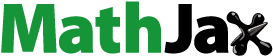
ABSTRACT
The prevalence and occurrence of mucin-degrading (MD) bacteria, such as Akkermansia muciniphila and Ruminococcus gnavus, is highly associated with human health and disease states. However, MD bacterial physiology and metabolism remain elusive. Here, we assessed functional modules of mucin catabolism, through a comprehensive bioinformatics-aided functional annotation, to identify 54 A. muciniphila genes and 296 R. gnavus genes. The reconstructed core metabolic pathways coincided with the growth kinetics and fermentation profiles of A. muciniphila and R. gnavus grown in the presence of mucin and its constituents. Genome-wide multi-omics analyses validated the nutrient-dependent fermentation profiles of the MD bacteria and identified their distinct mucolytic enzymes. The distinct metabolic features of the two MD bacteria induced differences in the metabolite receptor levels and inflammatory signals of the host immune cells. In addition, in vivo experiments and community-scale metabolic modeling demonstrated that different dietary intakes influenced the abundance of MD bacteria, their metabolic fluxes, and gut barrier integrity. Thus, this study provides insights into how diet-induced metabolic differences in MD bacteria determine their distinct physiological roles in the host immune response and the gut ecosystem.
Introduction
Intestinal homeostasis is a prerequisite for stabilizing the gut ecosystem and involves collaboration between microbiota and intestinal epithelial cellsCitation1. The highly o-glycosylated mucus layer is vital in selecting the intestinal gut microbiota and improving gut barrier functions of the mucosal epitheliumCitation2,Citation3. This healthy gut ecosystem is highly associated with host innate immunityCitation4 and genetic variationCitation5. Intestinal epithelial cells (IECs) and macrophages in the intestinal immune system play a role in maintaining gut homeostasis as the first defense mechanism and as a messenger of signals from microbiota to other host cellsCitation6. A large body of evidence on the impact of microbiota on human gut health and disease supports the notion that host diet alters the interaction between gut microbiota (and their metabolites) and host cells or microbiotaCitation7,Citation8. Indeed, inadequate fiber intake reshapes the thickness of mucus layers, mainly due to the abundance of mucin-degrading (MD) bacteriaCitation9, causing gut microbial dysbiosis and dysregulation of the immune systemCitation10. Leaky gut-induced dysbiosis and a dysfunctional colonic epithelium increase the O2 concentration in the gastrointestinal (GI) tract, leading to a significant decrease in obligate anaerobesCitation11. Since gut dysbiosis affects the host immune system, it is highly associated with many non-communicable human diseasesCitation12 such as obesityCitation13, diabetesCitation14, allergyCitation15,Citation16, cancerCitation17, and neurological disorder.
The gut microbiome in healthy individuals mainly comprises various types of microorganisms, including bacteria (e.g., Firmicutes, Bacteroidetes, Actinobacteria, Proteobacteria, Verrucomicrobia)Citation18, fungi, and viruses. Among these, glycan-utilizing anaerobes in the intestinal mucus layer have emerged as critical players in shaping the gut ecosystem and reprogramming the metabolism of the host intestinal epitheliumCitation19–21. MD bacteria provide essential nutrients such as acetate, propionate, and 1,2-propanediol (1,2-PDO) for developing commensal bacteria through cross-feeding networks with butyrate-producing bacteriaCitation22. Metabolites produced by MD bacteria contribute to the homeostasis of the human gut ecosystem and to niche protection by inhibiting pathogenic bacteriaCitation18, activating the immune systemCitation4,Citation23, and modulating host signaling and metabolismCitation24.
Certain MD bacteria are linked to various human diseases, such as inflammatory bowel diseases (IBD), prosthesis infections, hematological malignancies, and atopic dermatitis. For example, the beneficial bacterium Akkermansia muciniphila is associated with a healthy gut microbiota and has been found in higher abundance in healthy individualsCitation16,Citation25,Citation26. It has been linked to improved metabolic health, including the regulation of blood sugar levels and weight lossCitation27. Furthermore, A. muciniphila has anti-inflammatory properties and can enhance the gut barrier functionCitation28. Low abundance or absence of A. muciniphila has also been observed in individuals with obesityCitation29, type 2 diabetesCitation30, and IBDCitation31. While A. muciniphila has several potential health benefits, its role in disease is not yet fully understood. Some studies have suggested that overgrowth of A. muciniphila could be associated with the development of colorectal cancerCitation32,Citation33 and aggravation of type 2 diabetes and neurodegenerative diseasesCitation34.
By contrast, increased levels of Ruminococcus gnavus and R. torques cause leaky gut-induced inflammationCitation35–37. The mucolytic activity of R. gnavus produces inflammatory glucorhamnan polysaccharides that induce inflammatory cytokines such as TNFαCitation36. This bacterium produces an antioxidant defense-related NADH oxidase, iron ABC transporters, and enterochelin esterase, which are involved in host pathologic phenotypeCitation38, and a lanti-peptide synthase, which inhibits other gut microbesCitation39. Collectively, empirical evidence supports the notion that the prevalence and occurrence of MD bacteria, as symbionts, play a crucial role in modulating the gut ecosystem and maintaining intestinal homeostasisCitation3,Citation22. However, the physiological features of MD bacteria and their metabolic impacts on the host are still not clearly understood.
In this study, we performed comprehensive multi-omics analyses to investigate the distinct phenotypes of R. gnavus and A. muciniphila under various culture conditions. To understand the physiological characteristics and metabolic features of both bacteria, we also analyzed their detailed fermentation profiles on various mucus constituents and examined the effect of their fermentation products on host immune cells. Based on the integration of these data with reconstructed anaerobic core metabolic pathways, we discuss the nutrient-dependent metabolic features of MD bacteria underlying diet-induced alterations of gut microbiota and how these affect host-microbe interactions in the human gut environment.
Results
Inconsistent functional annotation impedes understanding of the metabolic features of MD bacteria
According to the NCBI prokaryotic genome annotation pipeline (PGAP), the genomes of A. muciniphila ATCC BAA−835 (2.66 Mb) and R. gnavus ATCC 29149 (3.55 Mb) have 2,122 and 3,350 protein-coding sequences (CDSs), respectively (Table S1). To investigate their genomic features, we performed the Clusters of Orthologous Genes (COG) analysis with updated versions of Gene Ontology in the eggNOG database (Figure S1). However, we discovered that there are discrepancies in functional annotations between the NCBI PGAP and other pipelines (PathwayTools and ModelSEED) for both bacterial genomes. The agreement of functions and length of CDSs between annotation pipelines was 23.5% (38.1%) for A. muciniphila and 23.9% (66.5%) for R. gnavus (). When we examined the mismatches (−2 to 2) in gene annotation for individual CDSs, we found that only 40.4% of proteins were well-matched (0 to 2) for A. muciniphila and 41.7% for R. gnavus (Figure S2a,b). By contrast, the genomes of A. muciniphila and R. gnavus had 97 and 104 mismatched genes (including 13 and 5 MD genes), respectively (Figure S2).
Figure 1. Functional annotations of the MD bacterial genomes and growth profiles of A. muciniphila and R. gnavus grown in different media. (a-b) Inconsistent function and CDS for A. muciniphila (a) and R. gnavus (b). Gene predictions by gene callers coincide only if both start and stop codons are predicted to be in the same positions. Several missing and unmatched genes are based on the current functional annotations with different algorithms and databases used. (c-d) Growth curves and fermentation profiles of (c) A. muciniphila grown in BHI and ADM and (d) R. gnavus grown in TSB and defined medium supplemented with various sugars. Growth curves data from (c) were adopted from previous dataCitation40. (e-f) The effects of various nutrients on SCFA production and flux balance analysis for the fermentation products of A. muciniphila (e) and R. gnavus (f).
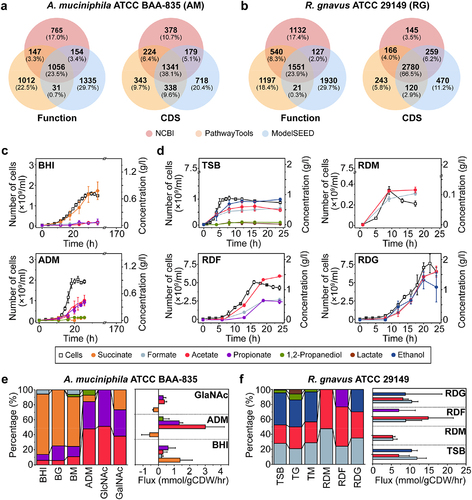
PGAP and PathwayTools showed relatively consistent results on the CDS and RNAs of A. muciniphila and R. gnavus because the latter relies on NCBI BLAST search to predict metabolic and transport reactions using the MetaCyc database (DB). On the other hand, ModelSEED annotates proteins with k-mer based on the CoreSEED-based DB (core.theseed.org), revealing 2,576 CDSs and 62 RNAs for A. muciniphila and 3,629 CDSs and 68 RNAs for R. gnavus, respectively (Table S1). Consequently, we found that the total number of genes, CDSs, and RNAs for MD bacteria varies significantly in DB and algorithm-dependent manners. In particular, genes associated with the assimilatory pathways responsible for producing the primary metabolites of MD bacteria also remained elusive. Thus, the current versions of genome annotations and core metabolic pathways, for both of the MD bacteria being analyzed, need to be revised, particularly for glycan degradation, sugar metabolism, amino acid and vitamin biosynthesis, and mucolytic enzymes (Figure S2c,d).
Reconstructed core metabolism elucidated the mucin-dependent fermentation of MD bacteria
We attempted to reannotate mismatched/unassigned 1,066 A. muciniphila genes (~50.2% of the total CDSs after Phase I) and 1,799 R. gnavus genes (~53.7%) (Figure S3 and Dataset S1). Firstly, we performed comprehensive domain and structure similarity searches for the CDSs of both MD bacteria using an InterPro DB-based analysis (Phase II). We further analyzed the genome contexts of the relevant protein-encoding genes by constructing gene arrays and clusters, from conserved gene pairs, to identify several mismatched and unassigned genes using the PATRIC DB (www.patricbrc.org). In Phase III, we reassigned 1,086 A. muciniphila genes (51.2% of the total genes) and 1,843 R. gnavus genes (55.0%) (Dataset S2). Further, we annotated unmatched and/or mismatched genes involved in core metabolism and mucolytic enzymes using CAZy, MEROPS, and SulfAtlas DBs (Phase IV). For further validation, these annotated genes were subjected to protein structure homology modeling, using SWISS-MODEL, before structural comparison to previously published homologues (Dataset S3). Consequently, we reassigned 1,110 proteins (52.3%) for A. muciniphila and 1,847 proteins (55.1%) for R. gnavus.
The PGAP- and ModelSEED-based annotations revealed that A. muciniphila possess a functional module for the Embden-Meyerhof-Parnas (EMP) and propionate fermentation pathway (Figure S4a). The ModelSEED-based AMUC_RS10850 annotation revealed that A. muciniphila could not ferment mucin-derived sugars and amino acids to propionate. However, our annotations revealed that AMUC_RS10850 was reassigned as pyruvate carboxylase, coincided with the PGAP annotation (Dataset S4), indicating that this bacterium can produce propionate via pyruvate. Although AMUC_RS08380 and AMUC_RS10200 were annotated as alcohol dehydrogenases in all DBs, A. muciniphila is unlikely to encode the pyruvate decarboxylase enzyme needed for ethanol production. We concluded, therefore, that A. muciniphila can produce propionate through a reductive TCA cycle, via methylmalonyl-CoA, from various mucin-derived sugars.
