Abstract
This research reveals the magnitude of mangrove area loss and future impacts on mangrove species composition and distribution due to rise in Mean Sea Level (MSL). In this study, a geospatial model of potentially inundated areas was developed using Digital Elevation Model (DEM) data to assess the potential impacts of sea level rise (SLR) on the spatial distribution of mangrove species and estimate the potential inundation and subsequent mangrove area loss. The mangrove areas of 2646 ha, 9599 ha and 74,720 ha are projected to be inundated and subsequently lost by the end of the twenty first century for the low, medium and high SLR scenarios respectively under the net subsidence rate −2.4 mm/year relative to the baseline year 2000. All the major five mangrove species of the Bangladesh Sundarbans will be affected and this can potentially contribute to a change in the present species composition and biodiversity of the forest. Results suggest that, under the extreme scenario, inundation and subsequent loss of different mangrove species will be substantial and this can bring a massive change in the species composition and their spatial distribution in the Bangladesh Sundarbans.
1. Introduction
Mangrove forests thrive in tropical and subtropical coastal regions globally and provide support to coastal communities’ livelihoods and contribute at least US $1.6 billion each year in ecosystem services (Costanza et al. Citation1997). The mangroves provide buffer from floods, erosion and natural threats to coastal populations (Chatenoux and Peduzzi Citation2007; Das and Vincent Citation2009; Ismail et al. Citation2012; Kathiresan Citation2012). However, declines for all the known 70 world mangrove species (Polidoro et al. Citation2010) are occurring in many regions due to climate change and anthropogenic threats (FAO Citation2007). Accelerated global warming and climate variabilities have been blamed for the decline in mangrove forests (Gilman et al. Citation2008; Eslami-Andargoli et al. Citation2009). Among the climate related factors, sea level rise (SLR) affects coastal wetlands and mangroves in the long term (Field Citation1995; Gilman Citation2004; LaFever et al. Citation2007; Kassakian et al. Citation2017). Loss of fringe mangrove species, locally and regionally, exposes coastal areas to storms, erosion and flood hazards, and tidal waves, resulting in reduced habitat quality (Ewel et al. Citation1998; Glaser Citation2003; Barbier et al. Citation2008) and may lead to biodiversity loss and ecosystem services disruption that include impacting negatively on human livelihoods (Polidoro et al. Citation2010). Thus there is a need to have mitigation adaptation strategy plans for sustainable mangrove forest management based on sound and robust information.
The Bangladesh Sundarbans is a globally important mangrove ecosystem which lies at the mean elevation of approximately 2 m above mean sea level (MSL) (Payo et al. Citation2016), making it the most vulnerable to potential effects of the SLR due to climate change threats to the mangrove forest composition and distribution (Field Citation1995). Investigation has shown that climatic variables of temperature and rainfall influence the composition and distribution of major mangrove species viz. Heritiera fomes, Sonneratia apelatala and Ceriops decandra in the Sundarbans (Ghosh et al. Citation2017). The global SLR is projected to be approximately 1 m higher or above by the year 2100 (Church et al. Citation2013; Payo et al. Citation2016), but future impacts on composition and distribution of mangrove species remains largely unknown. In the pristine Bangladesh Sundarbans Mangrove Forest, an analysis of tidal gauge records has indicated an increasing east–west trend of 4 mm–7.8 mm year−1 rise in sea level from 1977 to 1998 (Alam Citation2003; SMRC Citation2003), which is well above the average global SLR estimate during the same period (Bindoff et al. Citation2007). Climate change driven rise in the sea level will affect floodplain low elevation areas of the Sundarbans. Research has also predicted that, with the increase in sea level due to global warming, more storm surges in the form of hurricanes and cyclones will be experienced in many coastal areas. Prediction of the SLR and its impacts on the Sundarbans mangrove forest composition and species distribution, given the low-lying elevation of the ecosystem and its vulnerability to any significant change in sea level, is extremely important.
Many prediction models have been developed for studying potential impacts of sea-level rise on coastal ecosystems and environmental changes. Among them are: SimCLIM (Warrick and Cox Citation2007) which uses a database of regional grid cells and down-scaled Global Circulation Models to generate localized rates of sea-level and climate impacts and the Sea-Level Rise Rectification Program (Keim et al. Citation2008). Also available are: Temperature-Based Sea-Level Rise Model (Rahmstorf Citation2007), University of Arizona Web Map Visualization Tool (Strauss et al. Citation2012), Sea Level over Proportional Elevation (SLOPE) model (Doyle et al. Citation2010), Sea Level Affecting Marshes Model (SLAMM) (Geselbracht et al. Citation2011) and recently available Geographic Information System (GIS) sea-level rise mapping tools. None of these models is comprehensive enough to analyze and visualize the impacts of SLR on composition and spatial distribution of mangrove species and their hazard scenarios related to sea level.
A host of researchers have used GIS and geospatial analysis tools to investigate SLR impacts on the coastal ecosystems in pursuit of developing informed policy in many parts of the world (Gravelle and Mimura Citation2008; Li et al. Citation2009; Natesan and Parthasarathy Citation2010; Malik and Abdalla Citation2016). GIS and geospatial analysis techniques have proven useful for predicting the impact under various scenarios related to SLR. Malik and Abdalla (Citation2016) created an ArcGIS model of potentially inundated areas, based on a Digital Elevation Model (DEM) to analyze the local impact of SLR on Richmond, British Columbia, Canada. El-Nahry and Doluschitz (Citation2010) used GIS and field based data in conjunction with Landsat and ASTER imagery to study SLR impacts on the Nile Delta. They created three SLR scenarios of 1.0 m rise, 1.5 m rise, and 2.0 m rise for their study. Li et al. (Citation2009) applied GIS methods to develop a comprehensive universal model for assessing and visualizing the potential inundation based on a global SLR of 1–6 m.
