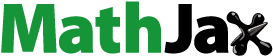
Abstract
Interferometric Synthetic Aperture Radar (InSAR) is an important method for acquiring surface deformation. Considering the difficulty of the identification work, the identification of landslides needs to be combined with the context of the pregnant disaster and the precipitating conditions. To identify potential landslides, we applied spatial and temporal filtering to the InSAR results, which consists of rainfall and landslide susceptibility mapping. In this paper, taking the Badong Ecological Barrier Zone of the Three Gorges reservoir area as the study area, the deformation aggregation areas in the study area were obtained by applying Small Baseline Subset InSAR (SBAS-InSAR) technology and spatial statistical analysis. We screened deformation aggregation areas by combining the susceptibility map and the correlation analysis of rainfall and deformation. Field verification and investigation were conducted on the suspected deformation areas, and 11 landslides were found to have signs of deformation, two of them are newly discovered landslides. In addition, we selected one of the landslides, the Songjiawuchang landslide, and compared the InSAR results with the GPS accumulated displacement to verify the reliability of the results. This research demonstrated the feasibility of combining InSAR results with spatial susceptibility maps and monthly rainfall factors for landslides identification methods.
Applied Spatial filtering and temporal filtering on the InSAR results.
Evaluated in conjunction with geological hazards themselves.
Landslide identification accuracy significantly improved.
Key policy highlights
1. Introduction
Identification of potential landslides is essential for disaster mitigation and prevention. InSAR is a new spatial surface measurement technology, that is unaffected by natural weather effects and can observe the surface at all hours of day and night, allowing it to be used for the identification of potential landslides. InSAR technology can collect high-resolution and high-precision information on small surface deformations over a large area of the ground. Applying InSAR to identify potential hidden hazards in key areas, we can realize the investigation of key areas from the perspective of time and space, thus providing enough reference basis for the prevention and control of regional geological hazards (Akbarimehr et al. Citation2013; Medhat et al. Citation2022; Milillo et al. Citation2022; van Natijne et al. Citation2022). Many researchers have already applied InSAR technology to identify regional landslides, update landslide inventory maps, determine potential landslide extent, and identify potential landslides precursor features (Intrieri et al. Citation2018; Devara et al. Citation2021; Guo et al. Citation2021; Su et al. Citation2021).
Some key challenges are still in the use of InSAR for landslides identification, such as the low-density scatterers in mountainous areas, error sources masking the displacement signals, deformation in line-of-sight, define of warning thresholds, and deformation patterns (Schlögl et al. Citation2022). The SAR image and the range of applications for InSAR technology make it clear that it is not sufficient to identify a geological hazard solely on the basis of InSAR results. It is required to increase the identification accuracy by doing a fundamental analysis of the study region, in addition to the conditions of the study area that foster disasters and the landslide’s contributing causes (Ge et al. Citation2019).
To investigate a novel idea of universal landslide hazard, it is necessary to create a comprehensive hazard identification system using multi-source data and multi-dimensional monitoring. In this system, we can start from two aspects, one is the accuracy of InSAR for landslide identification, and the other is the development environment of landslide. Most scholars focus on one perspective, and few carry out landslide identification from both perspectives. In terms of the analysis of the pregnant environment, landslide susceptibility is a good choice (Singh et al. Citation2005; Ciampalini et al. Citation2015; Rosi et al. Citation2018; Novellino et al. Citation2021; Hussain et al. Citation2022). The combination of InSAR and landslide susceptibility is a regional disaster research method. Landslide susceptibility has been employed as one of the key ways to examine regional landslide differentiation and has important recommendations for the regional control of landslides (Wang et al. Citation2020). Landslide susceptibility can be used to determine the likelihood of a landslide occurring in the research region and can be used to offer a basic guarantee for landslide detection.
The concept of integrating spatial and temporal filtering was used in this paper to identify potential landslides in the Badong ecological barrier zone. Spatial filtering is the process of implementing landslide susceptibility to filter the research area’s low-susceptibility landslide zones. The use of Pearson correlation coefficients to filter areas where deformation is little correlation with precipitation during the study period is known as temporal filtering. In the identification process, four technical approaches namely, SBAS-InSAR technology, GIS spatial analysis, Pearson correlation coefficient, and landslide susceptibility analysis were mostly used. The identification technique that combines spatial and temporal filtering complements the shortage of single information to identify landslides and improves the accuracy of detecting active landslides. Additionally, based on monthly rainfall and Global Positioning System (GPS) monitoring data, the time-series deformation of the Songjiawuchang landslide was examined, and the findings not only support the accuracy of the identification results but also aid in landslide prevention and warning.
2. Study area and data
2.1. Study area
The Badong Ecological Barrier Zone is in the northern part of Badong County, Hubei Province, China, and covers the Yangtze River’s main channel and tributaries, with longitudes ranging from 110°5′E to 110°29′E and latitudes ranging from 31°16′N to 30°57′N, covering an area of around 309 km2. The barrier zone has a well-developed water infrastructure and is a highly populated area as well as an important ecological zone. The research area is part of the Chuan-E folded mountainous area, with high topography from the north and south and low terrain in the centre. The geological conditions in the area are complex, with abundant precipitation and strong human engineering activities, resulting in frequent geological disasters (Fourniadis et al. Citation2007; Juang Citation2021). The lithology of the exposed strata is mudstone, sandstone interspersed with marl and tuff of the Middle Triassic Badong Formation (T2b). The slope structure type is soft and hard rocky with a compliant slope. Due to the deep cutting of the Yangtze River and the strong human economic and engineering activities, the landslide is extremely developed, like most large and medium-sized landslides. In total, there are 242 landslides in the area (). Statistically, 174 landslides’ deformation was mainly caused by rainfall. Considering that the InSAR method can be useful for extremely slow and very slow landslide deformation monitoring during the primary and secondary stages (Xiong et al. Citation2020), we aimed to identify landslides that produce slow deformation under the influence of rainfall.
