ABSTRACT
Increasing evidence suggests a role of the gut microbiota in patients’ response to medicinal drugs. In our recent study, we combined genomics of human gut commensals and gnotobiotic animal experiments to quantify microbiota and host contributions to drug metabolism. Informed by experimental data, we built a physiology-based pharmacokinetic model of drug metabolism that includes intestinal compartments with microbiome drug-metabolizing activity. This model successfully predicted serum levels of metabolites of three different drugs, quantified microbial contribution to systemic drug metabolite exposure, and simulated the effect of different parameters on host and microbiota drug metabolism. In this addendum, we expand these simulations to assess the effect of microbiota on the systemic drug and metabolite levels under conditions of altered host physiology, microbiota drug-metabolizing activity or physico-chemical properties of drugs. This work illustrates how and under which circumstances the gut microbiome may influence drug pharmacokinetics, and discusses broader implications of expanded pharmacokinetic models.
Introduction
Drug response can vary widely between patients, and these differences have been mainly explained by human genetic and physiological factors, such as age, gender, gut motility, blood flow, enzymatic reactions and organ volumes.Citation1 However, several studies have revealed specific examples of microbiome drug metabolism,Citation2–Citation9 suggesting that the effect of the gut bacteria on in vivo drug metabolism could be more widespread than anticipated.
In our recent study, we developed a gnotobiotic mouse model to separate host and microbiota contributions to drug metabolism.Citation10 We used tissue-specific drug and drug metabolite kinetics from animals that either did or did not harbor drug-metabolizing gut bacteria to build a physiology-based pharmacokinetic (PBPK) model.Citation1,Citation11,Citation12 This model incorporates an intestinal compartment of microbiome drug metabolism that enables quantification of the microbial contribution to systemic drug metabolite exposure. Even if drug-metabolizing bacteria are unknown and thus cannot be experimentally removed from a gnotobiotic mouse gut community, we demonstrated that this model can accurately predict systemic drug metabolite exposure and quantify the microbiome contribution using data from germ-free and conventional mice.
In this addendum, we apply this model to simulate different scenarios, such as altered drug absorption in the small intestine or enterohepatic circulation, to highlight factors with the greatest potential to influence microbiota contribution to in vivo drug metabolism.
An overview of the host-microbiome model of drug metabolism
Drug-metabolizing activity by host and microbiome can result in the same drug metabolites, which makes it challenging to determine their origin solely from serum measurements.Citation6,Citation13–Citation15 To separate host and microbiome contribution to drug metabolism, we built a PBPK model that includes a microbiome component. The model consists of eight compartments: three sections of the small intestine (duodenum, jejunum, and ileum), three sections of the large intestine (cecum, proximal and distal colon), gallbladder, and a central compartment, representing processes occurring in serum, liver and other tissues (). Following oral administration, a drug is either absorbed from the proximal small intestine into the central compartment, or it propagates through the intestinal tract. These processes are described by linear rates (first order and unsaturated) for i) initial drug absorption from proximal small intestine kaP (superscript “P” designates parent drug); ii) drug absorption from distal small intestine and large intestine (kaSIP and kaLIP); iii) drug propagation between intestinal tissues (kp1 to kp4, subscript “p” designates propagation); iv) drug elimination from circulation (keP). Host drug biotransformation occurs in the central compartment with a coefficient kcH, and metabolite elimination is described by keM (superscript “M” designates drug metabolite). Bacterial drug biotransformation occurs in the cecum with the conversion coefficient kcB, and the produced metabolite is either absorbed into the central compartment with a coefficient kaLIM, or it propagates through the large intestine with the coefficients kp3 and kp4. Additionally, the drug can undergo biliary secretion from the central compartment to gallbladder and back to the intestine with a coefficient kEH (enabling enterohepatic drug circulation, if the drug is re-absorbed from the intestine). Initial parameter values were set based on the results of our previous study, in which we fit the model to drug and drug metabolite measurements performed on samples from germ-free and conventional mice following oral brivudine administrationCitation10 ().
Table 1. Initial values and sampled distribution for the model parameters.