In the Bangladesh Sundarbans, Lovelock et al. (Citation2015) developed a model for predicting the time of submergence of mangroves within a suitable inundation regime (between Highest Astronomical Tide and MSL). The authors’ model assumes estimation based on MSL rise and does not consider tides, coastal erosion and subsidence. Several modelling on the impacts of SLR on the Sundarbans coastal areas have used relatively coarse (>1 m vertical accuracy) elevation data (Chowdhury and Faruqee Citation2000; Sarwar Citation2005). Payo et al. (Citation2016) applied the latest DEM data and the SLAMM to investigate the response to various SLR scenarios on the Sundarbans mangrove ecosystem. The authors’ used remote sensing and prediction modelling to study potential impacts of three SLR scenarios on the Bangladesh Sundarbans. Their work was about Sundarbans mangrove ecosystem inundation and area loss. Loucks et al. (Citation2010) employed geospatial tools and scale-appropriate elevation data to assess the potential impact of SLR on Bangladesh’s Sundarbans tiger population. None of the studies covers the potential impacts of inundation induced by SLR on mangrove composition and species distribution. Diversity and distribution of the mangroves are of immense importance for the present and future Sundarbans ecosystem services and functions. Many mangrove types can tolerate inundation due to tides but when frequency and duration of inundation exceeds physiological thresholds for the specific species, the death of the trees occurs and their occupancy can convert to open water or tidal mud flats (Payo et al. Citation2016).
This present study examines the potential inundation levels in the Sundarbans mangrove forests and identifies species likely to be affected by the SLR using remote sensing and GIS techniques. The uniqueness of this investigation is that it recognizes the low elevation conditions and geomorphologic dynamics in the Sundarbans mangrove ecosystem and applies DEM data and geospatial techniques to develop a detailed and robust model for understanding SLR associated inundation levels and their impacts on composition and spatial distribution of major mangrove species under different hazard scenarios while considering net subsidence rate uncertainties (sinking and no change). The present research identifies vulnerable mangrove species as well as investigates the severity of future risk of sink caused by SLR in order to develop the database for relevant stakeholders to develop a plan for a sustainable management of the forest. The study models which areas will be inundated under future climate change scenarios, however it does not project the actual future distributions of the species as this will depend on changes in salinity levels, adaptation abilities and ecological conditions under future SLR projections. This work is of significant importance as it provides a glimpse of the inundation areas under future SLR scenarios, identifying those areas that may see the largest changes in species composition as mangrove species flourish or decline due to changes in salinity levels and ecological conditions.
2. Materials and methods
Geospatial techniques have been used in this study to develop the SLR scenarios and to assess the effect of SLR on the permanent submergence of Sundarbans mangrove forest floor and its consequences. The methodological framework applied was divided into three sections; the first section describes the study site, data used and their relevancy in this work, while the second and third sections deal with geospatial analysis that includes DEM processing, SLR scenarios preparation, identification of mangrove species composition and spatial analysis of impacted areas. The entire spatial analysis was carried out using ArcGIS 10.4 and ENVI 5.1 software.
2.1. Study site and focus
The present study site is the Bangladesh Sundarbans located approximately between 21° 32′ to 22° 30′N latitude and 89° 00′ to 89° 51′ E longitude (). It covers an area of 6017 km2 (Sarker et al. Citation2016) and is characterized by a tropical climate with temperature ranging from 11 °C to 37 °C and four seasonal patterns. Hot and humid pre-monsoon season starts from March to May followed by monsoon period from June to September and then October and November post-monsoon months and finally December to February being dry winter period. The Sundarbans is a low-lying floodplain with elevation that varies from 0.9 to 2.11 m above sea level (Spalding Citation2010; Ghosh et al. Citation2015) and land slope is 0.03 m vertically per km of horizontal distance moving from north to south (Islam and Gnauck Citation2011). An average relative sea-level rise (RSLR) of 1.07 mm/year from early to mid-Holocene period has been estimated for this region (Islam and Tooley Citation1999). However, recent estimates indicate SLR of 3.14 mm/year, which is way beyond the global SLR estimate of 2.0 mm/year and, based on this rate, the compound sea level elevation is predicted to be close to the 1 m scenario by 2050 (Hazra et al. Citation2002). The Sundarbans mangrove ecosystem is likely to be quite vulnerable to SLR effects, particularly during the pre- and post-monsoon phases when most of the cyclonic storms occur. The Bangladesh Sundarbans hosts 19 species of mangroves (Sarker et al. Citation2016) out of which five species are dominant (Ghosh et al. Citation2016). The focus of this work is the impact of SLR on those five major mangrove species in the Bangladesh Sundarbans.