Figure 1. (a) Location of the Three Gorges reservoir area and Badong County. The star indicates the location indication of the study area in the Three Gorges reservoir area. (b) Badong County and ALOS-2 PALSAR-2 images coverage. (c) Shape location of the study area, and the distribution of landslides and water systems in the study area.
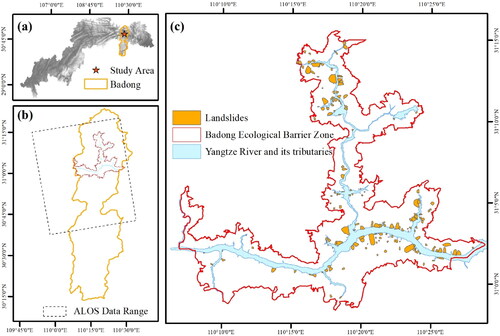
2.2. SAR data
This study used ALOS-2 PALSAR-2 images in the L-band (1.2 GHz band) with Ultra-Fine mode, HH + HV polarization, greater than 3 m resolution, and a 55 km × 70 km width. PALSAR-2 images have the advantage of high resolution and strong penetration, which is useful for detecting small deformations in the disaster body and has better application benefits in monitoring areas with dense foliage cover (Suzuki et al. Citation2011). The SAR data is available from 2020/4/11 to 2021/8/14 and a total of 14 frames of SAR images were utilized (), with a centre incident angle of 42.9°.
Table 1. ALOS-2 PALSAR-2 images schedule.
2.3. Landslide causative factors
The Geological Environmental Center of Hubei Province provided the research area’s landslide inventory map, which included 182 sites, and this landslide inventory is official data that has been manually fielded and verified. The 182 landslides were then digitized and rasterized in the Environmental Systems Research Institute (ESRI)’s ArcGIS software (version 10.5.0) at a spatial resolution of 12.5 m, which was the same as the Digital Elevation Model (DEM) data used in this study.
The spatial location of landslide occurrence is predicted via landslide susceptibility mapping. For the susceptibility analysis of landslides, six factors that are more important for landslides were selected based on the information from previous studies, including elevation, slope, Topographic Wetness Index (TWI), lithology groups, land use/land cover, and Normalized Difference Vegetation Index (NDVI) (Chen et al. Citation2018; Mallick et al. Citation2018; Rong et al. Citation2020). The study’s DEM data sources were ALOS PALSAR 12.5 m data from Alaska Satellite Facility-Distributed Active Archive Centers (ASF DAAC, https://search.asf.alaska.edu/#/) for extracting elevation, slope, and TWI; a 1:200,000 geological map for extracting lithology groups factor; 2017 landuse change survey data for extracting land use/land cover data; and NDVI was produced from two Landsat-8 Operational Land Imager (OLI) images (path/row 125/38, 125/39) obtained on September 18, 2020. To facilitate machine learning model training, the six variables were categorized according to the landslide frequencies (Niu et al. Citation2014; Chen et al. Citation2016), displayed hierarchically (), and assigned to the training datasets ().
Figure 2. Landslide causative factors maps: (a) Elevation; (b) slope; (c) NDVI; (d) lithology groups; (e) TWI; (f) land use/land cover. The lithology groups are described as follows: I: Hard medium to thick-bedded massive dolomite metamorphic conglomerate rock group; II-1: Hard medium to thickly laminated quartz sandstone quartzite fine sandstone rock group; II-3: Hard harder medium to thick-bedded sandstone mud siltstone interbedded with shale coal seams and mudstone shale interbedded rock group; II-4: Harder to softer thinly to moderately thickly bedded shale sandstone mudstone rock group; II-5: Soft thin to medium-thick bedded muddy siltstone shale rock group; III-1: Hard medium to thick-bedded strongly karsted carbonate rock group; III-2: Harder harder medium to thickly bedded strongly to moderately karsted carbonate rock group; III-3: Harder thin to medium-thick bedded weakly karsted carbonate rock group.
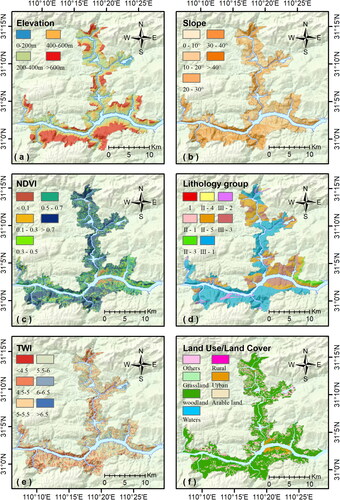
Table 2. Values for each category of causative factors.
As a precipitating factor, rainfall plays a crucial influence in the deformation of landslides (Bayer et al. Citation2018). The Hubei Provincial Hydrological and Water Resources Center supplied monthly precipitation data from 23 hydrological stations. The monthly precipitation distribution maps from April 2020 to July 2021 were created by interpolating the hydrological stations using the Spline tool in ArcGIS 10.5.0 software to assist the spatial calculation with the monthly cumulative deformation maps of InSAR. Interpolation predicts values for cells in a raster from a limited number of sample data points. The Spline tool uses an interpolation method that estimates values using a mathematical function that minimizes overall surface curvature, resulting in a smooth surface that passes exactly through the input points (Hadi and Tombul Citation2018).
3. Methodology
3.1. General method and technical route
In this research, we highlight SBAS-InSAR, rainfall and deformation correlation computation, obtaining landslide susceptibility, and identification as the four crucial steps in the process of identifying landslides. In general, the Pearson correlation coefficient can be used to characterize the temporal correlation between the deformation location of rainfall-type landslides and the rainfall (Liu et al. Citation2022). When we performed correlation analysis for each point of the study, the Pearson correlation coefficient result of rainfall and deformation can reflect the areas that vary over time. The spatial distribution probability of landslides in the studied area can be determined using landslide susceptibility zoning. The article filters the InSAR results in time and space using the Pearson correlation coefficient and the landslide susceptibility distribution map to obtain the final identification results.