Unlike A. muciniphila, R. gnavus has mixed-acid fermentation pathways for various SCFAs except for succinate (Figure S4b). For instance, we reannotated several functional genes (i.e., RGna_15055, RGna_15060, and RGna_15070) involved in 1,2-PDO degradation (Dataset S4). Accordingly, R. gnavus may ferment mucin-derived fucose to propionate using lactaldehyde reductase (RGna_15100) through the 1,2-PDO degradation pathway. In addition, the genome context analysis assigned RGna_15025 as a glycyl radical protein, which activates pyruvate formate lyase for 1,2-elimination and 1,2-PDO utilization reactions. Consequently, our comprehensive annotations enabled us to manually curate 14 enzymes involved in the core metabolic pathways for A. muciniphila and R. gnavus (Dataset S4). We also curated 25 enzymes potentially involved in mucin degradation for these bacteria. Indeed, A. muciniphila and R. gnavus have a variety of mucolytic enzymes, including carbohydrate-binding modules (CBMs), glycoside hydrolases (GHs), peptidases, and sulfatases (Figure S4c). However, the GHs of A. muciniphila markedly differ from those of R. gnavus (Figure S4d). Therefore, the distinct sets of mucolytic enzymes in these MD bacteria may allow them to utilize different mucin-derived substrates, resulting in their different metabolic profiles.
The growth rates and metabolic features of MD bacteria vary in nutrient-dependent manners
The two MD bacteria were cultivated in rich and defined media supplemented with various mucin constituents to quantify biomass and production yields. A. muciniphila grown in BHI medium primarily produced succinate (0.78 g/L), with much lower levels of propionate, acetate, and formate (). The addition of 1% glucose or 0.5% mucin to BHI increased biomass yields (Yx/s) 1.58- and 1.82-fold, succinate production to 1.13 g/L and 0.94 g/L, and propionate production to 0.21 g/L and 0.19 g/L, respectively (Fig. S5a and Table S2). On the other hand, A. muciniphila grown in its defined medium (AD) supplemented with 0.5% mucin (ADM) mainly produced propionate and acetate (). Production yield (Yp/s) and cell yield (Yx/s) were 6.3-fold and 10-fold higher, respectively, than those in BHI (Table S2). Moreover, despite significant growth retardation, A. muciniphila grown in AD with N-acetylated galactosamine (GalNAc) and 25 mM threonine produced acetate and propionate exclusively with a 2.6-fold higher Yp/s than A. muciniphila grown in BHI (Figure S5b). These results suggest that SCFA production is influenced by the availability of sugars derived from mucin (). Notably, the highest specific growth rate (μ) of A. muciniphila in ADM indicates a higher substrate preference for mucin than for other sugars. Collectively, the fermentation of sugars from mucin by A. muciniphila results in the production of propionate and acetate, as the predominant SCFAs, and succinate, 1,2-PDO, and formate as minor metabolites.
R. gnavus grown in the Tryptic Soy Broth (TSB) medium produced ethanol, acetate, and formate, with low levels of lactate and 1,2-PDO (). The addition of glucose or mucin to TSB altered neither the organic acid profiles nor Yp/s and Yx/s. However, this bacterium exhibited the highest specific growth rate in TSB supplemented with mucin (Table S3). R. gnavus grown in a defined medium (RD) supplemented with other sugars except for mucin and fucose, fermented sugars to yield the same primary fermentation products ( and S5c-d). Cells grown in RD supplemented with various sugars and amino acids exhibited 5- to 8-fold higher Yp/s and Yx/s, respectively, than in TSB (Table S3). However, supplementation with either amino acids (alone) or mucin did not increase yield coefficients, indicating that, unlike A. muciniphila, R. gnavus did not grow well in defined medium supplemented solely with mucin. The fermentation profiles of R. gnavus varied when it was grown in RD supplemented with mucin (RDM), fucose (RDF), or galactose (RDG) (). Cells grown in the presence of mucin produced acetate and formate primarily, whereas growth in RDF resulted in the production of acetate, formate, and propionate via 1,2-PDO degradation ().
Multivariate principal component analysis (PCA) was performed to compare the metabolites derived from different nutrients. The PCA plot showed a clear distinction between the bacterial cultured media and the uncultured media samples (Figure S6a,b). Of the metabolites significantly altered by bacterial culture broths, 54 metabolites were identified using accurate mass and/or MS/MS fragmentation with the help of databases and the literature (Dataset S5). Among them, 3-(indol−3-yl) lactate (ILA, C11H11NO3) was found in significant amounts in the cultures of both MD bacteria grown on mucin or its constituents (Figure S6c). In A. muciniphila, ILA production was significantly higher in AD medium supplemented GalNAc (341.3-fold) than in ADM (11.3-fold) (Figure S6d). In R. gnavus, ILA production was increased in RDM (485.7-fold), RDF (2.5-fold), and RDG (34.7-fold), respectively. Although both MD bacteria lack indole pyruvate-mediated pathways for the production of ILA from tryptophan, they still produce ILA. Additionally, an ion (204.0688 m/z) that is predicted to be an ILA derivative was found only when R. gnavus was cultured in RDF. By contrast, this metabolite was produced when A. muciniphila, was grown in AD supplemented with either mucin or GalNAc, indicating that mucin constituents may induce the production of ILA derivatives in both MD bacteria.
Both MD bacteria exhibited nutrient-dependent changes in morphology and membrane fatty acid composition
Electron microscopy revealed that A. muciniphila cells grown in BHI or AD supplemented with GalNAc were oval-shaped (Figure S7a,b). However, the surface layer of A. muciniphila cells grown in ADM was rougher than that of A. muciniphila cells grown in BHI, and the cells also formed long chains. The unsaturated fatty acid content of cells grown in ADM was higher than in other media (Figure S7c). Intriguingly, the levels of C16:0 and C18:0 fatty acids increased when cells were grown on a mucin-containing medium, whereas those of iso-C14:0 and anteiso-C15:0 fatty acids decreased significantly (Dataset S6). R. gnavus cells predominantly had a coccus-shape, but this varied in a nutrient-dependent manner (Figure S7d,e). Cells grown in RDM aggregated, and the thickness of each cell was slightly wider than cells grown in TSB. When R. gnavus was grown in RDF, cells were more compact and formed longer chains than those grown in TSB. In addition, a net-like structure was observed around the cell wall when R. gnavus was cultured in RDM and RDF (Figure S7e). The unsaturated fatty acid content of cells grown in TSB was lower than those grown in other media. In contrast to the levels of C14:0 and C16:0 dimethoxyhexadecane (DMA), those of C16:0 and C18:0 increased when cells were grown on mucin (Figure S7f and Dataset S6). Thus, changes in membrane thickness and cell morphology correlated with the unsaturated fatty acid contents of cellular membranes and the lengths of fatty acids, indicating that cellular membrane fluidity is associated with mucin utilization by MD bacteria.
Comparative transcriptomic and proteomic analyses revealed the unique metabolic features of A. muciniphila and R. gnavus with distinct MD activities
When gene expression in exponential-phase cells of A. muciniphila grown in ADM or AD with GalNAc and Thr was compared with gene expression in cells grown in BHI, 549 differentially expressed genes (DEGs) and 285 DEGs, respectively, were identified (). COG analysis revealed DEGs between cells grown in ADM and those grown in BHI involved in energy production and conversion, carbohydrate transport and metabolism, amino acid transport and metabolism, and cell wall/membrane biogenesis (). Remarkably, GlcNAc−6-phosphate deacetylase (AMUC_RS05110) was increased by 13.75-fold, indicating that mucin-derived GlcNAc serves as a primary nutrient for bacterial growth and acetate production, consistent with the fermentation data (). In the reductive TCA cycle, AMUC_RS09035 and 09040 involved in the production of succinyl-CoA from 2-oxoglutarate were up-regulated, which might be related to an increase in propionate in ADM. In particular, up-regulated expression levels of AMUC_RS06705 (glutamine synthetase III), AMUC_RS00215 (glutaminase A), AMUC_RS06715 (glutamate synthase), and amino acid permease (AMUC_RS00210/03895) might furnish amino acids and building blocks through arginine biosynthesis for bacterial growth. Furthermore, genes, including the chaperones HtpG/GroES/DnaK and protein turnover, were up-regulated, demonstrating that A. muciniphila might express these genes together with glycan degradation enzymes (e.g., GH2/20/29 and sulfatase) when mucin is the primary nutrient for bacterial growth. On the other hand, rmlB and gmd genes involved in lipopolysaccharides (LPS) synthesis were down-regulated, indicating that A. muciniphila generates lower amounts of LPS when it is grown in ADM than when it is grown in BHI. Thus, A. muciniphila showed unique expression patterns for utilizing mucin or its constituents.
Figure 2. Nutrient-dependent distribution of gene expression for A. muciniphila and R. gnavus. (a) Volcano plot of RNA-seq transcriptome data of A. muciniphila grown in ADM versus BHI. Up represents significantly up-regulated genes [≥2-fold change (FC) in normalized transcripts per million (TPM), P-value (P) <0.05]. (b) Proposed metabolic features when A. muciniphila was grown in ADM versus those when it was grown in BHI. Red color: up-regulated genes and metabolic features in mucin-containing medium. Blue color: up-regulation in each media. (c) Dot plot of enriched analysis performed using the KEGG database when A. muciniphila was grown in ADM versus BHI. (d) Volcano plot of RNA-seq transcriptome data of A. muciniphila grown in ADM versus GalNAc. (e) Proposed metabolic features of A. muciniphila grown in ADM versus GalNAc. (f) Dot plot of results of enrichment analysis when A. muciniphila was grown in ADM versus GalNAc. (g) Volcano plot of RNA-seq transcriptome data of R. gnavus grown in RDM versus TSB. (h) Proposed metabolic features of R. gnavus grown in RDM versus TSB. (i) Dot plot of the results of enrichment analysis when R. gnavus was grown in RDM versus TSB. Dot plot showing the four enriched pathways. (j) Volcano plot of RNA-seq transcriptome data of R. gnavus grown in RDM versus RDF. (k) Proposed metabolic features of R. gnavus grown in RDM versus RDF. (l). Dot plot of results of enrichment analysis when R. gnavus was grown in RDM versus RDF.
