ABSTRACT
Antibiotics profoundly affect the gut microbiome and modulate microbial communities. We recently observed that antimicrobial drugs also impact the abundance and distribution of antibiotic resistance genes. In this addendum, we reanalyze our ∼1 trillion nucleotide shotgun metagenomic dataset to quantify comprehensive genomic differences at the sequence level before and after antibiotic treatment. We show that 7 day exposure to cefprozil leads to a statistically significant loss of metagenome sequences. Recovery of gut microbiomes 3 months after antibiotherapy was characterized by the emergence of new genome sequences not observed prior to antibiotic exposure. Participants with low initial gut microbiome diversity had an increased amount of sequences related to antibiotic resistance. Therefore, we suggest that while the taxonomical composition of microbiomes is partially affected by the antibiotic, the genomic content and population structure of bacterial communities is noticeably impacted.
Introduction
Metagenomic studies demonstrated an uneven recovery of the human gut microbiome after treatment with antibiotics.Citation1-3 Researchers have even suggested that early exposure to antibiotics can impact future health.Citation4-6 In our recent study, we explored how a widely prescribed antibiotic, used to cure mild infections, can alter the human gut microbiome.Citation7 Our goal was to evaluate the impact of antibiotic treatment on the gut microbiome. To test this, we gave a 7 day course of cefprozil to 18 healthy volunteers and sequenced the microbial content of stool samples before, at the end of the 7 days course of antibiotic treatment and 90 days later. Six control volunteers, receiving no antibiotic, were also enrolled and analyzed at the same time points (day 0, day 7 and day 90). We then evaluated the impact of cefprozil on the gut microbiome of healthy individuals and derived 3 conclusions. By comparing the taxonomical content of stool samples through time and between individuals, we observed that a limited number of bacteria were consistently affected in all treated participants. Only three genera were significantly increased while a dozen other genera were decreased by antibiotic exposure (). In fact, all the taxa significantly and uniformly affected by the antibiotic had an average abundance below 10%. Therefore, our first conclusion was that the antibiotic had a reproducible effect mostly visible on the lower abundance taxa. Our second conclusion was that the initial composition of the gut microbiome was linked to the effect of the antibiotic on this microbiome. Indeed, exposed participants with lower initial microbiome diversity at day 0 had blooms of Enterobacter cloacae complex bacteria at day 7. Our third conclusion was that the antibiotic treatment modulated the gut microbiota in a fashion that led to the apparition of resistance genes that were not detected prior to treatment.
Figure 1. Modulation of bacterial genera by cefprozil. Average change in abundance ranking between day 7 and day 0 samples for exposed participant. Only the 13 genera for which relative abundance was statistically significant between day 7 and day 0 after antibiotics treatment are displayed (p < 0.05 after Benjamini & Yekutieli false discovery rate correction, see Raymond et al.Citation7 for details). Error bars represent confidence interval at 95%.
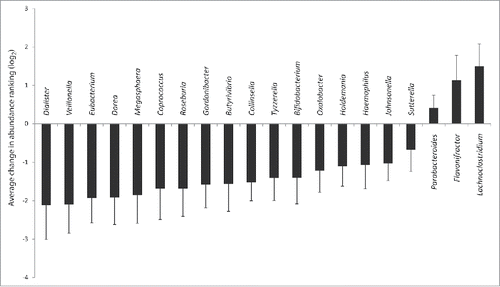
The initial analysis of our shotgun metagenomics data set (∼1 trillion nucleotides) was performed using taxonomic profiling based on k-mers using the Ray Meta software.Citation8 In our original publication, we used taxonomical profiling and gene annotation to provide critical information on the impact of antibiotics on the gut microbiome. The results were interpreted with a microbiology perspective, allowing the generation of new hypotheses for better management of antibiotics. This methodology had 2 main limitations. First, it reduced microbial communities to categories of microbes and did not differentiate bacteria of the same taxa that may harbor wholly different sets of genes.Citation9 Second, it is biased by the genome sequences available at the time of analysis and therefore will not consider genomes absent from the reference database.
In this paper, we focused on how microbiomes were affected as a whole and performed quantitative analysis of DNA sequence content before and after antibiotic treatment. Indeed, we generated a taxonomy-independent quantitative measure of the similarity between microbiomes to evaluate the impact of exposure to antibiotics. To do so, we compared the k-mer content of assembled metagenomes using our new software implementation, Ray Surveyor (https://github.com/zorino/ray).