To acquire the deformation aggregation areas in the research area, the spatial statistical analysis was first done utilizing the deformation rates obtained by SBAS-InSAR. Then, Pearson correlation coefficient analysis was done using monthly rainfall data and monthly cumulative deformation variables to create the distribution map of deformation correlation coefficients in the area. The GBDT model was used to calculate the influencing factors and landslide data set at the spatial distribution level to generate the landslide susceptibility distribution map of the barrier zone. Two steps make up the filtering procedure for the deformation aggregate region, one for the temporal level and the other for the spatial level. The first screening of deformation aggregation areas was performed at the time change level to reserve deformation areas with rainfall correlation. The second screening of deformation aggregation areas was performed at the spatial change level to reserve deformation areas with medium or high susceptibility. Finally, an outdoor geological survey of the final reserved deformation aggregation areas was conducted to obtain the landslides identification results of the study area ().
3.2. SBAS-InSAR
The SBAS-InSAR technique is used to extract surface deformations. It was formally proposed by Berardino et al. (Citation2002) to solve the decoherence problem caused by too-long spatial baselines in the D-InSAR technique. SBAS has become one of the most representative techniques for extracting time series surface deformation (Tizzani et al. Citation2007; Dong et al. Citation2014; Zhao et al. Citation2016; Novellino et al. Citation2017).
From the principle standpoint, the adoption of the least-squares principle and singular value decomposition is the most fundamental feature of the small baseline set technique (Berardino et al. Citation2002). The small baseline set technique screens the SAR images with short time and spatial baselines according to the threshold value solves the time-series surface deformation of each set using the least-squares principle, and then solves the least-squares solution in the sense of the smallest parametric value for all small baseline sets jointly, to the time-series surface deformation covering the entire monitoring period using the method of singular value decomposition. This technique interferometrically processes SAR images with a short time and space, which effectively avoids temporal and spatial decoherence of radar interferometric processing in the Badong Mountain area, increases the temporal sampling rate, improves the quality of interferograms, and also improves the comprehensive utilization rate of data in the current project with fewer time-series radar images, and ensures the timeliness and accuracy of surface deformation monitoring.
The following are the main processes in the SBAS-InSAR approach to extracting ground subsidence (Berardino et al. Citation2002). The experimental data were processed by Swiss GAMMA commercial software, and we did the atmospheric correction with GACOS (Jolivet et al. Citation2014). depicts the spatial and temporal baselines for SAR data, with a spatial baseline of 100 m and an unset temporal baseline. After ensuring that data alignment is within 0.1 pixels, differential interference is performed on all image pairs (60 pairs) with an adaptive filtering window of 128, and a stable point is chosen as the starting point for the deconvolution with a deconvolution threshold of 0.3, followed by phase transformation processing to obtain the results.
3.3. Spatial statistical analysis of InSAR rate result
We employed hot spot analysis () and kernel density analysis techniques to perform spatial statistical analysis of InSAR deformation rate results to identify deformation aggregation locations and find strong deformation sites (Morelli et al. Citation2011; Lu et al. Citation2019; Zhu et al. Citation2021).
The statistic is a local spatial statistic that measures the degree of spatial correlation within a defined range and can be used to evaluate the degree of spatial aggregation of deformation rates.
is calculated as follows
(1)
(1)
is the deformation rate value of the point target;
is the spatial of the weight of point targets i and j; n is the number of point targets in the total dataset. When
is positive (hot spot) and highly significant, it suggests a high value spatial aggregation; when
is negative (cold spot) and very significant, it indicates a low value spatial aggregation. The
of each point can be determined using hot spot analysis (Getis-Ord
). The greater the absolute value of
the stonger the aggregation relationship of the deformation points.
Kernel density analysis is frequently used to compute the density of elements in their immediate surroundings, and the results can reflect the degree of aggregation of the input data in terms of spatial arrangement. Based on a smooth surface can be fitted by applying kernel density analysis to generate the final deformation aggregation map, with the kernel density expression as
(2)
(2)
is the kernel density estimate; k () is the kernel density function; n is the number of existing points;
is the distance between the deformation rate point
and
h represents the search radius. The kernel density analysis of
score of deformation points can turn the area of significant movement of the aggregation of deformation points into an area of hot and cold spots with good visibility, highlighting the deformation area and more closely matching the actual landslide movement. High absolute density readings indicate unusual surface activity in this region, which could be caused by geological risks such as landslides.
3.4. Pearson correlation coefficient
According to the statistics, rainfall is the primary cause of the Badong ecological barrier. Locating the deformation area affected by rainfall in the high landslide area can improve the accuracy of landslides identification. Pearson’s correlation coefficient method is a statistical approach to measure the proximity of the relationship between two variables, which can be used for the spatial correlation between two variables in a given spatial environment, and then to provide an objective interpretation of the relationship between the variables (Buytaert et al. Citation2006; Di et al. Citation2013; Singh et al. Citation2020). In this work, the correlation between rainfall and deformation is determined using Pearson’s coefficient. Several sets of data can be obtained throughout time for two variables, rainfall and cumulative form variable
denoted as
then the mathematical expression of the correlation coefficient is:
(3)
(3)
In the formula, and
are the mean values of the
data, respectively. The correlation coefficient
ranges between
and
The closer
is to 1, the higher the degree of linear correlation between
and
If
it shows that
and
are fully linearly correlated; if
it indicates a completely negative linear correlation between
and
The degree of correlation can be categorized into the following cases based on the study scale: when
1, it is regarded as high correlation; when
it is regarded as medium correlation; when
it is regarded as low correlation; when
it means that the correlation between the two variables is extremely weak and can be regarded as non-linear correlation.