![Figure 2. Nutrient-dependent distribution of gene expression for A. muciniphila and R. gnavus. (a) Volcano plot of RNA-seq transcriptome data of A. muciniphila grown in ADM versus BHI. Up represents significantly up-regulated genes [≥2-fold change (FC) in normalized transcripts per million (TPM), P-value (P) <0.05]. (b) Proposed metabolic features when A. muciniphila was grown in ADM versus those when it was grown in BHI. Red color: up-regulated genes and metabolic features in mucin-containing medium. Blue color: up-regulation in each media. (c) Dot plot of enriched analysis performed using the KEGG database when A. muciniphila was grown in ADM versus BHI. (d) Volcano plot of RNA-seq transcriptome data of A. muciniphila grown in ADM versus GalNAc. (e) Proposed metabolic features of A. muciniphila grown in ADM versus GalNAc. (f) Dot plot of results of enrichment analysis when A. muciniphila was grown in ADM versus GalNAc. (g) Volcano plot of RNA-seq transcriptome data of R. gnavus grown in RDM versus TSB. (h) Proposed metabolic features of R. gnavus grown in RDM versus TSB. (i) Dot plot of the results of enrichment analysis when R. gnavus was grown in RDM versus TSB. Dot plot showing the four enriched pathways. (j) Volcano plot of RNA-seq transcriptome data of R. gnavus grown in RDM versus RDF. (k) Proposed metabolic features of R. gnavus grown in RDM versus RDF. (l). Dot plot of results of enrichment analysis when R. gnavus was grown in RDM versus RDF.](/cms/asset/5a1e0d90-3e05-4d39-a013-b031e923411a/kgmi_a_2221811_f0002_oc.jpg)
Gene set enrichment analysis (GSEA) of DEGs revealed that bacterial defense systems were all down-regulated when A. muciniphila was grown in the presence of mucin (, S8 and S9). On the other hand, nitrogen, Ala, Asp, and Glu metabolic modules were up-regulated in ADM, indicating that the biosynthesis of these amino acids is required for bacterial growth. Some proteins (i.e., AMUC_RS06705, AMUC_RS00215, and AMUC_RS02075) were highly expressed in cells grown in ADM compared with cells grown in BHI or were exclusively identified in cells grown in ADM (i.e., AMUC_RS00215). Additionally, amino sugar and nucleotide sugar metabolism, other glycan degradation, and sphingolipid metabolism were highly up-regulated when A. muciniphila was grown in the presence of mucin compared with growth in the presence of GalNAc ( and S8b).
Transcriptome analysis revealed 440 DEGs between R. gnavus grown in RDM and R. gnavus grown in TSB and 249 DEGs between cells grown in RDM and those grown in RDF (). COG analysis revealed that cells grown in RDM induced genes involved in energy production and conversion, nucleotide transport and metabolism, carbohydrate transport and metabolism, transcription, replication/recombination and repair, and cell wall/membrane biogenesis (). In particular, fucose isomerase (RGna_15280) and carbohydrate kinase (RGna_15275) were 11.16-fold and 4.41-fold higher in cells grown in RDM than in those grown in TSB, respectively. ManNAc−6-phosphate 2-epimerase (RGna_08350), sialidase (GH33, RGna_08355) were also 7.81-fold and 8.81-fold higher, respectively. These results indicated that mucin-derived fucose and neuraminate serve as nutrients for R. gnavus, in agreement with the growth data (). Moreover, the expression of RGna_ 04335 and 04340 encoding ABC transporter permeases involved in localization was 24.29-fold and 26.59-fold higher, respectively, in cells grown in RDM than in those grown in RDF, suggesting that these transports might be neuraminate transport. Following glycolysis, pfl (RGna_15515), ldh (RGna_09990), and adhE (RGna_08150) were down-regulated, resulting in decreased production of formate, lactate, and ethanol, in support of the fermentation data in RDM (). Interestingly, the expression of RGna_15045 and RGna_15100 involved in the degradation of fucose and 1,2-PDO was significantly higher in R. gnavus grown in RDM than in R. gnavus grown in RDF (). Together with the proteomic data (Figure S10d), the transcriptomic results confirmed that R. gnavus grown on fucose produced propionate but rarely ethanol (). Moreover, the transcriptomic and/or proteomic data validated gene functions, such as RGna_15035 (fuculose-phosphate aldolase), RGna_15100 (propanal dehydrogenase), RGna_15055 (EutM/PduA/PduJ-like protein 2), and RGna_15060/15065 (propanediol utilization protein PduA), which were highly up-regulated in RDF (Datasets S8–9 and Figure S10d). In addition, the DeoR/GlpR transcriptional regulator (RGna_07620), involved in posttranscriptional processing, and the Crp/Fnr family transcriptional regulator (RGna_07660), involved in responses to environmental changes, were up-regulated in RDM. However, FtsE (RGna_07475) and FtsX (RGna_07470) involved in cell division were down-regulated in RDM compared to RDF. In addition, sortase (RGna_09235) and SpaH/EbpB family (RGna_09240), involved in pilus polymerization, were down-regulated in RDM compared with TSB or RDF. Those genes might be involved in determining cell morphological shape, resulting in more compact growth in RDF (Figure S7).
GSEA revealed that two metabolic modules, oxidative phosphorylation and ascorbate/aldarate metabolism, were commonly up-regulated in cells grown in RDM compared with cells grown in TSB or RDF ( and S7c-d). Genes involved in ascorbate/aldarate metabolism were also specifically up-regulated after growth in RDM compared with growth in RDF, suggesting that R. gnavus might use ascorbate as a carbon source via pentose and glucuronate interconversions, using several genes (i.e., RGna_07065, 07615, 15135, and 15,275) highly up-regulated in RDM (Dataset S8). By contrast, genes involved in fatty acid biosynthesis and metabolism were down-regulated following growth in RDM compared with growth in TSB (Figure S8c), whereas genes involved in Arg metabolism, 2-Oxocarboxylic acid metabolism, and microbial metabolism in diverse environments were down-regulated when R. gnavus was grown in RDM compared with growth in RDF (Figure S8d).
A comparison of DEGs related to glycan degradation and GSEA revealed that A. muciniphila has a much broader substrate preference for mucin than R. gnavus (), thereby promoting a higher biomass yield for A. muciniphila grown on mucin. Indeed, GHs (GH 2, 16, 20, 89, 95, and 110), peptidases (M60 metallopeptidase), and sulfatase were highly expressed when A. muciniphila was grown in ADM (), in partial agreement with previous proteomic dataCitation40. However, R. gnavus does not express GHs 16, 20, and 89 or sulfatases. In addition, among five GH 2 enzymes, only RGna_07950 was highly up-regulated following growth in RDM. Rather, R. gnavus showed higher expression levels of distinct glycolytic enzymes such as GHs 29, 33, 95, 98, and peptidases (). Some putative mucolytic enzymes (i.e., GH 2 and 42) were more highly expressed in cells grown in TSB than in those growth in RDM, indicating that these enzymes, including GH 2 (RGna_11790 and RGna_12080) and GH 42 (RGna_14530) are probably not specifically involved in mucin degradation. Among GHs, CBMs 32 and 51 were highly up-regulated in A. muciniphila following growth in mucin, whereas CBMs 40, 48, and 50 were highly up-regulated in R. gnavus, suggesting that after bacterial adhesion and degradation of mucin, the utilization of available nutrient sources differs between MD bacteria (). Collectively, growth medium-specific DEGs-based enrichment analyses indicated that MD bacteria use markedly different metabolic modules, including mucolytic enzymes for mucin catabolism, reflecting distinct bacterial growth, fermentation capacity, and mucolytic activity.
Figure 3. Mucin-degrading enzyme expression profiles based on transcriptome analysis of MD bacteria. (a-b) Heatmap visualization of the nutrient-dependent expression profiles of enzymes involved in mucin-degradation in A. muciniphila (a) and R. gnavus (b). (c) A. muciniphila-induced cleavage pattern of mucin. (d) R. gnavus-induced cleavage pattern of mucin. *, carbohydrate binding module (CBM). Bold letters: mucin-specific up-regulated enzymes. Regular letters: up-regulation of enzymes in mucin and complex media. Gray letters: the enzymes not up-regulated in media containing mucin.
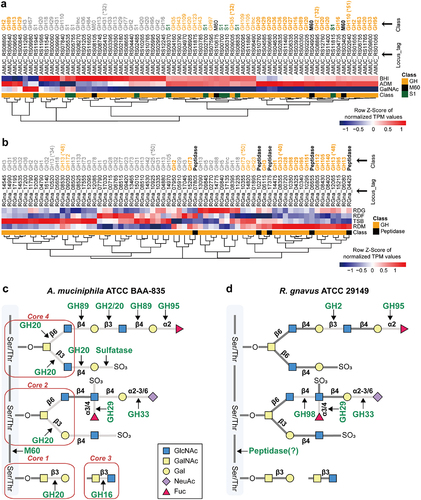
MD bacteria grown in specific nutrients differentially regulate host immune responses
To investigate the effect of MD bacterial metabolites on the host immune responses, we quantified the expression levels of metabolite receptors in RAW 264.7 macrophages treated with A. muciniphila- (AMCS) or R. gnavus-culture supernatants (RGCS). Notably, the expression level of Gpr41, which is activated by propionate and butyrate, was not significantly increased in macrophages treated with BHI-derived AMCS. By contrast, Gpr41 expression was significantly up-regulated in macrophages treated with ADM-derived AMCS (). Neither BHI-derived nor ADM-derived AMCS altered the expression level of Gpr43, which is activated by propionate, butyrate, and acetateCitation41. In addition, the expression level of the Gpr91 gene encoding GPR91 (SUCNR1), which is induced by succinateCitation42, was not significantly increased in macrophages treated with BHI-derived AMCS (). Thus, the increased concentration of propionate in ADM-derived AMCS specifically enhanced the expression level of Gpr41 in macrophages. These results correspond with SCFA profiles of A. muciniphila (), in which propionate was the predominant SCFA. In addition, the expression of the Mrgprd gene, which is induced by β-alanineCitation43, was also increased following exposure of macrophages to ADM-derived AMCS (). The n-methyl-d-aspartate receptor (NMDAR) is activated through either glutamate or aspartate bindingCitation44. The mRNA expression of Glun2a, which encodes a subunit of NMDAR, was up-regulated in macrophages exposed to both ADM- and BHI-derived AMCS. However, the expression level was higher in macrophages exposed to the ADM-derived AMCS (). These data correspond to the up-regulation of the metabolic modules associated with the synthesis of β-alanine, aspartate, and glutamate/nitrogen seen in the enrichment pathway when A. muciniphila was grown in ADM (Figure S8a). The mRNA expression of AhR was up-regulated only in ADM-derived AMCS together with a significant increase in ILA production (Figure S6d). Collectively, the results show that different metabolites produced by A. muciniphila in nutrient-dependent conditions differently activate the receptors on host macrophages. Moreover, metabolites secreted following growth in ADM are more potent stimulators of host immune cells than those secreted following growth in BHI.