Microbiome drug metabolism and large-intestinal absorption
In our previous study, we used the model to predict systemic exposure of drugs and drug metabolites in conventional mice. These predictions were based on the parameters of host drug metabolism fitted to drug and drug metabolite tissue kinetics from germ-free mice, and microbiome drug metabolism fitted to the same measurements from the large intestine of conventional mice. Based on these fitted parameters, the model predicted drug and metabolite kinetics in the serum of conventional animals that were verified by direct experimental measurement. Whereas serum drug metabolite levels were substantially affected by microbiota drug metabolism, serum drug levels remained indistinguishable between germ-free and conventional mice for the three tested drugs brivudine, sorivudine, and clonazepam. However, we observed substantial reduction of the drug and concomitant accumulation of the metabolite in the large intestine of conventional mice compared to germ-free mice. We therefore hypothesized that different microbiota-contributed effects on drug and drug metabolite levels in serum could be explained by different absorption properties of the drug and drug metabolite in the large intestine.
To follow this hypothesis and identify conditions under which bacterial drug metabolism in the large intestine could affect drug serum kinetics, we performed 10,000 Monte Carlo simulations of the model with altered parameter sets. Parameters were varied across rates between 10−4 h-1 and 104 h−1, except propagation parameters kp1 to kp4 were altered within the range of estimated values (). For each parameter, we performed local sensitivity analysis to assess the effect of its variation on systemic drug exposure, whereas all other parameters were set to initial values. Both systemic and intestinal levels of the parent drug were most strongly impacted by initial drug absorption, elimination, and biliary secretion coefficients (). The microbial drug conversion coefficient substantially affected cecal, but not serum drug levels (). To identify factors preventing microbiome contribution to systemic drug exposure under initial model conditions, we simulated the model for each of the 10,000 parameter sets with two extreme values for the bacterial drug conversion, kcB = 10−4 h-1 and kcB = 104 h−1, and calculated the difference between systemic drug exposure resulting from these two conditions. The largest effect of microbial metabolism on the systemic drug exposure was observed with the increase of the drug absorption coefficient kaLI. As expected, these simulations demonstrate that microbiome drug biotransformation in the large intestine affects systemic drug levels, if the drug is substantially absorbed from the large intestine.
We next repeated this type of analysis to identify parameters that impact systemic metabolite exposure under the initial model conditions. We found that bacterial drug conversion influences both intestinal and systemic drug metabolite levels (). However, the main factors determining whether bacterial metabolic activity will affect systemic drug metabolite levels were systemic metabolite elimination and biliary secretion rates of the drug (). In fact, if the metabolite elimination coefficient is low, both host- and microbiota-derived drug metabolites remain in circulation, leading to prolonged exposure and larger differences between conditions of no-microbial- and high-microbial-contribution. High enterohepatic cycling of drugs also increases the difference in systemic drug metabolite exposure between the two conditions due to increased drug exposure of the intestinal bacteria, which will be discussed in more detail below.
Microbiome contribution to metabolism of highly absorbed drugs
Oral drugs are typically optimized for absorption from the small intestine, enabling high oral bioavailability. This implies that only small amounts of drug could reach the large intestine and be potentially metabolized by bacteria. However, previous studies identified microbial drug metabolism even after intravenous administration.Citation16 Furthermore, we observed high microbial contribution to metabolism of drugs with high absorption (quantified as oral bioavailability of up to 81%).Citation10 Based on these observations, we applied our modeling framework to analyze factors that allow bacterial contribution to systemic drug metabolism under conditions of high drug absorption from the small intestine.
We simulated different scenarios of drug absorption by varying the ratio between initial absorption kaP and propagation kp1, assuming that the non-absorbed drug propagates to the large intestine. For each set of the 10,000 random parameters simulated earlier, we set the ratio between kaP and kp1 to either 10 or 0.1 (which corresponds to 93% or 21% drug absorption into the central compartment), and compared microbiota contribution to systemic metabolite exposure between the two absorption conditions. Although bacterial contribution generally decreased with increased drug absorption from the small intestine, there were many parameter sets within the high drug absorption condition that resulted in substantial microbiome contribution to systemic drug metabolite exposure (). We performed local sensitivity analysis to identify parameters that determine bacterial contribution under conditions of high drug absorption. The three parameters allowing microbiota contribution of up to 100% to the systemic drug metabolite exposure were drug elimination keP, biliary secretion kEH, and host drug to metabolite conversion kcH (). Indeed, if a well-absorbed drug is rapidly removed from circulation either by elimination (urinary or biliary secretion), or if host drug biotransformation is neglectable, drug metabolites produced by the gut microbiota can substantially contribute to systemic exposure, given considerable metabolite absorption from the large intestine. Even in the absence of biliary drug elimination (kEH = 0), higher drug biotransformation by microbiota compared to the host can lead to substantial microbiome contribution to systemic drug metabolite exposure at nearly any level of drug absorption (). Additionally, varying the large intestine propagation parameter kp3 allows a microbiome contribution to systemic metabolite exposure of up to 50%, even if 93% of the oral drug dose is absorbed from small intestine into the central compartment (). An increase in the kp3 parameter simulates constipation, which is a common drug side effect (reported for 49% (703 out of 1430) drugs from the SIDER databaseCitation17).