2.2. Data
In this research, elevation data acts as the main parameter in the determination of the SLR impacts on the spatial distribution of the future mangrove species of the Bangladesh Sundarbans. High resolution elevation data is essential for this kind of research where every centimetre counts due to the low-lying characteristics of the study area. The high resolution (<1 m vertical error) DEM data used in this study was obtained from Water Resources Planning Organization (WRPO), Bangladesh. The elevation information used to construct the DEM was originally collected by a Finnish consulting firm known as FINNMAP in 1991 for the Bangladesh government. FINNMAP measured 80,584 GPS elevation points in mm above sea level. These points were used to create a continuous DEM of the Sundarbans. Initially, the data was georeferenced in accordance with the Survey of Bangladesh (SOB) datum. The SOB datum is a stable datum representing the present MSL. The DEM data was restructured using the Google images from 2006 to 2007 to correct obsolete FINNMAP data by the Institute of Water Modelling (IWM) Bangladesh. This updated data was resampled at 30 m resolution and transferred to the Public Works datum (PWD). The PWD datum has been recognized by the Bangladesh government at 0.459 m below MSL (Payo et al. Citation2016). The vertical resolution of the DEM data is expressed in centimetres and varies from 0 to 608 cm.
In addition to the DEM data, Landsat Operational Land Imager (OLI) data, obtained from the Center for Earth Resources Observation and Science (EROS) website (www.glovis.usgs.gov), was also used in this study to map the mangrove species composition of the Bangladesh Sundarbans. This OLI image was acquired in February 2015. The OLI image information is as follows: 30 m resolution, 137–138/45 path/row and 11 Spectral band (covering visible, near infrared, short-wave infrared and thermal infrared regions of the electromagnetic spectrum).
2.3. Data processing, SLR scenarios selection and preparation
To model and understand the impact of SLR on the future distribution of mangrove species, it was crucial to create potentially inundated areas and the information of DEM was used to create this. However, DEM data provided by WRPO had several sinks which presented negative values for the corresponding cells and could be problematic for further analysis. To remove anomalies and to keep the reliability in the generated results, sinks were removed by altering the negative values into meaningful values using the ‘fill’ tool in ArcGIS software and a depression less DEM was created.
Three different SLR scenarios of 0.46 m (low), 0.75 m (medium) and 1.48 m (extreme) were selected for this study. The 0.46 m and 0.75 m SLR scenarios are based on the mean and peak projections under the RCP 4.5 scenario for West Bengal, India (Pachauri et al. Citation2014; Payo et al. Citation2016) and the extreme case scenario resembles the H++ scenario range which considers the 95% value for RCP 8.5 (0.98 m) plus 0.5 m associated with Ice Sheet melting (Levermann et al. Citation2014; Lovelock et al. Citation2015). The extreme case scenario is considered plausible but unlikely (Jevrejeva et al. Citation2014).
Subsidence rate considered for this model was selected based on Brown and Nicholls (Citation2015), who found the mean net subsidence rate of 2.8 mm/year and median net subsidence rate 2.0 mm/year for the Sundarbans. This mean subsidence rate of the Sundarbans was lowest compared to other land uses in the Ganges delta. The authors studied the difference between the sedimentation, isostatic rebound and subsidence rates in the Ganges–Brahmaputra–Meghna delta to come up with the net subsidence rates based on a range of approaches and temporal scales. They considered natural geological processes and compaction of soil, anthropogenic effects and sediment supply delivered by the river system. They preferred the median rate of subsidence to the mean because some large measurements of subsidence and anthropogenic effects skewed their mean results. In this study, therefore, a value of −2.4 mm/year of net subsidence in the Sundarbans was adopted as a representative value taking into account the shortcomings of Brown and Nicholls (Citation2015) to investigate possible inundation in response to different SLR scenarios on the Sundarbans mangrove ecosystem. To assess the sensitivity of the results to the ambiguity on net subsidence rate in our model, net subsidence rate of 0.0 mm/year (in case of no subsidence) was also applied. The detailed information of data used to model the impact of SLR to examine the potential inundation levels and its consequences on the spatial distribution of the different mangrove species in the Bangladesh Sundarbans are shown in .
Table 1. Information of data used to model the impact of SLR on the spatial distribution of the different mangrove species in the Bangladesh Sundarbans.
Raster Calculator in ArcGIS was used to generate new rasters from the desired cells. Using the elevation values of 46 cm, 75 cm and 148 cm under the net subsidence rate of −2.4 and 0.0mm/year, six raster files were generated for developing the SLR scenarios. The newly generated raster files contained the cells with two values 0 and 1, where 1 characterized the cells of interest while 0 characterized the remaining cells. Therefore, reclassification approach was used to reclassify those newly generated rasters to assign the 0 values to no data. Afterwards, reclassified rasters were converted to polygons for further analysis.
The newly created shape files were used to prepare SLR scenarios for studying the impact of SLR on future spatial distribution of mangrove species. For 46 cm SLR under the two different subsidence rates (−2.4 mm/year and 0.0 mm/year) SLR scenarios were prepared for the year 2100 in respect to base year 2000. Following a similar procedure, SLR scenarios were also created for 75 cm and 148 cm SLR under the two different subsidence rates.