Figure 4. The differential expression of metabolite receptors (a and b) and pro-inflammatory and anti-inflammatory markers (c and d) in macrophages regarding the different nutrients for MD bacteria. (a) mRNA expression of Gpr41, Gpr43, Gpr91, Mrgprd, and Glun2a in RAW 264.7 macrophages treated with culture supernatants of A. muciniphila grown in BHI or ADM for 24 h. (b) mRNA expression of Gpr41, Gpr43, AhR, and Cubn on RAW 264.7 macrophages treated with culture supernatants of R. gnavus grown in TSB, mucin, fucose, or galactose containing medium for 24 h. Results were obtained by RT-PCR and normalized to β-actin expression as relative expression. Uncultured media were used as the control. (c) mRNA expression of Il1b, Il6, Nos2, Il10, and Arg1 on RAW 264.7 macrophages treated A. muciniphila grown in BHI or ADM. (d) mRNA expression of Il1b, Il6, Nos2, Il10, and Arg1 on RAW 264.7 macrophages treated with R. gnavus grown in TSB, mucin, fucose, or galactose-containing media. The results were measured by RT-PCR and normalized with β-actin expression. Data are shown as mean SD, representative of two independent experiments. The comparison was made using a one-way ANOVA test. *P < 0.05, **P < 0.01, ***P < 0.001, ****P < 0.0001.
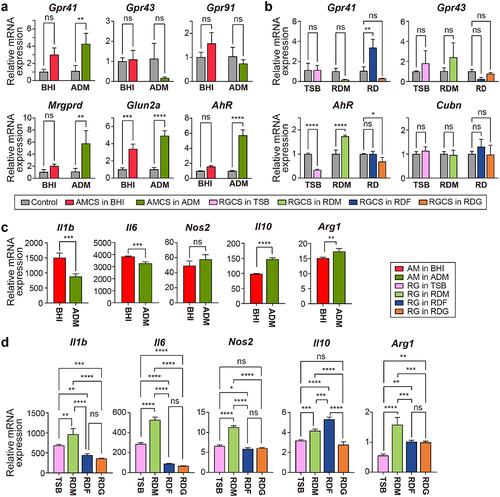
For R. gnavus, Gpr41 expression increased only in macrophages treated with RDF-derived supernatant (), which correlates with the increased propionate production observed when R. gnavus was grown in the presence of fucose (). However, there was no significant difference in Gpr43 expression level in macrophages treated with supernatants derived from any of the growth media. Thus, these data revealed that R. gnavus metabolites from fucose were likely to communicate with the host via GPR41. The expression level of AhR, a nuclear receptor induced by tryptophan metaboliteCitation45,Citation46, increased in macrophages treated with RDM-derived supernatant only () and is likely to be due to the presence of ILA, which was produced most abundantly following growth of R. gnavus in RDM (Figure S6d). Although media supplemented with fucose and galactose also stimulated the production of ILA by R. gnavus, RGCS derived following growth in these media did not induce higher AhR expression in macrophages. The expression of the cubilin receptor gene (Cubn) was comparable in macrophages exposed to RGCS derived from all MD bacteria cultures (), indicating that R. gnavus does not produce cubilin ligand(s). Collectively, these results suggest that R. gnavus likely produces metabolites that communicate with host cells.
Next, we examined whether A. muciniphila and R. gnavus cells following growth on different media elicited inflammatory-related signals in the host by measuring the cytokines and polarization markers in macrophages. ADM-derived A. muciniphila induced significantly lower levels of expression of the pro-inflammatory cytokines interleukin (Il)−1b and Il6 than BHI. By contrast, the expression level of the anti-inflammatory cytokine Il10 was higher in macrophages treated with the ADM than in those treated with the BHI (). Similarly, the expression level of Arginase 1 (Arg1), a marker of anti-inflammatory macrophages, was also significantly increased in macrophages treated with ADM-derived A. muciniphila (). The expression of inducible nitric oxide synthase (Nos2), a marker of pro-inflammatory macrophage, was comparable in macrophages treated with A. muciniphila derived from each growth media. Thus, these data suggest that when cultured in mucin-containing media, A. muciniphila produces extracellular metabolites with anti-inflammatory properties. On the other hand, R. gnavus grown in RDM showed higher expression of pro-inflammatory genes, Il1b, Il6, and Nos2 than R. gnavus grown in TSB, whereas R. gnavus grown in RDF or RDG showed significantly lower levels of pro-inflammatory gene expression than R. gnavus grown in RDM or TSB. Remarkably, R. gnavus following culture in RDF showed pronounced expression of Il10, whereas R. gnavus following growth in TSB or RDG did not. Finally, R. gnavus showed the highest expression of Arg1 when grown in RDM and lower expression when RDF-, RDG-, and TSB-grown R. gnavus (). Thus, when grown in media supplemented with mucin, R. gnavus induces both anti-inflammatory and pro-inflammatory immune responses.
Different dietary intakes significantly modulated the abundance of MD bacteria and the host gut mucosa response in vivo
We conducted an animal study using specific pathogen-free conditioned mice to investigate the effects of different diets on the abundance of MD bacteria and their metabolic features in the gut microbial community. The mice were fed with a high-fat diet containing 60% fat (HFD) or a fiber-rich diet supplemented with 10% inulin (ID) (). The HFD-fed mice gained significantly more body weight than the ID group (), and had increased inguinal white adipose tissue (iWAT) and epididymal white adipose tissue (eWAT) weights compared to the ID group (). More importantly, the modified diets differentially regulated the thickness of the mucus layer and goblet cell numbers in the colon, with thinner mucus layers and lower goblet cell numbers observed in the HFD-fed mice compared to ID-fed mice (). In addition, the expression of Il10 anti-inflammatory cytokine was significantly higher in the IECs isolated from colon of ID-fed mice compared to HFD-fed mice (Figure S11a). These results suggest that HFD induces improper mucus production, while ID maintains proper mucus production and regulates host gut immunity by inducing the anti-inflammatory cytokine expression.
Figure 5. Effects of high-fat diet and high-fiber diet on gut microbiota composition in mice. (a) Schematic of dietary intervention and sample collection. (b) Weekly body weight measurements of mice fed high-fat diet and high-fiber diet (n = 8). (c) Length of colon and weight of iWAT, and eWAT at 6 weeks of diet intervention (n = 8). (d) Inner colonic mucus thickness and goblet cell count (per crypt) (n = 8) (Significance of *P < 0.05 and ****P < 0.0001 was determined by unpaired-t-test). (e) Representative of colonic section stained by Alcian Blue/Periodic Acid-Schiff. (f) Alpha diversity of microbiota from mice fed high-fat diet and high-fiber diet. (g) Principal coordinate analysis of gut microbiota composition based on Bray-Curtis dissimilarity in mice fed high-fat diet and high-fiber diet. (h) Bar plots of gut microbial family composition in mice fed with high-fat diet and high-fiber diet, respectively. *P < 0.05, significant difference between the two groups indicated by asterisk. (i) Growth rates of MD bacteria and probiotics from mice fed high-fat diet and high-fiber diet. The comparison was performed using a paired t-test. *P < 0.05, **P < 0.01, ****P < 0.0001. Outliers are represented with the black diamonds. (j) Metabolic fluxes from mice fed high-fat diet and high-fiber diet.
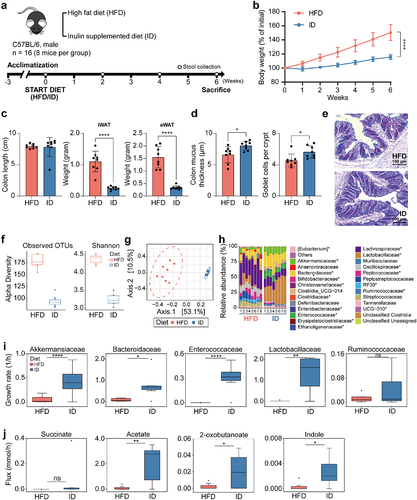
To investigate the effects of different diets on gut microbiota including MD bacteria, we analyzed the composition of gut microbiota in feces from mice fed with HFD and ID diets after 4-week diet intervention. Alpha diversity was significantly lower in mice fed with ID compared to HFD (; P = 0.001), while diet explained about 48% of the variability in microbiota composition (R2 = 0.48, P = 0.001). At the family levels, microbes belonging to 19 families showed significant differences between HFD and ID diets, excluding the unclassified group (P < 0.05), with Akkermansiaceae, Bacteroidaceae (including MD bacteria), Lactobacillaceae, and Enterococcaceae, significantly higher in mice fed with the ID compared to HFD, and Ruminococcaceae higher in mice fed with HFD (). We built a microbial community model at the family level using MICOMCitation47, which indicated that the growth rates of Akkermansiaceae, Bacteroidaceae, Lactobacillaceae, and Enterococcaceae were higher in the ID diet group (). Predicted metabolic fluxes showed that acetate, indole, and 2-oxobutanoate (as a precursor of propionate) fluxes were significantly higher in the ID diet, while succinate flux was not significantly different ( and Dataset S10). Our findings suggest that mucin-preferable bacteria, such as Akkermansia and Bacteroides, may affect the growth of commensal bacteria. Therefore, our results provide insights into the importance of dietary intake on taxonomic profiles of MD bacteria and their predicted metabolites.
The expression of Gpr41 and Gpr43, which are responsible for SCFA signaling, was slightly increased in ID-fed mice than HFD-fed mice (Figure S11b). However, the Ahr expression was comparable between HFD- and ID-fed mice. Our data suggest that a healthy mucus layer condition promoted by an inulin-supplemented diet plays a significant role in maintaining gut microbiota homeostasis, primarily by boosting MD bacteria function in the community. These results emphasize the importance of nutrition availability context for modulating MD bacteria activities, which could determine its phenotypes toward inflammation.
Discussion
In the present study, we reconstructed the core metabolic and fermentation pathways of A. muciniphila and R. gnavus to determine whether the metabolic features of these MD bacteria would differ when they are grown with and without mucin. Growth kinetics and multi-omics data suggested that MD bacterial SCFA profiles are influenced by the availability of mucin constituents (). In the presence of mucin, A. muciniphila produces propionate through the succinate pathway (Figure S4a), suggesting that mucolytic activity renders this bacterium beneficial for the gut ecosystem and host immune systemCitation48. By contrast, BHI-grown cells predominantly produced succinate and much less propionate and acetate than when grown in ADM (). Indeed, MD bacteria under nutrient-rich conditions, in the absence of mucin, are more detrimental than beneficial to host immunityCitation49. The variable fermentation profiles observed in this study point to microbe-specific phenotypes and this is likely to impede the discrimination between beneficial and harmful MD bacteria in terms of their effects on host health and disease progression. In the presence of mucin, R. gnavus produces formate and acetate, presumably due to its unavailability of mucolytic enzymes (). Nevertheless, this bacterium also produces pronounced amounts of propionate via the 1, 2-PDO degradation pathway, but only when fucose is abundant (). These results demonstrate that both of the MD bacteria have distinct substrate preferences for mucin constituents and express distinct profiles of mucolytic enzymes in a nutrient-dependent manner (). The differences in the fermentation profiles correlated with cell morphology and membrane fatty acid compositions (Figure S7). Cell envelope stress responses are highly associated with the modification of outer- and inner membranes which, in turn, affects cellular integrity, growth rates, and fermentation featuresCitation50. In this regard, the nutrient-dependent nature of SCFA production, metabolism, and cell morphology may contribute to niche adaptation and pathogenesis of MD bacteriaCitation51.