3.5. Landslide susceptibility mapping
Landslide susceptibility analysis is to analyze under what conditions landslides are most prone to occur, where the conditions include various characteristics, such as geological structure, topography, land use, slope structure, and meteorology and hydrology (Marjanović et al. Citation2011; Ghorbanzadeh et al. Citation2018).
Machine learning can be used to predict the probability of landslide occurrence and can achieve good results, which can provide a basis for landslide monitoring and forecasting. This experiment used the gradient boosting decision tree (GBDT) model (Yao et al. Citation2008; Tehrany et al. Citation2015; Tien Bui et al. Citation2016). GBDT is one of the best fitting algorithms for real-world distributions among traditional machine learning methods, with strong generalization capabilities. It can be used in both classification and regression problems, as well as in regular functions to improve training results and reduce model overfitting (Chen et al. Citation2020; Rong et al. Citation2020). Unlike the AdaBoost method, GBDT generates a weak classifier through multiple iterations, with each classifier being trained based on the residuals of the previous iteration. The weak classifiers are weighted and summed in each iteration to get the result. The GBDT model can be described as:
(4)
(4)
where
is the number of iterations,
is the weak classifier generated at each iteration, and
is the loss function, which can be described as:
(5)
(5)
is the current iteration, and the GBDT algorithm builds the parameters of the next classifier by minimizing
Each training round attempts to lower the loss function as much as possible to reach a locally optimal solution or a globally optimal solution. GBDT is highly generalizable and well-suited for classification and prediction. The GBDT algorithm was developed in Python by the scikit-learn library (Song et al. Citation2018).
After the landslide susceptibility values of each pixel in the study area are calculated by machine learning models, the landslide susceptibility is generally classified into four or five categories according to the natural breaks classification method, such as (a) no susceptibility, (b) low susceptibility, (c) moderate susceptibility, (d) high susceptibility, and (e) very high susceptibility (Zhao et al. Citation2020; Youssef and Pourghasemi Citation2021).
We implemented the GBDT model in python and evaluated the prediction accuracy of the landslide susceptibility model using the receiver operating characteristic (ROC) curve, also known as the perceptibility curve. The ROC curve is a composite indicator, showing the relationship between two continuous variables. Vertical coordinate means susceptibility and horizontal coordinate is specificity (Cantarino et al. Citation2019; Goyes-Peñafiel and Hernandez-Rojas Citation2021; Sun et al. Citation2021; Fustos-Toribio et al. Citation2022). The area under the curve is referred to as AUC, and the larger the AUC, the more accurate the model is.
4. Results
4.1. InSAR deformation results
The SBAS-InSAR approach was used to monitor the deformation of the barrier zone. shows the annual average deformation rate along the radar line of direction of sight in the research region from April 2020 to August 2021, with the maximum settlement rate of −148 mm/a. The locations with larger deformation are mostly found on the south bank of the Yangtze River and the eastern section of the north bank of the Yangtze River. The average coherence value of the 60 interference pairs was 0.580, with a maximum of 0.621 and a minimum of 0.541. The overall coherence of the images was good, demonstrating that L-band SAR images were more applicable in the lush vegetation of Badong. The deformation results are distributed throughout the study region. We performed hot spot analysis and kernel density analysis of deformation rate and obtained the kernel density of deformation rate. We circled the aggregation areas and obtained 27 deformation aggregation areas. We then performed landslides identification in these 27 deformation areas ().
Figure 5. (a) Annual average deformation rate along the radar line of sight direction. The negative value represents the displacement of the target feature away from the satellite direction, which is shown in red in the figure, and the positive value represents the displacement of the target feature close to the satellite direction, which is shown in blue in the figure. (b) Nuclear density map.
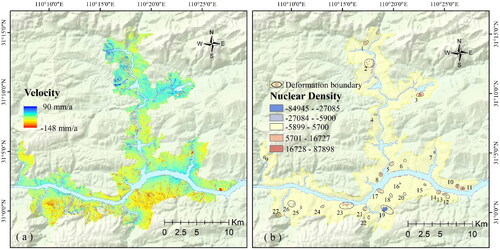
4.2. Pearson correlation analysis of rainfall and InSAR deformation results
Previously, the correlation calculation between rainfall and deformation was for a single point. In this experiment, we used GIS spatial analysis to calculate the correlation between rainfall and deformation for the whole region, which improves the visualization effect and facilitates the identification of potential landslides. The rainfall station data were interpolated to obtain the rainfall distribution maps for each month, together with the monthly accumulated deformation for the corresponding months as shown in . A raster calculation was performed for each pixel of the study area using the formula of Pearson’s correlation coefficient to obtain the correlation distribution of monthly accumulated deformation with rainfall for the period from April 2020 to July 2021 and as shown in the study area was classified into four categories according to the definition of Pearson coefficient. Eight of the 27 deformation aggregates are uncorrelated, seven have a low correlation, ten have a medium correlation and two have a high correlation. We performed the first filtering at the temporal level for the 27 deformation clusters. 19 clusters with a correlation greater than or equal to Low correlation were retained (No. 1, 2, 3, 5, 6, 7, 8, 9, 10, 11, 12, 13, 15, 16, 17, 18, 19, 23, 26).
4.3. Landslide susceptibility mapping results and analysis
This experiment generates a total of 1,741,207 points, of which 84,689 are landslide points and 1,656,518 are non-landslide points, based on a raster size of 12.5 m. We randomly selected 150,000 points for model training and testing (70% for training the GBDT model and 30% for verifying the model performance). A 2:3 ratio of slippery and non-slippery points (60,000 slippery points and 90,000 non-slippery points) came with the best results.
