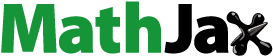
Abstract
This study aimed to examine flood hazard zoning and assess the role of check dams as effective hydraulic structures in reducing flood hazards. To this end, factors associated with topographic, hydrologic and human characteristics were used to develop indices for flood mapping and assessment. These indices and their components were weighed for flood hazard zoning using two methods: (i) a multi-criterion decision-making model in fuzzy logic and (ii) entropy weight. After preparing the flood hazard map by using the above indices and methods, the characteristics of the change‐point were used to assess the role of the check dams in reducing flood risk. The method was used in the Ilanlu catchment, located in the northwest of Hamadan province, Iran, where it is prone to frequent flood events. The results showed that the area of ‘very low’, ‘low’ and ‘moderate’ flood hazard zones increased from about 2.2% to 7.3%, 8.6% to 19.6% and 22.7% to 31.2% after the construction of check dams, respectively. Moreover, the area of ‘high’ and ‘very high’ flood hazard zones decreased from 39.8% to 29.6%, and 26.7% to 12.2%, respectively.
1. Introduction
As the most destructive natural disaster across the world, flood constitute about one-third of the global geophysical hazards (Smith and Ward Citation1998; Novelo-Casanova and Rodríguez-Vangort Citation2016; Matheswaran et al. Citation2019). The floods have been taken into account as the cause for loss of life and financial damage. However, this phenomenon can be managed and mitigated with a range of appropriate strategies (Gan et al. Citation2018; Sepehri et al. Citation2018). Flood hazard mapping is not a measure on its own to reduce flood damages; rather, it should be combined with other corrective actions. There are several interventions such as water supply, diversion and construction of the check dam that can be made to change the hydrological behaviour of the catchments, for example changes in the flow velocity, soil erosion and sedimentation (Yazdi et al. Citation2018; Ildoromi et al. Citation2019). While these can have significant ecological effects over time and across space, such changes cause the natural conjunction of river ecosystems to undergo significant alterations. In fact, when the riverbed’s slope is corrected by measures such as check dams, the flow depth and velocity in the downstream of check dams can be altered, and the upstream slope of the check dams approaches the limit gradient (Bombino et al. Citation2008; FitzHugh and Vogel Citation2011; Zema et al. Citation2018). In recent decades, several studies have evaluated the effect of the check dams on catchments hydrology and geomorphology. Applying the WaTEM/SEDEM model and six land use scenarios, Boix-Fayos et al. (Citation2008) investigated the effect of the presence or absence of check dams on the sediment yield of the Rogativa basin in Spain. The results pointed to 77% reduction in the sediment load after the construction of check dams. Ma et al. (Citation2014), using the SWAT model, quantified the effects of climate change, vegetation and check dams on reduction of suspended sediment yield of a catchment in southwestern China. The results showed that 47.8% of sediment reduction was related to rehabilitated vegetation cover, 19.8% to climate change and 26.1% and 6.3% to check dams, and simulation bias, respectively. Roshani (Citation2003) examined the effect of check dams on the peak flow of the flood hydrograph of catchment. Their results revealed that the slope of sub-basins, as the main parameter, contributes significantly to the performance of check dams. Moreover, these researchers argued that in a catchment with 536 check dams, a 31% decrease in the peak discharge may occur, which was related to changes in the concentration time in the sub-catchment. After simulating the river flow in the study area using the HEC-HMS and HEC-RAS models, Shieh et al. (Citation2007) simulated the effect of check dams on the river process. Bombino (Citation2009) examined the effect of check dams on the shapes of the channel, sediment and vegetation in the upstream region of Calabria in southern Italy. Mizuyama (Citation2008) reported that check dams can prevent debris flows by changing the stream bed gradient and successive small dams can prevent cutting of channels and drainage networks. Sediment-filled check dams create a layer of wedge-shaped sedimentary deposits that can be used for agricultural purposes with a yield two to three times greater than that of terraced lands and 6–10 times greater than that of hill slopes. Including the impact of check dams on flood hazard mapping, by considering several assessment indices, is required to develop such maps for catchments with constructed check dams. However, the complex and non-linear behaviour relationships between indices and flood risk is a challenge for an accurate modelling approach. Various systematic methods such as the Analytic Hierarchy Process (AHP) (Liu et al. Citation2008; Stefanidis and Stathis Citation2013; Chakraborty and Joshi Citation2016), Set Pair Analysis (SPA) (Wu et al. Citation2012, Citation2019; Guo et al. Citation2014), Imprecise Shannon’s Entropy (Sepehri et al. Citation2019b; Lotfi and Fallahnejad Citation2010) and Fuzzy Comprehensive Evaluation (Lai et al. Citation2015; Ildoromi et al. Citation2019; Sepehri et al. Citation2019a) have been developed to overcome this complexity. Although these methods have been widely used to analyze flood hazard as an efficient tool, they are associated with weaknesses and uncertainties due to their difficult complicated design (Fernández and Lutz Citation2010).
In this article, we have applied the change-point approach to assess the role of check dams in reducing flood hazard using two methods of the multi-criterion decision-making (MCDM) model within a fuzzy logic framework and entropy weight.
Nowadays, change-points are widely used approach in the analysis of data series in hydrology and engineering studies. The change-points approach has been used in hydrology to explore the impact of human activities for example land use changes, the construction of check dams and climate to identify potential sudden changes over time and space (Jeon et al. Citation2016; Militino et al. Citation2018; Zhou et al. Citation2018; Xie et al. Citation2019).