The GSEA data revealed that nitrogen metabolism (Ala, Asp, and Glu) was significantly up-regulated, concomitantly with amino acid biosynthesis by the enzymes glutamine synthase, glutamate: GABA antiporter, and amino acid permease, when A. muciniphila was grown in ADM (). Since A. muciniphila possesses a variety of GHs and peptidases for the utilization of mucin as a nutrient source, the metabolic products of these enzymes are likely to have knock-on effects on microbial glutamine auxotrophs and microbes that are sensitive to GABA, as observed previously for lactic acid bacteria (LABs) in the cross-feeding network of the gut ecosystemCitation52. Therefore, the metabolites produced by A. muciniphila might have potent effects in mucin-rich environments. The GSEA and metabolome results showed that R. gnavus had both beneficial and adverse effects on host gut immunity when it was grown in medium containing mucin. When R. gnavus adheres to mucin and produces glucorhamnan, it induces inflammatory cytokine secretion in the host gutCitation36. However, the expression of genes (RGna_17540 ~ 17650) involved in glucorhamnan polysaccharide biosynthesis was lower in R. gnavus in medium containing mucin than in TSB (Dataset S8). Oxidative phosphorylation, in addition to CBM expression, was up-regulated in R. gnavus grown in RDM, which might increase bacterial adhesion to mucusCitation53 (). In addition, R. gnavus produces ILA in the presence of mucin (Figure S6), which can have anti-inflammatory effects in the immature intestineCitation54. Furthermore, although high concentrations of long chain fatty acids (C12–20) can induce the expression of virulence genes in E. coli (EHEC)Citation55, R. gnavus probably does not induce virulence genes in the presence of mucin because the expression of fatty acid synthesis genes, including accB, fabD, fabF, fabG, and fabK, were lower when it was grown in RDM than when it was grown in TSB (Figure S8c). Taken together, these results demonstrate that the distinct metabolic features of MD bacteria play a crucial role in the maintenance of intestinal gut microbiota and the regulation of host gut epithelial immunity.
The dominance of MD bacteria and/or their mucolytic activities in the gut are directly related to the functionality of the highly o-glycosylated mucus layer in maintaining gut homeostasis. The o-glycosylated layer serves as a source of carbon, nitrogen, and sulfur for MD bacteria and other commensal bacteria in the human GI tract. Several specific mucolytic enzymes, including GHs (e.g., GH 16 family enzymes from Bacteroides sppCitation56, peptidases, and sulfatases, have been identified but have not yet been fully characterized. Since A. muciniphila has a high preference for mucin, it may flourish in environments such as the gut, where mucin is likely to be a major carbon source. The comparative transcriptomic data suggest that CBM 32/51 and sulfonate binding proteins (SBPs) (i.e., AMUC_RS00610, 02255, 02260, 06930, and 06940), which were highly up-regulated in ADM, may be novel mucolytic enzymes ( and Dataset S7). These cell surface enzymes directly bind to a component of mucin. In addition, GH 2 encoding CBM contributes to the cleavage of the Gal-β−1,3-GlcNAc bond, and GH 20 cleaves the core 1, 2, and 4 regions of o-glycan mucin. Furthermore, potential mucolytic enzymes include GHs 16, 29, 33, 89, 95, M60, and sulfatases (). In the case of R. gnavus, GH 33 encoding CBM 40 is likely to cleave the Gal-α2,3/6-GlcNAc bond because CBM 40 was highly up-regulated in RDM, and potential mucolytic enzymes also include GHs 2, 95, 98 (). However, this bacterium does not seem to cleave the core 1, 2, and 4 regions of o-glycan mucin due to lack of GH 20. Although peptidase might be used to cleave the glycoprotein backbone, further study is needed to determine which site is preferentially cleaved. Collectively, the results indicate that GH, CBM, and SBP enzymes play a crucial role in binding and cleaving mucin, indicating that A. muciniphila has a broader range of MD enzymes including GHs than R. gnavus.
Nutrient-dependent changes in propionate and butyrate produced by gut microbiota contribute to immune development and improve gut barrier function by stimulating GPR41 and GPR43Citation57. By contrast, succinate exacerbates autoimmune and autoinflammatory diseases via the receptor GPR91Citation58. Indeed, these SCFAs activated GPR 41 in macrophages () and higher GPR41 and GPR43 expression in colon epithelial cells was found in fiber-rich diet-fed mice (Figure S11b). Furthermore, the nutrient-dependent metabolism of A. muciniphila may also affect endocytosis and trafficking by host epithelial cells and macrophages. Such metabolic variations raises the issue of whether MD intestinal bacteria are pathobionts like mucolytic Helicobacter pylori Citation59 or (nonpathogenic) symbionts. Interestingly, MD bacteria exhibiting variable phenotypes can induce differential host inflammatory responsesCitation35. Recent studies have highlighted the beneficial role of MD bacteria such as A. muciniphila in ameliorating disease symptoms, such as IBD and obesityCitation60,Citation61. A. muciniphila has been suggested to attenuate inflammation by stimulating AhRCitation62. Furthermore, AhR was significantly up-regulated when this bacterium was grown in mucin (). Our in vivo experiment data also suggest that ID diet compared to HFD diet can increase indole, which is beneficial for intestinal barrier integrityCitation63 (). However, A. muciniphila, grown in mucin-deficient media (e.g., BHI and Columbia broth), exhibited pathogenicity in colitis-associated colorectal cancer and Salmonella typhimurium-induced gut inflammation in gnotobiotic miceCitation32,Citation64. Indeed, pathogen interference and inflammation such as ulcerative colitis and high-fat-induced obesity might disrupt the function of goblet cells, leading to mucin depletionCitation11,Citation65,Citation66. Since different mucin levels in culture media resulted in different bacterial metabolic profiles, it suggests that MD bacteria may provide endogenous and exogenous factors that can influence the complex structure of gut microbiotaCitation12,Citation67. Indeed, MD bacterial and probiotics abundance were significantly increased in ID diet compared to HFD diet (), and might affect iWAT, eWAT and mucus thickness (). Besides, IL−10 as the anti-inflammatory cytokine, which is important in maintaining intestinal homeostasis, was expressed higher in colon epithelial cells of ID-fed mice (Figure S11a). Furthermore, such physiological changes associated with nutrient-dependent MD bacterial metabolites, including bacterial muramyl dipeptides, can influence the pathogenesis of Crohn’s DiseaseCitation68. This idea is supported by our data showing that macrophages exposed to a culture supernatant obtained from A. muciniphila, following growth in complex medium without any mucin supplementation, produced higher levels of pro-inflammatory markers. By contrast, R. gnavus supernatants obtained after culture in mucin-containing media showed high pro-inflammatory attributes. R. gnavus showed low pro-inflammatory characteristics when it was grown in RDF, together with ILA derivative only produced in RDF. Intriguingly, R. gnavus, a pathobiont for IBDCitation35, has been reported as a predominant strain in the intestine of infants, along with Lactobacillus, Bifidobacterium, and Clostridium Citation69, hence the nature of Ruminococcus metabolites warrants further investigation. Nutrient-dependent variations in MD bacterial metabolite production can be regarded as bacterial adaptations to the host niche, but they can also influence host physiology, tissues, and intestinal homeostasis. Diet-induced variations in physiology and metabolism of MD bacteria can impact host health and disease status. In this regard, nutrient-dependent variations in SCFA profiles and cell membrane fatty acid compositions can activate tissue-resident macrophages to produce pro-inflammatory cytokines.
Previous studies have shown that dietary modifications can alter microbial composition and mucosal layer functionCitation9,Citation66,Citation70. The functional phenotype of MD bacteria can be reflected by host physiological and pathological conditions, such as mucin availability and inflammatory status. A. muciniphila can serve as a beneficial microbe in a homeostatic environment or mucin-rich environment, owing to its supporting metabolites. However, in a highly inflamed or mucin-depleted environment, A. muciniphila might degrade the remaining mucin in a more pathogenic manner, leading to the growth of pathobiontsCitation71. In contrast, the R. gnavus has a different functional phenotype, as it requires a more specific mucin composition to enhance its beneficial role. For example, a diet containing fucose, such as fucosyllactose, might reveal this phenomenonCitation72.
In conclusion, A. muciniphila plays a vital role in degrading mucin into its monomeric constituents, which are further metabolized, yielding propionate and acetate (). R. gnavus could utilize fucose as its primary carbon source to beneficially boost its growth and support the beneficial role of A. muciniphila. The reprogramming possibilities are complicated by variations in nutrient-specific MD capabilities. Thus, A. muciniphila cannot always be considered a beneficial microbe since it induces higher expression of pro-inflammatory markers on macrophages following growth in complex media lacking mucin. Similarly, a disease-associated organism such as R. gnavus may not be harmful to the host when it is present under certain environmental conditions. This research has revealed the context-dependency of MD bacteria, whose metabolism and host effects are altered by nutrient availability. Furthermore, in vivo models also showed histological, and gut microbiota including MD bacteria and commensal bacteria changes depending on dietary intakes. Thus, controlling microbial communities in the GI tract by influencing the abundance of MD bacteria and their metabolic functions via nutrient availability is a promising therapeutic strategy for rebalancing the colonic microbiota in many human diseases.
Figure 6. Proposed mechanism of metabolic features of MD bacteria. (a-b) Schematic diagram of the core metabolic pathways of A. muciniphila (a) and R. gnavus (b). The pathway that converts fucose into the core pathways is highlighted in red. Yellow- and purple-colored pathways represent the conversion of galactose and NeuAc into the core pathways, respectively. Blue- and green-colored pathways indicate the conversion of GlcNAc and GalNAc into the core pathways, respectively. Carbon sources described above share the orange-colored pathway for the production of acetate or propionate. (c) The schematic view shows the proposed MD bacterial adhesion and mucin-degradation mechanism, fermentation products, and host-microbe interactions.
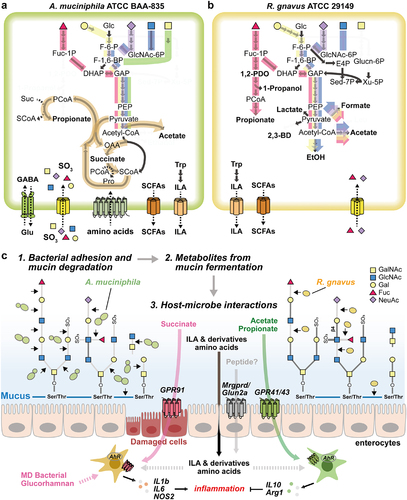
Materials and methods
Additional details are provided in the supplementary file
Bacterial strains and growth conditions
A. muciniphila MucT (ATCC BAA−835) and R. gnavus (ATCC 29149) were grown anaerobically in serum bottles sealed with butyl-rubber stoppers at 37°C. A. muciniphila was cultured in either complex medium, BHI or a minimal mediumCitation40 supplemented with type III hog gastric mucin (Sigma-Aldrich, St. Louis, USA) or various nutrients. For R. gnavus, cells were grown in either TSB as a complex medium, or minimal medium supplemented with mucin, glucose, n-acetyl glucosamine, galactose, or fucose.
High-performance liquid chromatography (HPLC)
Bacterial culture broths (1 ml) were centrifuged for fermentative metabolite analysis, and analyzed using an HPLC instrument (Waters 2695) equipped with an HPX−87 H column that performs separation with diluted sulfuric acid based on ion-exchange ligand-exchange chromatography at a column temperature of 47°C and an eluent flow of 0.45 ml/min with 5 mM sulfuric acid as previously describedCitation73.
