ABSTRACT
Local regression has an advantage over global regression by allowing coefficients that qualify variables relationships being heterogeneous, where such varying regression relationships are nonstationarity. Spatiotemporally Varying Coefficients (STVC) model is the first Bayesian-based local spatiotemporal regression approach, intending to simultaneously detect spatial and temporal nonstationarity for heterogeneous response-covariate variables relationships, through separately estimating posterior local-scale coefficients over different space areas and time frames. In this paper, we first presented a general Bayesian STVC modelling paradigm as a specification guide to show its commonality in broader geospatial research. Then, we employed it to solve a real-world issue concerning spatiotemporal healthcare-socioeconomic relations, for which we derived data of county-level hospital beds number per capita, as well as data of related socioeconomic factors in northeast China during 2002–2011. Results showed that the STVC model surpassed all the other comparative regressions, in terms of both Bayesian model fitness and predictive ability. Globally, resident savings, financial institutions loans, GDP, and primary industry were identified as key socioeconomic conditions affecting healthcare resources in Northeast China. Temporally, with Time-Coefficients (TC) plots, we found that after 2011, GDP and primary industry would further help improve the overall healthcare level of northeast China. Spatially, with Space-Coefficients (SC) maps, we could directly identify the relative contribution of four socioeconomic covariates’ impacts on healthcare within each administrative county. Bayesian STVC model is an essential development and extension of the local regression family for exploring the spatiotemporal heterogeneous variables relationships, especially under Bayesian statistics, as well as GIScience and spatial statistics.
Graphic abstract
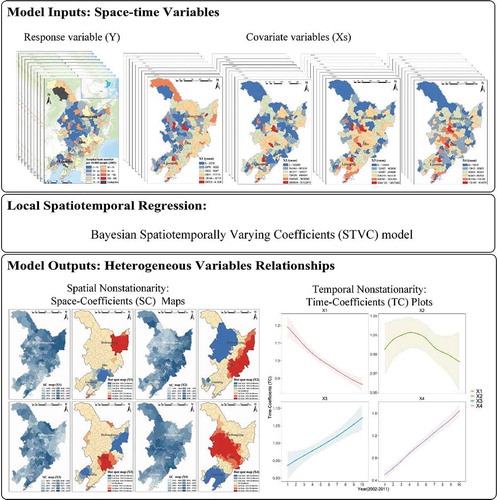
Acknowledgements
The data were initially collected by Beijing Normal University under the guidance of Yanchen Bo and processed by the Spatial Information Technology and Big Data Mining Research Center in Southwest Petroleum University. We appreciate the International association of Chinese professionals in geographic information sciences (CPGIS) and Jiangxi Normal University for holding the frontier forum of Application of GIS analysis in Public Health. We would like to thank the editors and three anonymous reviewers for their constructive comments and valuable suggestions on improving this manuscript.
Disclosure statement
No potential conflict of interest was reported by the authors.
Supplementary materials
Supplemental data for this article can be accessed here