Check dams are the principal soil- and water-conservation structures in the Ilanlo catchment, with nearly 70% of the area controlled by them. The objective of this research is to develop a methodology to assess the effect of check dams on flood hazard zoning. This will help to develop accurate hazard maps, evaluate effect of hydraulic structure.
2. Materials and methods
2.1. Study area
The Ilanlo catchment with an area of 17 km2 is located in the northwest of Hamadan Province, Iran (). Temperature data from the Asadabad meteorological station shows that the temperature varies between –15 °C and +34 °C with February and August, representing the coldest and hottest months of the year, respectively. On the other hand, precipitation data from the same station shows that the average annual rainfall is 313 mm. In the last decade, the study area has witnessed several severe floods that prompted the local authorities to construct check dams to reduce flood-induced damages. However, regional studies show that a number of these check dams have been destroyed, either partially or completely, due to the occurrence of flood events (Ildoromi et al. Citation2019).
2.2. Methodology
This study was conducted drawing on the summaries and methodologies used in Kalantari et al. (Citation2014), Gigović et al. (Citation2017), Malekinezhad et al. (Citation2017) and Hazarika et al. (Citation2018). Therefore, an index model was developed to identify flood-prone areas with a regional focus in Geographic Information System (GIS) environment. The proposed model performs the flood hazard index using multi-criteria analysis. In general, the flood hazard index is to identify flood-prone regions and perform comparative analyses on different catchments. shows the proposed method. Initially, information from various databases was imported to ArcGIS 10.1. After primary data analysis, the indices were then weighted using the fuzzy logic and entropy weight methods, and a flood index map was prepared by combining the indices. In the next step, similar properties to the change-point were used to assess the effect of the check dams on the reduction of flood hazards ().
2.3. Flood indices
Before performing flood susceptibility assessment, it is essential to first determine the flood-conditioning factors (Elmahdy and Mostafa Citation2013; Chapi et al. Citation2017; Al-Juaidi et al. Citation2018; Sepehri et al. Citation2019b). An acceptable flood hazard map is highly dependent on the quality of the spatial and temporal data, which need to be acquired from the case study. Unfortunately, many case studies, particularly in developing countries, are ungauged or poorly gauged (Sivapalan Citation2003). In some cases, the number of existing gauging stations has decreased. On the other hand, the existence of multivariate and nonlinear relationships between indices and risk levels is a major intrinsic challenge to flood hazard risk assessment (Wagener et al. Citation2004; Razavi and Coulibaly 2012; Sepehri et al. Citation2019b). Therefore, preparing a flood hazard map in these areas is a significant challenge. The first step in this regard is to select appropriate indices (Wagener et al. Citation2004; Razavi and Coulibaly 2012; Sepehri et al. Citation2019b). Flood risk variables vary from region to region based on the specific features of each (Tehrany et al. Citation2019). An indicator that may be important in flood studies in a region may not be important in another area (Kia et al. Citation2012). In this study, the flood index is derived from the combination of topographic (i.e. slope, plan curvature and profile curvature), hydrologic (i.e. distance to discharge channel, soil type and land use [STLU] and topographic wetness index [TWI]) and human indices (i.e. erosion and check dam). These indices were selected based on the data of various case studies with similar characteristics.
2.4. Fuzzy membership function
In an evaluation system, fuzzy sets are used to show the reliability level for further assessment. The reliability levels in a fuzzy set are used to indicate the membership of indices in obscure sets. Zadeh et al. (Citation1996) introduced various membership functions in order to graphically represent and simplify the measurement of performance in a fuzzy set whose values vary from 0 to 1. In an evaluation system, the selection of membership functions and determination of their parameters are based on the priorities of decision makers in the field of study. Therefore, all effective indices related to flood hazard studies are expected to have weights of almost similar values. In this study, two common membership functions, that is linear and Gaussian, were used to assign weights to effective indices. EquationEquation (1)(1)
(1) is used for indices that have a direct or indirect relationship with the flood degree. Gaussian membership function was solely used for assigning a weight to the fractal dimension as sub-index for distance to the discharge channel index (Ildoromi et al. Citation2019; Sepehri et al. Citation2019a).
(1)
(1)
(2)
(2)
(3)
(3)
Where a represents the feet or the minimum linear vector and b specifies the peak or maximum linear vector. In Gaussian function, m is the median of input data and is the variance.
2.5. Entropy
In an evaluation system, it is a necessity to define the weights of indices to measure their effect on the target (Smithson Citation1989). When a high weight is assigned to an index, it means that it has a great effect on the target and vice versa. In other words, an index with low weight has a smaller effect on the target. The entropy concept can be used to provide useful information about the distribution, uncertainty, disorder and variation of the indices as well as to assign weights to indices (Singh Citation1997; Kawachi et al. Citation2001; Lotfi and Fallahnejad Citation2010). The main criteria for the entropy weight method are as follows:
In order to eliminate the dimension of the indices, it is a necessity to normalize the indices by EquationEqs. (4)
(4)
(4) and Equation(5)
(5)
(5) . EquationEquation (4)
(4)
(4) is used for indices that have a direct relationship with flood hazard degree, otherwise, EquationEq. (5)
(5)
(5) will be used.
(4)
(4)
(5)
(5)
Where Xij denotes the value of the ith index (i = 1, 2, 3…., m) used for flood hazard zoning and subscript j (j = 1, 2, 3…, n) is the number considered for the purpose of this study, that is flood zoning.
To evaluate the problem with m indexes and n targets, the entropy value pi for the ith index can be defined as follows:
(6)
(6)
Where (fij = 0, it is assumed that fij ln fij = 0).
2.6. Final flood hazard map
A significant point to bear in mind in the index scoring method using fuzzy logic is that the weight of each index is considered individually and independent of other indices.