Transcriptome and proteome analysis
Total RNA was isolated from bacterial cells using the RNeasy minikit (Qiagen GmbH, Hilden, Germany) and ribosomal RNA was removed using the NEBNext rRNA Depletion Kit (NEB) according to the manufacturer’s instructions. cDNA libraries were sequenced using the Illumina NovaSeq (Illumina, Inc., San Diego, CA, USA), and an average of 27.6 million reads per sample were obtained after quality trimming (See supplementary Materials and Methods for detailed procedures). Differential gene expression was assessed while taking into consideration gene length and sequencing coverage, by using Transcripts Per Million (TPM) reads data. Genes with a fold change ≥ 2 in normalized TPM and a P-value < 0.05 were considered differential genes. The biological functions and interactions of the differentially expressed genes were determined using gene pathway over-representation tests, and gene set enrichment analysis (GSEA)Citation74 was performed using the R packages clusterProfiler.
For proteomic analysis, the preparation of protein extracts and analysis of protein digests were performed by reversed-phase HPLC-ESI-MS/MS using a NanoLC−2D Ultra system (Eksigent, Dublin, CA, USA) coupled to a nano-ESI LTQ-XL mass spectrometer (Thermo Fisher Scientific, Bremen, Germany) as previously describedCitation40 (See supplementary Materials and Methods for detailed procedures). Data analysis was conducted using integrated tools in PeptideShaker. MS and MS/MS spectra were processed using MSConvert (ProteoWizard) to convert raw data into peak lists (.mgf format). Processed spectra were compared with the FASTA database of R. gnavus ATCC 29149 (UniProt Accession: UP000004410), using the target decoy database search strategy. A list of proteins and peptides was obtained, with a false discovery rate (FDR) of less than 0.01 (1%).
Electron microscope (EM) analysis
Bacterial cells grown on different media were analyzed by scanning electron microscopy (SEM) and transmission electron microscopy (TEM) (See supplementary Materials and Methods for detailed procedures). The SEM samples were covered with a layer of gold-palladium using a Carbon Coating Unit (EM ACE600, LEICA, Austria) and viewed with an FE-SEM (Merin, Carl ZEISS, Germany) at 2.0 kV in high-vacuum mode. In the case of the TEM, ultrathin sections were cut and stained. Bacterial cells were then observed with a JEOL, JEM−1011 transmission electron microscope, using an accelerating voltage of 80 kV.
Fatty acid analysis
Cellular fatty acids were saponified, methylated, and extracted from bacterial cells according to the protocol of MIDI/Hewlett Packard Microbial Identification SystemCitation75. The fatty acids were then analyzed by a Gas chromatograph (model 6890N; Agilent) equipped with a flame ionization detector (FID) (See supplementary Materials and Methods for detailed procedures).
LC-MS analysis of the extracellular metabolome
Bacterial cells were pelleted by centrifugation at 13,000 × g for 10 min, and the cell-free supernatant was collected and injected into an ultra-performance liquid chromatography/quadrupole time-of-flight mass spectrometer (UPLCQ/TOF-MS; Waters Corporation, Milford, MA, USA) (See supplementary Materials and Methods for detailed procedures). Briefly, the metabolites were separated using an Acquity UPLC BEH C18 column (2.1 × 100 mm, 1.7 µm particle size; Waters Corporation). Data processing including mass ion alignment and peak picking was performed using Progenesis QI software (Waters Corporation). The intensities of the mass peaks for each sample were applied to multivariate statistical analysis with Pareto scaling using the SIMCA-P + 12 software (Umetrics, San Jose, CA, USA).
Fecal DNA extraction and 16S rRNA amplicon sequencing
Fecal DNA was extracted using the QIAamp PowerFecal Pro DNA Kits (QIAGEN, Germany) according to the manufacturer’s instructions. For PCR, 12.5 ng of DNA from each sample was amplified by PCR with Illumina 16S metagenomic sequencing library preparation. V1-V3 16S rRNA region was amplified with the 27F and 534 R primers. Sequencing was performed using the Illumina Miseq platform with 2 × 300 bp paired-end reads. Trimmed forward and reverse sequences by fastp version 0.23.2Citation76 were concatenated by JTaxCitation77. An amplicon sequence variant table was generated by filtering, dereplicating, and denoising the sequences using DADA2Citation78. Reads were assigned taxonomy using SILVA version 138.1 within the tool QIIME 2 version 2022.2Citation79. DADA2 was used as a plugin within QIIME 2. Relative abundance and richness, including observed OTUs and Shannon index, were shown using the phyloseq, vegan, and ggplot2 R packages.
Growth rates and metabolic fluxes depending on the microbial community composition
Each model corresponding on the gut microbial communities of the HFD and ID groups in mice was constructed by matching the AGORA models version 1.03Citation80 at the family level. The growth rates and metabolic fluxes were predicted at a trade-off value of 0.3 using MICOMCitation47 and compared between the HFD and ID groups. Statistical analysis was performed using a paired t-test. Nutrient flux models for the high-fat and high-fiber diets were obtained from the MICOM media repository (https://github.com/micom-dev/media).
Treatment of RAW 264.7 cells with bacteria culture supernatant
The murine macrophage cell line, RAW 264.7 (KCLB), was cultured in Dulbecco’s modified eagle medium (DMEM) (Corning, USA) supplemented with 10% (v/v) fetal bovine serum (FBS; Gibco, United States) and 1% penicillin/streptomycin (Corning). Culture supernatants were obtained by sequential centrifugation of liquid culture broths of A. muciniphila and R. gnavus. Then, 7.5% (v/v) A. muciniphila-culture supernatant, R. gnavus-culture supernatant, or uncultured media in a total volume of 2 mL were added to the serum-free media, and the cells were cultured for 24 hours. A. muciniphila and R. gnavus were grown in different media and were heat-killed at 100°C for 30 minutes. Then, 3 × 108 CFU in 150 μL DPBS was incubated with RAW 264.7 macrophages in the serum-free media in a total volume of 2 mL and incubated for 24 hours. Cells were collected and resuspended in TRIZOL for RNA isolation. For quantitative Real-Time PCR, the primers are listed in Table S4. β-actin was used to normalize the CT value before the expression of genes was calculated as the relative mRNA expression. The results were representative of at least two independent experiments and were analyzed using a one-way ANOVA test.
Animal studies
Male C57BL/6 mice were purchased from Orient Bio Korea and housed in a specific pathogen-free animal facility. Experimental diets were obtained from DooYeol Biotech (Seoul, Korea). Seven-week-old mice from each group were fed either a high-fat diet containing 60% of fat or inulin-supplemented diet containing 10% inulin for 6 weeks. Fecal samples were collected bi-weekly for gut microbiota analysis. At the end of experiment, the colon and WAT were collected for further analysis. All animal experimental protocols were performed according to regulations and experimental procedures were approved by the Institutional Animal Care and Use Committee at Soonchunhyang University (SCH22–0009, SCH23–0005).
Histologic analysis of intestine
The mouse colons were fixed in Carnoy’s solution and embedded in Tissue-Tek OCT compound. OCT blocks were sectioned at 10 μm thickness and the slides were stained with Alcian Blue/Periodic acid-Schiff (AB/PAS) for mucus measurement. Mucin thickness was measured using ImageJ software (NIH, USA).
The isolation of intestinal epithelial cells
The colons were isolated and flushed with cold 1× PBS to remove luminal contents. Mesenteric fat tissues were removed, and the colon was vertically opened and cut into small pieces. The pieces were then transferred into pre-digestion buffer (1× Hank’s balanced salt solution without Ca, Mg, and phenol red) (Cellgro), 1 mM DTT (Sigma-Aldrich), 5 mM EDTA (Sigma-Aldrich), 10 mM HEPES (Gibco), and 2% FBS (Gibco). Samples were incubated at 37°C in a shaker for 20 min at 40 rpm. Intestinal epithelial cells were obtained by passing through a 70 μm cell strainer. The cells were washed with PBS and resuspended in Trizol™ for RNA isolation.
Author’s contributions
K.S. Kim, E. Tiffany, J.Y. Lee, A. Oh, H.S. Jin, Y.K. Lee, and D.W. Lee formulated the research plan. K.S. Kim, E. Tiffany, J.Y. Lee, A. Oh, H.S. Jin, J.S. Lee, and M.H. Nam performed the experiments. K.S. Kim, E. Tiffany, A. Oh, M.H. Nam, B.S. Kim, Y.K. Lee, and D.W. Lee analyzed the data. K.S. Kim, Y.K. Lee, and D.W. Lee wrote the manuscript. S.J. Hong, H. Koh, B.S. Kim, Y.K. Lee, and D.W. Lee conceived, planned, supervised, and managed the study.
Supplemental Material
Download MS Word (55.7 MB)Supplemental Material
Download Zip (8.3 MB)Disclosure statement
No potential conflict of interest was reported by the author(s).
Data availability statement
The complete genome sequence of R. gnavus ATCC 29149 has been deposited in NCBI/GenBank under the accession number CP027002. The RNA-seq data generated in this study have been submitted to the NCBI BioProject database (https://www.ncbi.nlm.nih.gov/bioproject/) under accession number PRJNA967152. Annotation data sets and multi-omics data are available in Datasets and Supplementary File. Detailed materials and methods are available in Supplementary File.
Supplementary material
Supplemental data for this article can be accessed online at https://doi.org/10.1080/19490976.2023.2221811
Correction Statement
This article has been corrected with minor changes. These changes do not impact the academic content of the article.
Additional information
Funding
References
- Fan Y, Pedersen O. Gut microbiota in human metabolic health and disease. Nat Rev Microbiol. 2021;19(1):55–23. doi:10.1038/s41579-020-0433-9.
- Hansson GC. Mucins and the microbiome. Ann Rev Biochem. 2020;89(1):769–793. doi:10.1146/annurev-biochem-011520-105053.
- Taherali F, Varum F, Basit AW. A slippery slope: on the origin, role and physiology of mucus. Adv Drug Deliv Rev. 2018;124:16–33. doi:10.1016/j.addr.2017.10.014.
- Medzhitov R. Recognition of microorganisms and activation of the immune response. Nature. 2007;449(7164):819–826. doi:10.1038/nature06246.
- Hall AB, Tolonen AC, Xavier RJ. Human genetic variation and the gut microbiome in disease. Nat Rev Genet. 2017;18(11):690–699. doi:10.1038/nrg.2017.63.
- Moreira Lopes TC, Mosser DM, Goncalves R. Macrophage polarization in intestinal inflammation and gut homeostasis. Inflamm Res. 2020;69(12):1163–1172. doi:10.1007/s00011-020-01398-y.
- Martino ME, Joncour P, Leenay R, Gervais H, Shah M, Hughes S, Gillet, B., Beisel, C, Leulier, F Bacterial adaptation to the host’s diet is a key evolutionary force shaping drosophila-Lactobacillus symbiosis. Cell Host & Microbe. 2018;24(1):109–19.e6. doi:10.1016/j.chom.2018.06.001.
