheses (i.e., formal rejection of the null hypothesis). Taken together the confirmatory strategy defines a statistical procedure that permits conclusions about the corresponding hypotheses with pre-determined control of the Type I error rate. Usually, the strategy also needs to define the set of hypotheses that all need to be rejected before a general confirmatory conclusion on efficacy can be drawn. Among the vaccine trials discussed in this article, the Jcovden vaccine trial, for example, required the two vaccine efficacy hypotheses, counting events after two and after four weeks, respectively, to be rejected to confirm efficacy.
However, as soon as the plan foresees interim analyses, or the assessment of multiple hypotheses it is not possible to anticipate at which planned analysis the null hypothesis will be rejected, it is useful to define specific terminology for the trial planning and analysis stages. We propose the following endpoint-specific terminology for the planning and analysis stage separately (see for an overview), as we consider this instrumental to address the inferential considerations more precisely.
Table 2: Overview of proposed terminology for trials with interim analyses or updates; Note that the definitions are usually endpoint-specific and might differ from one endpoint to another.
3.1.1 At the Planning Stage
In a clinical trial planned with one or more interim analyses under Type I error control for the specific endpoint, we define the primary analysis as the analysis based on a pre-specified total number of events driving sample size and power. This is the last analysis time point which might lead to a “statistically significant” result, that is, the rejection of the null hypothesis. The term “final analysis” is often used in this context. It leads to a misconception that this analysis time point, that is, number of events, is the most important, most informative, and the definitive assessment of efficacy. As we will see when we discuss the analysis stage, this is often not the case. Hence, we consider it preferable to refer to this analysis as the primary analysis—which also ensures consistency with the Type I error control construction for the primary hypothesis in the design with interim analyses. Follow-up analyses or updates, subsequent to the primary analysis, might already be planned at the design stage. These analyses can have a specific scientific or regulatory value, for example, they can be required to allow sufficient follow-up for safety, to inform about durability of protection, to allow a more robust assessment of most important endpoints or to obtain results in subgroups. In that case, it is beneficial to preplan the timing of these analyses in the study protocol based on when the required information is predicted to become available. For example, the primary analysis can be based on number of events and the updates based on minimum follow-up, calendar time or number of events for another important endpoint.
3.1.2 At the Analysis Stage
Once the trial is underway it will at some point reach conclusions on the hypotheses included in the confirmatory strategy. A null hypothesis may be rejected at one of the scheduled analyses under Type 1 error control. In this case the null hypothesis can be rejected with predetermined Type I error control. In the situation where the null hypothesis was not rejected up to and including the preplanned primary analysis for that endpoint, the null hypothesis on that endpoint can no longer be rejected in the trial.
Therefore, from an inferential and communication point of view it is considered useful to be able to refer concisely to the analysis that led to one of the above conclusions, in a way that signifies the confirmatory nature of the analysis (i.e., in the confirmatory strategy and with control of the Type I error rate at a pre-determined level). We hence propose to call this analysis the confirmatory analysis and the corresponding data cutoff the confirmatory analysis data cutoff, irrespective if it refers to an interim or the primary analysis at planning stage. In case the null hypothesis was not rejected at any preplanned analysis time point, the primary analysis and confirmatory analysis coincide, and the study outcome is negative, at least for that endpoint. Note that calling a confirmatory analysis or the corresponding results “interim” is no longer of key relevance at the analysis stage and might even give the wrong impression of preliminary and mediocre results as was seen during the pandemic.
After the confirmatory analysis, there is no further Type I error-controlled inferential analysis time point for that endpoint. All further analyses for that endpoint are exploratory in nature. We call these updated analyses of that endpoint, which happen at the so-called updated analysis data cutoffs. Importantly, analyses with longer follow-up than the confirmatory analysis may be of relevance. However, from a statistical point of view, these are all considered purely exploratory; such analyses cannot turn a failed trial into a positive one, nor can they overturn a previous confirmatory analysis in terms of its statistical significance. Strong inconsistency with the confirmatory analysis may render a previous positive conclusion less convincing and affect regulatory decision making. Usually, further insight into potential reasons for these inconsistencies is required.
3.1.3 Decision Making
As indicated above, regulatory decision making has multiple stages. The first step is a conclusion that the study was formally positive, that is, the primary endpoint was met, at the confirmatory analysis at a preplanned time/information point. For the marketing approval, regulators subsequently need to decide on a positive benefit/risk balance. We define the analysis cutoff that was the basis for decision making on benefit/risk (including the labeling information; SmPC) as the decisive data cutoff and call the corresponding analysis the decisive analysis. We note that the decisive analysis is often, but not always, the same as the confirmatory analysis. The confirmatory analysis and decisive analysis data cutoffs may differ where more mature data is needed to conclude on benefit/risk in relevant subgroups and/or assess other important endpoints. Specification of the decisive data cutoff thus supports clarity and transparency on the main body of evidence used for benefit/risk assessment.
