ABSTRACT
Invasive species are ranked as one of the leading drivers of global biodiversity loss. To mitigate their impact, we must understand the future risks caused by invasive species, particularly to flora of conservation concern. Here we used species distribution modelling (SDM) to project the current and future (RCP45 and RCP85 2050) distributions of four deer species and 13 plant species of conservation concern for the island of Ireland, quantifying changes in distributions and overlap. Large areas of suitable habitat for the deer species were predicted with high accuracy across all counties, with future climate scenarios identifying an expansion in sika deer distributions and a decrease in muntjac and fallow deer distributions. Red deer declined under the moderate climate change scenario but increased under the worst-case projection. Future projections predicted the (local) extinction of six (out of 13) endangered and vulnerable plant species. An expansion in distributions was observed for four plant species; however, these areas had large overlap with the future predictions of deer, placing further pressures on these plant species. These findings suggest that targeted conservation and management measures are required to alleviate the pressures on ‘at-risk’ plant species due to grazing from native and non-native deer.
Introduction
Climate change and concomitant human activities are placing ecosystems under unparalleled pressures (Chen et al. Citation2011; Linders et al. Citation2019; Pejchar and Mooney Citation2009). The recent Intergovernmental Science Policy Platform on Biodiversity and Ecosystem Services (IPBES) report (Díaz et al. Citation2019) identified that over 1 million species are threatened with accelerated extinction. This deterioration is directly linked to human activity, with the spread of invasive species ranked as one of the primary drivers of this loss. Invasions by non-native species directly and indirectly affect biodiversity and, as a result, ecosystem functions (Linders et al. Citation2019). In order to mitigate the potentially devastating scenarios for biodiversity and ecosystems, it is imperative to better understand the future extinction risk caused by invasive species (Bellard, Bernery, and Leclerc Citation2021).
Deer species, including sika (Cervus nippon), muntjac (Muntiacus reevesi) and fallow (Dama dama), are preponderant examples of invasive species in Europe (Saggiomo, Esattore, and Picone Citation2020). Following their introduction throughout Europe, sika deer have undergone rapid population increase (Carden et al. Citation2010; Homolka and Heroldová Citation2003), becoming naturalized in some countries with extensive and invasive populations found in Germany, the Czech Republic, United Kingdom and Ireland (Carden et al. Citation2010; Homolka and Heroldová Citation2003; Pérez-Espona, Pemberton, and Putman Citation2009). Muntjac deer are also expanding rapidly across Europe, with predictions that this species will expand its range to encompass every European country (Ward, Richardson, and Mergeay Citation2021). Large-scale browsing and grazing by non-native deer species has cascading effects on biodiversity due to direct interactions with other species, including plants, invertebrates, smaller species and other herbivores (Akashi and Nakashizuka Citation1999; Homolka and Heroldová Citation2003; Rooney and Waller Citation2003). For example, Pollard and Cooke (Citation1994) identified that muntjac deer preferentially grazed on lower leaves of honeysuckle (Lonicera periclymenum) which are the preferred egg laying sites of the white admiral butterfly (Ladoga Camilla), leading to their population decline. This subsequently raises numerous management and control issues (Carden et al. Citation2010).
Overabundance of deer, and the additional effects that are associated therewith, such as effects on biodiversity or ecology, is extensively discussed in their native ranges (Iida, Soga, and Koike Citation2016; Kato and Okuyama Citation2004; Ohashi et al. Citation2014; Uno et al. Citation2019). For example, Iida, Soga, and Koike (Citation2016) studied the effects of sika deer overpopulation on flora and invertebrates in Western Hokkaido in Northern Japan. Two sites were investigated, the shore of Lake Troya (a control site) and Nakanoshima Island (experimental site). During the 1950’s and 60’s, three sika deer were introduced on the island for tourism (Kaji, Koizumi, and Ohtaishi Citation1988). Over this period there was a significant increase in sika deer density on the island (Ikeda et al. Citation2013), which resulted in a dramatic decrease in both understory vegetation and beetle diversity. This study quantifies how populations of sika deer can alter native species richness, abundance and likely the ecological functions of an area.
Saggiomo, Esattore, and Picone (Citation2020) conducted a recent bibliometric analysis which presented all published scientific work on sika deer that has been accomplished to date. It was found that most studies came from Asia, Europe, Africa and North America. The literature on native populations focused mainly on the effects of sika overpopulation and its effects on vegetation such as the dwarf bamboo, whereas the literature on sika deer in their introduced range mainly explored hybridization (Bailey Citation2013; Gélin et al. Citation2019; Huxel Citation1999). This is in contrast to the impact of muntjac and fallow deer on native and non-native vegetation that has been widely researched (Pollard and Cooke Citation1994; Cooke and Farrell Citation2001, Morecroft et al. Citation2003; Husheer and Frampton Citation2005; Marozas et al. Citation2009; Fuller and Gill Citation2010; Cooke Citation2021; Cunningham et al. Citation2022). The consensus so far is that deer species, particularly non-native species, negatively impact vegetation diversity in regions, with cascading effects on insect and bird species. However, studies have not been conclusive, with differences across species and geographic regions. Studying one species in isolation can lead to species-specific results that are not generalizable to the wider and connected ecosystem (Meyer et al. Citation2019), particularly for organizations and practitioners managing these ecosystems. Subsequently, extensive studies exploring the impact of multiple deer species distributions and populations on the flora of non-native ecosystems are lacking (Saggiomo, Esattore, and Picone Citation2020).
In Ireland, between 1978 and 2008 there was a range expansion of 565%, 353% and 174%, for red, sika and fallow deer, respectively (Carden et al. Citation2010). The increase in both human and deer populations has heightened the potential for human-deer conflict in recent years, with research predominantly focusing on their impact to the agriculture and forestry sectors (Hawthorne, Colombaroli, and Mitchell Citation2021; Liu and Nieuwenhuis Citation2014; Wilson, Wirz, and Byrne Citation2021), deer-vehicle collisions (Liu, Nieuwenhuis, and McCullagh Citation2018), their role as disease vectors and/or hosts (Crispell et al. Citation2020; Kelly, Mullen, and Good Citation2021; McDonough and Holloway Citation2020), hybridization with the native red deer (Carden et al. Citation2010; Gélin et al. Citation2019; Huxel Citation1999; McDevitt et al. Citation2009; Smith et al. Citation2014), and their impact on the native vegetation (Perrin, Mitchell, and Kelly Citation2011), although the latter is the most depauperate research area at a national scale.
The diet of deer species is varied, with that of the non-native sika and native red deer overlapping in south-west Ireland, causing competition (Burkitt Citation2009). Although the bark from saplings and grasses are the preferred diet of sika, they have been known to browse and graze unconditionally on forbs, berries, heather and other vegetation (Mann and Putman Citation1989; Yokoyama, Kaji, and Suzuki Citation2000). Muntjac deer have also been found to graze plant species that are avoided by other deer species, such as bluebells (Hyacinthoides non-scripta), which can shift the equilibrium of native ecosystems (Dolman et al. Citation2010). In Ireland, the Red Data List of Irish Vascular Plants (National Botanical Gardens Citation2017) identifies that there are currently seven critically endangered plant species, 52 endangered species and 69 vulnerable species. Subsequently, any expansion of non-native (or even native) deer distributions in the future may place further pressures on these plant species that are already at risk due to climate change and habitat degradation. Despite the potential impact of invasive species, many of their effects can be mediated through vegetation abundance, species richness and diversity (Linders et al. Citation2019). To that end, non-native deer must be focused on as they are a key species for conservation and management efforts, particularly in supporting the Irish Biodiversity Action Plan (2019). To achieve sustainability in ecosystems and biodiversity, the prevention of any further spread, or establishment of an invasive species has been recognized as a more efficient and a less costly method of control than large-scale culling or barrier control (Barbet-Massin et al. Citation2018). Moreover, if it is known where future spreads may occur, then adaptation efforts can be implemented to ensure minimal impact on any native and/or flora of conservation concern. Therefore, the initial step for effective management is quantification of how deer and flora distributions will change in the future (Carden et al. Citation2010).
Species distribution models (SDMs) are one of the most important GIScience application areas (Burch et al. Citation2020; Laffan, Skidmore, and Franklin Citation2014; Miller et al. Citation2020), and a powerful spatial analytical tool for studying the geographic distribution of a range of taxa (Franklin Citation2010; Peterson et al. Citation2011), providing a methodological framework for researchers and practitioners to quantitatively assess the relationship between species distributions and environmental factors. SDMs project relationships in both environmental and geographic space using a variety of statistical methods and machine learning algorithms (Miller and Holloway Citation2017) and have been widely used for various applications, including projecting suitable aquaculture sites (O’Mahony, de la Torre Cerro, and Holloway Citation2021), forecasting global change impacts on biodiversity (Ehrlén, Morris, and Buckley Citation2015), locating nature reserves (Araújo et al. Citation2004), and predicting future ranges of invasive species (Barbet-Massin et al. Citation2018; McMahon et al. Citation2021). For example, McMahon et al. (Citation2021) modelled the distribution of 15 non-native invasive grass and forb species of conservation concern in western North America. The aim of the study was to characterize the species environmental niches and identify potential invasion risks. The results showed that the species are likely to expand their ranges and coupled with continued increases in aridity, temperature and burned areas the risk of future invasions is high. Through the use of GIScience and SDMs, this study was able to aid in identifying possible new invasion sites and prioritize the prevention and control of the invasive species.