The obtained model was then used to calculate landslide susceptibility for the entire area points, yielding the largest area of the ROC curve and AUC = 0.89 ().
According to , highly susceptibility areas for landslides are mainly concentrated along the Yangtze River in the eastern part of the study area and the western bank of the Yangtze River tributaries in the north part of the study.
Of the 27 deformation aggregation areas, four were in the no-susceptibility area, six in the low susceptibility area, three in the moderate susceptibility area, two in the high-susceptibility area, and twelve in the very high susceptibility area. Of the 19 temporally filtered deformation aggregates, 13 deformation aggregates that are greater than or equal to moderate susceptibility were retained. According to , the secondary filtering was completed (No. 2, 3, 5, 6, 7, 8, 10, 11, 12, 15, 16, 19, 23).
4.4. Potential landslides identification results
27 deformation aggregation zones were circled using hot spot analysis of InSAR rate results. We extracted the Pearson correlation coefficients and sensitivities of the deformation aggregation zones, and the deformation zones with Pearson correlation coefficients greater than or equal to 0.2 and higher than medium susceptibility were retained. Through Pearson correlation coefficient results and susceptibility results, we screened 13 deformation aggregation areas caused by rainfall. Then those 13 areas were verified in the field, with two areas not being reached (No. 3, 5). The remaining 11 (No. 2, 6, 7, 8, 10, 11, 12, 15, 16, 19, 23) aggregation areas all had larger or smaller deformations after field verification and comparison with the survey data in 2020. Finally, after determining the locations of these 11 landslides with deformation, we refined the boundaries of the 11 landslides based on the survey data and high-resolution images and other information, and obtained the final identification results, as shown in .
In , we give the basis for our identification, with the minimum, maximum and mean values of the annual average deformation rate. The identification results include two new landslides, No. 12 and No. 19, along with nine historical landslides. In the direction away from the satellite, the absolute values of deformation rates of Dugongci landslide (Shijiapo) (No. 8), Songjiazhuang landslide (No. 23) and Songjiawuchang landslide (No. 11) are larger, exceeding 75 mm/a.
Table 3. Identifying landslides information table.
5. Discussion
5.1. Analysis of Songjiawuchang landslide deformation
The Songjiawuchang landslide in deformation zone No. 11, which has the larger deformation, was selected for deformation analysis (). The landslide of Songjiawuchang is located along the Yangtze River, with a slope direction of about 175°. Scholars used ground truth for results verification, e.g. GPS, levelling data, and Global Navigation Satellite System (GNSS) (Yin et al. Citation2010; Wasowski and Bovenga Citation2014; Dong et al. Citation2019). We selected the GPS points P1, P2, and P3 in the landslide body for the analysis, and the profiles are shown in . The landslide is rocky, and the landslide body is primarily made up of landslide debris interspersed with soil, forming a multi-phase sliding accumulation. As demonstrated in , the cumulative deformation curves of InSAR and GPS exhibit good agreement, and the trends are consistent, demonstrating the validity of the InSAR results of this experiment.
Figure 11. Songjiawuchang landslide deformation analysis figures. (a) The map of the whole landslide and the indication of the location of the A–A′ profile line; (b) the map of the Songjiawuchang landslide profile; (c–e) combined maps of GPS, InSAR, and rainfall; (f–h) geological investigation site maps.
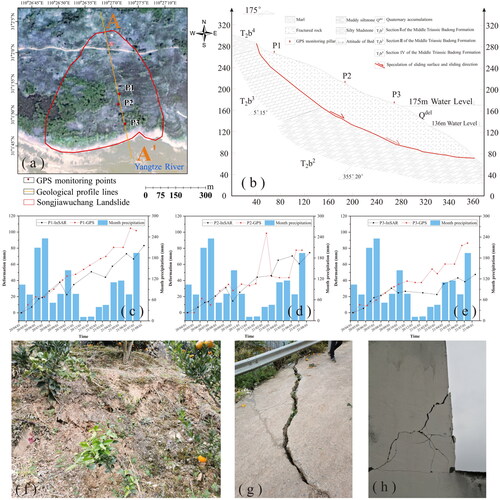
The precipitation in the region is mostly concentrated between May and October. The highest monthly rainfall occurs in July 2020, reaching 232 mm, while the lowest monthly precipitation is less than 10 mm in December 2020. We compared the data of InSAR with GPS and found that the subsidence in the region is consistent with the trend of precipitation. The slope of the deformation curves observed by InSAR and GPS increases after the surge of precipitation in May each year, when the flood season begins, indicating that the deformation trend accelerates with the increase of precipitation, reflecting that the surge of precipitation accelerates the deformation trend of the monitored sites in the region.
From the deformation curves, the GPS and InSAR results showed that the landslide had large deformation in April to September 2020 and April to August 2021, indicating that the surface deformation in the region was significantly affected by the increase or decrease of precipitation. We should strengthen the monitoring density for the slope during the rainy season. According to the cumulative deformation of the three points, P1 has a larger displacement than P2 and P3, indicating that the higher part of the slope body has a larger displacement. The higher part is more sensitive to rainfall. It is a pushed landslide. We need to strengthen the monitoring of the trailing edge of the landslide to ensure the safety of the place.
We conducted two field surveys of the Songjiawuchang landslide during the study period and found tensile deformation in the soil of orange trees (), a 2 cm-wide road crack (), and house cracks in villagers’ homes (). We photographed the tensile cracks under orange trees and the road cracks on December 12, 2020, and photographed house cracks in the villager’s house on April 20, 2021. According to the findings of the visit, earthquakes struck the site in 2019 and 2020. There are 1 cm-wide house cracks at No. 23 of Group 6 and No. 25 of Group 7 of Green Bamboo Raft Village. June 2020 to the present, the road is being built within this landslide, which has had an impact on the road surface in front of the houses, causing cracks in the road and making them unstable. As a result, it appears that, in addition to rainfall, earthquakes and human engineering activities play a role in the Songjiawuchang landslide.