4 Case Studies: The First Four Approved COVID-19 Vaccines
For three of the COVID-19 vaccines (Comirnaty, Spikevax and Vaxzevria), the estimated vaccine efficacy estimates that were part of benefit/risk assessment were based on an updated analysis data cutoff. In one case (Jcovden) both confirmation and estimation of efficacy were based on the first presented data. That analysis was, however, far beyond any preplanned number of events in the sequential design due to an additional requirement of minimum follow-up time for the confirmatory analysis. Together with further complexities and issues in the conduct and analysis of the studies this resulted in a multitude of reported estimates (see ).
Table 3: Overview of a selection of key trial results as published in the European Public Assessment Report (EPAR) and the information for prescribers as covered in the Summary of Product Characteristics (SmPC) at the time of the initial approval.
The decisive data cutoff(s) did not correspond to the confirmatory analysis data cutoff(s) for three of the four vaccines. The timing of the confirmatory analysis also did not correspond to any of the planned interim or primary analyses: in some cases, planned interim analyses were not conducted while in other cases interim analyses and/or the primary analysis were conducted based on a greater number of events than planned. This gave rise to possible concerns that the timing of analyses might have been influenced by the observed efficacy data or other factors stemming from Sponsor internal decision-making and, thus, estimates might be biased. However, in all cases reasonable explanations were provided for this “overrunning”: Due to the attack rate (Nauta Citation2020) being greater than expected at least for the Comirnaty and Spikevax trials, events accrued faster than anticipated with the first interim analysis then occurring much earlier than expected, that is, with a smaller number of patients and shorter follow-up than initially anticipated according to the protocol assumptions. In case of Vaxzevria, it also turned out to be difficult to predict the onset and duration of waves which hence impacted trial conduct. Sponsors cited logistical challenges in keeping up with the rapid accrual of events and the aim to have sufficient follow-up times, both for efficacy and safety as reasons for the deviations from the initial plans. Additionally, in all cases the estimated vaccine efficacy was very large, and formal proof of efficacy was clearly established at the first analysis which was considered to be the confirmatory analysis. Results were so convincing that they outweighed any possible concerns about Type I error control or bias to such an extent that benefit/risk would become negative. In cases where multiple data cutoffs were submitted, the results were all well in line, further mitigating the risk of bias.
Only a fraction of the information regarding clinical efficacy eventually submitted during the rolling review was available at the time of the confirmatory analysis, except for Jcovden. Submission of updated analyses based on updated data cutoffs were important to provide further information for the final benefit/risk assessment; updated analyses provided reliable effect estimates in important subgroups such as age and risk groups as well as more robust estimates of VE, durability of VE with longer follow-up, and other important secondary endpoints, such as severe COVID-19 disease. Last but not least the safety profile could be refined based on the updated analysis data cutoffs. The confirmatory analyses providing formal proof of efficacy and the decisive analyses used in the benefit/risk assessment were based on substantially different amounts of information which resulted in additional (statistical) complexity. As the observed vaccine efficacy estimates substantially exceeded initial expectations and minimum requirements, these updates did not directly affect decision making (derivation of a positive benefit/risk balance) for the current vaccines. It did however affect the estimated vaccine efficacy as included in the SmPC in terms of (slightly) different point estimates (see ). Furthermore, it was difficulty to adequately convey the uncertainty of the updated estimate via confidence intervals as there Type I error control was no longer in place.
5 Reflection on the Confirmatory Analyses: Formal Proof of Efficacy
Three of the vaccine efficacy trials employed frequentist group sequential study designs with one or more planned interim analyses under Type I error control for the primary endpoint; the fourth used a Bayesian design. The success criterion differed between the trials (at least 30% or 20%), as did the primary endpoint with respect to the precise case definition. Due to the differences in eligibility criteria and staggered entry of subjects of older age in the Oxford/Astra Zeneca and Janssen trials, populations at time of formal proof of efficacy also differed between vaccines and slightly differed between the confirmatory and decisive analysis.
At the planning stage, there was great uncertainty concerning the expected accrual of cases, as the incidence of COVID-19 disease was unforeseeable. The studies were planned as event-driven studies, with analyses triggered by a certain number of observed events. In all cases, the studies were planned to be continued after interim analyses, specifically patients were still followed for efficacy and safety and to provide more information in important subgroups for purpose of regulatory decision making and benefit/risk assessment (European Medicines Agency, Committee for Medicinal Products for Human Use 2019). This is common practice in clinical trials, including the provision to regulatory authorities of updated analyses during the submission process (e.g., in oncology). Thus, after an interim analysis was successful and this confirmatory analysis was communicated globally, case accrual continued to further inform the decisive analysis. This happened at unforeseen speed, affecting the timing and extent of information available at subsequent updates and the decisive analyses. How updated analyses of the primary endpoint should be interpreted in relation to Type I error control and the original analysis plan, including the uncertainty associated with these updated estimates, was not pre-defined. Despite efforts to monitor the accrual of cases in a timely manner, the planned interim analyses for all vaccines took place at a greater number of cases than specified in the protocol. Both the group sequential designs with defined alpha spending as well as the Bayesian design can in principle accommodate deviations from the originally planned timing such that interim analyses remain under Type I error control up to the rejection of the null hypothesis, as long as these changes are not data driven. Key results for all four trials are summarized in . More details on the planned study characteristics and results are provided in the supplementary materials.