Despite the fact that non-native deer species are of ecological and economic concern in Ireland, coupled with the ability of SDMs to project current and future distributions, there is a paucity of research that focuses on predictions of deer distributions in Ireland, particularly related to native vegetation. Such information is vital in determining which locations require regulation, management, or conservation. Here we explore four main research questions with the overall aim of identifying the factors that drive deer distributions and to reveal areas at greatest risk to biodiversity loss for the island of Ireland: 1) What is the current suitable distribution of the four deer species currently established in Ireland? 2) What are the current suitable distributions of endangered/vulnerable plant species? 3) How will the distributions of the deer species and plant species differ under future climate change scenarios? 4) Where will the deer species and plant species future distributions overlap?
Methods
Data collection
Presence data for the four deer species and 13 endangered/vulnerable plant species were obtained from the Global Biodiversity Information Facility (GBIFa-q) using the rgbif package (Chamberlain et al. Citation2021) in R version 4.1.2 (R Core Team Citation2021). Following data cleaning as of December 2021, 309 records for C. nippon, 299 records for C. elaphus, 835 records for D. dama and 281 for M. reevesi were returned, while plant species were retained if they had at least 10 occurrence records. All data observations were returned for the period after 2000 only, meaning any observations prior to then were disregarded. Plant species were identified from The Red Data List of Irish Vascular Plants (National Botanical Gardens Citation2017) and are outlined in . In total, 13 plant species were used including 5 endangered and 8 vulnerable plant species. Most plant species in The Red Data List of Irish Vascular Plants had little or no occurrence records, meaning they were omitted from the analysis.
Table 1. Information on the species used in the modelling framework along with IUCN status.
All observations that were utilized within this study came from human observations, predominantly from citizen science programmes such as the National Biodiversity Data Centre and iNaturalist. For all but four of the species, coordinate uncertainty was reported for over 90% of the observations for each species, with the majority of the species reporting uncertainty at less than 1000 m, which is the approximate spatial resolution of the SDM. P. alpina, R. islandica and V. lathyroides had over 50% of their observations with a high uncertainty; however, these represented some of the smaller sample sizes. Recent research has identified that data quantity is more important than spatial and/or other bias in the predictive performance of SDMs (Gaul et al. Citation2020). Due to the low number of observations reported for these species, we opted for data quantity over spatial bias and uncertainty introduced by using these locations where uncertainty in spatial positioning was higher than the resolution of the SDM. Please see the Supplementary Material for a breakdown of this information of the GBIF observations.
Environmental data included climate and land cover data. Climate data were obtained from the WorldClim database (Fick and Hijmans Citation2017) using the sdmpredictors package (Bosch et al. Citation2017) in R version 4.1.2 (R Core team Citation2021). Corine land cover (EEA, 2018) data was used to calculate the percentage of arable, forest, grass, pasture, urban and water cover. All variables had a spatial resolution of 30 arc seconds, approximately 1 km. To represent the future climatic predictors, the Community Climate System Model version 4 (CCSM 4.0) was selected under the Representative Concentration Pathways (RCP) 4.5 and 8.5. RCP was chosen over the Shared Socio-economic Pathways (SSP) due to the unavailability of SSP at the required scale in the WorldClim database, which would hinder reproducible research. This model is a coupled climate model for simulating the earth’s climate maintained by the Climate and Global Dynamics laboratory at the National Centre for Atmospheric Research, USA (Gent et al. Citation2011).
In this study, RCP4.5 and RCP8.5 were selected to model the future distributions of four deer species and the 13 endangered/vulnerable plant species in the years 2050 (average predicted conditions of 2041–2060). RCP 4.5 is an ‘intermediate’ scenario with an emissions peak around 2040 and a steady decline thereafter. RCP 8.5 is the basis for the ‘worst-case’ scenario were emissions to continue to increase until 2100 (Meinshausen et al. Citation2011). We opted to assume that land cover will remain consistent in the future, which is most likely a simplification of reality. While there have been recent global products of future land use, such as ESRI’s Living Atlas Land Use 2050 data, this currently is not projecting in our opinion realistic covers. For example, the product predicts the vast removal of cropland across Ireland, being replaced with grassland, the conversion of Killarney forests into swampland and the conversion of large parts of Dublin into cropland. A recent study by Kang et al. (Citation2022) found that ESRI’s current land cover data had the lowest overall accuracy when land cover was assessed. Therefore, in the absence of a realistic future projection, we feel that the assumption that land cover remains unchanged is justified and is standard practice when future land cover projections are missing in SDM research (e.g. Stanton et al. Citation2012).
Data analysis
SDMs generate a prediction of habitat suitability in both environmental and geographic space. The most common response variables used are data representing species presence-absence or presence-only (with corresponding pseudo-absences), which are coupled with a set of corresponding and georeferenced environmental variables (Franklin Citation2010). Species-environment relationships are then estimated using a range of statistical approaches that can then be extrapolated in space and time, generating a habitat suitability map that identifies the probability that the environmental conditions at that location are favourable for the study species. A categorical representation of habitat suitability is created by using a threshold that delineates between suitable-unsuitable, with habitat suitability values above and below this predicted as suitable and unsuitable, respectively. provides a methodological workflow of the SDM steps used in this study.
Figure 1. Conceptual workflow of the species distribution modelling framework implemented in this study.
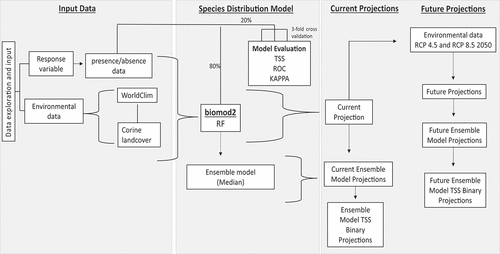
GBIF provides information on presence-only, meaning we did not have a true consideration of absence. As such, 10000 pseudo-absences (or background points) were randomly drawn from the study area, following the established standards set out by Barbet-Massin et al. (Citation2012) to ensure a binomial function could be applied to the statistical models. According to Barbet-Massin et al (Citation2012, Citation2018), the results of SDM projections can be impacted by the location of pseudo-absences. Therefore, we decided to replicate data analysis three times with different pseudo-absence selections to account for such model uncertainty.
Three different statistical models were implemented within the biomod2 package (Thuiller et al. Citation2016) in R version 4.1.2 (R Core team Citation2021). The methods we selected for initial analysis included Generalized Linear Model (GLM), Maximum Entropy (Maxent) and Random Forest (RF). Studies have shown that different modelling techniques can produce different results for the same species and datasets, with ensembles across different statistical models suggested (Araujo and Guisan Citation2006; Elith and Graham Citation2009; Thuiller Citation2004). However, the results for GLM and Maxent were poor in comparison to RF (results not shown), and when coupled with recent research that has identified there is no particular benefit to using an ensemble approach across different statistical models over individually tuned statistical models (Hao et al. Citation2020), we opted to progress this research using only RF. RF constructs many decision trees using bootstrap samples (Liaw and Wiener, Citation2002) averaging these results into one projection. By default, RF can be modelled in the form of classification and/or regression trees (Zhang et al. Citation2019) and Artificial Neural Networks (ANN) (Lek and Guégan Citation2000; Ripley Citation1996).
Models were evaluated after parameterization to justify the acceptance of projections for their intended purpose (Araujo and Guisan Citation2006). We performed 3-fold cross-validation of the data by splitting the occurrence records and pseudo-absences using an 80:20 split into training and testing data following best practice in the SDM literature when independent test data are not available (Hijmans Citation2012; O’Mahony, de la Torre Cerro, and Holloway Citation2021; Peterson et al. Citation2011). The model’s discriminatory power between suitable and unsuitable was assessed using three different metrics which can be used to provide an accurate assessment of the models. The area under the curve (AUC) statistic of a receiver operating characteristic (ROC) (Hanley and McNeil Citation1982) is a threshold-independent metric and widely used in SDM research (Franklin Citation2010). It has a value range between 0 and 1 with a value below 0.5 deemed no better than a random selection and a value of 1 representing a highly accurate model. True Skill Statistic (TSS) measures the performance of models generating presence-absence predictions with values of 0 indicating no agreement and 1 indicating perfect agreement (McHugh Citation2012). Cohen’s kappa corrects the overall accuracy of model predictions by the accuracy expected to occur by chance (Allouche, Tsoar, and Kadmon Citation2006). It ranges from values −1 to + 1, where + 1 indicates perfect agreement and values of 0 or less indicate a performance no better than random (Cohen Citation1960).
For each pseudo-absence configuration, we therefore ran three different RF models to obtain a consensus distribution, calculating an ensemble forecast distribution. This meant that for each species, we had nine possible distribution projections. The projections from these individual models were ensembled using the median habitat suitability value. The accuracy values were also reported based on the mean of the nine individual models. The ensemble model uses a continuous probability distribution which was then discretized into a binary suitable-unsuitable map using the probability threshold that maximized the TSS value (Liu et al. Citation2005).
The same SDM procedure was undertaken for the 13 plant species. To determine plant species richness under current conditions and future scenarios RCP45 and RCP85 2050, the binary maps produced for each plant species were stacked. For example, if all 13 species were projected in the same 1 km grid cell, then the value would be 13.
The Biomod.RangeSize function within the biomod2 package was used to calculate the current and future distribution in square kilometres (km2) for each species. It was also used to calculate the overlap of the four deer future distributions with each plant species future distribution.