The Ray Surveyor software was built to easily perform large comparative genomics analyses using k-mers as the comparison unit. Briefly, it compares the k-mer content of genome assemblies to measure the similarity between samples at the DNA sequence level. K-mers are substrings of DNA of length k. We use a k-mer length of 31 nucleotides because it is sufficiently specific to allow confidence in genomic comparisons.Citation8 Ray Surveyor identifies k-mers shared between 2 or more samples by calculating the presence/absence of k-mers in all the samples. The distinctive function of Ray Surveyor is the capability of comparing genome sequences either based on their complete k-mer content or on a specified subset of k-mers, such as an annotation dataset.
Comparison of microbiomes using k-mers
We therefore compared assembled metagenomes (Accession PRJEB8094 in the European Nucleotide Archive) using Ray Surveyor in order to precisely quantify the short-term and long-term impact of a 7 day course of cefprozil on the gut microbiome of each individual without a priori and biases introduced by taxonomical classification. Overall, the genomic content of antibiotic-exposed microbiome assemblies was more different from their initial state than the content of controls without antibiotics (). Indeed, we observed an average concordance (Jaccard similarity index) of 57.7% in average between day 7 and day 0 for control patients versus 38.4% similarity for patients that were exposed to the antibiotic cefprozil (p = 3.5e-7, Welch Two Sample t-test). These results indicate that the effect of the antibiotic is greater than the temporal metagenomic variability of controls during the course of a week. The measure of unique k-mers lost or gained after antibiotic treatment confirms a loss of genomic diversity after the use of antibiotics compared to controls (). We then quantitatively assessed how much microbiomes reverted back to their initial sequence composition after 3 months. The Jaccard similarity index between day 90 and day 0 was 56.0% for controls, which was similar to the difference between day 7 and day 0 (). For exposed participants, the similarity of 43.8% between day 90 and day 0 represented a significant decrease (p = 0.001, Welch Two Sample t-test) but indicated a partial reversion to the initial metagenome composition. In most samples, there is still a marked difference between the controls and the exposed participants at day 90, suggesting that gut microbiomes are more different from their original sequence composition when they were subjected to cefprozil. However, we observed that this difference in exposed participants is now driven by the emergence of sequences not observed at day 0 rather than by loss of k-mers at day 90 (). This new analysis sketches a complementary yet congruent portrait of our original analysis, which was based on taxonomical profiling. Indeed, in our initial study, we observed that most of the participants returned to their initial composition in terms of taxa. However, our new analysis shows that gut microbiomes do not necessarily recover all the lost metagenomic sequences. A possible explanation would be that there is a shift in dominant strains or isolates belonging to particular taxa after antibiotic treatment. This metagenomic drift could alter the functional content of microbiomes and affect clinically relevant genetic determinants such as resistance genes.Citation10,11 In line with the observations of Morowitz and collaborators,Citation12 our analysis confirms that limiting microbiome studies to taxonomical profiling overlooks the genetic diversity of microbiota.
Figure 2. Comparison of complete assembled metagenomes between A) day 7 vs day 0 and B) day 90 vs day 0 for exposed participants and controls. Horizontal lines indicate the mean of groups. Participants enriched in E. cloacae at day 7 are marked with stars (*).
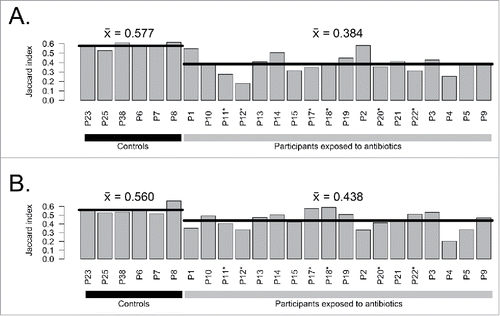
Figure 3. Comparison of total k-mers content between A) day 7 and day 0 and B) day 90 and day 0. Counts are normalized by the number of reads sequenced per sample. Participants enriched in E. cloacae at day 7 are marked with stars (*).