2.4. Analysis of satellite image to identify the mangrove species composition
Maximum likelihood classifier (MLC) algorithm that is widely used in remote sensing applications to classify traditional satellite remote sensing data (Green et al. Citation1998; Gao Citation1999) was adopted in this study to identify the mangrove population by species. Prior to fieldwork, the first author’s extensive field experience, and information from published literature (Chaffey et al. Citation1985; Treygo and Dean Citation1989; Emch and Peterson Citation2006; Giri et al. Citation2007; Ghosh et al. Citation2016, Citation2017) were used to select five major mangrove species out of 19 known species of Bangladesh Sundarbans (Sarker et al. Citation2016) for this classification. The main aim of this research was to model the impact of SLR on the spatial distribution of major mangrove species in the Sundarbans, Bangladesh. Thus, we purposively mapped five major mangrove species of the Sundarbans that constitute higher density cover. These species were Heritiera fomes, Excoecaria agallocha, Ceriops decandra, Sonneratia apelatala and Xylocarpus mekongensis. The majority of the remaining 14 species in the Sundarbans are distributed in lower density, spreading over wide areas in small patches, and do not form large enough mono-specific patches for training site selection and therefore cannot be detected through medium-resolution satellite data, thus were classified under the major five mangrove species on the basis of dominance. This also helped to achieve acceptable accuracy of classified maps and met the aim of the study using Landsat-8 OLI. Training and validation sites for this study were created using field data collected in February–March 2016. Training and validation samples were selected randomly and stratified by land cover class and care was also taken to ensure samples were well distributed and the minimum requirements for validating the accuracy assessment process were met (Congalton and Green Citation2008; Sinha et al. Citation2014). Field data were collected using handheld GPS. The GPS locations of different mangrove species were overlaid on the 2015 OLI image to extract the spectral signatures for each of the species (). Thereafter, spectral signatures were used to classify the image to identify the mangrove population by species. Validation of the image classification was also performed to evaluate the efficacy of the classification and overall accuracy of 89% and kappa coefficient of 0.87 were achieved.
2.5. Spatial analysis of impacted area
The impact of SLR induced inundation on the Sundarbans mangrove forest was analyzed through spatial analysis using newly generated SLR scenarios. The SLR scenarios were overlaid on the species composition map and the impact of inundation levels on overall area and individual mangrove species were analyzed. The analysis included impacts on overall inundated mangrove area, and identification of the affected regions and species under different SLR scenarios. shows the methodological structure used to generate the SLR scenarios and study the SRL impacts.
3. Results
Five major mangrove species viz. H. fomes, E. agallocha, C. decandra, S. apelatala and X. mekongensis and their spatial distribution was identified and mapped in this research (). According to our findings H. fomes is the most dominant species in terms of occupancy in the Bangladesh Sundarbans. Nearly 50% of Bangladesh Sundarbans are occupied by the fresh water loving H. fomes species, followed by E. agallocha (30.1%), C. decandra (17.8%), S. apelatala (2.2%) and X. mekongensis (0.4%) respectively.
Figure 4. Mangrove species composition map of the Bangladesh Sundarbans for the year 2015. Source: Author.
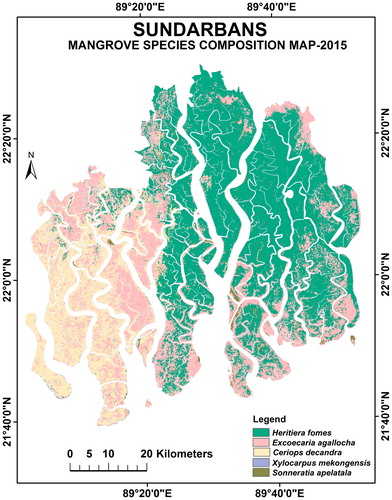
The results indicate that potential inundated mangrove area by the end of the twenty first century will be nominal for the low and medium SLR scenarios, but substantial for the extreme SLR scenario ( and ). The potential inundated mangrove area is predicted to increase for the scenarios under 0 mm/year (or absence of net subsidence) and −2.4 mm/year net subsidence. The mangrove area is projected to be inundated by 2646 ha, 9599 ha and 74,720 ha by the end of the year 2100 for the low, medium and high SLR scenarios respectively under the net subsidence rate −2.4 mm/year relative to the baseline year 2000. On the other hand, the mangrove area is projected to be inundated by 1037 ha, 3231 ha and 44,002 ha by the end of the twenty first century for the low, medium and high SLR scenarios respectively under no net subsidence (0 mm/year).
Figure 5. Image showing potential inundation of mangrove areas by the end of the 21st century under three different SLR scenarios and two subsidence levels in the Bangladesh Sundarbans. Source: Author.
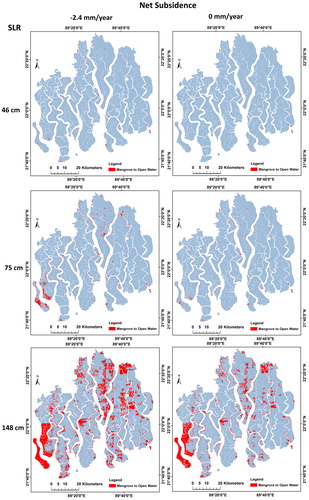
Figure 6. Total area under potential inundation by the end of the 21st century under the selected SLR scenarios and subsidence levels in the Bangladesh Sundarbans.
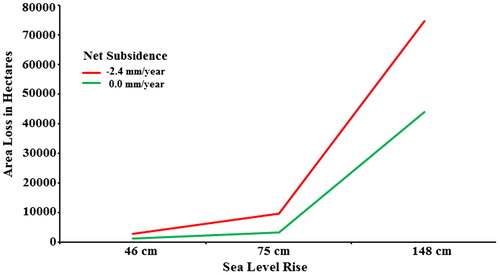
The results also reveal that the impact on different mangrove species will be minimal for low (46 cm) and medium (75 cm) SLR scenarios while extensive for the extreme (148 cm) SLR scenario ( and ). C. decandra, S. apelatala and X. mekongensis will likely be the most affected species for all the SLR scenarios. 0.85%, 5.70% and 30.53% area of C. decandra will be inundated for low, medium and extreme SLR scenarios respectively under the net subsidence rate of −2.4 mm/year while 4.04%, 6.85% and 25.03% area of S. apelatala will be inundated for the same SLR scenarios and subsidence rate respectively. Although X. mekongensis does not make up a substantial share of the forest, it will be one of the most affected species of the Sundarbans. 2.69%, 9.65% and 31.50% area of X. mekongensis will be inundated for low, medium and extreme SLR scenarios respectively under −2.4 mm/year net subsidence rate. On the other hand, two major mangrove species of Bangladesh Sundarbans, namely H. fomes and E. agallocha, which form nearly 80% of the forest will be inundated by 0.41%, 1.29%, 16.73% and 0.72%, 1.94%, 14.88% for low, medium and extreme SLR scenarios respectively under −2.4 mm/year net subsidence rate. More details regarding inundation under 0 mm/year net subsidence rate are provided in .