Enterohepatic circulation and microbiome drug metabolism
Biliary secretion is a prevalent mechanism to eliminate drugs from the body. This elimination route typically follows conjugative (Phase II) drug metabolism; for example, glucuronidation of the parent drug or drug metabolites is commonly observed. Bile is secreted into the duodenum, where re-absorption of conjugated compounds is hampered due to their high hydrophilicity (). The gut microbiome encodes hundreds of glucuronidase enzymes covering a broad substrate spectrum, so that deconjugation likely occurs upon intestinal propagation.Citation18 Enterohepatic circulation is observed, if re-absorption of de-glucuronidated compounds is possible.Citation19 Our recent study and other studies provide examples of hepatic drug glucuronidation, their biliary secretion followed by de-glucuronidation by microbes in the large intestine.Citation10,Citation16 We therefore expanded our model to include host glucuronyl-transferase activity (kglP) and bacterial de-glucuronidation in the cecum (kdglB), and tested under which conditions enterohepatic circulation affects systemic drug and drug metabolite exposure.
Figure 4. Interplay between enterohepatic circulation and microbiome contribution to drug metabolism.
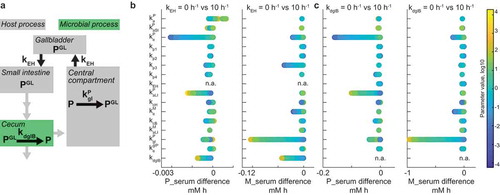
To this end, we simulated the model for each of the 10,000 random parameter sets with two (low and high) values of either biliary secretion coefficient kEH, or bacterial glucuronidase activity kdglB, and calculated the systemic exposure differences of both drug and drug metabolites between conditions. Enterohepatic circulation most strongly affects drug exposure under conditions that lead to an intact drug in the intestine that can be reabsorbed into circulation (). This is achieved if the drug is readily absorbed from the large intestine, if bacterial glucuronidase activity is high, or if bacterial drug metabolism activity is low. Under the same conditions, systemic metabolite exposure is determined by microbial glucuronidase and host glucuronyl-transferase activity. The propagation coefficient in the large intestine (kp3) also affected systemic metabolite exposure, underlining the importance of intestinal motility in determining host and microbiome contributions to metabolism of enterohepatically cycled drugs. Bacterial glucuronidase activity affected systemic drug levels under the same conditions as enterohepatic circulation, as it directly depends on the latter ().