- Tramontano M, Andrejev S, Pruteanu M, Klünemann M, Kuhn M, Galardini M, Jouhten P, Zelezniak A, Zeller G, Bork P, et al. Nutritional preferences of human gut bacteria reveal their metabolic idiosyncrasies. Nature Microbiol. 2018;3(4):514–522. doi:10.1038/s41564-018-0123-9.
- Desai MS, Seekatz AM, Koropatkin NM, Kamada N, Hickey CA, Wolter M, Pudlo NA, Kitamoto S, Terrapon N, Muller A, et al. A dietary fiber-deprived gut microbiota degrades the colonic mucus barrier and enhances pathogen susceptibility. Cell. 2016;167(5):1339–53 e21. doi:10.1016/j.cell.2016.10.043.
- Camilleri M. Leaky gut: mechanisms, measurement and clinical implications in humans. Gut. 2019;68(8):1516–1526. doi:10.1136/gutjnl-2019-318427.
- Litvak Y, Byndloss MX, Bäumler AJ. Colonocyte metabolism shapes the gut microbiota. Science. 2018;362(6418):eaat9076. doi:10.1126/science.aat9076.
- Byndloss MX, Baumler AJ. The germ-organ theory of non-communicable diseases. Nat Rev Microbiol. 2018;16(2):103–110. doi:10.1038/nrmicro.2017.158.
- Henao-Mejia J, Elinav E, Jin C, Hao L, Mehal WZ, Strowig T, Thaiss CA, Kau AL, Eisenbarth SC, Jurczak MJ, et al. Inflammasome-mediated dysbiosis regulates progression of NAFLD and obesity. Nature. 2012;482(7384):179–185. doi:10.1038/nature10809.
- Cani PD, Bibiloni R, Knauf C, Waget A, Neyrinck AM, Delzenne NM, Burcelin R. Changes in gut microbiota control metabolic endotoxemia-induced inflammation in high-fat diet–induced obesity and diabetes in mice. Diabetes. 2008;57(6):1470–1481. doi:10.2337/db07-1403.
- Trompette A, Gollwitzer ES, Yadava K, Sichelstiel AK, Sprenger N, Ngom-Bru C, Blanchard C, Junt T, Nicod LP, Harris NL, et al. Gut microbiota metabolism of dietary fiber influences allergic airway disease and hematopoiesis. Nat Med. 2014;20(2):159–166. doi:10.1038/nm.3444.
- Lee MJ, Kang MJ, Lee SY, Lee E, Kim K, Won S, Suh DI, Kim KW, Sheen YH, Ahn K, et al. Perturbations of gut microbiome genes in infants with atopic dermatitis according to feeding type. J Allergy Clin Immunol. 2018;141(4):1310–1319. doi:10.1016/j.jaci.2017.11.045.
- Yoshimoto S, Loo TM, Atarashi K, Kanda H, Sato S, Oyadomari S, Iwakura Y, Oshima K, Morita H, Hattori M, et al. Obesity-induced gut microbial metabolite promotes liver cancer through senescence secretome. Nature. 2013;499(7456):97–101. doi:10.1038/nature12347.
- Lloyd-Price J, Abu-Ali G, Huttenhower C. The healthy human microbiome. Genome Med. 2016;8(1):51. doi:10.1186/s13073-016-0307-y.
- Zmora N, Suez J, Elinav E. You are what you eat: diet, health and the gut microbiota. Nat Rev Gastroenterol Hepatol. 2019;16(1):35–56. doi:10.1038/s41575-018-0061-2.
- Pruss KM, Marcobal A, Southwick AM, Dahan D, Smits SA, Ferreyra JA, Higginbottom SK, Sonnenburg ED, Kashyap PC, Choudhury B, et al. Mucin-derived O-glycans supplemented to diet mitigate diverse microbiota perturbations. Isme J. 2021;15(2):577–591. doi:10.1038/s41396-020-00798-6.
- Lee J-E, Kim KS, Koh H, Lee D-W, Kang NJ. Diet-induced host–microbe interactions: personalized diet strategies for improving inflammatory bowel Disease. Curr Dev Nutr. 2022;6(8):nzac110. doi:10.1093/cdn/nzac110.
- Belzer C, Chia LW, Aalvink S, Chamlagain B, Piironen V, Knol J, de Vos WM. Microbial metabolic networks at the mucus layer lead to diet-independent butyrate and vitamin B12 production by intestinal symbionts. mBio. 2017;8(5). doi:10.1128/mBio.00770-17.
- Ranjbar R, Vahdati SN, Tavakoli S, Khodaie R, Behboudi H. Immunomodulatory roles of microbiota-derived short-chain fatty acids in bacterial infections. Biomed Pharmacother. 2021;141:111817. doi:10.1016/j.biopha.2021.111817.
- Derrien M, Veiga P. Rethinking diet to aid human–microbe symbiosis. Trends Microbiol. 2017;25(2):100–112. doi:10.1016/j.tim.2016.09.011.
- Trastoy B, Naegeli A, Anso I, Sjogren J, Guerin ME. Structural basis of mammalian mucin processing by the human gut O-glycopeptidase OgpA from Akkermansia muciniphila. Nat Commun. 2020;11(1):4844. doi:10.1038/s41467-020-18696-y.
- Collado MC, Derrien M, Isolauri E, de Vos WM, Salminen S, de Vos WM. Intestinal integrity and Akkermansia muciniphila, a mucin-degrading member of the intestinal microbiota present in infants, adults, and the elderly. Appl Environ Microbiol. 2007;73(23):7767–7770. doi:10.1128/AEM.01477-07.
- Depommier C, Van Hul M, Everard A, Delzenne NM, De Vos WM, Cani PD. Pasteurized Akkermansia muciniphila increases whole-body energy expenditure and fecal energy excretion in diet-induced obese mice. Gut Microbes. 2020;11(5):1231–1245. doi:10.1080/19490976.2020.1737307.
- Grander C, Grabherr F, Spadoni I, Enrich B, Oberhuber G, Rescigno M, Tilg H. The role of gut vascular barrier in experimental alcoholic liver disease and A. muciniphila supplementation. Gut Microbes. 2020;12(1):1851986. doi:10.1080/19490976.2020.1851986.
- Everard A, Belzer C, Geurts L, Ouwerkerk JP, Druart C, Bindels LB, Guiot Y, Derrien M, Muccioli GG, Delzenne NM, et al. Cross-talk between Akkermansia muciniphila and intestinal epithelium controls diet-induced obesity. Proc Natl Acad Sci U S A. 2013;110(22):9066–9071. doi:10.1073/pnas.1219451110.
- Roshanravan N, Mahdavi R, Alizadeh E, Ghavami A, Rahbar Saadat Y, Mesri Alamdari N, Alipour S, Dastouri MR, Ostadrahimi A. The effects of sodium butyrate and inulin supplementation on angiotensin signaling pathway via promotion of Akkermansia muciniphila abundance in type 2 diabetes; a randomized, double-blind, placebo-controlled trial. J Cardiovasc Thorac Res. 2017;9(4):183–190. doi:10.15171/jcvtr.2017.32.
- Lloyd-Price J, Arze C, Ananthakrishnan AN, Schirmer M, Avila-Pacheco J, Poon TW, Andrews E, Ajami NJ, Bonham KS, Brislawn CJ, et al. Multi-omics of the gut microbial ecosystem in inflammatory bowel diseases. Nature. 2019;569(7758):655–662. doi:10.1038/s41586-019-1237-9.
- Wang F, Cai K, Xiao Q, He L, Xie L, Liu Z. Akkermansia muciniphila administration exacerbated the development of colitis-associated colorectal cancer in mice. J Cancer. 2022;13(1):124–133. doi:10.7150/jca.63578.
- Osman MA, Neoh HM, Ab Mutalib NS, Chin SF, Mazlan L, Raja Ali RA, Zakaria AD, Ngiu CS, Ang MY, Jamal R. Parvimonas micra, Peptostreptococcus stomatis, Fusobacterium nucleatum and Akkermansia muciniphila as a four-bacteria biomarker panel of colorectal cancer. Sci Rep. 2021;11(1):2925. doi:10.1038/s41598-021-82465-0.
- Khedr EM, Omeran N, Karam-Allah Ramadan H, Ahmed GK, Abdelwarith AM. Alteration of gut microbiota in Alzheimer’s disease and their relation to the cognitive impairment. J Alzheimer’s Disease: JAD. 2022;88(3):1103–1114. doi:10.3233/JAD-220176.
- Png CW, Linden SK, Gilshenan KS, Zoetendal EG, McSweeney CS, Sly LI, McGuckin MA, Florin THJ. Mucolytic bacteria with increased prevalence in IBD mucosa augment in vitro utilization of mucin by other bacteria. Am J Gastroenterol. 2010;105(11):2420–2428. doi:10.1038/ajg.2010.281.
- Henke MT, Kenny DJ, Cassilly CD, Vlamakis H, Xavier RJ, Clardy J. Ruminococcus gnavus, a member of the human gut microbiome associated with Crohn’s disease, produces an inflammatory polysaccharide. Proc Natl Acad Sci U S A. 2019;116(26):12672–12677. doi:10.1073/pnas.1904099116.
- Gren C, Spiegelhauer MR, Rotbain EC, Ehmsen BK, Kampmann P, Andersen LP. Ruminococcus gnavus bacteraemia in a patient with multiple haematological malignancies. Access Microbiol. 2019;1(8):e000048. doi:10.1099/acmi.0.000048.
- Hall AB, Yassour M, Sauk J, Garner A, Jiang X, Arthur T, Lagoudas GK, Vatanen T, Fornelos N, Wilson R, et al. A novel Ruminococcus gnavus clade enriched in inflammatory bowel disease patients. Genome Med. 2017;9(1):103. doi:10.1186/s13073-017-0490-5.
- Dabard J, Bridonneau C, Phillipe C, Anglade P, Molle D, Nardi M, Ladiré M, Girardin H, Marcille F, Gomez A, et al. Ruminococcin A, a new lantibiotic produced by a Ruminococcus gnavus strain isolated from human feces. Appl Environ Microbiol. 2001;67(9):4111–4118. doi:10.1128/AEM.67.9.4111-4118.2001.
- Lee JY, Jin HS, Kim KS, Baek JH, Kim BS, Lee DW. Nutrient-specific proteomic analysis of the mucin degrading bacterium Akkermansia muciniphila. Proteomics. 2022;22(3):e2100125. doi:10.1002/pmic.202100125.
- Brown AJ, Goldsworthy SM, Barnes AA, Eilert MM, Tcheang L, Daniels D, Muir AI, Wigglesworth MJ, Kinghorn I, Fraser NJ, et al. The orphan G protein-coupled receptors GPR41 and GPR43 are activated by propionate and other short chain carboxylic acids. J Biol Chem. 2003;278(13):11312–11319. doi:10.1074/jbc.M211609200.
- Littlewood-Evans A, Sarret S, Apfel V, Loesle P, Dawson J, Zhang J, Muller A, Tigani B, Kneuer R, Patel S, et al. GPR91 senses extracellular succinate released from inflammatory macrophages and exacerbates rheumatoid arthritis. J Exp Med. 2016;213(9):1655–1662. doi:10.1084/jem.20160061.
