ABSTRACT
Resistance to antibiotics in newborns is a huge concern as their immune system is still developing, and infections and resistance acquisition in early life have short- and long-term consequences for their health. Bifidobacterium species are important commensals capable of dominating the infant gut microbiome and are known to be less prone to possess antimicrobial resistance genes than other taxa that may colonize infants. We aimed to study the association between Bifidobacterium-dominated infant gut microbiota and the antibiotic resistant gene load in neonates, and to ascertain the perinatal factors that may contribute to the antibiotic resistance acquisition. Two hundred infant fecal samples at 7 days and 1 month of age from the MAMI birth cohort were included in the study and for whom maternal-neonatal clinical records were available. Microbiota profiling was carried out by 16S rRNA amplicon sequencing, and targeted antibiotic resistance genes (ARGs) including tetM, tetW, tetO, blaTEM, blaSHV and ermB were quantified by qPCR. Infant microbiota clustered into two distinct groups according to their Bifidobacterium genus abundance: high and low. The main separation of groups or clusters at each time point was performed with an unsupervised non-linear algorithm of k-means partitioning to cluster data by time points based on Bifidobacterium genus relative abundance. Microbiota composition differed significantly between both groups, and specific bifidobacterial species were enriched in each cluster. Lower abundance of Bifidobacterium in the infant gut was associated with a higher load of antibiotic resistance genes. Our results highlight the relevance of Bifidobacterium genus in the early acquisition and establishment of antibiotic resistance in the gut. Further studies are needed to develop strategies to promote a healthy early colonization and fight against the spread of antibiotic resistances.
Introduction
Antibiotic resistance in infants is a growing concern. Early exposure to antibiotics can lead to the development of antibiotic-resistant bacteria and disrupt the delicate balance of the gut microbiome, having consequences for future infant health, immune development and metabolism. In the early stages of life, antibiotic acquired resistance can result from the horizontal transfer of genes between bacteria, vertical transmission from the mother at birth or during lactation, and/or antibiotic exposure of the infant.Citation1
The gut microbiota composition is the main source of most antibiotic resistant genes (ARGs) that are carried by a limited number of taxa. Members of the family Enterobacteriaceae, such as Escherichia, Klebsiella, Citrobacter, and Enterobacter, responsible for many perinatal infections, contribute the most abundant and unique ARGs in the infant gut.Citation2 Bifidobacterium are negatively correlated with antibiotic resistance load;Citation3 these species are important commensals capable of dominating the gut microbiome of breastfed infants, in part because of their capacity to use human milk oligosaccharides (HMOs) and other carbohydrates efficiently.Citation4,Citation5 Bifidobacterium species are also less likely to possess ARGs than other taxa such as Enterococcus, Streptococcus, Staphylococcus, and Bacteroides genus.Citation6,Citation7 It has also been reported that the resistance genes detected in anaerobes (such us Bifidobacterium or Lactobacillus) are distinct from the ARGs found in pathogenic bacteria that grow aerobically,Citation8,Citation9 which suggest that horizontal gene transfer between commensals and pathogens is more unlikely than the transfer of ARGs between pathogens.Citation10,Citation11
The infant gut microbiota changes significantly during the first days and weeks of life, with external factors, such as breastfeeding, mode of delivery, antibiotic exposure, and diet, exerting notable influences on the composition and diversity of the infant gut microbiota, which in turn affects the resistome.Citation12 Understanding these influences is crucial for developing interventions and strategies aimed at promoting a healthy gut microbiota and mitigating the risks associated with the emergence and spread of antibiotic resistance in infants. Our objective was to study the influence of the presence of Bifidobacterium spp. on the early gut microbiota and the acquisition of antibiotic resistance gene load in neonates from 7 days to 1-month old. Further, we examined which perinatal factors may contribute to the initial microbiota composition and antibiotic resistance acquisition.