Results
We projected the four deer species distributions with high accuracy values across the island of Ireland for all accuracy metrics (, ). The current habitat suitability of C. nippon is approximately 1257 km2 (, ) with suitable areas identified in all counties with the exception of Galway and Mayo. There are four large geographic areas of continuous suitable climate and habitat, these are the Wicklow mountains, the upland areas of County Kerry, the upland areas of West Cork, the upland area between Carlow and Wexford and some suitable areas in the Burren region of Clare. The current habitat suitability of M. reevesi is approximately 231 km2 (, ) with a suitable area mainly confined to the eastern Peninsula of Strangford Lough, Co. Down. The current habitat suitability of C. elaphus is approximately 571 km2 (, ) with suitable areas identified in all counties, although there are two large areas of continuous suitable climate and habitat, these are Killarney, Co. Kerry and Co. Donegal. The current habitat suitability of D. dama is approximately 382 km2 with suitable areas in all counties (, ). However, there are six large geographic areas of continuous suitable climate and habitat, these are Phoenix Park, Co. Dublin, the Mourne mountains, Co. Down, the Tyrone-Monaghan border region, Co. Sligo, the uplands of the Laois/Offaly border and Co. Waterford with bordering areas of southern Tipperary and Kilkenny.
Figure 2. Species richness of flora of conservation concern projected into current and future scenarios (a-c). Distributions of sika deer (d-f), muntjac deer (g-i), Red deer (j-l) and Fallow deer (m-o).
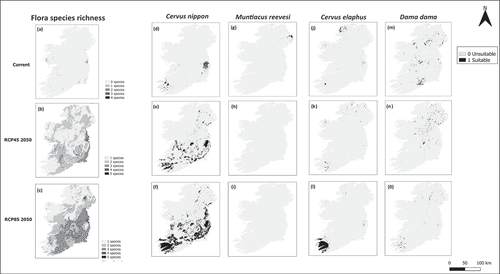
Table 2. Mean accuracy metrics for the nine iterations of the random forest models run per species. AUC=area under the curve. TSS=true skill statistics.
Table 3. The current, future RCP45 2050 and future RCP85 2050 range size for the four deer species and 13 flora species.
We also projected the distributions of the 13 flora species of conservation concern with medium to high accuracy (), with AUC scores ranging from 0.724 to 0.999, kappa scores ranging from 0.253 to 0.963 and TSS scores ranging from 0.501 to 0.999, suggesting good predictive models. While kappa had the lowest individual scores, this is not out of line with criticism of its predictive power (Pontius and Millones Citation2011). When distribution outputs were stacked to represent species richness of species of conservation concern (), the areas of high flora species richness did not overlap with the current distribution of three deer species, with D. dama being the only exception as it is the only deer species to have a current area of continuous suitable climate and habitat in Co. Dublin where current flora species richness (of conservation concern) is highest. For flora of conservation concern in general, high levels of species richness mainly occur in and/or around regions with a mixture of urban and agricultural environments such as Dublin, Waterford, Cork and Dundalk (). Other areas that have lower levels of flora species richness occurring outside these regions included the more rural regions of West Galway/Connemara, the Burren, the Shannon Estuary, Killarney, small areas of West Cork, the Three Sister Estuary (Waterford Harbour) and the East coast of Co. Wexford.
The area of high ‘at-risk’ floral species richness in the climate scenario RCP45 2050 is projected to increase (), with large areas of the east reporting a projected higher richness. These are areas where the models project a suitable climate and habitat for multiple endangered/vulnerable plant species, which occur in the south, south-east and mid-west of Ireland (). The climate scenario RCP85 2050 predicts a substantial increase in plant species richness across the island compared to current plant species richness (). Areas with the highest levels of species richness include large parts of the east and south of the island. Also, areas of high flora species richness are not just confined to the urban areas.
The area projected as suitable for C. nippon substantially increases from the current 1,257 km2 when projecting into future climate scenarios, with suitable areas of 6,392 km2 and 14,446 km2 for RCP45 and RCP85, respectively (). The climate scenario RCP45 2050 reveals that the habitat of C. nippon will increase, expanding its current primary locations, such as the Wicklow mountains, the upland areas of County Kerry, the upland areas of west Cork, the upland areas of Carlow/Wexford border and the Co. Clare region. Also indicating more large areas of continuous suitable climate and habitat in the mid-west, midlands, southern, eastern and north-eastern parts of the island (). The climate scenario RCP85 2050 reveals that the habitat of C. nippon will substantially increase, expanding its current primary locations and with an increase in areas of suitable climate and habitat in all regions of the island with the exception of the northwest ().
For M. reevesi, there are no suitable areas projected for both future climate scenarios (). The area projected as suitable for C. elaphus decreases from the current 571km2 to 392km2 for the future climate scenario RCP45, but has a large increase for the future climate scenario RCP85 with an area of 2835km2 (). The climate scenario RCP85 2050 reveals that the habitat of C. elaphus will be substantially increased, with a large increase in Kerry/West Cork region and a slight decrease in current primary locations (). The area projected as suitable for D. dama decreases from the current 1,459km2 when projecting into future climate scenarios, with suitable areas of 1,088km2 and 543km2 for RCP45 and RCP85, respectively (). The climate scenario RCP45 2050 reveals that the habitat of D. dama will decrease, losing some of its current primary locations, such as Co. Waterford with bordering areas of southern Tipperary and Kilkenny, and Phoenix Park, Co. Dublin. However, other primary locations have a smaller decrease in size (). The climate scenario RCP85 2050 reveals that the habitat of D. dama will be substantially decreased, losing some of its current primary locations and a new suitable area in the Kerry/West Cork region. With the other suitable areas being in small areas of the midlands and North-East of the island ().
Out of the 13 plant species, four plant species have a range increase from their current range for both future climate scenarios. These species are A. schoenoprasum, D. cantabrica, H. secalinum and L. subbiflorus, with all species having a large increase compared to their predicted current range size (). There are six plant species, C. trachelium, E. mackaiana, G. sylvaticum, P. alpina, T. subterraneum and V. lathyroides which have no suitable geographic locations for both future climate scenarios with a projection of extinction reported by our models. Three plant species have a decrease in both future climate scenarios. These species are C. cyanus, F. alnus and R. islandica ().
When the distributions of C. nippon and the 13 plant species are considered together, all six of the flora species with a predicted future area of suitability are predicted to have some overlap with the future range of C. nippon (see ); however, the areas of overlap vary in size. For example, F. alnus is the only flora species whose future range size is predicted to have a 100% overlap with C. nippon for both future climate scenarios. For the future scenario RCP45 2050, R. islandica had a predicted overlap of 18.18%, D. cantabrica 11.59%, A. schoenoprasum 9.99%, H. secalinum 9.20% and L. subbiflorus 7.55% (). For future scenario RCP85 2050, D. cantabrica had an predicted overlap of 32.99%, H. secalinum 25.04%, A. schoenoprasum 21.39%, L. subbiflorus 17.07% and R.islandica 16.67% ().
Table 4. The future RCP45 2050 overlap of the four Deer species in km2 and per cent with the 13 flora species.
Table 5. The future RCP85 2050 overlap of the four Deer species in km2 and per cent with the 13 flora species.
When the distributions of C. elaphus and the 13 plant species are considered together, five of the flora species with a predicted future area of suitability are predicted to have some overlap with the future range of C. elaphus (see ); however, the areas of overlap are not extensive. F. alnus is the only flora species whose future range size is predicted to have an extensive overlap with C. elaphus for both future climate scenarios with a 100% predicted overlap in scenario RCP45 2050 and a predicted overlap of 92.86% in scenario RCP85 2050 (). The future RCP45 2050 distribution for H. secalinum has no predicted overlap with C. elaphus and the lowest predicted overlap of 0.29% for the future RCP85 2050 distribution. All the other flora species are predicted to have an overlap of less than 3.5% with C. elaphus for both future scenarios, largely due to the restricted south-west distribution of C. elaphus under future climate scenarios.
When the distributions of D. dama and the 13 plant species are considered together, four of the flora species with a predicted future area of suitability are predicted to have some overlap with the future range of D. dama (see ); however, the areas of overlap are not extensive, with all the flora species predicted to have an overlap of less 3% for both future climate scenarios (). However, there are two species, C. cyanus and R. islandica who are both predicted to have no future overlap with D. dama for both future climate scenarios. Although, the future RCP45 2050 distribution for F. alnus has the highest predicted overlap of 100% with D. dama; the RCP85 2050 distribution also has the highest predicted overlap for all flora species with a predicted overlap of 92.86%.
Discussion
The main aim of this research was to identify the geographic distribution of the four deer species currently found on the island of Ireland (three of which are non-native) and to reveal areas that may be at risk of biodiversity loss, focusing on endangered and vulnerable plant species. In doing so, we aimed to support recent calls in the literature to support the decision-making spectrum of related management and conservation dilemmas on the flora in the non-native ranges of deer species (Carden et al. Citation2010; Saggiomo, Esattore, and Picone Citation2020). This research is to our knowledge the first attempt to use a species distribution modelling framework to estimate the current and future distributions of deer and plant species of conservation concern at an all-Ireland level. We projected species distributions to high accuracy levels (), with large areas of currently suitable habitat for deer species across counties. Future climate scenarios identified an increase in sika deer, while muntjac and fallow deer are projected to reduce their distributions under climate change. Red deer appeared to decline under the moderate climate change scenario but increase under the worst-case projection. However, climate change projections appeared to project the local extinction of six (out of 13) endangered and vulnerable plant species (). Moreover, while an increase in area was identified for four plant species, several of these areas overlap with the future projections of deer species (), which could cause further pressures on these ‘at-risk’ plant species.