However, in an evaluation system, assigning weights to indices relative to each other is an important factor that can show the importance of indices relative to each other. Therefore, the entropy weight method is used in this study to demonstrate the importance of the indices relative to each other (Li et al. Citation2010; Zeng et al. Citation2010; Ge et al. Citation2013). Combination of the entropy weight and fuzzy logic methods provides a better and more effective flood hazard map than when each method is applied on its own. The final flood hazard map for each point of the study area (pixels) is calculated using a simple multiplication of two fuzzy maps and entropy weighting:
(7)
(7)
Where H(X) is related to entropy and is related to the membership function of the indices. The phrase in parentheses is related to the final fuzzy map.
3. Results and discussion
Floods are among the most serious threats in areas and countries where other natural hazards hardly occur. Flood in the Ilanlu watershed is affected by the above-mentioned indices. Indices are weighted based on the following methods:
As one of the most effective flood prevention measures, check dams play a vital role in flood control (Yazdi et al. Citation2018; Abbasi et al. Citation2019). In the study area, a large number of check dams had been constructed in a concentrated fashion around the drainage networks. Given the lack of data on the storage capacity of check dams, the height of the check dams, which is directly related to their capacity, was used as an indicator for flood resistance. Regarding the role of check dams in reducing flood hazard, EquationEq. (2)(2)
(2) , which is the inverse of EquationEq. (1)
(1)
(1) , was used to calculate the fuzzy scores. As shown in , areas without any check dams had the highest fuzzy score and vice versa. The entropy values of this index are also in the range of 0–0.34. A challenging question in this regard is to see whether the flood hazard map of the region will change in the absence of any check dams. In this study, a similar property to the change-point was used to answer this question. For flood hazard mapping, a fixed map with a value of 1, which refers to areas without any check dams, was added to other indices before the construction of check dams. In the next step, after the construction of check dams and given their diminishing effect on flood hazards, a number smaller than 1 (depending on the height of the check dams) was added to other indices where the change-point occurs (). It should be noted that the addition of a map with a constant value of 1, instead of the index map for the presence of check dam, changes the weighted entropy values of indices. Therefore, they are calculated relative to other indices.
Figure 4. The general outline of the change-point for assessing the effect of check dams on the flood hazard map.
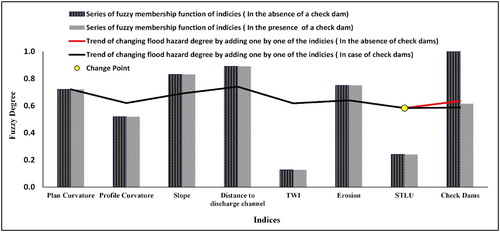
shows an example of how the change-point is applied to assess the performance of check dams in flood hazard zoning using fuzzy logic at the point of the study area with the metric coordinates being X: 221,726.91 and Y: 3,925,699. The pointed black and shaded histograms related to the initial fuzzy scoring are flooding indexes. The only difference between these two histograms is the check dam index. Given the diminishing role of check dams in flood hazard zoning, the height value of the check dam index is 3.5 m in the desired point. The fuzzy membership degree of this point is then calculated to be 0.65 using EquationEq. (2)(2)
(2) . In the absence of any check dams in the area (i.e. the height of the check dam is 0), the membership degree of this function (i.e. black spot histogram) at the point is equals to 1. The black and blue lines also show the flooding change trend that is obtained using the average fuzzy membership rates (the left-hand side of EquationEq. (2)
(2)
(2) ) for the two scenarios (i.e. the presence and absence of check dams).
The two black and blue linear charts merge until they are separated by the STLU index point. In the next step, an index map for the presence and absence of check dams, that is the flood hazard change trend, is added.
Soil erosion is one of the most important environmental issues in the world (Yin and Li Citation2001). Soil erosion hazards involve damaging the aquatic and terrestrial environment by reducing nutrients, increasing runoff and affecting aquatic life (Langdale and Shrader Citation1982; Pimentel and Burgess Citation2013; Quinteiro et al. Citation2017; Mamedov and Levy Citation2019). Soil erosion is divided into two major groups of water and wind erosions. Water erosion is classified into sub-categories of sheet and gully erosion (Morgan and Rickson Citation2003). This deformation is accompanied by increased degradation and reduced permeability. There are three types of erosion in the study area, including sheet, rill and gully erosions. Sheet erosion, which is the first form of erosion, occurs in upstream regions of the catchment and gradually changes to gully erosion as it progresses to downstream regions. To assign fuzzy and entropy weights to these forms of erosion, an initial score from 0 to 10 is first assigned to them (). It should be noted that a 0 score refers to areas in which erosion has not occurred. Therefore, in the fuzzy entropy weight method, EquationEq. (1)(1)
(1) is used for measuring this index, where the threshold value of the function is zero (the score of the area without erosion) and the final threshold value is related to the gully erosion score (i.e. the final threshold of land degradation). Accordingly, the fuzzy map related to erodability ranges from about 0.625 to 1. The entropy of this index ranges from 0.33 to 0.46 and 0.33 to 0.47 in the presence and absence of check dams, respectively ().
Table 1. Initial scoring of the erosion index (Ildoromi et al. Citation2019).
Plan curvature is the curvature of the imaginary line passing through a particular pixel that can, under certain conditions, function as the drainage point of the hillslopes (Zaharia et al. Citation2017; Siahkamari et al. Citation2018; Costache Citation2019). Therefore, fuzzy logic and EquationEq. (1)(1)
(1) were used to assign a weight to this index, such that pixels with high plan values would have a membership degree of about 1. The entropy of this index is changed from 0 to 0.463 and 0 to 0.464 in the absence and the presence of check dams, respectively ().