For the BioNTech/Pfizer vaccine (Comirnaty) the planned analyses for the primary endpoint were Bayesian, and the choice of the prior distribution and success criteria used properties similar to classical Type I error control: to control the probability of false conclusions, the overall probability of success when the true VE is 30% was determined to be below 2.5%. The results of the Bayesian confirmatory analysis were complemented by Clopper-Pearson confidence intervals to ensure that the frequentist perspective was reflected in the interpretation. Only small differences between the credible and confidence intervals were observed. Given the observed overwhelming vaccine efficacy, this discrepancy was considered negligible for formal proof of efficacy. However, in situations where vaccine efficacy is borderline, the choice of the statistical method might play a crucial role and Bayesian methods may not be accepted if there is a lack of “self-standing evidence” from the pivotal trial given the use of prior information, or if there is a lack of analytical, study-wise Type I error control in the strong sense. The confirmatory analysis for the BioNTech/Pfizer vaccine was performed using 94 cases and an updated analysis, which became the decisive analysis, was performed using 170 cases.
The Moderna vaccine (Spikevax) delivered formal proof of efficacy in a relatively straightforward group sequential design with the first analysis at 95 events, where interim analyses were planned for 53 and 106 events. Despite deviating from original timing, the alpha spending allowed for a frequentist interpretation under the assumption that deviations from the original plan were implemented independently from efficacy results. This analysis rejected the null hypothesis and hence was considered the confirmatory analysis. The updated analysis to estimate VE included 196 events and was considered the decisive analysis. This analysis was no longer under Type I error control of the group sequential design. Thus, it is not immediately clear what the (possibly adjusted) level needs to be for a confidence interval to present the uncertainty of the updated point estimate: the group sequential design does not provide adjusted levels for analyses after the null hypothesis is rejected. For product labeling, the unadjusted 95% confidence interval waspresented.
Formal proof of efficacy for the Oxford/AstraZeneca (Vaxzevria) vaccine was based on the pooled analysis of two studies. The sample size and interim analysis plan to support this was developed independently from the composing studies, in parallel with the actual conduct and case accrual of the studies. The decision to reduce the number of interim analyses to one was taken close to the actual data cutoff for this first analysis. This analysis rejected the null hypothesis and hence was considered the confirmatory analysis. Although the results were accepted as proof of efficacy, there were substantial uncertainties remaining. Partly by original design, partly by accident and partly by deliberate change in regimen, some subjects were exposed to one dose only, some to two standard doses, and some to one standard and one low dose. While the protocol originally stated to give the two doses at least two weeks apart, some were treated with an exceptionally large window between doses varying from 3 to 23 weeks apart, because the second dose was included later in the study and was also affected by supply challenges during the pandemic. The primary proof of efficacy under Type I error control was based on seronegative subjects that completed a two-dose regimen, irrespective of dose or dosing interval and predominantly younger than 55 years of age due to the staggered entry. However, the regimen submitted for approval was two standard doses, given 4–12 weeks apart and formal testing of this regimen was not pre-specified. Due to these uncertainties and the limited data available at the confirmatory analysis, an updated analysis was essential. The updated analysis was considered the decisive analysis to inform benefit/risk. Results were in line with the confirmatory analysis, but challenging to interpret from a statistical inferential perspective.
The confirmatory analysis for the Janssen COVID-19 vaccine (Jcovden) was based on a sequential approach designed to provide formal demonstration of efficacy at the earliest time point possible. Safeguards were incorporated to guarantee the presence of severe cases and adequate follow-up time (median follow-up 8 weeks). The sequential approach was not corrected for the over-representation of the elderly group. According to the protocol, the truncated sequential analysis should have been performed at a much smaller number of events. However, the protocol additionally specified the requirement of a minimum follow up time to start the sequential analyses. Ultimately, this resulted in conduct of a single analysis for the Janssen trial at a data cutoff, on which initial safety assessment, efficacy-testing and subgroup analyses were based. Type I error control for the primary efficacy analysis was preserved and confirmation of efficacy and decision making were based on the same data cutoff making it also the decisive analysis data cutoff. The Jcovden program was the first to present (partial) information with respect to variants: the trial was conducted in three regions (United States, South Africa and several Latin American countries) and variants were assessed for a large number of the COVID-19 patients. Different variants dominated depending on the region. The observed overall VE was in principle applicable to a time dependent mixture of variants. VE was reported for the United States, South Africa, Brazil (countries with more than 100 cases) on partially sequenced (about 70%) data. Despite this mixture, due to the clear positive results formal proof of efficacy for the target population was considered confirmed. Nevertheless, this trial highlights a potential general issue with respect to estimation in the presence of multiple variants varying over time and positioning of the vaccines relative to others.