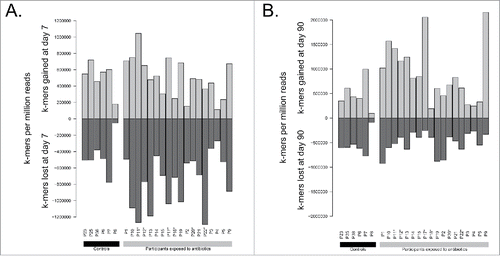
In our previous work, we created a database of resistance genes (MERGEM database) that can be used for k-mer filtering. Citation13 In this new analysis, we compared metagenomes using MERGEM as a filter for resistance gene k-mers, thereby measuring similarity between gut microbiomes based on sequences potentially associated with antibiotic resistance. The Jaccard similarity index calculated between day 7 and day 0 for resistance gene k-mers in participants with antibiotics (39.0%) was significantly lower than controls (64.9%) (p = 0.023, Welch Two Sample t-test) (). Difference between exposed (50.1%) and controls (63.4%) for the comparison between day 90 and day 0 is not statistically significant (p = 0.075, Welch Two Sample t-test) (). At day 7, the number of k-mers previously observed in resistance genes is increased in 8 out of 18 samples (). Notably, participants that had blooms of Enterobacter cloacae complex bacteria had a strong increase in resistance gene-related k-mers compared to controls. The opportunistic pathogen E. cloacae is known as a reservoir of antibiotic resistance genes.Citation14,15 Our results suggest that a pervasive effect of antibiotics on the gut microbiota could create ecological niches fostering the emergence of multi-resistant opportunistic pathogens, especially in individuals presenting a reduced microbial diversity.
Figure 4. Comparison of resistance gene related k-mers between A) day 7 vs day 0 and B) day 90 vs day 0 for exposed participants and controls. Horizontal lines indicate the mean of groups. Participants enriched in E. cloacae at day 7 are marked with stars (*).
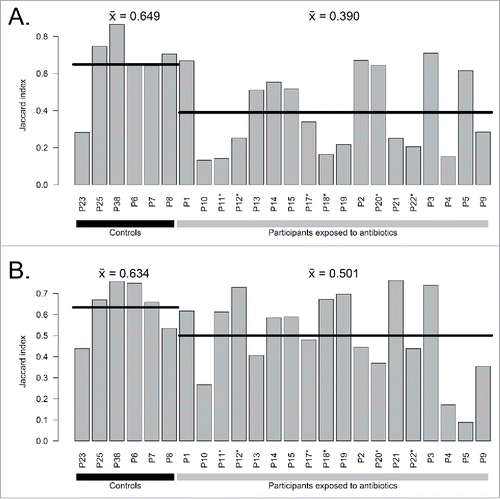
Figure 5. Comparison of k-mers related to antibiotic resistance between A) day 7 and day 0 and B) day 90 and day 0. Counts are normalized by the number of reads sequenced per sample. Participants enriched in E. cloacae at day 7 are marked with stars (*).
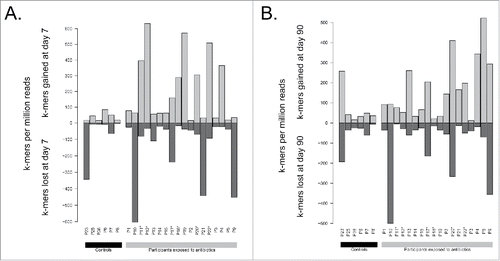
As a control experiment, we investigated how the number of reads used to assemble metagenomes affected the Jaccard similarity index for 4 representative participants: 2 controls (C6 and C38) and 2 exposed to antibiotics (E18 and E21). We compared the assemblies generated by subsampling reads from the day 0 samples (with increments of 8 million reads) to the assemblies obtained using all the reads of the day 7 samples. For the 4 samples, we observed that the Jaccard similarity index reached a plateau between 80 and 100 million reads (). We therefore suggest that a depth of 100 million reads should provide in most cases a consistent approximation of similarity and could limit bias caused by sequencing depth when comparing gut microbiome assemblies using k-mers. Comparing assemblies at a lower sequencing depth using k-mers would generate inconsistent results. Differences in curve shapes between controls and exposed participants () might indicate that accurately comparing samples that show little perturbation require more reads than comparing highly affected participants. When looking at how sequencing depth influenced assemblies, we observed that assembly size still continued to grow when more reads were added (). In contrast, sequencing depth of more than 24 million reads did not seem to affect taxonomical profiling ().
Figure 6. Influence of sequencing depth on comparison, assembly and profiling of metagenomes for 4 participants (controls 6 and 38, and exposed to antibiotic 18 and 21). A) Comparison of k-mer content of day 0 assemblies with day 7 assemblies using Ray Surveyor. Day 7 assemblies were performed using all the reads sequenced while day 0 assemblies were performed by subsampling reads using 8 million reads increments. Similarity was calculated using the Jaccard similarity index. B) Influence of the number of reads used for assembly on the number of nucleotides assembled into contigs for day 0 samples. Subsampling of reads was in 8 million reads increments. C) Taxonomical profiling at the family rank using Ray Meta 2.3.1 on subsampled reads. Subsampling increments of 8 million nucleotides are indicated by dashes under the plot.