Profile curvature, defined as the surface curvature in the maximum slope direction, plays a major role in the surface flow discharge velocity. Ponding will occur in regions with negative profile values, also referred to as convex regions (Zaharia et al. Citation2017; Siahkamari et al. Citation2018; Costache Citation2019). Therefore, in this study, EquationEq. (2)(2)
(2) is used in the profile shape index in a way that higher profile index values indicate less significant flood hazards. EquationEquations (5)
(5)
(5) and Equation(6)
(6)
(6) were used for this index in the entropy weight method in the range of 0–0.46 and 0–0.461 in the presence and absence of check dams, respectively ().
The slope parameter, defined as the elevation gradient, plays an important role in surface and sub-surface hydrological processed such as flow direction, water table depth and flood hazard potential estimation of different regions in the study area (Fernández and Lutz Citation2010; Kazakis et al. Citation2015; Siahkamari et al. Citation2018). Since low-slope regions serve as ponding areas, the fuzzy scoring of this index is similar to the profile shape, that is low-slope areas have higher fuzzy scores than those with steep slopes. Using EquationEqs. (4)(4)
(4) and (6), the entropy of this index ranges from 0 to 0.48 and 0 to 0.49 in the presence and absence of check dams, respectively ().
Distance to discharge channel is an important concern in the occurrence of a flood event (Fernández and Lutz Citation2010; Kazakis et al. Citation2015; Sepehri et al. Citation2017; Siahkamari et al. Citation2018). In most flood hazard studies, this index is considered alone without any internal weights. For example, there is no distinction between upstream and downstream drainage networks. However, downstream areas in the drainage networks play a key role in flood hazards due to their low slope and great width and depth. Accordingly, four sub-indices of the drainage network in slope, fractal dimension, stream order and the impact angle of the drainage network were considered to determine the distance-from-river index. Weights were assigned to these sub-indices using only fuzzy membership degree.
In mathematics, fractal is used to describe irregularly shaped or complex natural objects. In fact, fractal is defined as an object or quantity, which is almost technically self-similar in all scales. Mandelbrot (Citation1982) showed that the computational accuracy of the total length of the coastline of Greta Britain, calculated by L = Nr, is dependent on the length measurement scale (r) in the sense that by decreasing the size of r, the number of measurement scales (N) and the computational accuracy of the total length (L) will increase. It should be noted that this linear equation is suitable for unbranched objects such as individual rivers or coastlines. Therefore, it is better to use the following equations for complex features such as drainage networks:
(8)
(8)
(9)
(9)
In this article, the box counting method of Fractalyse 2.4.1 was applied to assess the drainage network and determine its fractal dimension. This method is similar to the environmental measurement method applied in the above example to calculate the length of the British coastline. The authors of this study placed all the drainage sub-catchments of the study area on a gridded plate of specific dimensions and then proceeded to count the grids (N) in which the drainage network was located. Similarly, the same procedure was repeated for other grids with different sizes (r). It should go without saying that decreasing the grid size results in an increase in the number of grids in which the drainage network is available. In the box counting method, a linear equation is obtained by placing log (N) and log (r) on the y and x-axes, respectively, whose slope is equals to the fractal dimension. The fractal dimension ranges from 1 to 2, with 1 denoting features that are linear and non-branching. The fractal dimension approaches 2 by branching the drainage network or other features. Given the increase of flood hazard rate by increasing the fractal dimension values of the drainage network, EquationEq. (1)(1)
(1) was used for fuzzy scoring of this sub-index () (Ildoromi et al. Citation2019).
Figure 5. Weight values of the distance to the discharge channel: (a) Fractal dimension factor; (b) impact angle of drainage network lines; (c) local channel slope and (d) Stream order.
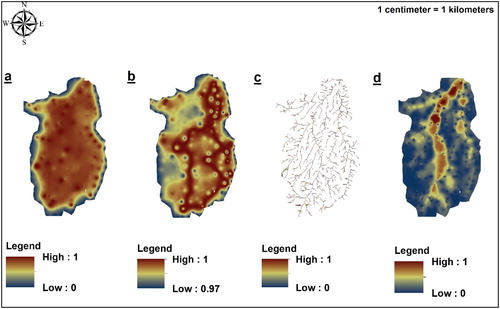
The impact angle of the drainage network lines is one of the sub-indices that can contribute significantly to creating flood hazards, which was obtained using the linear directional mean (LDM) function in ArcGIS 10.1 (EquationEq. 10
(10)
(10) ).
(10)
(10)
It is worth noting that in the fractal method, the impact angle of the drainage network lines lies in the fractal dimension. Additionally, only the relationship between fractal dimension and flood hazard is examined in flood hazard studies. Therefore, the fractal dimension values vary for a line with a fixed length and different angles. Regarding a line that is perpendicular to the horizontal or vertical axis of the plate, the fractal dimension is at its lowest value. As a result, the fractal dimension increases by changing the angle to 45°. However, this does not apply to flood hazard studies. In order to estimate the sub-index of the impact angle of the drainage network, we used the LDM equation. The entire study area was first classified into two main sub-catchments according to the Digital Elevation Model (DEM) map. The main drainage network route in each sub-catchment was then extracted using a DEM. Finally, a map was prepared that corresponded to the impact angle of the drainage network lines towards the north in the clockwise direction. This was done based on the route and direction of the main drainage network and by determining the impact of sub-catchment drainage network lines on the main drainage. This map was in the range of 1.5–349°. The majority of the flooding sites are naturally drainage networks that are discharged into the main drain with a 180° angle. Therefore, the fuzzy Gaussian function was used to weigh this index () (Ildoromi et al. Citation2019).