Our results are incongruent with the current thinking that all deer species will continue to expand their habitat in Ireland (Carden et al. Citation2010). While we projected an expansion of sika under both RCP scenarios, which is congruent with predictions (Carden et al. Citation2010), we identified a decrease in range size for fallow and muntjac deer, and a decrease under RCP45 for red deer, albeit with an increase under RCP85. This does not imply that all deer species will not be present in currently occupied areas at the time of this prediction, it simply forecasts the most suitable habitat for the modelled species in relation to the environmental variables of that period. A central assumption of SDM assumes that a species is at equilibrium with its environment, which is where it occurs in all suitable sites, while being absent from all unsuitable sites (Araújo, Pearson, and Rahbek Citation2005). However, introduced species often violate this assumption as occurrence records of the species do not always inform its potential distribution (Gallien et al. Citation2012). This could have been improved by using global distribution records from the native ranges (O’Mahony, de la Torre Cerro, and Holloway Citation2021); however, given the length of time the deer species have been present on the island of Ireland (e.g. sika were introduced in the 1860s – Purser et al. 2009), these species are most likely in equilibrium with the environment. As such they are no longer expanding their ranges, which may account for the reduction in range size projected by our models. Muntjac are the species that has been present on the island for the shortest period, meaning the low habitat suitability projected for this species may be an artefact of the assumed equilibrium, and as such should be treated with caution.
Moreover, we opted to threshold our habitat suitability projections from a continuous probability to a binary output. This was to provide the framework to test the areas of overlap with ‘at-risk’ plant species. While there is a low probability of occurrence in these regions based on climate and land cover, it is feasible for the species to be found there. This is particularly relevant for invasive species, which are often released and dispersed through less-than-ideal environmental conditions (Barbet-Massin et al. Citation2018).
Despite this, expansion of invasive deer species in other countries has slowed, with muntjac range expansion dropping to 2–3% in England from 2011 to 2016 compared to an expansion of almost 12% in 2007 (Ward, Richardson, and Mergeay Citation2021). When this concept is coupled with the lower-than-average forest cover in Ireland compared to other European countries (<1% at the turn of the century – Mitchell Citation2000), it is likely that deer species in Ireland are inhabiting areas of lower suitability, or at the very least climatically different areas than it may favour given complete forest coverage. We opted to keep land cover consistent in the models, in part due to a lack of reliable alternatives, which is in line with the SDM literature (Stanton et al. Citation2012). However, with active afforestation policies in Ireland (i.e. Forest Action Plan 2022) that are aiming to increase the area of forests as well as the native components of these woodlands, it is likely that our models are an underestimation of future geographic distributions of all deer species. Despite this, our models provide a baseline of the minimum potential impact to ‘at-risk’ plant species, and as such will support and inform future management strategies.
We projected a local extinction of six ‘at-risk’ plant species under future climate scenarios. The local extinction of any species could have cascading effects on the ecosystem, but our models have projected that almost 50% of the plant species considered here may go extinct (). Given that the 13 species included in this analysis were the only ones to reach a minimum number of observations in GBIF, the reality is that there may be a much higher rate of extinction for flora species of conservation concern, as these are in theory the most common of the endangered and vulnerable plant species. As such, there is a pressing concern for research to be directed towards understanding how these species will respond to climate change. It should be noted here that the drivers of vascular plant extinctions are nuanced, with numerous pressures globally and within Ireland (Nic Lughadha et al. Citation2020; O’Rourke, Lughadha, and Bacon Citation2022). Therefore, the potential for extinction based on these climate models must be considered alongside many other complex and interlinked ecosystem functions and pressures, such as habitat loss, exploitation, intensification of agriculture and non-native herbivory.
Contrary to the extinction risk, we did identify that some ‘at-risk’ species are projected to increase their geographically suitable area. ‘At-risk’ plant species richness appeared to increase nationally, with some of the highest projected richness occurring in urban and agricultural areas (). We posit here the importance of the landscape matrix (i.e. multiple habitats) and urban wildflower meadows that have become increasingly popular in city centres in recent years to allow citizens to observe and enjoy the pleasures of nature (Bretzel et al. Citation2016) and more recently support the biodiversity crisis (Mody et al. Citation2020). These green spaces help to mitigate the negative impacts of urbanization on biodiversity such as habitat loss, fragmentation, decreased population size and loss of genetic diversity across a multitude of species (Lambert, Cawkwell, and Holloway Citation2021; Vega and Küffer Citation2021). Moreover, Ireland has been reported as a location whereby species have expanded their geographic ranges in recent years in response to climate change (Bellard et al. Citation2018; Donnelly et al. Citation2014), and while such research warrants further investigation, our findings suggest that certain plant species that are currently restricted locally on the island may benefit from changes to the existing climate.
For example, the Biodiversity Audit of the Áras an Uachtaraín in Dublin in 2019–2020 identified 297 species of plant in the 130-acre site (Gaughran and Stout Citation2020). While significantly larger than the average urban greenspace in Ireland, the importance of these refuges to native species is clear, with the dispersal of plants from the plantation sites to support urban range expansion well documented (Gilbert and Anderson Citation1998). Moreover, given the avoidance of urban centres by deer species in Ireland, urban areas could provide a refuge for many plant species, including endangered and vulnerable species to not only persist, but thrive under future climates. Almost 50% of the plant species included in this analysis are available to purchase from horticulturalists, suggesting this urban refuge may support these species.
Conversely, it is also important to consider the areas predicted to have a low richness of ‘at-risk’ species. Linders et al. (Citation2019) state that one of the most effective mediation strategies for invasive species is to support vegetation abundance, species richness and diversity, so that any grazing pressure is ‘shared’ among species. It can be seen from that areas of lower species richness under the two RCP scenarios are found in the south-west, including Co. Kerry and Cork, the north-west and large areas of Northern Ireland. When these outputs are compared with the future outputs of C. nippon and C. elaphus distributions (), the area in the south-west of Ireland can be identified as an area that may need to be explicitly monitored due to the presence of one or two ‘at-risk’ plant species and a large geographic distribution of deer. Therefore, any management strategy should look to ensure that there is a diverse range of vegetation available, such that certain species are not subject to increased and unsustainable grazing. This is particularly pertinent given the multifaceted and nuanced implications of forest management, with Hawthorne, Colombaroli, and Mitchell (Citation2021) suggesting that the complete exclusion of grazing by deer in the region would be undesirable due to the potential encroachment of woody species leading to the decline of woodland specialists.
There was one plant species predicted to have a 100% overlap with three deer species under climate change. F.alnus is predicted to occur in the west of Ireland in and around current suitable areas in the Burren, Killarney and West Cork Region. This plant is mainly found in damp, peaty soil and the wooded areas on limestone soils (Goodwin Citation1943) some of which are land covers deer species do not frequent. Therefore, it may be possible that despite high overlap with deer species, F.alnus may occur in land cover less suitable; however, they should be monitored regularly for expansion of their ranges.
Based on the discrepancy of the findings, it is difficult to ascertain the exact role of climate or non-native pressure on the varying fortunes of the ‘at-risk’ plant species. While our models suggest extinction for 50% of the plant species studied here based on climate, which is congruent with other global studies (Román-Palacios and Wiens Citation2020), it is clear that the presence and overlap of non-native species could further propagate such considerations. Moreover, the importance of restoring functional ecosystems or trophic rewilding has become increasingly important within the conservation debate (Svenning et al. Citation2016), with studies suggesting that restoring native animal populations to their functional roles can enhance natural carbon capture and storage, and potentially negate climate warming (Schmitz et al. Citation2023). Extinct fauna in Ireland includes the grey wolf, the great elk and the brown bear, to name just a few (Hickey Citation2013), meaning that the native and non-native deer within the (largely restricted) forest ecoystems are not the top-herbivore and are currently uncontrolled by any natural predator, which further propogates their risk to endangered flora. Therefore, a diversification of plant flora in a region, coupled with a ‘rewilding’ of the ecosystems is currently considered the most effective management practice to support the impact of non-native deer species on native plants.
We only considered abiotic variables in model parameterization, including climate and habitat. However, deer are herbaceous species whose distribution is dependent on food sources. We did not explicitly include biotic factors, such as the distribution of food sources or competition, in the model as has been suggested (Peterson et al. Citation2020; Soberon and Peterson Citation2005). In part this was due to the fact that we wanted to compare the distributions of endangered and vulnerable plant species with those of the deer species, and any inclusion of vegetation in parameterization of these distributions would have introduced circularity into the model. However, given the importance of climatic variables in determining deer distributions (results not shown), it is possible that such considerations were incorporated in the model as latent variables (Schymanski et al. Citation2013). While this is important to note, so too is the fact that perfect statistical independence between environmental variables is perhaps unrealistic of real-world variables, with propagation of environmental variables most likely present in all SDM research (Holloway and Miller Citation2017). It is infeasible to omit all variables based on the assumption that they may already be included elsewhere in the model, and when coupled with the high accuracy of the models (), our overarching aim to accurately predict future distributions of these species has been achieved.