- Liu Q, Sikand P, Ma C, Tang Z, Han L, Li Z, Sun S, LaMotte RH, Dong X. Mechanisms of Itch Evoked by β-Alanine. J Neurosci. 2012;32(42):14532. doi:10.1523/JNEUROSCI.3509-12.2012.
- Lockridge A, Gustafson E, Wong A, Miller RF, Alejandro EU. Acute D-serine co-agonism of β-cell NMDA receptors potentiates glucose-stimulated insulin secretion and excitatory β-cell membrane activity. Cells. 2021;10(1):10. doi:10.3390/cells10010093.
- Roager HM, Licht TR. Microbial tryptophan catabolites in health and disease. Nat Commun. 2018;9(1). doi:10.1038/s41467-018-05470-4.
- Ehrlich AM, Pacheco AR, Henrick BM, Taft D, Xu G, Huda MN, Mishchuk D, Goodson ML, Slupsky C, Barile D, et al. Indole-3-lactic acid associated with Bifidobacterium-dominated microbiota significantly decreases inflammation in intestinal epithelial cells. BMC Microbiol. 2020;20(1):357. doi:10.1186/s12866-020-02023-y.
- Diener C, Gibbons SM, Resendis-Antonio O, Chia N. MICOM: metagenome-scale modeling to infer metabolic interactions in the gut microbiota. mSystems. 2020;5(1). doi:10.1128/mSystems.00606-19.
- Liu Q, Lu W, Tian F, Zhao J, Zhang H, Hong K, Yu, L. Akkermansia muciniphila exerts strain-specific effects on DSS-induced ulcerative colitis in mice. Front Cell Infect Microbiol. 2021;11:698914. doi:10.3389/fcimb.2021.698914.
- Seregin SS, Golovchenko N, Schaf B, Chen J, Pudlo NA, Mitchell J, Baxter NT, Zhao L, Schloss PD, Martens EC, et al. NLRP6 protects Il10 mice from colitis by limiting colonization of Akkermansia muciniphila. Cell Rep. 2017;19(4):733–745. doi:10.1016/j.celrep.2017.03.080.
- Willdigg JR, Helmann JD. Bacterial membrane composition and its modulation in response to stress. Front Mol Biosci. 2021;8:634438. doi:10.3389/fmolb.2021.634438.
- Van Teeseling MCF, de Pedro MA, Cava F, van Teeseling MCF, de Pedro MA. Determinants of bacterial morphology: from fundamentals to possibilities for antimicrobial targeting. Front Microbiol. 2017;8:1264. doi:10.3389/fmicb.2017.01264.
- Lapujade P, Cocaign-Bousquet M, Loubiere P. Glutamate biosynthesis in Lactococcus lactis subsp. lactis NCDO 2118. Appl Environ Microbiol. 1998;64(7):2485–2489. doi:10.1128/AEM.64.7.2485-2489.1998.
- Huang L, Huang L, Zhao L, Qin Y, Su Y, Yan Q. The regulation of oxidative phosphorylation pathway on Vibrio alginolyticus adhesion under adversities. Microbiologyopen. 2019;8(8):e00805. doi:10.1002/mbo3.805.
- Meng D, Sommella E, Salviati E, Campiglia P, Ganguli K, Djebali K, Zhu W, Walker WA. Indole-3-lactic acid, a metabolite of tryptophan, secreted by Bifidobacterium longum subspecies infantis is anti-inflammatory in the immature intestine. Pediatr Res. 2020;88(2):209–217. doi:10.1038/s41390-019-0740-x.
- Mitchell MK, Ellermann M. Long chain fatty acids and virulence repression in intestinal bacterial pathogens. Front Cell Infect Microbiol. 2022;12:928503. doi:10.3389/fcimb.2022.928503.
- Crouch LI, Liberato MV, Urbanowicz PA, Baslé A, Lamb CA, Stewart CJ, Cooke K, Doona M, Needham S, Brady RR, et al. Prominent members of the human gut microbiota express endo-acting O-glycanases to initiate mucin breakdown. Nat Commun. 2020;11(1):4017. doi:10.1038/s41467-020-17847-5.
- Ang Z, Ding JL. GPR41 and GPR43 in obesity and inflammation -protective or causative? Front Immunol. 2016;7:28–. doi:10.3389/fimmu.2016.00028.
- Mills E, O’Neill LA. Succinate: a metabolic signal in inflammation. Trends Cell Biol. 2014;24(5):313–320. doi:10.1016/j.tcb.2013.11.008.
- Murty VL, Piotrowski J, Czajkowski A, Slomiany A, Slomiany BL. Inhibition of Helicobacter pylori glycosulfatase activity towards human gastric sulfomucin by a gastroprotective agent, sulglycotide. Gen Pharmacol. 1993;24(6):1463–1466. doi:10.1016/0306-3623(93)90436-2.
- Bian X, Wu W, Yang L, Lv L, Wang Q, Li Y, Ye J, Fang D, Wu J, Jiang X, et al. Administration of Akkermansia muciniphila ameliorates dextran sulfate sodium-induced ulcerative colitis in mice. Front Microbiol. 2019;10:2259. doi:10.3389/fmicb.2019.02259.
- Zhou Q, Zhang Y, Wang X, Yang R, Zhu X, Zhang Y, Chen C, Yuan H, Yang Z, Sun L, et al. Gut bacteria Akkermansia is associated with reduced risk of obesity: evidence from the American Gut Project. Nutr Metab (Lond). 2020;17(1):90. doi:10.1186/s12986-020-00516-1.
- Gu Z, Pei W, Shen Y, Wang L, Zhu J, Zhang Y, Fan S, Wu Q, Li L, Zhang Z, et al. Akkermansia muciniphila and its outer protein Amuc_1100 regulates tryptophan metabolism in colitis. Food Funct. 2021;12(20):10184–10195. doi:10.1039/D1FO02172A.
- Powell DN, Swimm A, Sonowal R, Bretin A, Gewirtz AT, Jones RM, Kalman D. Indoles from the commensal microbiota act via the AHR and IL-10 to tune the cellular composition of the colonic epithelium during aging. Proc Natl Acad Sci USA. 2020;117(35):21519–21526. doi:10.1073/pnas.2003004117.
- Ganesh BP, Klopfleisch R, Loh G, Blaut M, Ryffel B. Commensal Akkermansia muciniphila exacerbates gut inflammation in Salmonella Typhimurium-infected gnotobiotic mice. PLos One. 2013;8(9):e74963. doi:10.1371/journal.pone.0074963.
- McCormick DA, Horton LW, Mee AS. Mucin depletion in inflammatory bowel disease. J Clin Pathol. 1990;43(2):143–146. doi:10.1136/jcp.43.2.143.
- Zou J, Chassaing B, Singh V, Pellizzon M, Ricci M, Fythe MD, Kumar MV, Gewirtz AT. Fiber-mediated nourishment of gut microbiota protects against diet-induced obesity by restoring IL-22-mediated colonic health. Cell Host & Microbe. 2018;23(1):41–53 e4. doi:10.1016/j.chom.2017.11.003.
- Gilbert JA, Blaser MJ, Caporaso JG, Jansson JK, Lynch SV, Knight R. Current understanding of the human microbiome. Nat Med. 2018;24(4):392–400. doi:10.1038/nm.4517.
- Eckmann L, Karin M. NOD2 and Crohn’s disease: loss or gain of function? Immunity. 2005;22(6):661–667. doi:10.1016/j.immuni.2005.06.004.
- Hattori M, Taylor TD. The human intestinal microbiome: a new frontier of human biology. DNA Res. 2009;16(1):1–12. doi:10.1093/dnares/dsn033.
- Schroeder BO, Birchenough GMH, Stahlman M, Arike L, Johansson MEV, Hansson GC, Bäckhed F. Bifidobacteria or fiber protects against diet-induced microbiota-mediated colonic mucus deterioration. Cell Host & Microbe. 2018;23(1):27–40 e7. doi:10.1016/j.chom.2017.11.004.
- Sugihara K, Kitamoto S, Saraithong P, Nagao-Kitamoto H, Hoostal M, McCarthy C, Rosevelt A, Muraleedharan CK, Gillilland MG, Imai J, et al. Mucolytic bacteria license pathobionts to acquire host-derived nutrients during dietary nutrient restriction. Cell Rep. 2022;40(3):111093. doi:10.1016/j.celrep.2022.111093.
- Grabinger T, Glaus Garzon JF, Hausmann M, Geirnaert A, Lacroix C, Hennet T. Alleviation of intestinal inflammation by oral supplementation with 2-fucosyllactose in mice. Front Microbiol. 2019;10:1385. doi:10.3389/fmicb.2019.01385.
- Kim HJ, Hou BK, Lee SG, Kim JS, Lee DW, Lee SJ. Genome-wide analysis of redox reactions reveals metabolic engineering targets for D-lactate overproduction in Escherichia coli. Metab Eng. 2013;18:44–52. doi:10.1016/j.ymben.2013.03.004.
- Subramanian A, Tamayo P, Mootha VK, Mukherjee S, Ebert BL, Gillette MA, Paulovich A, Pomeroy SL, Golub TR, Lander ES, et al. Gene set enrichment analysis: a knowledge-based approach for interpreting genome-wide expression profiles. Proc Natl Acad Sci USA. 2005;102(43):15545–15550. doi:10.1073/pnas.0506580102.
- Sasser M. Identification of bacteria by gas chromatography of cellular fatty acids. Newark, DE, USA: MIDI Technical Note 101. MIDI Inc; 1990.
- Chen S, Zhou Y, Chen Y, Gu J. Fastp: an ultra-fast all-in-one FASTQ preprocessor. Bioinformatics. 2018;34(17):i884–i90. doi:10.1093/bioinformatics/bty560.
- Liu T, Chen CY, Chen-Deng A, Chen YL, Wang JY, Hou YI, Lin M-C. Joining Illumina paired-end reads for classifying phylogenetic marker sequences. BMC Bioinform. 2020;21(1):105. doi:10.1186/s12859-020-3445-6.
- Callahan BJ, McMurdie PJ, Rosen MJ, Han AW, Johnson AJ, Holmes SP. DADA2: high-resolution sample inference from Illumina amplicon data. Nat Methods. 2016;13(7):581–583. doi:10.1038/nmeth.3869.
- Bolyen E, Rideout JR, Dillon MR, Bokulich NA, Abnet CC, Al-Ghalith GA, Alexander H, Alm EJ, Arumugam M, Asnicar F, et al. Reproducible, interactive, scalable and extensible microbiome data science using QIIME 2. Nat Biotechnol. 2019;37(8):852–857. doi:10.1038/s41587-019-0209-9.
- Magnusdottir S, Heinken A, Kutt L, Ravcheev DA, Bauer E, Noronha A, Greenhalgh K, Jäger C, Baginska J, Wilmes P, et al. Generation of genome-scale metabolic reconstructions for 773 members of the human gut microbiota. Nat Biotechnol. 2017;35(1):81–89. doi:10.1038/nbt.3703.