The local channel slope is defined as a change in the upstream altitude and route length (Kalantari et al. Citation2014). In this study, this sub-index was prepared using the drainage network and slope maps. First, a 5-m buffer was placed around each selected point (pixel) along drainage network lines. The average gradient per pixel along the drainage network route and inside the buffer was then calculated. EquationEquation (2)
(2)
(2) was used to calculate the fuzzy scores of this sub-index () (Ildoromi et al. Citation2019).
Stream order, which classifies drainage networks in terms of ranks on the basis of their direct relationship with sub-catchment dimensions, channel dimensions and drainage network discharge, plays a key role in hydrodynamic characteristics of a watershed. Given the upstream-to-downstream flow of water, the stream order value increases. Therefore, EquationEq. (2)
(2)
(2) was used to assign weights to this sub-index () (Ildoromi et al. Citation2019).
Finally, giving the reduction of flood hazards by increasing the distance to the discharge channel, EquationEq. (2)(2)
(2) was used to assign fuzzy weights to this index. Using EquationEqs. (5)
(5)
(5) and (6), the entropy of this index ranges from 0 to 0.47 and 0 to 0.49 in the presence and absence of check dams, respectively ().
Soil type and land use provides two main indices that influence the hydrological response of watersheds such as permeability characteristics (Wang et al. Citation2007; Skilodimou et al. Citation2019). In the study area, both indices had a low diversity, meaning that the study area was limited to loam and clay loam soil textures. The loam soil texture in the study area was classified based on the hydrological status, which is determined considering the surface soil texture, soil depth, surface vegetation cover and organic matter. This classification yields two groups: loam soil texture with the hydrological status D and loam soil texture with the hydrologic status C. In the next step, these two soil textures were divided into 10 different classes based on five classes of slope variation calculated according to the Jenks Natural Breaks Classification method (In this method, the classes are distinguished based on the natural gradient of the data.). In the study area, land use was limited to rangelands and gardens. Therefore, land use and soil texture indices cannot show the distribution of flood hazard in the region accurately owing to the lack of proper spatial distribution. In order to solve this problem, an initial scoring system was developed from 1 to 10 (from the lowest to the highest flood hazard impact). Next, a number from 0 to 10 was assigned to each index and sub-index based on their role and significance. Finally, land use and soil texture indices were integrated using the permutation law to create a new map (i.e. STLU) (). Since large values of this map indicate greater flood hazard impacts, EquationEq. (1)(1)
(1) was used to assign fuzzy weights to this index. Using EquationEqs. (4)
(4)
(4) and (6), the entropy of this index ranges from 0.04 to 0.46 and 0.04 to 0.48 in the absence and presence of check dams, respectively () (Ildoromi et al. Citation2019).
Table 2. Initial scoring of the STLU indices (Ildoromi et al. Citation2019).
The TWI is a marker of topographic effects on water saturation rate, which has a strong direct correlation with flood degree (EquationEq. 11(11)
(11) ). Topographic wetness index values, which vary from 0 to 20, depending on such characteristics as landscape parameters and the hydrological response of the area to heavy rainfall and ground-based flow. Accordingly, EquationEq. (1)
(1)
(1) was used to assign fuzzy weights to this index. Using EquationEqs. (4)
(4)
(4) and (6), the entropy of this index ranges from 0 to 0.43 and 0 to 0.44, in the absence and presence of check dams, respectively () (Wang et al. Citation2015; Sepehri et al. Citation2017; Tehrany et al. Citation2019).
(11)
(11)
Where WI is the wetness index; As is the local upslope contributing area (m2) from the flow accumulation raster and B is the local slope angle (°).
3.1. Advantages and disadvantages of the adopted method
In an evaluation system, the MCDM model is generally employed to prioritize options from the most to the least effective (Fernández and Lutz Citation2010). In this study, the fuzzy logic and entropy weight method, which are widely used in solving multiple-criteria decision problems as well as in sustainability and natural hazard analyses, were used for flood hazard zoning. One of the most important limitations in multi-criteria decision methods involves the subject of uncertainty, which can lead to major errors in the study objectives. According to Smithson (Citation1989) and Sepehri et al. (Citation2019b), fuzzy logic and entropy weight are classified among objective methods for weight assignment to indices that eliminate any uncertainty. This is contrary to the subjective methods specified in the introduction (such as AHP and SPA), where weights are solely determined according to the preference of decision makers. On the other hand, the disadvantages of these methods lie in their disregard for the significance of indices relative to each other and the target.
After assigning weights to indices using the fuzzy logic and entropy methods as well as providing a preliminary flood hazard map of the study area in the presence and absence of check dams, it is necessary to introduce a classification table of flood hazard risks in five flood susceptibility categories of ‘very high’, ‘high’, ‘moderate’, ‘low’ and ‘very low’. In this study, the internal values of the classification table were determined using the variation of flood hazard rates in the presence of check dams according to the Jenks Natural Breaks Classification method. The boundary conditions of this table, representing the maximum and minimum flood hazard, was determined using the maximum and minimum rates of the two initial flood hazard maps, respectively (Mahmoud and Gan Citation2018).
Finally, shows a flood hazard classification map in the absence of check dams. Approximately, 26.7% of the total study area is located in the ‘very high’, 39.7% in the ‘high’, 22.7% in the ‘moderate’, 8.6% in the ‘low’ and 2.2% in the ‘very low’ hazard zones.
Figure 6. (a): Flood map classification in the absence of check dams and (b) flood map classification in the presence of check dams.