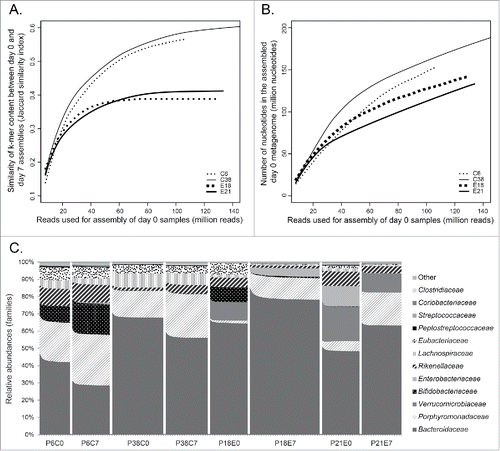
Conclusion
Here, we show that directly comparing the genomic content of microbiomes allows to quantify the extent of microbiome perturbations after antibiotic treatment. This k-mer comparison method takes into account the pan-genome of species and the variability that exists within a taxonomic unit, for example different strains of a species. We can now quantitatively explore how specific strains or genomic elements, such as resistance genes, are modulated by treatment. Since comparisons using k-mers and the Jaccard similarity index are strongly affected by increases in genomic distance,Citation16 this approach is well suited to analyze time-course experiments or before-and-after states in an individual. Comparison of k-mer content allowed to measure gain or loss of genetic material at the end of the antibiotic treatment and after 3 months of recovery, which could not be observed by taxonomical profiling. However, it remains difficult to answer the critical question of whether a microorganism has disappeared from a population after antibiotic treatment.Citation17 Indeed, detection of specific taxa is dependent upon sequencing depth, bacterial abundance and microbial diversity.Citation18
Comparing k-mers to analyze changes in microbiomes following antibiotic stress brings forth the question of how to consider diversity in shotgun microbiome analysis. In most studies, we consider microbial diversity as an index describing population structures that take into account the number of entities measured at a specific taxonomic rank and the evenness of their relative proportions. To extend this concept, researchers are now considering the single nucleotide polymorphisms found in bacterial populations to better understand the effect of environmental conditions or drugs on the strain diversity within metagenomes.Citation19,20 Unravelling the full richness of microbial genetic diversity in microbiomes will allow a thorough understanding of changes induced by any compounds, drugs or infections.Citation21
Disclosure of potential conflicts of interest
No potential conflicts of interest were disclosed.
Acknowledgment
Computations were performed under the auspices of Calcul Québec and Compute Canada. The operations of Compute Canada are funded by the Canada Foundation for Innovation (CFI), the National Science and Engineering Research Council (NSERC), NanoQuébec and the Fonds Québécois de Recherche sur la Nature et les Technologies (FQRNT).
Funding
This study was financed by the Québec Consortium for Drug Discovery (CQDM) and by the Canada Research Chair Program (JC). FR was supported by a Mitacs post-doctoral fellowship.
References
- Dethlefsen L, Relman DA. Incomplete recovery and individualized responses of the human distal gut microbiota to repeated antibiotic perturbation. Proc Natl Acad Sci U S A 2011; 108 Suppl:4554-61; PMID:20847294; http://dx.doi.org/10.1073/pnas.1000087107
- Dethlefsen L, Huse S, Sogin ML, Relman DA. The pervasive effects of an antibiotic on the human gut microbiota, as revealed by deep 16S rRNA sequencing. PLoS Biol 2008; 6:e280; PMID:19018661; http://dx.doi.org/10.1371/journal.pbio.0060280
- Rashid M-U, Rosenborg S, Panagiotidis G, Löfdal KS, Weintraub A, Nord CE. Ecological effect of ceftazidime/avibactam on the normal human intestinal microbiota. Int J Antimicrob Agents 2015; 46:60-5; PMID:25979639; http://dx.doi.org/10.1016/j.ijantimicag.2015.02.027
- Cox LM, Blaser MJ. Antibiotics in early life and obesity. Nat Rev Endocrinol 2015; 11:182-90; PMID:25488483; http://dx.doi.org/10.1038/nrendo.2014.210
- Zanvit P, Konkel JE, Jiao X, Kasagi S, Zhang D, Wu R, Chia C, Ajami NJ, Smith DP, Petrosino JF, et al. Antibiotics in neonatal life increase murine susceptibility to experimental psoriasis. Nat Commun 2015; 6:8424; PMID:26416167; http://dx.doi.org/10.1038/ncomms9424