Discussion
In this work, we use a coarse-grained pharmacokinetic model that explicitly models gut microbiota activity in the large intestine to identify conditions that promote microbiota contribution to in vivo drug metabolism. We demonstrate the potential for significant microbiota contributions to systemic drug or drug metabolite exposure under conditions that may not be intuitive. These scenarios include high drug bioavailability or the absence of observed differences in drug serum levels between germ-free and conventional animals. For drugs with a high reported bioavailability, the gut microbiota can produce the majority of a drug metabolite in case of extensive enterohepatic drug circulation, or large differences in microbial and host drug metabolism coefficients, as we observed experimentally for clonazepam and sorivudine, respectively.Citation10
Although the initial model parameter values were employed from the study of brivudine, the reported analysis is in principle generally applicable. Our model can also be further extended to incorporate processes relevant for particular drugs and physiological conditions. For example, the model could be expanded to include drug metabolism in the intestinal epithelium,Citation20,Citation21 or microbiota drug metabolism in the small intestine.Citation5 The effect of meal intake on gallbladder flowCitation22 or on intestinal transporter activityCitation23 could be represented as time-dependent coefficients of enterohepatic circulation and intestinal absorption, respectively. Pharmacokinetic models can be combined with host genome-scale models to simulate drug and nutrient effects on host physiology, which can lead to dietary recommendations for enhanced drug effect,Citation24 predicted drug–drug interactionsCitation25 or adverse drug reactions.Citation26–Citation28 Since intestinal motility, nutrient intake,Citation29,Citation30 and drugs affect bacterial growth,Citation31 physiology and microbiome composition,Citation32,Citation33 incorporation of genome-scale metabolic models of the microbiota into the pharmacokinetic modeling framework might further enhance the ability of these integrative models to predict in vivo metabolism of specific drugs. Improved in silico estimation of drug absorption,Citation34,Citation35 and putative hostCitation36–Citation39 and bacterialCitation40–Citation42 xenobiotic biotransformation in combination with systematic experimental studies of microbial drug metabolism,Citation6 will facilitate accurate prediction of in vivo drug metabolism with integrative pharmacokinetic models in the near future.Citation43,Citation44
Understanding the role of microbiota in drug metabolism may be instrumental for predicting and controlling adverse effects, because microbiota and host metabolism can be modulated with different interventions.Citation4 Although an extensive potential of gut microbes to metabolize drugs was demonstrated in vitro,Citation6 it is challenging to determine whether microbiota contributes to in vivo drug metabolism from patient’s serum, urine or feces data alone. Observations such as delayed pharmacokinetics between drug and drug metabolite in serum, drug metabolites whose levels or structures are inconsistent with the activities of host cells in vitro or preclinical animal models, or large differences between patients unexplained by human genetic variability, may be indicative of a necessity to evaluate the microbiome contribution to in vivo drug metabolism. By identifying the key processes affecting host drug metabolism, and taking into account physiological parameters of both host and microbiota, such integrative models can help to incorporate this knowledge at early stages of drug development, and enable design of rational therapies targeting gut microbes and their enzymes for minimized risks and maximized benefits of drug treatments in the future.
Materials and methods
Model overview – The multi-compartment pharmacokinetic model of drug metabolism in the mouse contained 8 compartments (small intestine sections jejunum, duodenum and ileum, large intestine sections cecum, colon, and distal colon, gallbladder, and central compartment). The central compartment incorporated processes occurring in the serum, liver, kidneys and all other body parts apart from the gastrointestinal tract (GI) lumen and gallbladder. An additional compartment was used as a reservoir for the initial drug dose. Drug propagation through the body was modeled by the flow of GI material in different GI tract sections and GI to central compartment diffusion coefficients. Enterohepatic circulation was modeled by enterohepatic cycling coefficient kEH, which determines the rate of compound diffusion from central compartment to gallbladder and from gallbladder to duodenum. All equations were defined for drug and metabolite amounts in a given compartment/tissue. For drug metabolite levels in serum, the metabolite levels contributed by the host (due to host drug metabolism, MH) were distinguished from the metabolite levels contributed by the microbiota (due to microbial metabolism in the cecum and metabolite absorption, MBAC). The model was created using the MatLab 2017b SimBiology Toolbox (MathWorks).
Initial parameter values – Initial parameter values were chosen based on the results of model fitting to germ-free and conventional mouse data administered brivudine, and are provided in .
Serum metabolite exposure prediction – To distinguish host and bacterial contributions to the systemic exposure of a drug metabolite, drug metabolites in the central compartment derived from either host or microbiome were modeled as separate metabolites (MH and MBAC). Microbial contribution to the systemic metabolite exposure was calculated as the ratio between areas under the curve of the microbial MBAC levels and total metabolite levels (the sum of microbial and host contributions, MH+MBAC) in the central compartment.
Sensitivity analysis – Local sensitivity analysis was performed to assess the effects of each parameter change on the total drug and drug metabolite exposure in the central compartment and cecum. Parameters that correspond to GI tract propagation (kp1-kp4) were sampled from a normal distribution with mean and standard deviation = mean/2 of the initial values from . All other parameters were sampled from a lognormal distribution in the base 10, which covered a range of values between 10−4 and 104. The model behavior was simulated for 10,000 sets of parameter values with the sbiosimulate function in MatLab.