Figure 7. Image showing inundation areas under future SLR scenarios overlayed with the current mangrove species distribution. The inundation and surrounding areas are likely to see the largest changes in species composition as mangrove species flourish or decline due to changes in salinity levels and ecological conditions, as well as their ability to adapt. Source: Author.
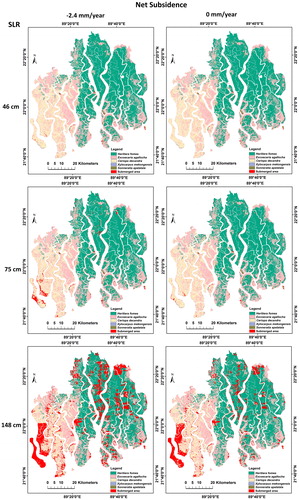
Figure 8. Areas coverage of different mangrove species under potential inundation by the end of the 21st century under selected SLR scenarios and subsidence levels in the Bangladesh Sundarbans.
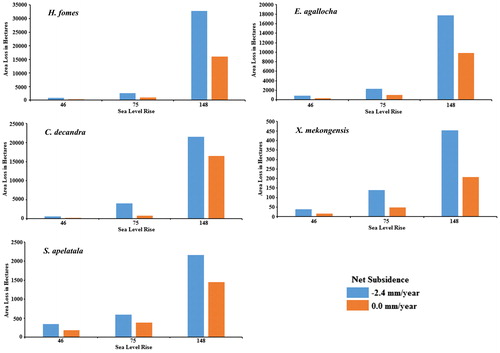
Table 2. Analysis of potential inundation area of different mangrove species under different SLR scenarios and subsidence levels by the end of the twenty first century in the Bangladesh Sundarbans.
4. Discussion
Mangroves are considered one of the important constituents as well as the most vulnerable species in the tropical and subtropical inter-tidal ecosystems of the world (Eslami-Andargoli et al. Citation2009). Climate change components, such as changes in rainfall patterns, temperature, concentrations of atmospheric CO2, sea-level, high water events, intensity of cyclones and storms, and patterns of ocean circulation are likely to affect mangrove forests (Gilman et al. Citation2008; Ghosh et al. Citation2017). Among them, SLR is considered one of the most important factors affecting the mangroves in terms of their distribution in the long run (Eslami-Andargoli et al. Citation2009; Ghosh et al. Citation2017). The results of this investigation are crucial for understanding the magnitude of the SLR in the Bangladesh coast as well as the Sundarbans mangrove forest. Payo et al. (Citation2016) projected a total loss of nearly 10% mangrove area of Bangladesh Sundarbans by 2100 because of SLR. This loss definitely could affect the ecological balance of this ecosystem. The protection and conservation of this important ecosystem is necessary as indicated by the potential impact of future SLR on the Sundarbans mangrove forests in this study.
According to Colette (Citation2013) a 45 cm global SLR by the year 2100 can potentially be a risk to 75% of the Sundarbans mangroves. Loucks et al. (Citation2010), using different DEMs, predicted that most parts of the Sundarbans will be inundated for a SLR of +28 cm. Payo et al. (Citation2016) work on the cumulative elevation frequency analysis indicated that even 2.19 m relative SLR would not completely inundate the Sundarbans but may potentially affect 50% of the area. Therefore, the accuracy of the DEM used plays a significant role in determining potential inundation levels in relatively flat plains such as the Sundarbans. The results of this study indicates a level of inundation which is contrary to the works of Loucks et al. (Citation2010) and Colette (2013) but close to the condition of the extreme scenario and projections by Payo et al. (Citation2016). The deviation of our results could be attributed to the fact that our study considered only the vegetated areas while that of Payo et al. (Citation2016), in addition, covered beach and mudflat areas. More plausible information on the fate of mangrove composition and distribution in the Sundarbans by the end of the twenty first century could be made clear with more accurate and up-to-date DEM data, possibly LiDAR data.
In terms of mangrove species composition and their spatial distribution, potential impacts of low (46 cm) and medium (75 cm) SLR scenarios are expected to be minimal while extreme (148 cm) SLR scenario will have more detrimental effects as the area under inundation will increase. C. decandra, X. mekongensis and S. apelatala will be at high risk as they will lose more than 25% of their habitat, although the other two (H. fomes and E. agallocha) will also be at risk in the long term. H. fomes and E. agallocha, which make up four-fifth of the forest, will be the most affected species in terms of area loss. The south-western part of the Bangladesh Sundarbans, which is occupied mainly by the salt loving C. decandra and northeastern part of central area that is occupied mainly by H. fomes, will be the main victims due to the SLR. The worst-case scenario is expected to be experienced under the Brown and Nicholls mean subsidence rate of −2.8 mm/year. More mangrove forest area would be inundated than the area inundated with the representative value of −2.4 mm/year used in this study.