Materials and methods
Study design and volunteers
A total of 200 infant fecal samples collected during the first month of life of healthy term (37 weeks or more) infants from the MAMI birth cohort,Citation13 recruited during 2015–2017, were included in this observational study (Supplementary figure S1). Maternal-infant clinical records including body mass index (BMI), gestational age, neonate gender, birth weight, mode of delivery, type of lactation (maternal lactation, referring to breastfeeding; mixed lactation, referring to combination of both breastfeeding and formula-feeding; and exclusive formula-feeding) and antibiotic exposure of the infant were collected. All participants received oral and written information about the study, and written consent was obtained. The study was approved by Ethical Committee of Hospital Clínico Universitario de Valencia and CSIC. The trial was registered on the ClinicalTrial.gov platform (number NCT03552939).
Neonatal biological samples and DNA extraction and quantification
Samples were collected at two time points (7 days and 1 month after birth) in sterile containers by the parents at home following detailed instructions. They were immediately stored at −20°C, transported within 24 h to primary health centers and stored at −80°C until analysis. Sample management and processing, as well as Total DNA was extraction, purification, and concentration were performed as described previously.Citation14
Quantification of antibiotic resistance genes and Bifidobacterium spp. By targeted qPCR
To analyze the antibiotic resistance gene load in infants’ gut, a subset of samples from each time point (7 days: n = 71, 1 month: n = 66) was used, and the presence and abundances (log copies/µl) of each ARG were compared between the two time points and the two Bifidobacterium relative abundance clusters. Quantifications of the total number of copies of genes conferring resistance to tetracycline (tetM, tetO and tetW), beta-lactam (blaTEM and blaSHV) and erythromycin (ermB) were performed using described primers (Supplementary Table S1) by qPCR in a LightCycler 480 real-time PCR system (Roche Technologies, Basel, Switzerland). The quantified ARGs were chosen according to their importance and high prevalence in infant resistome as described elsewhere.Citation2,Citation3,Citation15–18 Each reaction mixture of 10 μL was composed of 5 μL SYBR GreenPCR Master Mix (Roche Technologies, Basel, Switzerland), 0.25 μL of each of the primers at a concentration of 10 μM, 3.5 μL of distilled water and 1 μL of DNA. The amplification conditions were 35 cycles of: initial denaturation at 95°C for 5 min denaturation at 95°C for 30 s, specific annealing temperature for each primer pairCitation19 for 30 s, and a final extension step of 72°C for 30 s. The qPCR results were calculated as the antibiotic resistance gene copies per sample (log copies/µl). Bifidobacterium genus total counts were also determined by targeted quantitative PCR by the same quantitative system based on the amplification of specific 16S rRNA gene region. Melting curves were also assessed to test the specificity of the reaction. For all qPCR measurements, standard curves for the specific targeted gene were generated using Ct values and the calculated gene copy numbers were determined based on the fragment amplification length.
Gut microbiota profiling by specific 16S rRNA amplicon sequencing
The microbiota characterization was performed by amplification of the V3-V4 regions of the 16S rRNA. Amplicons were obtained with PCR amplification using barcoded conventional primers (341F 5′-CCTACGGGNGGCWGCAG-3′ and 806 R 5′GGACTACNNGGGTATCTAAT-3′) with a 466bp fragment length. Amplicons were checked with a Bioanalyzer DNA 1000 chip and libraries were sequenced using a 2 × 250 bp paired-end kit on a MiSeq-Illumina platform (FISABIO sequencing service, Valencia, Spain). Bacterial diversity analysis was done using raw reads, which were quality controlled and filtered (Quality; 25 and length; 125 bp) using TrimGalore (v0.6.4_dev; https://github.com/FelixKrueger/TrimGalore).
The paired-end reads with a minimum overlap of 30 bp were joined using Fastq-join.Citation20 Sequences were trimmed of primers and distal bases, and singletons were removed with USEARCH v11.Citation21 zOTUs (zero-radius operational taxon units) mapping to the human genome (GRCh38) using the Burrow – Wheeler Aligner in Deconseq v0.4.3 were filtered out. The resulting reads were denoised, and chimeras were filtered with UNOISE3.Citation22 Taxonomic assignment of zOTUs was performed in QIIME 2 v2018.2Citation23 using the QIIME 2 feature classifier pluginCitation24 and the Ribosomal Database Project (RDP).Citation25 The zOTUs were aligned with MAFFTCitation26 to then make a phylogenetic tree with FASTTREECitation27 that was midpoint rooted. The Bayesian LCA-based Taxonomic Classification Method (BLCA)Citation28 was used to assign zOTUs to species that were only assigned to Bifidobacterium genus with QIIME 2 (assignation of 80% confidence). The database used was a custom database, taking the reference sequences of all Bifidobacterium species from the SILVA database.Citation29 We filtered the zOTU data using filter_taxa function of “phyloseq” package, and only zOTUs present in at least 10% of samples were retained.