6 Reflection on Benefit/Risk Assessment: Estimating Vaccine Efficacy Based on a Decisive Data Cutoff
As described in earlier sections of the article, different estimation methods for vaccine efficacy were applied for each of the four vaccines. These methods do not necessarily provide estimates of the same population quantity, as in essence they represent different approaches to averaging the relative risk over the subjects’ follow-up time. That is, the estimate of vaccine efficacy for any one vaccine trial could have been influenced by one or more of multiple factors, including variable attack rates (which may be related to predominant virus variants in circulation), waning protection, composition of the study population in terms of natural exposure and/or risk of developing disease when infected and the duration of follow-up. These factors are relevant on top of the fact that the definition of symptomatic disease differed slightly between trials, leading to different quantities which were estimated. In this section we focus on the impact of the data cutoffs employed. Within the same vaccine study and using the same model, increasing follow-up of subjects may lead to a different VE estimate when efficacy is not constant over time. For all four vaccines trials median follow-up was relatively short, both for the confirmatory analysis as well as the decisive analysis, in the order of 2 months. No relevant differences in VE estimates were seen between these two timepoints, nor in sensitivity analyses using different models that were applied. This was consistent with stable and superior efficacy being demonstrated. In the urgent pandemic setting this was an adequate way to assess efficacy, but the level of protection for the longer term remained an important knowledge gap and required monitoring.
Challenges arise when confirmatory and decisive analyses provide apparently conflicting results, see also the general considerations of the relevant Reflection Paper on methodological issues in confirmatory clinical trials planned with an adaptive design (European Medicines Agency, Committee for Medicinal Products for Human Use 2007). One of the major challenges for estimation and informing stakeholders adequately on vaccine efficacy and uncertainties is that the information available at the confirmatory analysis and decisive analysis timepoints may be substantially different. Although this was not the case for the four approved vaccines, in other instances it is possible that the decisive analysis would no longer support the conclusions from the confirmatory analysis. On the other hand, there could be a concern that the decisive analysis may be biased and overly optimistic, for example, due to unblinding after the confirmatory analysis or opportunistic choices for the cutoff date. Thus, decisive data cutoffs that are not pre-defined and contain substantial additional data are controversial. The vaccine trials have shown that sometimes it cannot be avoided that confirmatory and decisive analyses are based on substantially different amounts of information. In particular in this pandemic situation, repeated updates were, and continue to be, used to inform vaccination campaigns and prescribers, but such updates are no longer subject to Type I error control. Estimates may be biased due to repeated analyses, and the probability coverage of confidence intervals from updates may not be well defined or controlled.
As a reflection, the alpha adjustment for the confirmatory analysis includes the uncertainty of the decision to claim the vaccine to be efficacious and is typically derived under the null hypotheses. A decision on a positive benefit/risk has subsequently to be made before the vaccine can be authorized. So, by definition for the benefit/risk assessment we act under the alternative that the vaccine is efficacious as the null hypothesis has been rejected. It is, however, questionable whether all stakeholders are best informed by the original decision-making uncertainty derived from a possibly much smaller dataset. The updated analyses with more events can lead to higher precision in estimation and a scientific discussion is needed on how updates should be incorporated into the protocol at planning stage and how updated information and associated uncertainties should be communicated for present and future approved products.
The Jcovden results were the first to highlight the challenges of evaluating vaccine efficacy in the presence of a complex mix of variants, differing between regions and changing over time. It is not obvious that confirmatory, decisive and update data cutoffs should lead to the same point estimates of overall vaccine efficacy if they substantially differ in time and stage of the pandemic. Moreover, they may actually not estimate the “same” population level vaccine efficacy. There is an increasing number of different strains of SARS-CoV-2 and it may not be the case that any vaccine that is efficacious against one strain would have a similar quantitative level of vaccine efficacy against all other possible strains. Observed vaccine efficacy is therefore a (dynamic) mixture of observed vaccine efficacy against each of the variants present during the course of the trial, depending on the attack rate and duration of observation in (sub)populations in the presence of each variant. The exact number of the vaccine efficacy estimates observed in clinical trials can only be interpreted at the level of variants. To support the clinical interpretation and obtain variant specific estimates of the VE, sequencing may be needed.
7 Discussion
The COVID-19 pandemic has provided numerous challenges for all stakeholders involved in the development and assessment of new vaccines. From the regulatory perspective, the circumstances under which the four first COVID-19 vaccines have been approved in the EU are unprecedented. The benefit/risk assessment was positive for all four vaccines, with efficacy being greater than initially expected by the sponsors. While cases were accrued in a short time period during “waves” of the pandemic allowing for rapid accumulation of shorter-term efficacy data, this posed challenges for assessment of duration of protection and safety. Had efficacy been less overwhelming, for example, borderline or slightly below the threshold, the regulatory decision-making process would have been much more complex.
Understandably, both the pharmaceutical development and regulatory assessment of these vaccines were under significant public interest and urgency. In this section, we would like to highlight several points arising from the pandemic experience for further discussion in the scientific and regulatory communities.
7.1 Regulatory Considerations
The methodological assessment of the design and results of the submitted vaccine efficacy trials was challenging due to various aspects related to time pressure; to provide early input at the planning stage rapid Scientific Advice procedures were established (European Medicines Agency Citation2020). To speed up the assessment of the marketing authorisation application (MAA), a rolling review process was established (European Medicines Agency Citation2020). Relevant evidence for efficacy and safety emerging from the pivotal trials was delivered on an ongoing basis in formats outside common standards of clinical trial reporting. Under these circumstances confirmation of compliance to fully pre-specified analysis strategies was not always possible, where the unpredictable development of the pandemic and accrual of cases were major contributing factors. There was an unusually high, but understandable, interest in the results (and regulatory assessment) of the first vaccine trials, both within the scientific community and the general public. Communication of results to the target audience was complicated by availability of results from several updated analyses. From a regulatory point of view, the decision as to which of these multiple results to include in the Summary of Product Characteristics to allow for adequate communication of estimates and related uncertainties has proven to be a challenge.