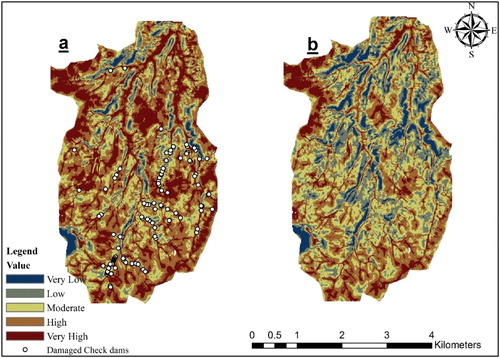
As shown in , the high-risk areas are mainly located in the downstream regions of the study area near drainage networks. In order to verify the accuracy of the map, the check dams destroyed by flood events were adapted to the map. Out of 102 destroyed check dams shown in , 43 and 40 dams were located in very-high-risk and high-risk zones, respectively. Out of the remaining 19 destroyed check dams, 15 were in moderate-risk and 4 in low-risk zones. Given that about 81.3% of the check dams were located in very-high-risk and high-risk zones, the flood map can be argued to be adequately accurate.
The flood hazard classification map in the presence of check dams indicates that 12.2%, 29.6%, 31.2%, 19.6% and 7.3% of the total study area are located in very-high, high, moderate, low and very-low hazard zones, respectively (). Comparison of the results of the two flood hazard classification maps demonstrates that the construction of check dams reduces the percentage of very-high to moderate hazard zones in the absence of check dams, whereas the percentage of low and very-low hazard zones increases.
4. Conclusion
Flood events, as devastating phenomena, may occur at any location. Flood control with a series of appropriate management measures is a necessary step in disaster management. Flood-susceptible areas should be identified to allow forecast and analysis for effective flood management measures in the future. In this study, the fuzzy logic and entropy weight methods were used to assess flood hazards and determine the role of check dams in flood risk zoning. Factors associated with topographic (i.e. plan shape, profile shape and slope), hydrologic (i.e. distance to the discharge channel, STLU and TWI) and human (i.e. erosion and check dam) characteristics were considered as flood-zoning assessment indices. In the next step, the change-point characteristics were used to assess the role of check dams in reducing flood hazards. The results showed that the area of ‘very low’, ‘low’ and ‘moderate’ flood hazard zones increased from about 2.23% to 7.34%, 8.62% to 19.62% and 22.69% to 31.23% after the construction of check dams, respectively. Moreover, the area of ‘high’ and ‘very high’ flood hazard zones decreased from 39.76% to 29.57%, and 26.69% to 12.24%, respectively. Although the results of this assessment are not quantitative, they can be used as a useful tool to the advantage of decision makers to implement similar measures.
Disclosure statement
No potential conflict of interest was reported by the authors.
References
- Abbasi NA, Xu X, Lucas-Borja ME, Dang W, Liu B. 2019. The use of check dams in watershed management projects: examples from around the world. Sci Total Environ. 676:683–691.
- Al-Juaidi AE, Nassar AM, Al-Juaidi OE. 2018. Evaluation of flood susceptibility mapping using logistic regression and GIS conditioning factors. Arab J Geosci. 11:765.
- Boix‐Fayos C, de Vente J, Martínez‐Mena M, Barberá GG, Castillo V. 2008. The impact of land use change and check‐dams on catchment sediment yield. Hydrol Process J. 22:4922–4935.
- Bombino G, Gurnell A, Tamburino V, Zema D, Zimbone S. 2008. Sediment size variation in torrents with check dams: effects on riparian vegetation. Ecol Eng. 32(2):166–177.
- Bombino G, Gurnell A, Tamburino V, Zema D, Zimbone S. 2009. Adjustments in channel form, sediment calibre and vegetation around check‐dams in the headwater reaches of mountain torrents, Calabria, Italy. Earth Surf Process Landf. 34:1011–1021.
- Chakraborty A, Joshi P. 2016. Mapping disaster vulnerability in India using analytical hierarchy process. Geomat Nat Hazards Risk. 7(1):308–325.
- Chapi K, Singh VP, Shirzadi A, Shahabi H, Bui DT, Pham BT, Khosravi K. 2017. A novel hybrid artificial intelligence approach for flood susceptibility assessment. Environ Model Softw. 95:229–245.
- Costache R. 2019. Flash-flood Potential Index mapping using weights of evidence, decision Trees models and their novel hybrid integration. Stoch Environ Res Risk Assess. 33(7):1375–1402.
- Elmahdy SI, Mostafa MM. 2013. Natural hazards susceptibility mapping in Kuala Lumpur, Malaysia: an assessment using remote sensing and geographic information system (GIS). Geomat Nat Hazards Risk. 4(1):71–91.
- Fernández D, Lutz M. 2010. Urban flood hazard zoning in Tucumán Province, Argentina, using GIS and multicriteria decision analysis. Eng Geol. 111(1–4):90–98.
- FitzHugh TW, Vogel RM. 2011. The impact of dams on flood flows in the United States. River Res Appl. 27(10):1192–1215.
- Gan B-R, Liu X-N, Yang X-G, Wang X-K, Zhou J-W. 2018. The impact of human activities on the occurrence of mountain flood hazards: lessons from the 17 August 2015 flash flood/debris flow event in Xuyong County, south-western China. Geomat Nat Hazards Risk. 9(1):816–840.
- Ge H, Huang Z, Wang Y, Li J. 2013. Application of fuzzy optimization model based on entropy weight in typical flood hydrograph selection. J Hydrol Eng. 18(11):1400–1407.