Bioinformatic and statistical analyses
All statistical analyses were performed with R version 3.6.0, and figures were drawn with the “ggplot2” R package.Citation30 Categorical variables are expressed as positive cases-prevalence and (percentage, %). Normally distributed data are presented as mean ± standard deviation (SD) and non-normal data as median and interquartile range [IQR]. Pearson’s-Chi-square test was used for categorical variables, and Mann–Whitnney U test or Fisher’s exact test were used for continuous variables, as appropriate, for calculating statistical significance. Statistical analyses were performed equally for both paired and unpaired data points, as the majority of the population was paired in both time points, and confirmation analyses confirmed there were no differences (data not shown).
The main separation of groups or clusters at each time point was performed with an unsupervised non-linear algorithm of k-means partitioning to cluster data by time points based on Bifidobacterium genus relative abundance. The elbow method and average silhouette method were performed using in the “factoextra” R packageCitation31 were used to determine the optimal number of clusters and k-means clustering of the data was performed with the “stats” R core package. Optimal centers of k-means were established as 2 clusters, which corresponded with high and low Bifidobacterium relative abundance (Supplementary figure S2).
Venn diagrams were generated to assess the core and unique microbiota, at the zOTU level, for the two clusters at each time point separately, using the R package “VennDiagram”.Citation32 Calculations of richness (Observed, Chao1 and ACE) and evenness indices (Shannon and Simpson) were done using the “phyloseq” R package.Citation33
Beta diversity was characterized by Principal Coordinate Analysis (PCoA) conducted by plotting the Bray-Curtis distance matrix of log transformed zOTU counts for each time point separately. The Adonis permutational test was used to evaluate overall differences in microbiota structure between the k-mean-categorized groups with the “vegan” R package.Citation34 The same analysis was performed according to the mode of delivery and antibiotic exposure of the study participants. A Mann – Whitney U test in the “rstatix” R packageCitation35 was performed on the log transformed abundances of genera to identify significantly different genera between the two clusters. Phylogenetic trees based on zOTUs assigned to Bifidobacterium genus were constructed using “plot_tree” function of “phyloseq” package.
Multivariate Redundancy Analysis (RDA) was conducted to confirm the correlation between ARGs and microbiota with respect to the clusters of Bifidobacterim abundance. Linear discriminant analysis effect size (LEfSe) was used to identify microbial genera significatively enriched in each cluster. An LDA score (log10) > 3 was considered significant. Spearman rank correlation coefficients were estimated to determine the linear association between the 20 top genera and the number of copies of ARGs.
The effect size and significance of each covariate were determined using the ‘envfit’ function in the “vegan” package comparing the difference in the centroids of each group relative to the total variation. Ordination was performed using NMDS based on Bray – Curtis dissimilarity. The significance value was determined based on 999 permutations. The squared correlation coefficient (R2) for each variable was calculated and scaled as a vector. In total, 6 covariates with known associations to gut microbiome development in neonates, infants, and children were included in the ‘envfit’ analysis and the grouping used within each variable is presented in . Specifically, we tested hierarchical clustering based on k-means according to Bifidobacterium relative abundance (high or low), mode of delivery, mode of lactation, antibiotic exposure during pregnancy, antibiotic exposure at birth, and direct antibiotic exposure of the infants. MaAsLin2Citation36 was used to build general linear models for longitudinal data to efficiently determine the multivariate association between factors and taxonomy in both clusters while accounting for potentially confounding covariates. MaAsLin2 relies on general linear models to accommodate most modern epidemiological study designs, including cross-sectional and longitudinal. In this case, the analysis with MaAsLin2 was a longitudinal analysis, so the first added variable was Time (7 days and 1 month). The following variables were added depending on the strength of each variable seen in the other analyses, so we added “kmeans_ab02” (Low vs Hight), “Delivery_mode” (C-section vs vaginal) and “Lactation” (maternal vs. mixed vs. formula). No more variable groups or other variables were added. The command runs MaAsLin2 on the data, running a multivariable regression model to test for the association between microbial species abundance versus Time, kmeans_ab02, Delivery_mode and Lactation. Finally, the input data were transformed to log format.