Efforts were made (Moderna, BioNTech/Pfizer) to define and discuss the (primary) estimand (ICH Citation2020) for the primary endpoint. More detailed specification of estimands following guidance in ICH E9(R1) remains important to address in settings where different choices could have larger impact on B/R assessment than seen in this case. Especially for vaccines, more explicitly defining the target of estimation and the estimand attributes from the start of the protocol design can improve interpretation as well as consistency across trials. Important attributes in this context include the target population, the actual treatment to be evaluated (e.g., in case of multiple doses over time to reach sufficient protection) and how important intercurrent events (such as those that lead to missing follow-up injections or that may affect immune response) are addressed.
7.2 Terminology
The intensive rolling regulatory review process paired with the generally high interest in the COVID-19 vaccine trials revealed a lack of consistent terminology for studies with (planned) interim analyses, follow-up analyses and time to event endpoints. In studies with time to event endpoints not all patients have experienced the event of interest at the time of analysis; for example, in the vaccine trials only a small fraction of the patients in the study had experienced the event of interest at the planned time of primary analysis. The analysis timing is usually defined in the protocol based on assumptions for the expected rate of events in the comparator arm, the expected magnitude of the treatment or protective effect, the pace of enrollment and statistical properties such as Type I error, power and the statistical test to be applied. This results in a specific figure for the number of events required for the primary analysis, which is a fraction of the patients in the study and fully dependent on the protocol assumptions. Patients usually remain in the study after the primary analysis. For these reasons studies with time to event endpoints rarely entail a final set of results at the time of submission to health authorities. Such a time point with fully final results does not actually exist by design. This poses challenges both at the design stage and after study read out. Interim analyses are often included in trials with time to event endpoints and this results in one or more analyses before the planned primary analysis triggered by accrual of a fraction of the targeted number of events. At the analysis stage it is usually not of primary interest whether an endpoint was rejected at the planned interim or primary analysis. Terming such a significant result “interim” gives a wrong impression of preliminary and mediocre results, as was evidenced when the first vaccine trials showed significant benefit. We hence proposed a set of terms for clinical trials which is intended to ensure: (a) that all stakeholders involved share a common understanding of the intended plans and nature of the results and (b) we can more fundamentally assess the inferential properties of the different analyses. In this respect, we look forward to future discussions and methodological refinement.
7.3 Consideration in Case of Multiple Endpoints under the Confirmatory Strategy
In this article, we have not discussed the proposed terminology in full depth and how it generalizes to more complex situations. The terminology was introduced in the relatively simple context of a single primary endpoint, under Type I error control. Clinical trial designs may include multiple hypotheses to be tested as part of a confirmatory strategy under Type I error control. These hypotheses may relate to different definitions of the endpoint (such as cases counted after 2 or 4 weeks for Jcovden), different endpoints, multiple treatment arms or subgroups. They can also include more complex scenarios with redistribution of the Type I error between hypotheses (e.g., related to doses, endpoints and superiority or noninferiority objectives). In principle, the proposed terminology can also be used in these situations, at least for endpoints under Type I error control planed with multiple analyses. However, care needs to be taken to avoid wrong impressions of importance of an endpoint: It is not always clearly articulated in study protocols what constitutes study success with respect to efficacy: which hypotheses need to have been rejected to confirm efficacy (confirmatory concept) at the study level? This adds potential complexity, as timing and amount of data at which the different individual hypotheses are rejected for the first time can diverge. The proposed terminology (especially the term “confirmatory analysis”) highlights the specific importance that needs to be attached to the analysis at which the hypotheses that define study success are actually rejected. It also emphasizes the need to pre-specify which hypotheses will define study success, as well as to consider other hypotheses in the hierarchy (e.g., where the applicant sees particular strengths in his product).
7.4 Different Data Cut-Offs for Testing and Estimation
The decoupling of statistical hypothesis testing and statistical estimation of the protective effect (i.e., vaccine efficacy) was a rather unusual feature of the regulatory statistical assessment, particularly in the context of vaccine trials. With this decoupling, the correspondence of Type I error controlled statistical testing and of the estimation of the treatment or protective effect with uncertainty estimates (i.e., confidence intervals) was no longer present. Based on the confirmatory analyses, it was obvious that all four vaccines discussed in this article would exceed the required vaccine efficacy threshold communicated earlier by EMA/FDA (European Medicines Agency, Committee for Human Medicinal Products Citation2020). Hence, actual statistical testing of predefined hypotheses reflecting this minimum threshold soon became moot. However, the issues of vaccine efficacy estimation and benefit/risk assessment became more relevant overall, and especially in the context of the benefit/risk balance across various subgroups.