Inundation, due to SLR, will increase water and soil salinity in the Sundarbans and is set to affect the mangrove ecosystem, especially the species composition. SLR will not only be responsible for area loss of different mangrove species through inundation for longer periods, but it will also expose the rest of the mangrove vegetated area to salinity. According to Ghosh et al. (Citation2016), in the Sundarbans, salinity tolerance levels of different mangrove species are different and vary spatially. Increasing soil and water salinity will have a detrimental effect on species such as H. fomes which is less salt tolerant. H. fomes is the most dominant species in the Bangladesh Sundarbans and this species is thought to suffer from die-back disease that is highly related to the level of salinity. In response to the increasing salinity, there will be some possibilities of expansion of salt tolerant species such as C. decandra by encroaching into the non-salt tolerant species areas such as H. fomes, which can play a vital role in changing the existing mangrove species composition and ecosystem of the forest. In the short period, these changes may not be that much significant but the continued process of such changes raises concerns with respect to the future sustainability for some of the mangrove species and the biodiversity of the Sundarbans. The Sundarbans mangrove species H. fomes, E. agallocha, X. mekongensis and S. apelatala are economically valuable timber species while C. decandra is a major non-timber forest product for the Sundarbans communities (Uddin et al. Citation2013). This implies that a change in the existing mangrove species composition and ecosystem of the forest due to climate change (SLR) would affect the economic and livelihood systems in this part of the world.
In this study, spatially variable impacts of isostatic rebound or land subsidence are considered in elevation computations taking account of the minimum elevation and slope of that cell. However, there are few limitations in this research that are important to emphasize. Firstly, as the result of this study is highly dependent on the accuracy of the DEM data used, any error in data collection for DEM or DEM interpolation can also be embedded in the result. Secondly, the subsidence rate of −2.4 mm/year considered for this study may not be uniform in the entire Sundarbans. Thirdly, different influential processes and interactions that might be effective for potential changes in the mangrove area are not considered in this research. For instance, the relation between sediment accretion and growth of mangroves, assumption of uniform net subsidence, sediment supply changes, or erosion rate caused by different natural forces such as cyclones and water surges are not considered. Finally, responsible anthropogenic forces of current time which may induce future mangrove area loss are also not considered here. Despite these limitations, nevertheless, the results of this study can potentially be used in conservation management of the Sundarbans. The results can be shared with relevant stakeholders, policy makers and sustainable management planners to develop a coping strategy against SLR impact on the mangrove forest and develop a sustainable plan.
5. Conclusion
Due to the low-lying physiographical setting, SLR may potentially pose a threat to Bangladesh Sundarbans mangrove forest. This mangrove forest has rich biological diversity and conservation values. The key objective of this research was to generate potentially inundated floodplains due to SLR using DEM data for Bangladesh Sundarbans Forest and understand the future consequences on spatial distribution of major mangrove species. We developed a geospatial model of inundation for this study. Three SLR scenarios viz. low (46 cm), medium (75 cm) and extreme (148 cm) were prepared and these scenarios were overlaid on mangrove species composition map of the Sundarbans to visualize and quantify the impacted areas.
According to our findings, potential impact of low (46 cm) and medium (75 cm) SLR scenarios will be minimal on mangrove species composition and their spatial distribution while the impact will be extensive under the extreme (148 cm) SLR scenario. All the five major mangrove species will be affected by the SLR and can potentially contribute to a change in the existing species composition and biodiversity of the forest. This present study provides new knowledge and crucial perspective on the impact of SLR on the permanent submergence of Sundarbans mangrove forest floor and its consequences. It is apparent from the present research that Bangladesh Sundarbans is more resilient to SLR as opposed to predictions by some previous researchers. The main reason for this is that most of the previous researchers used lower accuracy DEMs for modelling. Although this mangrove forest shows resilience to SLR, the trend of resilience will be highly dependent on the subsidence rate, which is uncertain. Furthermore, detailed investigations of the Bangladesh Sundarbans regarding future spatial distribution of tree species because of changes in salinity level, edaphic conditions and geomorphological change associated with sediment influx and also the adaptation capacity of mangrove species due to the changing climate scenario would be useful for the conservation and management of the Sundarbans.
Acknowledgement
The authors would like to acknowledge Water Resources Planning Organization (WRPO), Bangladesh which provided Digital Elevation Model (DEM) data used in this study.
Disclosure statement
No potential conflict of interest was reported by the authors.
References
- Alam, M. 2003. Bangladesh country case study. National Adaptation Programme of Action (NAPA) Workshop.
- Barbier EB, Koch EW, Silliman BR, Hacker SD, Wolanski E, Primavera J, Granek EF, Polasky S, Aswani S, Cramer LA, et al. 2008. Coastal ecosystem-based management with nonlinear ecological functions and values. Science 319(5861):321–323.
- Bindoff N, Willebrand J, Artale V, Cazenave A, Gregory J, Gulev S, Hanawa K, Le Quere C, Levitus S, Nojiri Y. 2007. Observations: oceanic climate and sea level. In: Climate change 2007: The physical Science Basis. Contribution of Working Group I to the Fourth Assessment report of the Intergouvernmental Panel on Climate Change. Cambridge, UK: Cambridge University Press; p.385–432.
- Brown S, Nicholls R. 2015. Subsidence and human influences in mega deltas: the case of the Ganges–Brahmaputra–Meghna. Science of the Total Environment. 527:362–374.
- Chaffey DR, Miller F, Sandom J. 1985. A forest inventory of the Sundarbans, Bangladesh. Surrey, U.K: Land Resources Development Centre.