Model simulation – To investigate microbiota contribution to the systemic drug metabolite exposure under different scenarios of drug availability in the GI tract, the model behavior was simulated for different drug initial absorption to propagation ratios and bacterial to host drug conversion ratios. To determine the relationship between initial absorption to propagation conversion ratios and percent of drug dose absorbed into the central compartment, the behavior of a simplified model that assumed that the drug remains either in the central compartment or in the small intestine was simulated. The ratio between the maximum amount of drug in the two compartments was calculated for each tested initial absorption to propagation ratios. The sbiosimulate function was used to determine the model behavior across all combinations of the parameter values in the range [0.01 1–10 100] for absorption to propagation ratio and for bacterial to host drug conversion ratios. All other parameters were set to the initial values from . For each model run, the area under the curve of the drug metabolite in the central compartment was calculated. The bacterial contribution was calculated as the ratio between microbial drug metabolite absorbed from cecum to the central compartment, and total drug metabolite in the central compartment.
Drug side effects analysis – To estimate the occurrence of constipation as a side effect of commonly used therapeutic drugs, side effect information was downloaded from the SIDER databaseCitation17 (file meddra_all_se.tsv.gz). The side effect column was filtered with a search term “constipation”, and the number of unique filtered compounds was divided by the total number of compounds in the database to calculate the frequency of the side effect.
The model and the code are available at https://github.com/mszimmermann/PBPK_host-microbiome_model.
Additional information
Funding
References
- Willmann S, Höhn K, Edginton A, Sevestre M, Solodenko J, Weiss W, Lippert J, Schmitt W. Development of a physiology-based whole-body population model for assessing the influence of individual variability on the pharmacokinetics of drugs. J Pharmacokinet Pharmacodyn. 2007;34:401–431. doi:10.1007/s10928-007-9053-5.
- Haiser HJ, Gootenberg DB, Chatman K, Sirasani G, Balskus EP, Turnbaugh PJ. Predicting and manipulating cardiac drug inactivation by the human gut bacterium Eggerthella lenta. Science. 2013;341:295–298. doi:10.1126/science.1235872.
- Klatt NR, Cheu R, Birse K, Zevin AS, Perner M, Noël-Romas L, Grobler A, Westmacott G, Xie IY, Butler J, et al. Vaginal bacteria modify HIV tenofovir microbicide efficacy in African women. Science. 2017;356:938–945. doi:10.1126/science.aai9383.
- Maini Rekdal V, Bess EN, Bisanz JE, Turnbaugh PJ, Balskus EP. Discovery and inhibition of an interspecies gut bacterial pathway for Levodopa metabolism. Science. 2019;364:eaau6323. doi:10.1126/science.aav6390.
- van Kessel SP, Frye AK, El-Gendy AO, Castejon M, Keshavarzian A, van Dijk G, El Aidy S. Gut bacterial tyrosine decarboxylases restrict levels of levodopa in the treatment of Parkinson’s disease. Nat Commun. 2019;10:310. doi:10.1038/s41467-019-08294-y.
- Zimmermann M, Zimmermann-Kogadeeva M, Wegmann R, Goodman AL. Mapping human microbiome drug metabolism by gut bacteria and their genes. Nature. 2019;570:462–467. doi:10.1038/s41586-019-1310-4.
- Elmer GW, Remmel RP. Role of the intestinal microflora in clonazepam metabolism in the rat. Xenobiotica. 1984;14:829–840. doi:10.3109/00498258409151405.
- Lindenbaum J, Rund DG, Butler VP, Tse-Eng D, Saha JR. Inactivation of digoxin by the gut flora: reversal by antibiotic therapy. N Engl J Med. 1981;305:789–794. doi:10.1056/NEJM198110013051403.
- Nakayama H, Kinouchi T, Kataoka K, Akimoto S, Matsuda Y, Ohnishi Y. Intestinal anaerobic bacteria hydrolyse sorivudine, producing the high blood concentration of 5-(E)-(2-bromovinyl)uracil that increases the level and toxicity of 5-fluorouracil. Pharmacogenetics. 1997;7:35–43. doi:10.1097/00008571-199702000-00005.
- Zimmermann M, Zimmermann-Kogadeeva M, Wegmann R, Goodman AL. Separating host and microbiome contributions to drug pharmacokinetics and toxicity. Science. 2019;363:eaat9931. doi:10.1126/science.aat9931.
- Ferl GZ, Theil F-P, Wong H. Physiologically based pharmacokinetic models of small molecules and therapeutic antibodies: a mini-review on fundamental concepts and applications. Biopharm Drug Dispos. 2016;37:75–92. doi:10.1002/bdd.1994.