Numerous sources communicated different estimates of vaccine efficacy: scientific publications in medical journals, press releases, pre-prints, and statements by various public health authorities. Estimates were provided from different stakeholders with different roles and responsibilities and hence a different focus. The reported results were also often based on different data cutoffs dependent on the study maturity at the time of estimation. This was a unique and unprecedented situation given the enormous public interest in these data. This showed once more the importance of a proper and in-depth scientific review and of an adequate preplanned analysis strategy that covers analyses and data cutoffs up to crucial decision making and the associated communication strategy.
It should also be taken into consideration at the planning stage whether in the case of very fast accumulating events in an event-driven trial a minimum follow-up should be specified as an additional criterion to ensure sufficient evidence is generated for B/R decision-making. This can help to ensure the same cutoff can be used both as the confirmatory analysis and the decisive analysis, and thereby possibly avoid multiple cutoffs because sufficiently mature results would be available at the time of the confirmatory analysis.
7.5 Variants
The COVID-19 vaccines were developed and licensed in a very complex research situation. Temporal and regional heterogeneity in the attack rate complicate the interpretation of trial outcome; the VE to be expected while implementing a vaccine will highly depend on the time dependent mixture of variants and the variant dependent vaccine efficacies. We therefore suggest foreseeing sequencing to determine variant variation as part of vaccine trials and implementing it as early as possible, taking into account that the epidemiology of variants is dynamic and novel variants can emerge at any moment. Real World Data (RWD) is being used to estimate vaccine effectiveness with upcoming variants and to inform on vaccine effectiveness according to the current epidemiological knowledge. Current attempts of addressing pandemic-related questions by means of RWD are for example (European Medicines Agency Citation2021; Andrews et al. Citation2022; European Centre for Disease Prevention and Control Citation2022; UK Health Security Agency Citation2022). RWD is also used to estimate duration of protection and in an attempt to address other missing information. Specific challenges of planning research with RWD are, however, not in scope of this article.
7.6 Better Standardization of Trials
For the pivotal studies of the first four vaccines, important differences in key elements of trial design were present. Most notably, there were differences between trials in the definition of outcomes, analysis populations and statistical analyses, including interim monitoring. The definition of symptoms that determined identification of COVID-19 cases to be confirmed by PCR testing differed between trials, whereas usually such primary outcomes would be standardized. In the early days it was not evident which symptoms should be part of the definition of symptomatic infections as the clinical features of the COVID-19 disease had not yet been fully characterized and were changing over time due to SARS-CoV-2 variants. The VE estimates were based on different analysis populations and statistical methods. This did not hamper the primary conclusions on efficacy and is understandable given the rapidly evolving knowledge of the COVID-19 disease and parallel urgent development of the trial protocols. Overall, this led to vaccine efficacy estimates that are not directly comparable, which was a challenge in the light of the necessary and very broad communication of trial results. We consider that better consistency for some of these key elements would have been possible also under the apparent constraints, as all parties suffered from the same lack of knowledge. Arranging the necessary collaboration across all stakeholders to achieve higher consistency across trials should be an essential element of pandemic preparedness.
7.7 Planning for the Future
The pivotal trials for the first four approved vaccine can be considered a ground-breaking scientific success because they provided convincing results quickly under enormous time pressure, and in a difficult operating environment. Important learnings and areas for future research were identified when these studies were assessed in detail during assessment of the conditional marketing authorization applications. In this article, we highlight some of these areas to stimulate further discussion between academia, industry and regulators.
First, there are some unique features in vaccine trials such as emerging variants or the population definition which typically includes only patients who received the full vaccination schedule and were followed up for a minimum of pre-specified number of days. This has implications for the choice of estimand in vaccine trials. Appropriate application of the estimand framework can facilitate a detailed upfront discussion of which questions can, and cannot, (and which questions should) be answered by the proposed trial and can help ensure the trial design is sufficient to answer the required question(s). Through such a framework, the discussion can lead to a better standardization and interpretation of trials within a particular disease area.
Second, we saw various updates of trial results during the rolling reviews. In general, we would like to see pivotal clinical trials planned such that the confirmatory analysis data cutoff provides all the relevant information for the benefit/risk assessment and, hence, multiple updates are not necessary for marketing authorization. There are foreseeable situations where updated results are needed, and these should be appropriately preplanned in the protocol. Hence, we would like to see better scenario planning for analyses and data cut offs in study protocols, especially when interim analyses are included.
Third, the updated analyses raised an interesting and relevant statistical issue. There does not appear to be a common standard across the field of clinical trials on how the uncertainty related to multiple, sequential updates on the same clinical endpoint can best be assessed and communicated. Especially when these updates follow a successful, positive confirmatory analysis. The most used method is a 95% confidence interval without multiplicity adjustment. We welcome further discussions around the desired properties of updated uncertainty estimates, also with respect to communication. The existence of alternatives to the 95% CI approach which, for example, would allow a form of error control even after the primary analysis would be of particular interest. We invite methodological discussions on this topic and around the general communication of updated estimates after the confirmatory analysis.
Fourth, we see that the field of clinical trials lacks standard terminology for studies with interim analyses and time-to-event endpoints. Precise definitions and standard terminology are a prerequisite for transparent communication and informed discussion on a study design. In this article, we propose standardized terminology and seek feedback from academia, industry and regulators. The proposed terminology is intended for all clinical trials and especially those with multiple analysis timepoints and time-to-events endpoints and is not limited to vaccine trials.