- Chatenoux B, Peduzzi P. 2007. Impacts from the 2004 Indian Ocean Tsunami: analysing the potential protecting role of environmental features. Nat Hazards. 40(2):289–304.
- Chowdhury S, Faruqee R. 2000. Bangladesh-Climate Change and Sustainable Development. World Bank Report.(21104-BD).
- Church JA, Clark PU, Cazenave A, Gregory JM, Jevrejeva S, Levermann A, Merrifield MA, Milne GA, Nerem RS, Nunn PD. 2013. Sea level change. Cambridge, UK: PM Cambridge University Press.
- Colette A. 2013. Case studies on climate change and World Heritage. Paris: UNESCO Publishing.
- Congalton RG, Green K. 2008. Assessing the accuracy of remotely sensed data: principles and practices. Boca Raton, FL: CRC press.
- Costanza R, d'Arge R, De Groot R, Faber S, Grasso M, Hannon B, Limburg K, Naeem S, O'neill RV, Paruelo J. 1997. The value of the world's ecosystem services and natural capital. Nature. 387(6630): 253.
- Das S, Vincent JR. 2009. Mangroves protected villages and reduced death toll during Indian super cyclone. Proceedings of the National Academy of Sciences. 106(18):7357–7360.
- Doyle TW, Krauss KW, Conner WH, From AS. 2010. Predicting the retreat and migration of tidal forests along the northern Gulf of Mexico under sea-level rise. Forest Ecology and Management. 259(4):770–777.
- El-Nahry AH, Doluschitz R. 2010. Climate change and its impacts on the coastal zone of the Nile Delta, Egypt. Environ Earth Sci. 59(7):1497–1506.
- Emch M, Peterson M. 2006. Mangrove forest cover change in the Bangladesh Sundarbans from 1989-2000: A remote sensing approach. Geocarto International. 21(1):5–12.
- Eslami-Andargoli L, Dale P, Sipe N, Chaseling J. 2009. Mangrove expansion and rainfall patterns in Moreton Bay, southeast Queensland, Australia. Estuarine, Coastal and Shelf Science. 85(2):292–298.
- Ewel K, Twilley R, Ong J. 1998. Different kinds of mangrove forests provide different goods and services. Global Ecology & Biogeography Letters. 7(1):83–94.
- FAO. 2007. The world's mangroves 1980-2005. A Thematic Study Prepared in the Framework of the Global Forest Resources Assessment. FAO Forestry Paper, 89. Rome: Food and Agriculture Organization of the United Nations.
- Field, CD. 1995. Impact of expected climate change on mangroves. Asia-Pacific Symposium on Mangrove Ecosystems. Dordrecht: Springer.
- Gao J. 1999. A comparative study on spatial and spectral resolutions of satellite data in mapping mangrove forests. Int J Remote Sens. 20(14):2823–2833.
- Geselbracht L, Freeman K, Kelly E, Gordon DR, Putz FE. 2011. Retrospective and prospective model simulations of sea level rise impacts on Gulf of Mexico coastal marshes and forests in Waccasassa Bay, Florida. Climat Change. 107(1–2):35–57.
- Ghosh A, Schmidt S, Fickert T, Nüsser M. 2015. The Indian Sundarban Mangrove Forests: history, utilization, conservation strategies and local perception. Diversity. 7(2):149–169.
- Ghosh MK, Kumar L, Roy C. 2016. Mapping long-term changes in mangrove species composition and distribution in the Sundarbans. Forests. 7(12):305.
- Ghosh MK, Kumar L, Roy C. 2017. Climate variability and mangrove cover dynamics at species level in the Sundarbans, Bangladesh. Sustainability. 9(5):805.
- Gilman E. 2004. Assessing and managing coastal ecosystem response to projected relative sea-level rise and climate change. Prepared for the International Research Foundation for Development Forum on Small Island Developing States: Challenges, Prospects and International Cooperation for Sustainable Development. Contribution to the Barbados. Port Louis, Mauritius, p 28.
- Gilman EL, Ellison J, Duke NC, Field C. 2008. Threats to mangroves from climate change and adaptation options: a review. Aquatic Botany. 89(2):237–250.
- Giri C, Pengra B, Zhu Z, Singh A, Tieszen LL. 2007. Monitoring mangrove forest dynamics of the Sundarbans in Bangladesh and India using multi-temporal satellite data from 1973 to 2000. Estuarine, Coastal and Shelf Science. 73(1–2):91–100.
- Glaser M. 2003. Interrelations between mangrove ecosystem, local economy and social sustainability in Caeté Estuary, North Brazil. Wetlands Ecology and Management. 11(4):265–272.
- Gravelle G, Mimura N. 2008. Vulnerability assessment of sea-level rise in Viti Levu, Fiji Islands. Sustain Sci. 3(2):171–180.
- Green EP, Clark CD, Mumby PJ, Edwards AJ, Ellis A. 1998. Remote sensing techniques for mangrove mapping. Int J Remote Sens. 19(5):935–956.
- Hazra S, Ghosh T, DasGupta R, Sen G. 2002. Sea level and associated changes in the Sundarbans. Science and Culture. 68(9/12):309–321.
- Islam MS, Tooley M. 1999. Coastal and sea-level changes during the Holocene in Bangladesh. Quaternary International. 55(1):61–75.
- Islam SN, Gnauck A. 2011. Water salinity investigation in the Sundarbans rivers in Bangladesh. IJW 6(1/2):74–91.