5.2. The reliability and applicability conditions of the identification method
InSAR provides direct evidence of deformation for identifying landslides. However, InSAR results have redundant information on deformation. Considering that the deformation of landslides is aggregated and non-fragmented, we introduced two spatial analysis tools, hot spot analysis, and density analysis. The results of the spatial analysis showed that the method successfully filtered some impurity information compared with the original deformation rate map, which helped us to select 27 deformation aggregation areas in the study area. The work avoided some subjectivity and helped us to retain objectivity.
We need to analyze the probability of spatial occurrence and temporal triggering factors of landslides based on the deformation zones. Therefore, we introduced landslide susceptibility analysis and Pearson correlation coefficients based on rainfall and deformation. We used those to filter and screen out the hidden dangerous areas at spatial and temporal levels. Finally, 11 landslide hazards were found at the 11 different locations. Our investigation found that all these 11 landslide hazards had either large or small deformation. The study also carefully analyzed the Songjiawuchang landslide, which has large deformation. The comparison of the curves from the landslide development and InSAR and GPS monitoring data all proved the effectiveness of the identification method.
The spatial filter, landslide susceptibility is relatively easy to obtain, based on the corresponding method. And it is generally more closely integrated with geology and does not change in a short time. On the other hand, the temporal filter must be considered carefully along with the study area. Since the study area is mainly along the Yangtze River, where the rock layer is significantly affected by rainfall and reservoir water level, a time filter method based on rainfall is more effective. Corresponding adjustments can be made for different study areas based on the main triggering factors of the corresponding area, which can include human engineering activities, earthquakes, groundwater, etc.
6. Conclusions
Identifying landslides is essential for sustainable human development, and InSAR is a good method to monitor surface deformation, especially for large-scale studies. How to explore the relationship between surface deformation and landslide is in building a bridge between InSAR and landslide identification. Therefore, this paper proposed an identification process method that integrated five means of InSAR, spatial analysis, landslide susceptibility, rainfall correlation, and field survey for identifying potential rainfall landslides. We proposed a rainfall-based landslide identification method that included temporal and spatial filtering. We used the SBAS-InSAR technique to process fourteen PALSAR-2 images acquired between April 14, 2020, and August 7, 2021, to generate ground displacement rates and identify potential landslides in the Badong barrier area.
Using InSAR for landslides identification necessitates taking into account both risk factors and hazards-pregnant background conditions. In this study, the temporal and spatial filtering of the results of the deformation aggregation area of InSAR was actually a method that takes into account the pregnant conditions and predisposing factors. Landslide susceptibility is a study to evaluate the spatial probability of landslide occurrence, which incorporates geological conditions, geomorphological factors, etc., and can realize the assessment and filtering of hazards-pregnant background conditions in the study area. In addition to background conditions, predisposing factors need to be considered. There were more rainfall induced landslides developed in the Badong ecological barrier area, so the rainfall factor was paid attention to in our research. Correlation analysis of monthly rainfall and InSAR results can further target potential impact areas. The addition of background conditions and predisposing factors to the InSAR results greatly improves the success rate of landslides identification.
Data availability statement
The data that support the findings of this study are available from the Hubei Geological Environment Station but restrictions apply to the availability of these data, which were used under license for the current study, and so are not publicly available. Data are however available from the authors upon reasonable request and with permission of the Hubei Geological Environment Station.
Additional information
Funding
References
- Akbarimehr M, Motagh M, Haghshenas-Haghighi M. 2013. Slope stability assessment of the Sarcheshmeh landslide, Northeast Iran, investigated using InSAR and GPS observations. Remote Sens. 5(8):3681–3700.
- Bayer B, Simoni A, Mulas M, Corsini A, Schmidt D. 2018. Deformation responses of slow moving landslides to seasonal rainfall in the Northern Apennines, measured by InSAR. Geomorphology. 308:293–306.
- Berardino P, Fornaro G, Lanari R, Sansosti E. 2002. A new algorithm for surface deformation monitoring based on small baseline differential SAR interferograms. IEEE Trans Geosci Remote Sens. 40(11):2375–2383.
- Buytaert W, Celleri R, Willems P, Bièvre BD, Wyseure G. 2006. Spatial and temporal rainfall variability in mountainous areas: a case study from the south Ecuadorian Andes. J Hydrol. 329(3–4):413–421.
- Cantarino I, Carrion MA, Goerlich F, Martinez Ibañez V. 2019. A ROC analysis-based classification method for landslide susceptibility maps. Landslides. 16(2):265–282.
- Chen T, Zhu L, Niu R-q, Trinder CJ, Peng L, Lei T. 2020. Mapping landslide susceptibility at the Three Gorges Reservoir, China, using gradient boosting decision tree, random forest and information value models. J Mt Sci. 17(3):670–685.
- Chen W, Pourghasemi HR, Naghibi SA. 2018. A comparative study of landslide susceptibility maps produced using support vector machine with different kernel functions and entropy data mining models in China. Bull Eng Geol Environ. 77(2):647–664.
- Chen W, Wang J, Xie X, Hong H, Van Trung N, Bui DT, Wang G, Li X. 2016. Spatial prediction of landslide susceptibility using integrated frequency ratio with entropy and support vector machines by different kernel functions. Environ Earth Sci. 75(20):1344.
- Ciampalini A, Raspini F, Bianchini S, Frodella W, Bardi F, Lagomarsino D, Di Traglia F, Moretti S, Proietti C, Pagliara P, et al. 2015. Remote sensing as tool for development of landslide databases: the case of the Messina Province (Italy) geodatabase. Geomorphology. 249:103–118.