Finally, albeit the first vaccine trials were clearly positive in the primary endpoint, important scientific questions remain, such as efficacy against new emerging variants and duration of protection. The pivotal clinical trial protocols should include appropriate plans on how to gather as much information as possible for these important secondary outcome measures. The ability to collect longer term data may depend on whether cross-over to effective vaccine for placebo participants is allowed once the trial has demonstrated a positive benefit/risk, or indeed when a vaccine program is rolled out. These competing priorities of incentives for trial participants to enroll, the requirements of national vaccination programmes and the need to collect longer term data or more data in trials needs to be carefully balanced and we expect to see this better reflected in future protocols.
In conclusion, the pivotal trials for the first four vaccines authorized by the European Commission fulfilled their purpose and provided adequate evidence for granting conditional marketing authorization. We should, however, leverage the learnings all involved parties made during the 2020–2021 pandemic experience to develop even better vaccine trial protocols in the future which would ideally allow quick generation of robust results, and better comparability between vaccines, even under severe time pressure. We think that this can be better achieved through collaboration between academia, industry and regulators.
Supplementary Materials
Appendix to Section 4: Detailed description of the four case studies.
Supplemental Material
Download PDF (255.2 KB)Disclosure Statement
The authors had been involved in the regulatory assessment of the considered vaccines.
The views expressed in this article are the personal views of the authors and may not be understood or quoted as being made on behalf of or reflecting the position of the regulatory agencies or organizations with which the authors are employed/affiliated.
BH, EA,WJ, KOR, LAD, MG, FK, MP, FPL, TL, AK, JZ and KR are affiliated with EMA’s Biostatistics Working Party and/or the European Specialized Expert Community of the Methodology Working Party. AK and JZ are affiliated with EMA’s Scientific Advice Working Party. FPL was affiliated with EMA’s Scientific Advice Working Party at the time of article submission and is affiliated with EMA’s Committee for Medicinal Products for Human Use since September 2022. FK is affiliated with EMA’s Emergency Task Force.
Martin Posch declares that he is the statistician of the trial “A Phase 2, Comparative Randomized Trial to Evaluate the impact of reduced COVID-19 mRNA vaccination regimens on immunological responses and reactogenicity in pediatric subjects with and without prior SARS-CoV-2 infection (CoVacc)” and contributes also the other clinical trials funded by the EU-Horizon 2020 Project Vaccelerate H2020-IBA-SC1-CORONAVIRUS-2020-4. Grant agreement number 101037867.
Additional information
Funding
References
- Andrews, N., Stowe, J., Kirsebom, F., Toffa, S., Rickeard, T., Gallagher, E., Gower, C., Kall, M., Groves, N., O’Connell, A.-M., Simons, D., Blomquist, P. B., Zaidi, A., Nash, S., Iwani Binti Abdul Aziz, N., Thelwall, S., Dabrera, G., Myers, R., Amirthalingam, G., Gharbia, S., Barrett, J. C., Elson, R., Ladhani, S. N., Ferguson, N., Zambon, M., Campbell, C. N. J., Brown, K., Hopkins, S., Chand, M., Ramsay, M., and Lopez Bernal, J. (2022), “Covid-19 Vaccine Effectiveness against the Omicron (B.1.1.529) Variant,” New England Journal of Medicine, 386, 1532–1546. DOI: 10.1056/NEJMoa2119451.
- European Centre for Disease Prevention and Control (2022), “Interim Analysis of COVID-19 Vaccine Effectiveness against Severe Acute Respiratory Infection Due to Laboratory-Confirmed SARS-CoV-2 Among Individuals aged 30 years and older, ECDC Multi-country Study – Second Update,” available at https://www.ecdc.europa.eu/sites/default/files/documents/covid-19-vaccine-effectiveness-interim-analysis-second-update.pdf
- European Commission (2006), “Commission Regulation (EC) No 507/2006 of 29 March 2006 on the Conditional Marketing Authorisation for Medicinal Products for Human Use Falling Within the Scope of Regulation (EC) No 726/2004 of the European Parliament and of the Council,” In Official Journal of the European Union, available at https://eur-lex.europa.eu/eli/reg/2006/507/oj
- European Medicines Agency (2020), “EMA Initiatives for Acceleration of Development Support and Evaluation Procedures for COVID-19 Treatments and Vaccines,” EMA/213341/2020 Rev.3 [online, accessed 20/01/2022], available at https://www.ema.europa.eu/en/documents/other/ema-initiatives-acceleration-development-support-evaluation-procedures-covid-19-treatments-vaccines_en.pdf
- European Medicines Agency (2021), “Heterologous Primary and Booster COVID-19 Vaccination,” EMA/349565/ 2021 [online, accessed 11/04/2022], available at https://www.ema.europa.eu/en/documents/report/heterologous-primary-booster-covid-19-vaccination-evidence-based-regulatory-considerations_en.pdf