- Ismail H, Wahab AA, Alias N. 2012. Determination of mangrove forest performance in reducing tsunami run-up using physical models. Nat Hazards. 63(2):939–963.
- Jevrejeva S, Grinsted A, Moore JC. 2014. Upper limit for sea level projections by 2100. Environ Res Lett. 9(10):104008.
- Kassakian J, Jones A, Martinich J, Hudgens D. 2017. Managing for no net loss of ecological services: an approach for quantifying loss of coastal wetlands due to sea level rise. Environ Manage. 59(5):736–751.
- Kathiresan K. 2012. Importance of mangrove ecosystem. Int J Marine Sci. 2(1): 70-89
- Keim B, Doyle T, Burkett V, Van Heerden I, Binselam S, Wehner M, Tebaldi C, Houston T, Beagan D. 2008. How is the Gulf Coast climate changing? Impacts of climate change and variability on transportation systems and infrastructure: Gulf Coast study. Phase I A Report by the US Climate Change Science Program and the Subcommittee on Global Change Research.3-1 to 3–331.
- LaFever DH, Lopez RR, Feagin RA, Silvy NJ. 2007. Predicting the impacts of future sea-level rise on an endangered lagomorph. Environ Manage. 40(3):430–437.
- Levermann A, Winkelmann R, Nowicki S, Fastook JL, Frieler K, Greve R, Hellmer HH, Martin MA, Meinshausen M, Mengel M, et al. 2014. Projecting Antarctic ice discharge using response functions from SeaRISE ice-sheet models. Earth Syst Dynam. 5(2):271.
- Li X, Rowley RJ, Kostelnick JC, Braaten D, Meisel J, Hulbutta K. 2009. GIS analysis of global impacts from sea level rise. Photogramm Eng Remote Sensing. 75(7):807–818.
- Loucks C, Barber-Meyer S, Hossain MAA, Barlow A, Chowdhury RM. 2010. Sea level rise and tigers: predicted impacts to Bangladesh’s Sundarbans mangroves. Climatic Change. 98(1–2):291.
- Lovelock CE, Cahoon DR, Friess DA, Guntenspergen GR, Krauss KW, Reef R, Rogers K, Saunders ML, Sidik F, Swales A, et al. 2015. The vulnerability of Indo-Pacific mangrove forests to sea-level rise. Nature. 526(7574):559–563.
- Malik A, Abdalla R. 2016. Geospatial modeling of the impact of sea level rise on coastal communities: application of Richmond, British Columbia, Canada. Modeling Earth Systems and Environment. 2(3):146.
- Natesan U, Parthasarathy A. 2010. The potential impacts of sea level rise along the coastal zone of Kanyakumari District in Tamilnadu, India. J Coast Conserv. 14(3):207–214.
- Pachauri RK, Allen MR, Barros VR, Broome J, Cramer W, Christ R, Church JA, Clarke L, Dahe Q, Dasgupta P. 2014. Climate change 2014: synthesis report. Contribution of Working Groups I, II and III to the fifth assessment report of the Intergovernmental Panel on Climate Change. IPCC.
- Payo A, Mukhopadhyay A, Hazra S, Ghosh T, Ghosh S, Brown S, Nicholls RJ, Bricheno L, Wolf J, Kay S, et al. 2016. Projected changes in area of the Sundarban mangrove forest in Bangladesh due to SLR by 2100. Climatic Change. 139(2):279–291.
- Polidoro BA, Carpenter KE, Collins L, Duke NC, Ellison AM, Ellison JC, Farnsworth EJ, Fernando ES, Kathiresan K, Koedam NE, et al. 2010. The loss of species: mangrove extinction risk and geographic areas of global concern. PloS One. 5(4):e10095.
- Rahmstorf S. 2007. A semi-empirical approach to projecting future sea-level rise. Science. 315(5810):368–370.
- Sarker SK, Reeve R, Thompson J, Paul NK, Matthiopoulos J. 2016. Are we failing to protect threatened mangroves in the Sundarbans world heritage ecosystem? Sci Rep. 6:21234
- Sarwar MGM. 2005. Impacts of sea level rise on the coastal zone of Bangladesh. http://staticweadaptorg/placemarks/files/225/golam_sarwarpdf.
- Sinha P, Kumar L, Drielsma M, Barrett T. 2014. Time-series effective habitat area (EHA) modeling using cost-benefit raster based technique. Ecol Informatics. 19:16–25.
- Smrc T. 2003. The vulnerability assessment of the SAARC coastal region due to sea level rise: Bangladesh case. Dhaka, Bangladesh: SAARC Meteorological Research Council (SMRC).
- Spalding M. 2010. World atlas of mangroves. Abingdon: Routledge.
- Strauss BH, Ziemlinski R, Weiss JL, Overpeck JT. 2012. Tidally adjusted estimates of topographic vulnerability to sea level rise and flooding for the contiguous United States. Environ Res Lett. 7(1):014033.
- Treygo W, Dean PB. 1989. The Environment and Development in Bangladesh: An Overview and Strategy for the Future. Canadian International Development Agency.
- Uddin MS, van Steveninck ER, Stuip M, Shah MAR. 2013. Economic valuation of provisioning and cultural services of a protected mangrove ecosystem: a case study on Sundarbans Reserve Forest, Bangladesh. Ecosystem Services. 5:88–93.
- Warrick, RA, Cox, G. 2007. New developments of SimCLIM software tools for risk-based assessments of climate change impacts and adaptation in the water resource sector. Proceedings of the 3rd International Conference on Climate and Water; 2007. Finish Environmental Institute (SYKE).