- Devara M, Tiwari A, Dwivedi R. 2021. Landslide susceptibility mapping using MT-InSAR and AHP enabled GIS-based multi-criteria decision analysis. Geomat Nat Hazards Risk. 12(1):675–693.
- Di B-f, Zhang K-s, Tang Y, Zhang M-h, Ustin SL. 2013. The development of a geographic information system (GIS) database for Jiuzhaigou national nature reserve and its application. J Mt Sci. 10(3):398–409.
- Dong J, Zhang L, Liao M, Gong J. 2019. Improved correction of seasonal tropospheric delay in InSAR observations for landslide deformation monitoring. Remote Sens Environ. 233:111370.
- Dong S, Samsonov S, Yin H, Ye S, Cao Y. 2014. Time-series analysis of subsidence associated with rapid urbanization in Shanghai, China measured with SBAS InSAR method. Environ Earth Sci. 72(3):677–691.
- Fourniadis IG, Liu JG, Mason PJ. 2007. Landslide hazard assessment in the Three Gorges Area, China, using ASTER imagery: Wushan–Badong. Geomorphology. 84(1–2):126–144.
- Fustos-Toribio I, Manque-Roa N, Vásquez Antipan D, Hermosilla Sotomayor M, Letelier Gonzalez V. 2022. Rainfall-induced landslide early warning system based on corrected mesoscale numerical models: an application for the southern Andes. Nat Hazards Earth Syst Sci. 22(6):2169–2183.
- Ge D, Dai K, Guo Z, Li Z. 2019. Early identification of serious geological hazards with integrated remote sensing technologies: thoughts and recommendations. Geomat Inf Sci Wuhan Univ. 44:949–956.
- Ghorbanzadeh O, Rostamzadeh H, Blaschke T, Gholaminia K, Aryal J. 2018. A new GIS-based data mining technique using an adaptive neuro-fuzzy inference system (ANFIS) and k-fold cross-validation approach for land subsidence susceptibility mapping. Nat Hazards. 94(2):497–517.
- Goyes-Peñafiel P, Hernandez-Rojas A. 2021. Landslide susceptibility index based on the integration of logistic regression and weights of evidence: a case study in Popayan, Colombia. Eng Geol. 280:105958.
- Guo R, Li S, Chen Y, Li X, Yuan L. 2021. Identification and monitoring landslides in longitudinal range-gorge region with InSAR fusion integrated visibility analysis. Landslides. 18(2):551–568.
- Hadi SJ, Tombul M. 2018. Comparison of spatial interpolation methods of precipitation and temperature using multiple integration periods. J Indian Soc Remote Sens. 46(7):1187–1199.
- Hussain S, Hongxing S, Ali M, Ali M. 2022. PS-InSAR based validated landslide susceptibility modelling: a case study of Ghizer valley, Northern Pakistan. Geocarto Int. 37(13):3941–3962.
- Intrieri E, Raspini F, Fumagalli A, Lu P, Del Conte S, Farina P, Allievi J, Ferretti A, Casagli N. 2018. The Maoxian landslide as seen from space: detecting precursors of failure with Sentinel-1 data. Landslides. 15(1):123–133.
- Jolivet R, Agram PS, Lin NY, Simons M, Doin M‐P, Peltzer G, Li Z. 2014. Improving InSAR geodesy using global atmospheric models. J Geophys Res Solid Earth. 119(3):2324–2341.
- Juang CH. 2021. BFTS – Engineering geologists’ field station to study reservoir landslides. Eng Geol. 284:106038.
- Liu Z, Qiu H, Zhu Y, Liu Y, Yang D, Ma S, Zhang J, Wang Y, Wang L, Tang B, et al. 2022. Efficient identification and monitoring of landslides by time-series InSAR combining single- and multi-look phases. Remote Sens. 14(4):1026.
- Lu P, Bai S, Tofani V, Casagli N. 2019. Landslides detection through optimized hot spot analysis on persistent scatterers and distributed scatterers. ISPRS J Photogramm Remote Sens. 156:147–159.
- Mallick J, Singh RK, AlAwadh MA, Islam S, Khan RA, Qureshi MN. 2018. GIS-based landslide susceptibility evaluation using fuzzy-AHP multi-criteria decision-making techniques in the Abha Watershed, Saudi Arabia. Environ Earth Sci. 77(7):276.
- Marjanović M, Kovačević M, Bajat B, Voženílek V. 2011. Landslide susceptibility assessment using SVM machine learning algorithm. Eng Geol. 123(3):225–234.
- Medhat NI, Yamamoto M‐y, Tolomei C, Harbi A, Maouche S. 2022. Multi‐temporal InSAR analysis to monitor landslides using the small baseline subset (SBAS) approach in the Mila Basin, Algeria. Terra Nova. 34(5):407–423.
- Milillo P, Sacco G, di Martire D, Hua H. 2022. Neural network pattern recognition experiments toward a fully automatic detection of anomalies in InSAR time series of surface deformation. Front Earth Sci. 9:728643.
- Morelli M, Piana F, Mallen L, Nicolò G, Fioraso G. 2011. Iso-kinematic maps from statistical analysis of PS-InSAR data of Piemonte, NW, Italy: comparison with geological kinematic trends. Remote Sens Environ. 115(5):1188–1201.
- Niu R, Wu X, Yao D, Peng L, Ai L, Peng J. 2014. Susceptibility Assessment of Landslides Triggered by the Lushan Earthquake, April 20, 2013, China. IEEE J Sel Top Appl Earth Observations Remote Sensing. 7(9):3979–3992.
- Novellino A, Cesarano M, Cappelletti P, Di Martire D, Di Napoli M, Ramondini M, Sowter A, Calcaterra D. 2021. Slow-moving landslide risk assessment combining machine learning and InSAR techniques. Catena. 203:105317.