- Korpela K, Salonen A, Virta LJ, Kekkonen RA, Forslund K, Bork P, de Vos WM. Intestinal microbiome is related to lifetime antibiotic use in Finnish pre-school children. Nat Commun 2016; 7:10410; PMID:26811868; http://dx.doi.org/10.1038/ncomms10410
- Raymond F, Ouameur AA, Déraspe M, Iqbal N, Gingras H, Dridi B, Leprohon P, Plante P-L, Giroux R, Bérubé È, et al. The initial state of the human gut microbiome determines its reshaping by antibiotics. ISME J 2016; 10:707-20; PMID:26359913; http://dx.doi.org/10.1038/ismej.2015.148
- Boisvert S, Raymond F, Godzaridis E, Laviolette F, Corbeil J. Ray Meta: scalable de novo metagenome assembly and profiling. Genome Biol 2012; 13:R122; PMID:23259615; http://dx.doi.org/10.1186/gb-2012-13-12-r122
- Zhu A, Sunagawa S, Mende DR, Bork P. Inter-individual differences in the gene content of human gut bacterial species. Genome Biol 2015; 16:82; PMID:25896518; http://dx.doi.org/10.1186/s13059-015-0646-9
- Gibson MK, Forsberg KJ, Dantas G. Improved annotation of antibiotic resistance determinants reveals microbial resistomes cluster by ecology. ISME J 2015; 9:207-16; PMID:25003965; http://dx.doi.org/10.1038/ismej.2014.106
- Olivares J, Bernardini A, Garcia-Leon G, Corona F, B Sanchez M, Martinez JL. The intrinsic resistome of bacterial pathogens. Front Microbiol 2013; 4:103; PMID:23641241; http://dx.doi.org/10.3389/fmicb.2013.00103
- Morowitz MJ, Denef VJ, Costello EK, Thomas BC, Poroyko V, Relman DA, Banfield JF. Strain-resolved community genomic analysis of gut microbial colonization in a premature infant. Proc Natl Acad Sci U S A 2011; 108:1128-33; PMID:21191099; http://dx.doi.org/10.1073/pnas.1010992108
- Raymond F, Ouameur AA, Déraspe M, Iqbal N, Gingras H, Dridi B, Leprohon P, Plante P-L, Giroux R, Bérubé È, et al. The initial state of the human gut microbiome determines its reshaping by antibiotics. ISME J 2016; 10:707-20; PMID:26359913; http://dx.doi.org/10.1038/ismej.2015.148
- Du H, Chen L, Chavda KD, Pandey R, Zhang H, Xie X, Tang Y-W, Kreiswirth BN. Genomic characterization of Enterobacter cloacae isolates from China that co-produce KPC-3 and NDM-1 carbapenemases. Antimicrob Agents Chemother 2016; 60:2519-23.
- Izdebski R, Baraniak A, Herda M, Fiett J, Bonten MJM, Carmeli Y, Goossens H, Hryniewicz W, Brun-Buisson C, Gniadkowski M. MLST reveals potentially high-risk international clones of Enterobacter cloacae. J Antimicrob Chemother 2014; 70:48-56; PMID:25216820
- Ondov BD, Treangen TJ, Melsted P, Mallonee AB, Bergman NH, Koren S, Phillippy AM. Mash: fast genome and metagenome distance estimation using MinHash. Genome Biol 2016; 17:132; PMID:27323842; http://dx.doi.org/10.1186/s13059-016-0997-x
- Blaser M. Antibiotic overuse: Stop the killing of beneficial bacteria. Nature 2011; 476:393-4; PMID:21866137; http://dx.doi.org/10.1038/476393a
- Rodriguez-R LM, Konstantinidis KT. Estimating coverage in metagenomic data sets and why it matters. ISME J 2014; 1-3
- Bendall ML, Stevens SL, Chan L-K, Malfatti S, Schwientek P, Tremblay J, Schackwitz W, Martin J, Pati A, Bushnell B, et al. Genome-wide selective sweeps and gene-specific sweeps in natural bacterial populations. ISME J 2016; 10:1589-601; PMID:26744812; http://dx.doi.org/10.1038/ismej.2015.241
- Yassour M, Vatanen T, Siljander H, Hämäläinen A-M, Härkönen T, Ryhänen SJ, Franzosa EA, Vlamakis H, Huttenhower C, Gevers D, et al. Natural history of the infant gut microbiome and impact of antibiotic treatment on bacterial strain diversity and stability. Sci Transl Med 2016; 8:343ra81; http://dx.doi.org/10.1126/scitranslmed.aad0917
- Brito IL, Alm EJ. Tracking Strains in the Microbiome: Insights from Metagenomics and Models. Front Microbiol 2016; 7:712; PMID:27242733