- Gigović L, Pamučar D, Bajić Z, Drobnjak S. 2017. Application of GIS-interval rough AHP methodology for flood hazard mapping in urban areas. Water. 9(6):360.
- Guo E, Zhang J, Ren X, Zhang Q, Sun Z. 2014. Integrated risk assessment of flood disaster based on improved set pair analysis and the variable fuzzy set theory in central Liaoning Province, China. Nat Hazards. 74(2):947–965.
- Hazarika N, Barman D, Das A, Sarma A, Borah S. 2018. Assessing and mapping flood hazard, vulnerability and risk in the Upper Brahmaputra River valley using stakeholders’ knowledge and multicriteria evaluation (MCE). J Flood Risk Management. 11:S700–S716.
- Ildoromi AR, Sepehri M, Malekinezhad H, Kiani-Harchegani M, Ghahramani A, Hosseini SZ, Artimani MM. 2019. Application of multi-criteria decision making and GIS for check dam Layout in the Ilanlu Basin, Northwest of Hamadan Province, Iran. Phys Chem Earth, Parts A/B/C. doi: 10.1016/j.pce.2019.10.002
- Jeon J-J, Sung JH, Chung E-S. 2016. Abrupt change point detection of annual maximum precipitation using fused lasso. J Hydrol. 538:831–841.
- Kalantari Z, Nickman A, Lyon SW, Olofsson B, Folkeson L. 2014. A method for mapping flood hazard along roads. J Environ Manag. 133:69–77.
- Kawachi T, Maruyama T, Singh VP. 2001. Rainfall entropy for delineation of water resources zones in Japan. J Hydrol. 246(1–4):36–44.
- Kazakis N, Kougias I, Patsialis T. 2015. Assessment of flood hazard areas at a regional scale using an index-based approach and Analytical Hierarchy Process: application in Rhodope–Evros region, Greece. Sci Total Environ. 538:555–563.
- Kia MB, Pirasteh S, Pradhan B, Mahmud AR, Sulaiman WNA, Moradi A. 2012. An artificial neural network model for flood simulation using GIS: Johor River Basin, Malaysia. Environ Earth Sci. 67(1):251–264.
- Lai C, Chen X, Chen X, Wang Z, Wu X, Zhao S. 2015. A fuzzy comprehensive evaluation model for flood risk based on the combination weight of game theory. Nat Hazards. 77(2):1243–1259.
- Langdale GW, Shrader W. 1982. Soil erosion effects on soil productivity of cultivated cropland. Chapter 4 in Determinants of soil loss tolerance. American Society of Agronomy special publication no. 45. Madison, WI: American Society of Agronomy, Soil Science Society of America.
- Li S, Yu P, Sun S, Wang Y-R. 2010. Entropy weight based fuzzy matter element model for evaluating and zoning of regional flood disaster vulnerability. J Nat Disaster. 19:124–131.
- Liu J-F, Li J, Liu J, Cao R. 2008. Integrated GIS/AHP-based flood risk assessment: a case study of Huaihe River Basin in China. J Nat Disaster. 17:110–114.
- Lotfi FH, Fallahnejad R. 2010. Imprecise Shannon’s entropy and multi attribute decision making. Entropy. 12(1):53–62.
- Ma X, Lu X, Van Noordwijk M, Li J, Xu J. 2014. Attribution of climate change, vegetation restoration, and engineering measures to the reduction of suspended sediment in the Kejie catchment, southwest China. Hydrol Earth Syst Sci. 18(5):1979–1994.
- Mahmoud SH, Gan TY. 2018. Multi-criteria approach to develop flood susceptibility maps in arid regions of Middle East. J Clean Prod. 196:216–229.
- Malekinezhad H, Talebi A, Ilderomi AR, Hosseini SZ, Sepehri M. 2017. Flood hazard mapping using fractal dimension of drainage network in Hamadan City, Iran. J Environ Eng Sci. 12(4):86–92.
- Mamedov AI, Levy GJ. 2019. Soil erosion–runoff relations on cultivated land: insights from laboratory studies. Eur J Soil Sci. 70(3):686–696.
- Mandelbrot BB. 1982. The fractal geometry of nature. New York: WH Freeman.
- Matheswaran K, Alahacoon N, Pandey R, Amarnath G. 2019. Flood risk assessment in South Asia to prioritize flood index insurance applications in Bihar, India. Geomatic Nat Hazard Risk. 10(1):26–48.
- Militino AF, Ugarte MD, Pérez-Goya U. 2018. Detecting change-points in the time series of surfaces occupied by pre-defined NDVI categories in continental Spain from 1981 to 2015. In: The mathematics of the uncertain. Switzerland: Springer; p. 295–307.
- Mizuyama T. 2008. Structural countermeasures for debris flow disasters. IJECE Eng. 1(2):38–43.
- Morgan RP, Rickson RJ. 2003. Slope stabilization and erosion control: a bioengineering approach. New York, NY: Taylor & Francis.
- Novelo-Casanova DA, Rodríguez-Vangort F. 2016. Flood risk assessment. Case of study: Motozintla de Mendoza, Chiapas, Mexico. Geomatic Nat Hazard Risk. 7(5):1538–1556.
- Pimentel D, Burgess M. 2013. Soil erosion threatens food production. Agriculture. 3(3):443–463.
- Quinteiro P, Van de Broek M, Dias AC, Ridoutt BG, Govers G, Arroja L. 2017. Life cycle impacts of topsoil erosion on aquatic biota: case study on Eucalyptus globulus forest. Int J Life Cycle Assess. 22(2):159–171.