- Novellino A, Cigna F, Sowter A, Ramondini M, Calcaterra D. 2017. Exploitation of the Intermittent SBAS (ISBAS) algorithm with COSMO-SkyMed data for landslide inventory mapping in north-western Sicily, Italy. Geomorphology. 280:153–166.
- Rong G, Alu S, Li K, Su Y, Zhang J, Zhang Y, Li T. 2020. Rainfall induced landslide susceptibility mapping based on Bayesian optimized random forest and gradient boosting decision tree models—a case study of Shuicheng County, China. Water. 12(11):3066.
- Rosi A, Tofani V, Tanteri L, Tacconi Stefanelli C, Agostini A, Catani F, Casagli N. 2018. The new landslide inventory of Tuscany (Italy) updated with PS-InSAR: geomorphological features and landslide distribution. Landslides. 15(1):5–19.
- Schlögl M, Gutjahr K, Fuchs S. 2022. The challenge to use multi-temporal InSAR for landslide early warning. Nat Hazards. 112(3):2913–2919.
- Singh LP, van Westen CJ, Ray PKC, Pasquali P. 2005. Accuracy assessment of InSAR derived input maps for landslide susceptibility analysis: a case study from the Swiss Alps. Landslides. 2(3):221–228.
- Singh N, Maddheshiya SK, Jha M, Tignath S, Singh BN. 2020. Hydrogeomorphic assessment of badlands in part of the Mandakini River watershed, Chitrakoot, India. Arab J Geosci. 13(20):1066.
- Song Y, Niu R, Xu S, Ye R, Peng L, Guo T, Li S, Chen T. 2018. Landslide susceptibility mapping based on weighted gradient boosting decision tree in Wanzhou section of the Three Gorges Reservoir Area (China). ISPRS Int J Geoinf. 8(1):4.
- Su X-j, Zhang Y, Meng X-m, Yue D-x, Ma J-h, Guo F-y, Zhou Z-q, Rehman MU, Khalid Z, Chen G, et al. 2021. Landslide mapping and analysis along the China-Pakistan Karakoram Highway based on SBAS-InSAR detection in 2017. J Mt Sci. 18(10):2540–2564.
- Sun D, Xu J, Wen H, Wang D. 2021. Assessment of landslide susceptibility mapping based on Bayesian hyperparameter optimization: a comparison between logistic regression and random forest. Eng Geol. 281:105972.
- Suzuki S, Kankaku Y, Osawa Y. 2011. Development status of PALSAR-2 onboard ALOS-2. In: Meynart R, Neeck SP, Shimoda H, editors. SPIE Remote Sensing. Bellingham, WA: International Society for Optics and Photonics. p. 81760Q.
- Tehrany MS, Pradhan B, Mansor S, Ahmad N. 2015. Flood susceptibility assessment using GIS-based support vector machine model with different kernel types. Catena. 125:91–101.
- Tien Bui D, Tuan TA, Klempe H, Pradhan B, Revhaug I. 2016. Spatial prediction models for shallow landslide hazards: a comparative assessment of the efficacy of support vector machines, artificial neural networks, kernel logistic regression, and logistic model tree. Landslides. 13(2):361–378.
- Tizzani P, Berardino P, Casu F, Euillades P, Manzo M, Ricciardi G, Zeni G, Lanari R. 2007. Surface deformation of Long Valley caldera and Mono Basin, California, investigated with the SBAS-InSAR approach. Remote Sens Environ. 108(3):277–289.
- van Natijne AL, Bogaard TA, van Leijen FJ, Hanssen RF, Lindenbergh RC. 2022. World-wide InSAR sensitivity index for landslide deformation tracking. Int J Appl Earth Obs Geoinf. 111:102829.
- Wang G, Lei X, Chen W, Shahabi H, Shirzadi A. 2020. Hybrid computational intelligence methods for landslide susceptibility mapping. Symmetry. 12(3):325.
- Wasowski J, Bovenga F. 2014. Investigating landslides and unstable slopes with satellite multi temporal interferometry: current issues and future perspectives. Eng Geol. 174:103–138.
- Xiong Z, Feng G, Feng Z, Miao L, Wang Y, Yang D, Luo S. 2020. Pre- and post-failure spatial-temporal deformation pattern of the Baige landslide retrieved from multiple radar and optical satellite images. Eng Geol. 279:105880. https://doi.org/10.1016/j.enggeo.2020.105880
- Yao X, Tham LG, Dai FC. 2008. Landslide susceptibility mapping based on support vector machine: a case study on natural slopes of Hong Kong, China. Geomorphology. 101(4):572–582.
- Yin Y, Zheng W, Liu Y, Zhang J, Li X. 2010. Integration of GPS with InSAR to monitoring of the Jiaju landslide in Sichuan, China. Landslides. 7(3):359–365.
- Youssef AM, Pourghasemi HR. 2021. Landslide susceptibility mapping using machine learning algorithms and comparison of their performance at Abha Basin, Asir Region, Saudi Arabia. Geosci Front. 12(2):639–655.
- Zhao L, Wu X, Niu R, Wang Y, Zhang K. 2020. Using the rotation and random forest models of ensemble learning to predict landslide susceptibility. Geomatics Nat Hazards Risk. 11(1):1542–1564.
- Zhao R, Li Z-w, Feng G-c, Wang Q-j, Hu J. 2016. Monitoring surface deformation over permafrost with an improved SBAS-InSAR algorithm: with emphasis on climatic factors modeling. Remote Sens Environ. 184:276–287.
- Zhu K, Xu P, Cao C, Zheng L, Liu Y, Dong X. 2021. Preliminary identification of geological hazards from Songpinggou to Feihong in Mao County along the Minjiang River using SBAS-InSAR technique integrated multiple spatial analysis methods. Sustainability. 13(3):1017.