Further, we also assumed unlimited dispersal capacity of all species, which is in line with species who have high dispersal capacities or the potential for translocation by humans (Holloway, Miller, and Gillings Citation2016). We also note the importance of the low number of occurrences. While this has been found to impact distribution projections, research has found that models can be reliably and accurately predicted using as little as three observations (van Proosdij et al. Citation2016). To ensure a minimum standard of reliability, we opted to use a minimum of ten observations for the plant species. However, of the 128 species listed on the Red Data Plant List, only 13 had a minimum of ten sightings on GBIF. Future research collecting verified plant species locations that are made available through open data repositories is needed to improve model calibration and support biodiversity conservation and supports recent calls for more comprehensive surveys and biodiversity audits in Ireland (Gaughran and Stout Citation2020).
Due to these limitations in data availability, some of our species reported high uncertainty in the spatial positioning (see Supplementary Material). As stated, recent research has identified that data quantity is more important than spatial bias and uncertainty in the predictive performance of SDMs (Gaul et al. Citation2020), meaning we opted to use these data points to ensure we could undertake this research. Despite these caveats, we do report high accuracy values (), allowing confidence in the discussion of the important environmental drivers of the plant species distributions. Moreover, of the three species that reported high uncertainty, only P. islandica has a lower than average accuracy metric, meaning the uncertainty has not appeared to overly impact model predictions. However, both P. aplina and V. lathyroides are two of the species projected to go extinct, but represent two of the smallest current distributions of plants. More research and data are therefore needed to investigate whether these species are projected to become extinct, or whether this is an artefact of data availability and their cryptic nature. Given that the 13 species included in this analysis were the only ones to reach a minimum number of observations, the reality is that there may be a much higher rate of extinction risk for flora species of conservation concern.
Finally, there persists a need to explore habitat suitability of deer and plant species in conjunction with other measures of biodiversity, such as density and abundance. New methods, such as Poisson point process models, are emerging as suitable tools to predict the distribution and abundance of species from presence-only data (Renner and Warton Citation2013; Schank et al. Citation2017; Warton and Shepherd Citation2010). Similary, new data fusion techniques are being used to combine presence-only data and presence-absence across multiple spatial scales that are resolving some of the issues related to data sparsity (Morera-Pujol et al. Citation2022). Given the uncertain relationships identified, future studies should focus on developing predictions of both the distribution and density of deer to inform forest management and biodiversity conservation.
Conclusion
It has been identified that over 1 million species are threatened with accelerated extinction (Díaz et al. Citation2019), with the spread of invasive species ranked as one of the primary drivers of this loss. Here we utilized a species distribution modelling framework, which is a prominent GIScience technique, to assess the current and future habitat suitability of four deer species (three non-native and one native) and 13 endangered/vulnerable plant species. To our knowledge, this is the first time this applied research has been undertaken at an all-Ireland scale. We then used the predicted future distributions to identify how much the deer and plant species distribution will overlap. We predicted large areas of current suitable habitat for the deer species across all counties. Future climate scenarios identified an increase in sika deer, while fallow deer are projected to reduce their distribution under climate change and our results predict a local extinction for muntjac. Also, our results predict the local extinction of six (out of 13) endangered and vulnerable plant species. Moreover, while an increase in area was identified for four plant species, several of these areas overlap with the future projections of deer species, which could cause further pressures on these ‘at-risk’ plant species. However, ‘at-risk’ plant species richness appeared to increase nationally, with some of the highest projected richness occurring in urban and agricultural areas. Finally, our results should support the conservation of these endangered/vulnerable plant species from the negative impact of native/non-native deer species and can be used to mediate the impact on the ‘at-risk’ plant species through different conservation and management measures. Not only has this research provided practitioners with a product with which to identify areas to prioritize conservation activities, saving their time, resources and research efforts, it has also resulted in a better understanding of the effects of climate change on projected distributions and extinction rates.
Acknowledgement
We would like to thank the editors, reviewers, and Astrid Wingler for their comments and suggestions.
Disclosure statement
No potential conflict of interest was reported by the author(s).
References
- Akashi, N., and T. Nakashizuka. 1999. “Effects of Bark-Stripping by Sika Deer (Cervus nippon) on Population Dynamics of a Mixed Forest in Japan.” Forest Ecology and Management 113 (1): 75–82. https://doi.org/10.1016/S0378-11279800415-0.
- Allouche, O., A. Tsoar, and R. Kadmon. 2006. “Assessing the Accuracy of Species Distribution Models: Prevalence, Kappa and the True Skill Statistic (TSS).” Journal of Applied Ecology 43 (6): 1223–1232. https://doi.org/10.1111/j.1365-2664.2006.01214.x.
- Araújo, M. B., M. Cabeza, W. Thuiller, L. Hannah, and P. H. Williams. 2004. “Would Climate Change Drive Species Out of Reserves? An Assessment of Existing Reserve‐Selection Methods.” Global Change Biology 10 (9): 1618–1626. https://doi.org/10.1111/j.1365-2486.2004.00828.x.
- Araujo, M. B., and A. Guisan. 2006. “Five (Or So) Challenges for Species Distribution Modelling.” Journal of Biogeography 33 (10): 1677–1688. https://doi.org/10.1111/j.1365-2699.2006.01584.x.
- Araújo, M. B., R. G. Pearson, and C. Rahbek. 2005. “Equilibrium of species’ Distributions with Climate.” Ecography 28 (5): 693–695.h. https://doi.org/10.1111/j.2005.0906-7590.04253.x.
- Bailey, J. 2013. “The Japanese Knotweed Invasion Viewed as a Vast Unintentional Hybridisation Experiment.” Heredity 110 (2): 105–110. https://doi.org/10.1038/hdy.2012.98.
- Barbet-Massin, M., F. Jiguet, C. Albert, and W. Thuiller. 2012. “Selecting Pseudo-Absences for Species Distribution Models: How, Where and How Many?” Methods in Ecology and Evolution 3 (2): 327–338. https://doi.org/10.1111/j.2041-210X.2011.00172.x.
- Barbet-Massin, M., Q. Rome, C. Villemant, F. Courchamp, and L. Bosso. 2018. “Can Species Distribution Models Really Predict the Expansion of Invasive Species?” PLOS ONE 13 (3): 0193085. https://doi.org/10.1371/journal.pone.0193085.
- Bellard, C., C. Bernery, and C. Leclerc. 2021. “Looming Extinctions Due to Invasive Species: Irreversible Loss of Ecological Strategy and Evolutionary History.” Global Change Biology 27 (20): 4967–4979. https://doi.org/10.1111/gcb.15771.
- Bellard, C., J. M. Jeschke, B. Leroy, and G. M. Mace. 2018. “Insights from Modeling Studies on How Climate Change Affects Invasive Alien Species Geography.” Ecology and Evolution 8 (11): 5688–5700. https://doi.org/10.1002/ece3.4098.
- Bosch, S., L. Tyberghein, K. Deneudt, F. Hernandez, O. De Clerck, and A. Syphard. 2017. “In Search of Relevant Predictors for Marine Species Distribution Modelling Using the MarineSpeed Benchmark Dataset.” Diversity and Distributions 24 (2): 144–157. https://doi.org/10.1111/ddi.12668.
- Bretzel, F., F. Vannucchi, D. Romano, F. Malorgio, S. Benvenuti, and B. Pezzarossa. 2016. “Wildflowers: From Conserving Biodiversity to Urban Greening—A Review.” Urban Forestry & Urban Greening 20:428–436. https://doi.org/10.1016/j.ufug.2016.10.008.
- Burch, C., R. Loraamm, T. Unnasch, and J. Downs. 2020. “Utilizing Ecological Niche Modelling to Predict Habitat Suitability of Eastern Equine Encephalitis in Florida.” Annals of GIS 26 (2): 133–147. https://doi.org/10.1080/19475683.2020.1730962.
- Burkitt, T. D. 2009. “A comparison of ecology between sympatric native red deer (Cervus elaphus Linnaeus 1758) and introduced Japanese sika deer (Cervus nippon nippon Temminck 1836) populations in southwest Ireland“ ( Doctoral dissertation). Manchester Metropolitan University.
- Carden, R. F., C. M. Carlin, F. Marnell, D. Mcelholm, J. Hetherington, and M. P. Gammell. 2010. “Distribution and Range Expansion of Deer in Ireland.” Mammal Review 41 (4): 313–325. https://doi.org/10.1111/j.1365-2907.2010.00170.x.
- Chamberlain, S., V. Barve, D. Mcglinn, D. Oldoni, P. Desmet, L. Geffert, and K. Ram. 2021. “Rgbif: Interface to the Global Biodiversity Information Facility API.” R package version 3.6.0.
- Chen, I. C., J. K. Hill, R. Ohlemüller, D. B. Roy, and C. D. Thomas. 2011. “Rapid Range Shifts of Species Associated with High Levels of Climate Warming.” Science: Advanced Materials and Devices 333 (6045): 1024–1026. https://doi.org/10.1126/science.1206432.
- Cohen, J. 1960. “A Coefficient of Agreement for Nominal Scales.” Education and Psychological Measurement 20 (1): 37–46. https://doi.org/10.1177/001316446002000104.
- Cooke, A. S. 2021. “Colonisation, Impacts in Conservation Woodland and Management of Reeves’ Muntjac (Muntiacus reevesi) in an English County.” European Journal of Wildlife Research 67 (3): 1–7. https://doi.org/10.1007/s10344-021-01480-8.