Table 1. Characteristics of study participants.
For all methods, p-values were adjusted for multiple comparisons using False Discovery Rate (FDR) based on Benjamini – Hochberg (BH).Citation37 Normality of the data was evaluated with Shapiro – Wilk tests.
Results
Bifidobacterium dominance associates with a specific microbial composition
Hierarchical clustering revealed two significantly distinct (p < .001) microbial clusters at each time point, characterized by a high (54.38% for 7 days; 61.72% for 1 month) and low (11.26% for 7 days; 17.08% for 1 month) relative abundance of Bifidobacterium members (). 71.43% of infants maintained this classification according to Bifidobacterium relative abundance through time (from 7 days to 1 months).
Figure 1. Principal coordinates analysis of the hierarchical clustering of Bifidobacterium relative abundance of the infants at (a) 7 days and (b) 1 month of age based on Bray Curtis distances regarding the microbial community composition (p < .001; ADONIS pairwise test). Samples are grouped by hierarchical clustering of Bifidobacterium relative abundance in intestinal microbiota. Orange ellipses represent the high-Bifidobacterium groups and blue ellipses represent low-Bifidobacterium groups. Supplementary figure 2 provides the optimal number of clusters found by the elbow and average silhouette methods of K-means partitioning clustering algorithm.
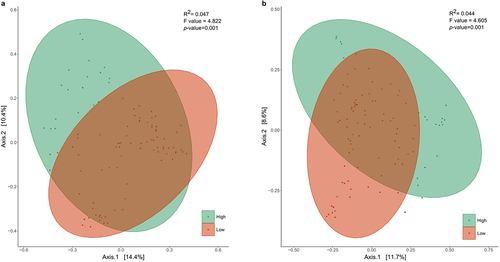
Clinical characteristics of the participating infants and their respective mothers at both time points according to Bifidobacterium cluster are shown in . Gender was distributed similarly between the two clusters. Regarding the mode of delivery, vaginal-born infants corresponded to 90.47% and 81.39% of the neonates clustered as high-Bifidobacterium at 7 days and 1 month, respectively. Most of the infants participating in the study were breastfed and no differences with other breastfeeding practices were found between clusters. Finally, antibiotic exposure was recorded during the gestation period until 1 month of age. 42.10% of infants clustered as low-Bifidobacterium at 7 days of age were exposed to antibiotics at delivery, significantly more than the high-Bifidobacterium infants (p = .0008). According to maternal characteristics, no statistical differences were found according to the Bifidobacterium cluster.
To assess if confounding variables might influence clustering, beta diversity was also compared across other metadata variables, separating the infants by their mode of delivery in combination with the two different clusters, corresponding to the Bifidobacterium relative abundance (Supplementary table S2). For both time points, vaginal-born infants clustered in two groups according to the Bifidobacterium relative abundance (Vaginal_High and Vaginal_Low) with significantly different bacterial communities (p = .006). The microbiota of c-section_Low infants was significantly different from Vaginal_Low and Vaginal_High infants (p < .05), both at 7 days and 1 month of age. Finally, in 1-month-old infants, C-section_High infants clustered separately from Vaginal_Low infants (p = .036). No differences between c-section-High and c-section Low were found at either time point.
The impact of antibiotic exposure microbial composition in the context of Bifidobacterium clustering was also examined (Supplementary table S3). Both high and low- Bifidobacterium groups of infants that did not receive antibiotics had different gut microbial compositions (p = .012 and p = .006 for 7 days and 1 month of age). Infants with a low abundance of Bifidobacterium have a significantly different microbial composition at 7 days of age depending on if they have received antibiotics or not (p =.012), which is not observed in high-Bifidobacterium cluster.
Microbiota is shaped by Bifidobacterium spp. abundance
In both time points, the percentage of the core zOTUs was 35.1%, while 48.8% (7 days) and 50.5% (1 month) are unique for the Low cluster and 16.1% (7 days) and 14.1% (1 month) were unique for the High cluster, signifying that the two groups, harbor different inhabitant niches, independent of time.