- Razavi T, Coulibaly P. 2013. Streamflow prediction in ungauged basins: review of regionalization methods. J Hydrol Eng. 18(8):958–975.
- Roshani R. 2003. Evaluating the effect of check dams on flood peaks to optimise the flood control measures (Kan case study in Iran). Enschede: The Netherlands: International Institute for Geo-information Science and Earth Observation. 54 p.
- Sepehri M, Ildoromi AR, Malekinezhad H, Hosseini SZ, Talebi A, Goodarzi S. 2017. Flood hazard mapping for the gonbad chi region, Iran. J Environ Eng Sci. 12(1):16–24.
- Sepehri M, Malekinezhad H, Hosseini SZ, Ildoromi AR. 2019a. Suburban flood hazard mapping in Hamadan city, Iran. Proc Inst Civil Eng. doi: 10.1680/jmuen.17.00029
- Sepehri M, Malekinezhad H, Hosseini SZ, Ildoromi AR. 2019b. Assessment of flood hazard mapping in urban areas using entropy weighting method: a case study in Hamadan city, Iran. Acta Geophys. 67(5):1435–1449.
- Sepehri M, Malekinezhad H, Ilderomi AR, Talebi A, Hosseini SZ. 2018. Studying the effect of rain water harvesting from roof surfaces on runoff and household consumption reduction. Sustain Cities Soc. 43:317–324.
- Shieh C-L, Guh Y-R, Wang S-Q. 2007. The application of range of variability approach to the assessment of a check dam on riverine habitat alteration. Environ Geol. 52(3):427–435.
- Siahkamari S, Haghizadeh A, Zeinivand H, Tahmasebipour N, Rahmati O. 2018. Spatial prediction of flood-susceptible areas using frequency ratio and maximum entropy models. Geocarto Int. 33(9):927–941.
- Singh V. 1997. The use of entropy in hydrology and water resources. Hydrol Process. 11(6):587–626.
- Sivapalan M. 2003. Prediction in ungauged basins: a grand challenge for theoretical hydrology. Hydrol Process. 17(15):3163–3170.
- Skilodimou HD, Bathrellos GD, Chousianitis K, Youssef AM, Pradhan B. 2019. Multi-hazard assessment modeling via multi-criteria analysis and GIS: a case study. Environ Earth Sci. 78(2):47.
- Smithson M. 1989. Cognitive science. In: Ignorance and uncertainty: emerging paradigms. New York, NY: Springer-Verlag Publishing. http://dx.doi.org/10.1007/978-1-4612-3628-3.
- Smith K, Ward R. 1998. Mitigating and Managing Flood Losses. Floods: Physical Processes and Human Impacts. Chichester: John Wiley & Sons.
- Stefanidis S, Stathis D. 2013. Assessment of flood hazard based on natural and anthropogenic factors using analytic hierarchy process (AHP). Nat Hazards. 68(2):569–585.
- Tehrany MS, Jones S, Shabani F. 2019. Identifying the essential flood conditioning factors for flood prone area mapping using machine learning techniques. Catena. 175:174–192.
- Wagener T, Wheater H, Gupta HV. 2004. Rainfall-runoff modelling in gauged and ungauged catchments. Singapore: World Scientific.
- Wang G, Liu J, Kubota J, Chen L. 2007. Effects of land‐use changes on hydrological processes in the middle basin of the Heihe River, northwest China. Hydrol Process. 21(10):1370–1382.
- Wang Z, Lai C, Chen X, Yang B, Zhao S, Bai X. 2015. Flood hazard risk assessment model based on random forest. J. Hydrol. 527:1130–1141.
- Wu C, Wang Y, Tang Y, Huang Q, Jin J. 2012. Variable fuzzy recognition model for the flood hazard assessment based on Set Pair Analysis. J Northwest A F Univ. 40:221–226.
- Wu M, Ge W, Li Z, Wu Z, Zhang H, Li J, Pan Y. 2019. Improved set pair analysis and its application to environmental impact evaluation of dam break. Water. 11(4):821.
- Xie P, Gu H, Sang Y-F, Wu Z, Singh VP. 2019. Comparison of different methods for detecting change points in hydroclimatic time series. J Hydrol. 577:123973.
- Yazdi J, Moghaddam MS, Saghafian B. 2018. Optimal design of check dams in mountainous watersheds for flood mitigation. Water Res Manage. 32(14):4793–4811.
- Yin H, Li C. 2001. Human impact on floods and flood disasters on the Yangtze River. Geomorphology. 41(2–3):105–109.
- Zadeh LA, Klir GJ, Yuan B. 1996. Fuzzy sets, fuzzy logic, and fuzzy systems: selected papers. Singapore: World Scientific.
- Zaharia L, Costache R, Prăvălie R, Ioana-Toroimac G. 2017. Mapping flood and flooding potential indices: a methodological approach to identifying areas susceptible to flood and flooding risk. Case study: the Prahova catchment (Romania). Front Earth Sci. 11(2):229–247.
- Zema DA, Bombino G, Denisi P, Lucas-Borja ME, Zimbone SM. 2018. Evaluating the effects of check dams on channel geometry, bed sediment size and riparian vegetation in Mediterranean mountain torrents. Sci Total Environ. 642:327–340.
- Zeng R, Chen H-K, Li J-Y. 2010. Fuzzy comprehensive evaluation method based on entropy in road flood risk assessment. Chongqing Jiotong Daxue Xuebao (Ziran Kexue Ban). 29:587–591.
- Zhou Q, Leng G, Huang M. 2018. Impacts of future climate change on urban flood volumes in Hohhot in northern China: benefits of climate change mitigation and adaptations. Hydr and Ear Syst Scie. 22:305–316.