- Cooke, A. S., and L. Farrell. 2001. “Impact of Muntjac Deer (Muntiacus reevesi) at Monks Wood National Nature Reserve, Cambridgeshire, Eastern England.” Forestry 74 (3): 241–250. https://doi.org/10.1093/forestry/74.3.241.
- Crispell, J., S. Cassidy, K. Kenny, G. McGrath, S. Warde, H. Cameron, G. Rossi, et al. 2020. “Mycobacterium Bovis Genomics Reveals Transmission of Infection Between Cattle and Deer in Ireland.” Microbial Genomics 6 (8). https://doi.org/10.1099/mgen.0.000388.
- Cunningham, C. X., G. L. Perry, D. M. Bowman, D. M. Forsyth, M. M. Driessen, M. Appleby, B. W. Brook, et al. 2022. “Dynamics and Predicted Distribution of an Irrupting ‘Sleeper’population: Fallow Deer in Tasmania.” Biological Invasions 24: 1131–1147.
- Díaz, S., J. Settele, E. S. Brondízio, H. T. Ngo, M. Guèze, J. Agard, A. Arneth, et al. 2019. “Summary for Policymakers of the Global Assessment Report on Biodiversity and Ecosystem Services of the Intergovernmental Science-Policy Platform on Biodiversity and Ecosystem Services.” Intergovernmental Science-Policy Platform on Biodiversity and Ecosystem Services 56.
- Dolman, P., R. Fuller, R. Gill, D. Hooton, and R. Tabor. 2010. “Escalating Ecological Impacts of Deer in Lowland Woodland.” British Wildlife 21 (4): 242.
- Donnelly, A., O. Crowe, E. Regan, S. Begley, and A. Caffarra. 2014. “The Role of Citizen Science in Monitoring Biodiversity in Ireland.” International Journal of Biometeorology 58 (6): 1237–1249. https://doi.org/10.1007/s00484-013-0717-0.
- Ehrlén, J., W. F. Morris, and Y. Buckley. 2015. “Predicting Changes in the Distribution and Abundance of Species Under Environmental Change.” Ecology Letters 18 (3): 303–314. https://doi.org/10.1111/ele.12410.
- Elith, J., and C. H. Graham. 2009. “Do They? How Do They? WHY Do They Differ? On Finding Reasons for Differing Performances of Species Distribution Models.” Ecography 32 (1): 66–77. https://doi.org/10.1111/j.1600-0587.2008.05505.x.
- Fick, S. E., and R. J. Hijmans. 2017. “WorldClim 2: New 1‐Km Spatial Resolution Climate Surfaces for Global Land Areas.” International Journal of Climatology 37 (12): 4302–4315. https://doi.org/10.1002/joc.5086.
- Franklin, J. 2010. Mapping Species Distributions: Spatial Inference and Prediction. Cambridge, UK: Cambridge University Press.
- Fuller, R. J., and R. M. Gill. 2010. The Spread of Non-Native Muntjac and Fallow Deer: A Problem for Lowland Woodland Birds. BOU ProcNet. https://www.researchgate.net/profile/Robin-Gill-2/publication/267413401_The_spread_of_non-native_Muntjac_and_Fallow_Deer_a_problem_for_lowland_woodland_birds/links/54b3c53f0cf26833efced5ee/The-spread-of-non-native-Muntjac-and-Fallow-Deer-a-problem-for-lowland-woodland-birds.pdf
- Gallien, L., R. Douzet, S. Pratte, N. E. Zimmermann, and W. Thuiller. 2012. “Invasive Species Distribution Models–How Violating the Equilibrium Assumption Can Create New Insights.” Global Ecology and Biogeography 21 (11): 1126–1136. https://doi.org/10.1111/j.1466-8238.2012.00768.x.
- Gaughran, A., and J. Stout. 2020. “Biodiversity Audit at Áras an Uachtaráin. Final Report.“ Accessed 1 May 2022. https://phoenixpark.ie/wp-content/uploads/2020/11/aras-an-uachtarain-biodiveristy-audit-final-report.pdf.
- Gaul, W., D. Sadykova, H. J. White, L. Leon-Sanchez, P. Caplat, M. C. Emmerson, and J. M. Yearsley. 2020. “Data Quantity is More Important Than Its Spatial Bias for Predictive Species Distribution Modelling.” PeerJ 8:e10411. https://doi.org/10.7717/peerj.10411.
- GBIF.2021a. “GBIF Occurrence Download.“ Accessed 7 March 2022. https://doi.org/10.15468/dl.cb2hsf.
- GBIF. 2021b. “GBIF Occurrence Download.“ Accessed 7 March 2022. https://doi.org/10.15468/dl.qm4v2t.
- GBIF.2022a. “GBIF Occurrence Download.“ Accessed December 2022. https://doi.org/10.15468/dl.92jj3s.
- GBIF. 2022b. “GBIF Occurrence Download.“ Accessed December 2022. https://doi.org/10.15468/dl.be7krr.
- GBIF. 2022c. “GBIF Occurrence Download.“ Accessed December 2022. https://doi.org/10.15468/dl.yc59mu.
- GBIF. 2022d. “GBIF Occurrence Download.“ Accessed December 2022. https://doi.org/10.15468/dl.qgemaq.
- GBIF. 2022e. “GBIF Occurrence Download.” Accessed December 2022. https://doi.org/10.15468/dl.ucepps.
- GBIF. 2022f. “GBIF Occurrence Download.“ Accessed December 2022. https://doi.org/10.15468/dl.zrhwa2
- GBIF. 2022g. “GBIF Occurrence Download.“ Accessed December 2022. https://doi.org/10.15468/dl.nvnscr.
- GBIF. 2022h. “GBIF Occurrence Download.“ Accessed December 2022. https://doi.org/10.15468/dl.ah5fq7.
- GBIF.2022i. “GBIF Occurrence Download.“ Accessed December 2022. https://doi.org/10.15468/dl.waw9qx.
- GBIF. 2022j. “GBIF Occurrence Download.“ Accessed December 2022. https://doi.org/10.15468/dl.dcatgu.
- GBIF. 2022k. “GBIF Occurrence Download.“ Accessed December 2022. https://doi.org/10.15468/dl.gtnw2a.
- GBIF. 2022l. “GBIF Occurrence Download.“ Accessed December 2022. https://doi.org/10.15468/dl.txwcpa.
- GBIF. 2022m. “GBIF Occurrence Download.“ Accessed December 2022. https://doi.org/10.15468/dl.sz5x6g.
- GBIF. 2022n. “GBIF Occurrence Download.“ Accessed December 2022. https://doi.org/10.15468/dl.6qzmh7.
- GBIF. 2022o. “GBIF Occurrence Download.“ Accessed 7 March 2022. https://doi.org/10.15468/dl.2a5f6c.
- Gélin, U., M. Keller, V. de Beaupuis, R. Nowak, F. Lévy, and Y. Locatelli. 2019. “Impact of Hybridization Between Sika and Red Deer on Phenotypic Traits of the Newborn and Mother–Young Relationships.” Animal Behaviour 158:65–75. https://doi.org/10.1016/j.anbehav.2019.09.016.
- Gent, P. R., G. DANABASOGLU, L. J. Donner, M. M. Holland, E. C. Hunke, S. R. Jayne, D. M. Lawrence, et al. 2011. “The Community Climate System Model Version 4.” Journal of Climate 24 (19): 4973–4991. https://doi.org/10.1175/2011JCLI4083.1.
- Gilbert, O. L., and P. Anderson. 1998. Habitat Creation and Repair. Oxford, UK: Oxford University Press.
- Goodwin, H. 1943. “Frangula Alnus Miller.” The Journal of Ecology 31 (1): 77–92. https://doi.org/10.2307/2256793.
- Hanley, J. A., and B. J. McNeil. 1982. “The Meaning and Use of the Area Under a Receiver Operating Characteristic (ROC) Curve. Distributions and Projecting Potential Future Shifts Under.” Radiology 143 (1): 29–33. https://doi.org/10.1148/radiology.143.1.7063747.
- Hao, T., J. Elith, J. J. Lahoz‐Monfort, and G. Guillera‐Arroita. 2020. “Testing Whether Ensemble Modelling is Advantageous for Maximising Predictive Performance of Species Distribution Models.” Ecography 43 (4): 549–558. https://doi.org/10.1111/ecog.04890.
- Hawthorne, D., D. Colombaroli, and F. J. Mitchell. 2021. “Palaeoecological Records as a Guide for Fire Management in Killarney National Park, Ireland.“ Proceedings of the Geologists’, Association. https://doi.org/10.1016/j.pgeola.2021.09.004
- Hickey, K. R. 2013. Wolves in Ireland: A Natural and Cultural History. Ireland: Four Courts Press.
- Hijmans, R. J. 2012. “Cross-Validation of Species Distribution Models: Removing Spatial Sorting Bias and Calibration with a Null Model.” Ecology 93:679–688. https://doi.org/10.1890/11-0826.1.
- Holloway, P., and J. A. Miller. 2017. “A Quantitative Synthesis of the Movement Concepts Used within Species Distribution Modelling.” Ecological Modelling 356:91–103. https://doi.org/10.1016/j.ecolmodel.2017.04.005.
- Holloway, P., J. A. Miller, and S. Gillings. 2016. “Incorporating Movement in Species Distribution Models: How Do Simulations of Dispersal Affect the Accuracy and Uncertainty of Projections?” International Journal of Geographical Information Science 30 (10): 2050–2074. https://doi.org/10.1080/13658816.2016.1158823.