- Schmitt W, Willmann S. Physiology-based pharmacokinetic modeling: ready to be used. Drug Discov Today Technol. 2004;1:449–456. doi:10.1016/j.ddtec.2004.09.006.
- Spanogiannopoulos P, Bess EN, Carmody RN, Turnbaugh PJ. The microbial pharmacists within us: a metagenomic view of xenobiotic metabolism. Nat Rev Microbiol. 2016;14:273–287. doi:10.1038/nrmicro.2016.17.
- Wilson ID, Nicholson JK. Gut microbiome interactions with drug metabolism, efficacy, and toxicity. Transl Res. 2017;179:204–222. doi:10.1016/j.trsl.2016.08.002.
- Koppel N, Maini Rekdal V, Balskus EP. Chemical transformation of xenobiotics by the human gut microbiota. Science. 2017;356:eaag2770. doi:10.1126/science.aag2770.
- Wallace BD, Wang H, Lane KT, Scott JE, Orans J, Koo JS, Venkatesh M, Jobin C, Yeh L-A, Mani S, et al. Alleviating cancer drug toxicity by inhibiting a bacterial enzyme. Science. 2010;330:831–835. doi:10.1126/science.1191175.
- Kuhn M, Letunic I, Jensen LJ, Bork P. The SIDER database of drugs and side effects. Nucleic Acids Res. 2016;44:D1075-9. doi:10.1093/nar/gkv1075.
- Pellock SJ, Redinbo MR. Glucuronides in the gut: sugar-driven symbioses between microbe and host. J Biol Chem. 2017;292:8569–8576. doi:10.1074/jbc.R116.767434.
- Roberts MS, Magnusson BM, Burczynski FJ, Weiss M. Enterohepatic circulation: physiological, pharmacokinetic and clinical implications. Clin Pharmacokinet. 2002;41:751–790. doi:10.2165/00003088-200241100-00005.
- Ilett KF, Tee LBG, Reeves PT, Minchin RF. Mebolism of drugs and other xenobiotics in the gut lumen and wall. Pharmacol Ther. 1990;46:67–93. doi:10.1016/0163-7258(90)90036-2.
- Colburn WA, Bekersky I, Min BH, Hodshon BJ, Garland WA. Contribution of gut contents, intestinal wall and liver to the first-pass metabolism of clonazepam in the rat. Res Commun Chem Pathol Pharmacol. 1980;27:73–90.
- Hofmann AF, Cravetto C, Molino G, Belforte G, Bona B. Simulation of the metabolism and enterohepatic circulation of endogenous deoxycholic acid in humans using a physiologic pharmacokinetic model for bile acid metabolism. Gastroenterology. 1987;93:693–709. doi:10.1016/0016-5085(87)90430-6.
- Custodio JM, Wu C-Y, Benet LZ. Predicting drug disposition, absorption/elimination/transporter interplay and the role of food on drug absorption. Adv Drug Deliv Rev. 2008;60:717–733. doi:10.1016/j.addr.2007.08.043.
- Ben GM, Thiele I. Model-based dietary optimization for late-stage, levodopa-treated, Parkinson’s disease patients. NPJ Syst Biol Appl. 2016;2:16013. doi:10.1038/npjsba.2016.13.
- Sier JH, Thumser AE, Plant NJ. Linking physiologically-based pharmacokinetic and genome-scale metabolic networks to understand estradiol biology. BMC Syst Biol. 2017;11:141. doi:10.1186/s12918-017-0520-3.
- Sahoo S, Haraldsdóttir HS, Fleming RMT, Thiele I. Modeling the effects of commonly used drugs on human metabolism. Febs J. 2015;282:297–317. doi:10.1111/febs.13128.
- Cordes H, Thiel C, Baier V, Blank LM, Kuepfer L. Integration of genome-scale metabolic networks into whole-body PBPK models shows phenotype-specific cases of drug-induced metabolic perturbation. NPJ Syst Biol Appl. 2018;4:10. doi:10.1038/s41540-018-0048-1.
- Krauss M, Schaller S, Borchers S, Findeisen R, Lippert J, Kuepfer L. Integrating cellular metabolism into a multiscale whole-body model. PLoS Comput Biol. 2012;8:e1002750. doi:10.1371/journal.pcbi.1002750.