- European Medicines Agency, Committee for Human Medicinal Products (2017), “Guideline on Multiplicity Issues in Clinical Trials,” EMA/CHMP/44762/2017 [online], available at https://www.ema.europa.eu/en/documents/scientific-guideline/draft-guideline-multiplicity-issues-clinical-trials_en.pdf
- European Medicines Agency, Committee for Human Medicinal Products (2018), “Guideline on Clinical Evaluation of Vaccines,” EMEA/CHMP/VWP/164653/05 Rev. 1 [online], available at https://www.ema.europa.eu/en/documents/scientific-guideline/draft-guideline-clinical-evaluation-vaccines-revision-1_en.pdf
- European Medicines Agency, Committee for Human Medicinal Products (2020), “EMA Considerations on COVID-19 Vaccine Approval,” EMA/592928/ 2020 [online], available at https://www.ema.europa.eu/en/documents/other/ema-considerations-covid-19-vaccine-approval_en.pdf
- European Medicines Agency, Committee for Medicinal Products for Human Use (2006), “Guideline on Clinical Evaluation of New Vaccines,” EMEA/CHMP/VWP/164653/2005 [online], available at https://www.ema.europa.eu/en/documents/scientific-guideline/guideline-clinical-evaluation-new-vaccines_en.pdf
- European Medicines Agency, Committee for Medicinal Products for Human Use (2007), “Reflection Paper on Methodological Issues in Confirmatory Clinical Trials Planned with an Adaptive Design,” CHMP/EWP/2459/02 [online], available at https://www.ema.europa.eu/en/documents/scientific-guideline/reflection-paper-methodological-issues-confirmatory-clinical-trials-planned-adaptive-design_en.pdf
- European Medicines Agency, Committee for Medicinal Products for Human Use (2019), “Guideline on the Investigation of Subgroups in Confirmatory Clinical Trials,” EMA/CHMP/539146/2013 [online], available at https://www.ema.europa.eu/en/documents/scientific-guideline/guideline-investigation-subgroups-confirmatory-clinical-trials_en.pdf
- European Medicines Agency, Committee for Medicinal Products for Human Use (2021a), “Assessment Report - Comirnaty - Procedure No. EMEA/H/C/005735/0000,” EMA/707383/2020 Corr.1 [online, accessed 26/01/2022], available at https://www.ema.europa.eu/en/documents/assessment-report/comirnaty-epar-public-assessment-report_en.pdf
- European Medicines Agency, Committee for Medicinal Products for Human Use (2021b), “Assessment Report - COVID-19 Vaccine AstraZeneca - Procedure No. EMEA/H/C/005675/0000,” EMA/94907/2021 [online, accessed 20/01/2022], available at https://www.ema.europa.eu/en/documents/assessment-report/vaxzevria-previously-covid-19-vaccine-astrazeneca-epar-public-assessment-report_en.pdf
- European Medicines Agency, Committee for Medicinal Products for Human Use (2021c), “Assessment Report - COVID-19 Vaccine Janssen - Procedure No. EMEA/H/C/005737/0000,” EMA/158424/2021 [online, accessed 20/01/2022], available at https://www.ema.europa.eu/en/documents/assessment-report/covid-19-vaccine-janssen-epar-public-assessment-report/textunderscoreen.pdf
- European Medicines Agency, Committee for Medicinal Products for Human Use (2021d), “Assessment Report - COVID-19 Vaccine Moderna - Procedure No. EMEA/H/C/005791/0000,” EMA/15689/2021 Corr.1 [online, accessed 20/01/2022], available at https://www.ema.europa.eu/en/documents/assessment-report/spikevax-previously-covid-19-vaccine-moderna-epar-public-assessment-report/textunderscoreen.pdf
- European Medicines Agency, Committee for Proprietary Medicinal Products (2002), “Points to Consider on Multiplicity Issues in Clinical Trials,” CPMP/EWP/908/99 [online] available at https://www.ema.europa.eu/en/documents/scientific-guideline/points-consider-multiplicity-issues-clinical-trials_en.pdf
- ICH (1998), “Statistical Principles for Clinical Trials E9,” [online], available at https://database.ich.org/sites/default/files/E9_Guideline.pdf
- ICH (2020), “Addendum on Estimands and Sensitivity Analysis in Clinical Trials to the Guideline on Statistical Principles for Clinical Trials E9(R1),” [online], available at https://database.ich.org/sites/default/files/E9-R1_step4_guideline_2019_1203.pdf
- Nauta, J. (2020), Statistics in Clinical and Observational Vaccine Studies (2nd ed.), Cham: Springer. DOI: 10.1007/978-3-030-37693-2.
- UK Health Security Agency (2022), “Monitoring Reports of the Effectiveness of COVID-19 Vaccination,” [online], available at https://www.gov.uk/guidance/monitoring-reports-of-the-effectiveness-of-covid-19-vaccination
- World Health Organization (2020a), “An International Randomised Trial of Candidate Vaccines against COVID-19,” [online], available at https://www.who.int/publications/i/item/an-international-randomised-trial-of-candidate-vaccines-against-covid-19
- World Health Organization (2020b), “WHO Target Product Profiles for COVID-19 Vaccines,” Version 3 – 29 April 2020. [online], available at https://www.who.int/docs/default-source/blue-print/who-target-product-profiles-for-covid-19-vaccines.pdf