- Homolka, M., and M. Heroldová. 2003. “Impact of Large Herbivores on Mountain Forest Stands in the Beskydy Mountains.” Forest Ecology and Management 181 (1–2): 119–129. https://doi.org/10.1016/S0378-11270300121-X.
- Husheer, S. W., and C. M. Frampton. 2005. “Fallow Deer Impacts on Wakatipu Beech Forest.” New Zealand Journal of Ecology 29 (1): 83–94.
- Huxel, G. R. 1999. “Rapid Displacement of Native Species by Invasive Species: Effects of Hybridization.” Biological Conservation 89 (2): 143–152. https://doi.org/10.1016/S0006-32079800153-0.
- Iida, T., M. Soga, and S. Koike. 2016. “Effects of an Increase in Population of Sika Deer on Beetle Communities in Deciduous Forests.” ZooKeys 625:67. https://doi.org/10.3897/zookeys.625.9116.
- Ikeda, T., H. Takahashi, T. Yoshida, H. Igota, and K. Kaji. 2013. “Evaluation of Camera Trap Surveys for Estimation of Sika Deer Herd Composition.” Mammal Study 38 (1): 29–33. https://doi.org/10.3106/041.038.0103.
- Kaji, K., T. Koizumi, and N. Ohtaishi. 1988. “Effects of Resource Limitation on the Physical and Reproductive Condition of Sika Deer on Nakanoshima Island, Hokkaido.” Acta Theriologica 33 (13): 187–208, plate 15. https://doi.org/10.4098/AT.arch.88-15.
- Kang, J., X. Yang, Z. Wang, H. Cheng, J. Wang, H. Tang, Y. Li, Z. Bian, and Z. Bai. 2022. “Comparison of Three Ten Meter Land Cover Products in a Drought Region: A Case Study in Northwestern China.” Land 11 (3): 427. https://doi.org/10.3390/land11030427.
- Kato, M., and Y. Okuyama. 2004. “Changes in the Biodiversity of a Deciduous Forest Ecosystem Caused by an Increase in the Sika Deer Population at Ashiu, Japan.” Control Biology Lab Kyoto University 29:437–448.
- Kelly, D. J., E. Mullen, and M. Good. 2021. “Bovine Tuberculosis: The Emergence of a New Wildlife Maintenance Host in Ireland.” Frontiers in Veterinary Science 8. https://doi.org/10.3389/fvets.2021.632525.
- Laffan, S. W., A. K. Skidmore, and J. Franklin. 2014. “Species Distribution and Diversity, Habitat Selection and Connectivity: Introduction to the Third Special Issue on Spatial Ecology.” International Journal of Geographical Information Science 28 (8): 1527–1530. https://doi.org/10.1080/13658816.2014.902950.
- Lambert, L., F. Cawkwell, and P. Holloway. 2021. “The Importance of Connected and Interspersed Urban Green and Blue Space for Biodiversity: A Case Study in Cork City, Ireland.” Geographies 1 (3): 217–237. https://doi.org/10.3390/geographies1030013.
- Lek, S., and J. Guégan. 2000. “Artificial Neural Networks as a Tool in Ecological Modelling, an Introduction.” Ecological Modelling 120 (2–3): 65–73. https://doi.org/10.1016/S0304-3800(99)00092-7.
- Liaw, A., and M. Wiener. 2002. “Classification and Regression by RandomForest.” R news 2 (3): 18–22 .
- Linders, T. E. W., U. Schaffner, R. Eschen, A. Abebe, S. K. Choge, L. Nigatu, P. R. Mbaabu, H. Shiferaw, E. Allan, and P. Alpert. 2019. “Direct and Indirect Effects of Invasive Species: Biodiversity Loss is a Major Mechanism by Which an Invasive Tree Affects Ecosystem Functioning.” Journal of Ecology 107 (6): 2660–2672. https://doi.org/10.1111/1365-2745.13268.
- Liu, C., P. M. Berry, T. P. Dawson, and R. G. Pearson. 2005. “Selecting Thresholds of Occurrence in the Prediction of Species Distributions.” ECOGRAPHY 28 (3): 385–393. https://doi.org/10.1111/j.0906-7590.2005.03957.x.
- Liu, Y., and M. Nieuwenhuis. 2014 An Analysis of Habitat-Use Patterns of Fallow and Sika Deer Based on Culling Data from Two Estates in Co. Wicklow. Irish Forestry Journal 71 (1&2). https://journal.societyofirishforesters.ie/index.php/forestry/article/view/10132
- Liu, Y., M. Nieuwenhuis, and A. McCullagh. 2018. “The Effect of Roadside Land-Use on the Occurrence of Deer Vehicle Collisions.” Irish Forestry 75 (1&2): 8–25.
- Mann, J. C. E., and R. J. Putman. 1989. “Diet of British Sika Deer in Contrasting Environments.” Acta Theriologica 34 (6): 97–109. https://doi.org/10.4098/AT.arch.89-6.
- Marozas, V., K. Pėtelis, G. Brazaitis, and J. Baranauskaitė. 2009. “Early Changes of Ground Vegetation in Fallow Deer Enclosure.” Baltic Forestry 15 (2): 268–272.
- McDevitt, A. D., C. J. Edwards, P. O’Toole, P. O’Sullivan, C. O’Reilly, and R. F. Carden. 2009. “Genetic Structure Of, and Hybridisation Between, Red (Cervus elaphus) and Sika (Cervus nippon) Deer in Ireland.” Mammalian Biology 74 (4): 263–273. https://doi.org/10.1016/j.mambio.2009.03.015.
- McDonough, S., and P. Holloway. 2020. “Incorporating Host-Parasite Biotic Factors in Species Distribution Models: Modelling the Distribution of the Castor Bean Tick, Ixodes Ricinus.” Irish Geography 53 (2): 105–125.
- McHugh, M. 2012. “Interrater Reliability: The Kappa Statistic.” Biochemia Medica 276–282. https://doi.org/10.11613/BM.2012.031.
- McMahon, D. E., A. K. Urza, J. L. Brown, C. Phelan, J. C. Chambers, and I. Ibáñez. 2021. “Modelling Species Distributions and Environmental Suitability Highlights Risk of Plant Invasions in Western United States.” Diversity and Distributions 27 (4): 710–728. https://doi.org/10.1111/ddi.13232.
- Meinshausen, M., S. J. Smith, K. Calvin, J. S. Daniel, M. L. Kainuma, J. F. Lamarque, K. Matsumoto, et al. 2011. “The RCP Greenhouse Gas Concentrations and Their Extensions from 1765 to 2300.” Climatic Change 109 (1): 213–241. https://doi.org/10.1007/s10584-011-0156-z.
- Meyer, T., P. Holloway, T. B. Christiansen, J. A. Miller, P. D’Odorico, and G. S. Okin. 2019. “An Assessment of Multiple Drivers Determining Woody Species Composition and Structure: A Case Study from the Kalahari, Botswana.” Land 8 (8): 122. https://doi.org/10.3390/land8080122.
- Miller, J. A., and P. Holloway. 2017 Niche Theory and Models International Encyclopedia of Geography: People, the Earth, Environment and Technology: People, the Earth, Environment and Technology, 1–10. Hoboken, NJ, USA: John Wiley & Sons.
- Miller, J., S. Laffan, A. Skidmore, and J. Franklin. 2020. “Modeling Movement, Distributions, Diversity, and Disturbance: Introduction to the Fifth Special Issue on Spatial Ecology.” International Journal of Geographical Information Science 34 (8): 1503–1507. https://doi.org/10.1080/13658816.2020.1725017.
- Mody, K., D. Lerch, A. K. Müller, N. K. Simons, N. Blüthgen, M. Harnisch, and K. Del-Claro. 2020. “Flower Power in the City: Replacing Roadside Shrubs by Wildflower Meadows Increases Insect Numbers and Reduces Maintenance Costs.” PloS One 15 (6): 0234327. https://doi.org/10.1371/journal.pone.0234327.
- Morecroft, M. D. 2003. “Impacts of Deer Herbivory on Ground Vegetation at Wytham Woods, Central England.” Forestry 74 (3): 251–257. https://doi.org/10.1093/forestry/74.3.251.
- Morera-Pujol, V., P. S. Mostert, K. Murphy, T. Burkitt, B. Coad, B. J. McMahon, M. Nieuwenhuis, K. Morelle, A. Ward, and S. Ciuti. 2022. “Bayesian Species Distribution Models Integrate Presence-Only and Presence-Absence Data to Predict Deer Distribution and Relative Abundance.“ bioRxiv.
- National Botanical Gardens. 2017. “The Red Data List of Irish Vascular Plants.“ Accessed 1 May 2022. https://botanicgardens.ie/science-and-learning/threatened-plants-in-ireland/.
- Nic Lughadha, E., S. Bachman, T. Leão, F. Forest, J. Halley, J. Moat, C. Acedo, et al. 2020. “Extinction Risk and Threats to Plants and Fungi.” Plants, People, Planet 2 (5): 389–408. https://doi.org/10.1002/ppp3.10146.
- Ohashi, H., M. Yoshikawa, K. Oono, N. Tanaka, Y. Hatase, and Y. Murakami. 2014. “The Impact of Sika Deer on Vegetation in Japan: Setting Management Priorities on a National Scale.” Environmental Management 54 (3): 631–640. https://doi.org/10.1007/s00267-014-0326-7.