- Arnoldini M, Cremer J, Hwa T. Bacterial growth, flow, and mixing shape human gut microbiota density and composition. Gut Microbes. 2018:1–8. doi:10.1080/19490976.2018.1448741.
- Cremer J, Arnoldini M, Hwa T. Effect of water flow and chemical environment on microbiota growth and composition in the human colon. Proc National Acad Sci. 2017;114:6438–6443. doi:10.1073/pnas.1619598114.
- Maier L, Pruteanu M, Kuhn M, Zeller G, Telzerow A, Anderson EE, Brochado AR, Fernandez KC, Dose H, Mori H, et al. Extensive impact of non-antibiotic drugs on human gut bacteria. Nature. 2018;555:623–628. doi:10.1038/nature25979.
- Falony G, Joossens M, Vieira-Silva S, Wang J, Darzi Y, Faust K, Kurilshikov A, Bonder MJ, Valles-Colomer M, Vandeputte D, et al. Population-level analysis of gut microbiome variation. Science. 2016;352:560–564. doi:10.1126/science.aad3503.
- Zhernakova A, Kurilshikov A, Bonder MJ, Tigchelaar EF, Schirmer M, Vatanen T, Mujagic Z, Vila AV, Falony G, Vieira-Silva S, et al. Population-based metagenomics analysis reveals markers for gut microbiome composition and diversity. Science. 2016;352:565–569. doi:10.1126/science.aad3369.
- Thelen K, Coboeken K, Willmann S, Burghaus R, Dressman JB, Lippert J. Evolution of a detailed physiological model to simulate the gastrointestinal transit and absorption process in humans, Part 1: oral solutions. J Pharm Sci. 2011;100:5324–5345. doi:10.1002/jps.22726.
- Jamei M, Turner D, Yang J, Neuhoff S, Polak S, Rostami-Hodjegan A, Tucker G. Population-based mechanistic prediction of oral drug absorption. Aaps J. 2009;11:225–237. doi:10.1208/s12248-009-9099-y.
- Kirchmair J, Göller AH, Lang D, Kunze J, Testa B, Wilson ID, Glen RC, Schneider G. Predicting drug metabolism: experiment and/or computation? Nat Rev Drug Discovery. 2015;14:387–404. doi:10.1038/nrd4581.
- Wienkers LC, Heath TG. Predicting in vivo drug interactions from in vitro drug discovery data. Nat Rev Drug Discovery. 2005;4:825–833. doi:10.1038/nrd1876.
- Wu C-Y, Benet LZ. Predicting drug disposition via application of BCS: transport/absorption/elimination interplay and development of a biopharmaceutics drug disposition classification system. Pharm Res. 2005;22:11–23. doi:10.1007/s11095-004-9004-4.
- Zisaki A, Miskovic L, Hatzimanikatis V. Antihypertensive drugs metabolism: an update to pharmacokinetic profiles and computational approaches. Curr Pharm Des. 2015;21:806–822. doi:10.2174/1381612820666141024151119.
- Hadadi N, MohammadiPeyhani H, Miskovic L, Seijo M, Hatzimanikatis V. Enzyme annotation for orphan and novel reactions using knowledge of substrate reactive sites. Proc National Acad Sci. 2019;116:7298–7307. doi:10.1073/pnas.1818877116.
- Klünemann M, Schmid M, Patil KR. Computational tools for modeling xenometabolism of the human gut microbiota. Trends Biotechnol. 2014;32:157–165. doi:10.1016/j.tibtech.2014.01.005.
- Sharma AK, Jaiswal SK, Chaudhary N, Sharma VK. A novel approach for the prediction of species-specific biotransformation of xenobiotic/drug molecules by the human gut microbiota. Sci Rep. 2017;7:9751. doi:10.1038/s41598-017-10203-6.
- Jones H, Chen Y, Gibson C, Heimbach T, Parrott N, Peters S, Snoeys J, Upreti V, Zheng M, Hall S. Physiologically based pharmacokinetic modeling in drug discovery and development: a pharmaceutical industry perspective. Clin Pharmacol Ther. 2015;97:247–262. doi:10.1002/cpt.37.
- Doestzada M, Vila AV, Zhernakova A, Koonen DPY, Weersma RK, Touw DJ, Kuipers F, Wijmenga C, Fu J. Pharmacomicrobiomics: a novel route towards personalized medicine? Protein Cell. 2018;9:432–445. doi:10.1007/s13238-018-0547-2.