- O’Mahony, J., R. de la Torre Cerro, and P. Holloway. 2021. “Modelling the Distribution of the Red Macroalgae Asparagopsis to Support Sustainable Aquaculture Development.” AgriEngineering 3 (2): 251–265. https://doi.org/10.3390/agriengineering3020017.
- O’Rourke, H., E. N. Lughadha, and K. L. Bacon. 2022. “Can the Extinction Risk of Irish Vascular Plants Be Predicted Using Leaf Traits?” Biodiversity and Conservation 1–23. https://doi.org/10.1007/s10531-022-02500-y.
- Pejchar, L., and H. A. Mooney. 2009. “Invasive Species, Ecosystem Services and Human Well-Being.” Trends in Ecology & Evolution 24 (9): 497–504. https://doi.org/10.1016/j.tree.2009.03.016.
- Pérez-Espona, S., J. M. Pemberton, and R. Putman. 2009. “Red and Sika Deer in the British Isles, Current Management Issues and Management Policy.” Mammalian Biology 74 (4): 247–262. https://doi.org/10.1016/j.mambio.2009.01.003.
- Perrin, P. M., F. J. Mitchell, and D. L. Kelly. 2011. “Long-Term Deer Exclusion in Yew-Wood and Oakwood Habitats in Southwest Ireland: Changes in Ground Flora and Species Diversity.” Forest Ecology and Management 262 (12): 2328–2337. https://doi.org/10.1016/j.foreco.2011.08.028.
- Peterson, A. T., J. Soberon, R. G. Pearson, R. P. Anderson, E. Martinez-Meyer, M. Nakamura, and M. B. Araujo. 2011. Ecological Niches and Geographic Distributions, 315. Princeton, New Jersey: Princeton University Press.
- Peterson, A. T., J. Soberón, J. Ramsey, and L. Osorio-Olvera. 2020. “Co-Occurrence Networks Do Not Support Identification of Biotic Interactions.” Biodiversity Informatics 15 (1): 1–10. https://doi.org/10.17161/bi.v15i1.9798.
- Pollard, E., and A. S. Cooke. 1994. “Impact of Muntjac Deer Muntiacus reevesi on Egg-Laying Sites of the White Admiral Butterfly Ladoga Camilla in a Cambridgeshire Wood.” Biological Conservation 70 (2): 189–191. https://doi.org/10.1016/0006-32079490287-9.
- Pontius, R. G., Jr, and M. Millones. 2011. “Death to Kappa: Birth of Quantity Disagreement and Allocation Disagreement for Accuracy Assessment.” International Journal of Remote Sensing 32 (15): 4407–4429. https://doi.org/10.1080/01431161.2011.552923.
- R Core Team. 2021. “R: A Language and Environment for Statistical Computing.“ Vienna, Austria: R Foundation for Statistical Computing. https://www.R-project.org.
- Renner, I. W., and D. I. Warton. 2013. “Equivalence of MAXENT and Poisson Point Process Models for Species Distribution Modeling in Ecology.” Biometrics 69 (1): 274–281. https://doi.org/10.1111/j.1541-0420.2012.01824.x.
- Ripley, B. 1996. Pattern Recognition and Neural Networks. Cambridge, UK: Cambridge Univ. Press. https://doi.org/10.1017/CBO9780511812651.
- Román-Palacios, C., and J. J. Wiens. 2020. “Recent Responses to Climate Change Reveal the Drivers of Species Extinction and Survival.” Proceedings of the National Academy of Sciences 117 (8): 4211–4217. https://doi.org/10.1073/pnas.1913007117.
- Rooney, T. P., and D. M. Waller. 2003. “Direct and Indirect Effects of White-Tailed Deer in Forest Ecosystems.” Forest Ecology and Management 181 (1–2): 165–176. https://doi.org/10.1016/S0378-11270300130-0.
- Saggiomo, L., B. Esattore, and F. Picone. 2020. “What are We Talking About? Sika Deer (Cervus nippon): A Bibliometric Network Analysis.” Ecological Informatics 60:101146. https://doi.org/10.1016/j.ecoinf.2020.101146.
- Schank, C. J., M. V. Cove, M. J. Kelly, E. Mendoza, G. O’Farrill, R. Reyna‐Hurtado, N. Meyer, et al. 2017. “Using a Novel Model Approach to Assess the Distribution and Conservation Status of the Endangered Baird’s Tapir.” Diversity and Distributions 23 (12): 1459–1471. https://doi.org/10.1111/ddi.12631.
- Schmitz, O. J., M. Sylvén, T. B. Atwood, E. S. Bakker, F. Berzaghi, J. F. Brodie, J. P. Cromsigt, et al. 2023. “Trophic Rewilding Can Expand Natural Climate Solutions.” Nature Climate Change, (4): 1–10. https://doi.org/10.1038/s41558-023-01631-6.
- Schymanski, S. J., C. F. Dormann, J. Cabral, I. Chuine, C. H. Graham, F. Hartig, M. Kearney, et al. 2013. “Process, Correlation and Parameter Fitting in Species Distribution Models: A Response to Kriticos et al.” Journal of Biogeography 40 (3): 612–613. https://doi.org/10.1111/jbi.12075.
- Smith, P., M. Bustamante, and H. Ahammad. 2014. “Agriculture, Forestry and Other Land Use (AFOLU).” In Climate Change 2014: Mitigation of Climate Change. Contribution of Working Group III to the Fifth Assessment Report of the Intergovernmental Panel on Climate Change, edited by O. Edenhofer, R. Pichs-Madruga, and Y. Sokona, et al., 811–922. Cambridge: Cambridge University Press.
- Soberon, J., and A. T. Peterson. 2005. “Interpretation of Models of Fundamental Ecological Niches and species’ Distributional Areas.” Biodiversity Informatics 2:1–10. https://doi.org/10.17161/bi.v2i0.4.
- Stanton, J. C., R. G. Pearson, N. Horning, P. Ersts, and H. Reşit Akçakaya. 2012. “Combining Static and Dynamic Variables in Species Distribution Models Under Climate Change.” Methods in Ecology and Evolution 3 (2): 349–357. https://doi.org/10.1111/j.2041-210X.2011.00157.x.
- Svenning, J. C., P. B. Pedersen, C. J. Donlan, R. Ejrnæs, S. Faurby, M. Galetti, D. M. Hansen, et al. 2016. “Science for a Wilder Anthropocene: Synthesis and Future Directions for Trophic Rewilding Research.” Proceedings of the National Academy of Sciences 113 (4): 898–906.
- Thuiller, W. 2004. “Patterns and Uncertainties of species’ Range Shifts Under Climate Change.” Global Change Biology 10 (12): 2020–2027. https://doi.org/10.1111/j.1365-2486.2004.00859.x.
- Thuiller, W., D. Georges, R. Engler, F. Breiner, M. D. Georges, and C. W. Thuiller. 2016. “Package ‘Biomod2’. Species Distribution Modeling within an Ensemble Forecasting Framework.”
- Uno, H., Y. Inatomi, M. Ueno, and H. Iijima. 2019. “Effects of Sika Deer (Cervus nippon) and Dwarf Bamboo (Sasa senanensis) on Tree Seedlings in a Cool-Temperate Mixed Forest on Hokkaido Island, Japan.” European Journal of Forest Research 138 (6): 929–938. https://doi.org/10.1007/s10342-019-01214-1.
- van Proosdij, A. S., M. S. Sosef, J. J. Wieringa, and N. Raes. 2016. “Minimum Required Number of Specimen Records to Develop Accurate Species Distribution Models.” Ecography 39 (6): 542–552. https://doi.org/10.1111/ecog.01509.
- Vega, K. A., and C. Küffer. 2021. “Promoting Wildflower Biodiversity in Dense and Green Cities: The Important Role of Small Vegetation Patches.” Urban Forestry & Urban Greening 62:127165. https://doi.org/10.1016/j.ufug.2021.127165.
- Ward, A. I., S. Richardson, and J. Mergeay. 2021. “Reeves’ Muntjac Populations Continue to Grow and Spread Across Great Britain and are Invading Continental Europe.” European Journal of Wildlife Research 67 (3): 1–10. https://doi.org/10.1007/s10344-021-01478-2.
- Warton, D. I., and L. C. Shepherd. 2010. “Poisson Point Process Models Solve The“pseudo-Absence problem” for Presence-Only Data in Ecology.” The Annals of Applied Statistics 4 (3): 1383–1402. https://doi.org/10.1214/10-AOAS331.
- Wilson, E., R. Wirz, and L. Byrne. 2021. “Transformation of Sitka Spruce Plantations to Continuous Cover Forestry at Dunranhill Forest, County Wicklow, Ireland.” SCOTTISH FORESTRY 75 (4): 33.
- Yokoyama, M., K. Kaji, and M. Suzuki. 2000. “Food Habits of Sika Deer and Nutritional Value of Sika Deer Diets in Eastern Hokkaido, Japan.” Ecological Research 15 (3): 345–355. https://doi.org/10.1046/j.1440-1703.2000.00355.x.
- Zhang, L., F. Huettmann, X. Zhang, S. Liu, P. Sun, Z. Yu, and C. Mi. 2019. “The Use of Classification and Regression Algorithms Using the Random Forests Method with Presence-Only Data to Model species’ Distribution.” MethodsX 6:2281–2292. https://doi.org/10.1016/j.mex.2019.09.035.