Infants in the High cluster showed lower but not statistically significant microbial diversity and richness at 7 days and 1 month of age (Supplementary table S4). The observed diversity of Bifidobacterium species between the two clusters at the two sampling times was significantly different (p < .001 for both times): the high-Bifidobacterium group had more diversity of Bifidobacterium spp. than the low-Bifidobacterium group (Supplementary figure S3).
Differences in relative abundance of the 8 most abundant genera (more than 5% of relative abundance) were calculated according to zOTUs counts ( and Supplementary tables S5 and S6). Bifidobacterium relative abundance was 54.38% and 61.72% in the High-clustered samples of 7 days and 1 month respectively, and 11.26% and 17.08% of the Low-clustered samples. Bifidobacterium genus was quantified in 7 days old infant samples at 6.93 (3.71) log copies/µL (median (IQR)) for Low cluster and 7.71 (7.03) log copies/µL (median (IQR)) for High cluster. For 1-month samples, 7.08 (4.53) and 7.53 (6.14) log copies/µL (median (IQR)) were quantified in Low and High groups, respectively.
Figure 2. Microbial composition according to Bifidobacterium-abundance groups. Differences in relative abundance (%) of the 8 most abundant genera in fecal microbiota (more than 5% of relative abundance) among the first 7 days (a) and 1 month of life (b). Asterisks (*) indicate a significant difference between groups based on Mann–Withney U of the log-transformed relative abundances. Supplementary tables 5 and 6 provide genus abundances and statistical analyses of the groups for the two time points. Linear discriminant analysis (LDA) Effect Size (LEfSE) plot of taxonomic biomarkers identified in the gut microbiota of infants of 7 days (c) and 1 month (d) of age, according to Bifidobacterium dominance cluster (High and Low colored in green and orange, respectively). The threshold for the logarithmic discriminant analysis (LDA) score was 3 and p < .05 for Wilcoxon test was considered significant.
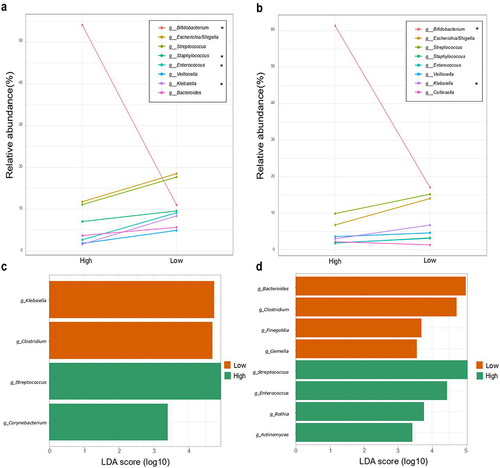
In 7 days old infants, Streptococcus, and Escherichia/Shigella spp. predominated in the low-Bifidobacterium group, appearing in higher relative abundances than the Bifidobacterium species, although no statistical differences were found between the two groups. Seven-day-old infants in the low-Bifidobacterium group had a significantly higher relative abundance of Staphylococcus (p < .01), Enterococcus (p < .05), and Klebsiella (p < .05), compared to the high-Bifidobacterium group. Veillonella and Bacteroides showed the same pattern but without statistical differences. On the other hand, low-Bifidobacterium 1-month-old infants had also higher relative abundance of Klebsiella (p < .05), whereas Collinsella appeared in higher proportions in the high-Bifidobacterium group, though differences between groups were not statistically significant. Similar to 7-day-old infants, Staphylococcus, Enterococcus, and Veillonella appeared in higher abundances in the low-Bifidobacterium group, but with no statistically significant difference compared to the high-Bifidobacterium group. Tendency analysis of the microbiota also showed that Bacteroides spp. was more abundant in high-Bifidobacterium infant than in low-Bifidobacterium infants at 1 month of age (p < .001) (Supplementary table S5 and S6).
LEfSe, performed on samples from 7-day-old infants, showed that Klebsiella (p = .031) and Clostridium (p = .0036) were significant characterized in the Low cluster and Streptococcus (p = .024), and Corynebacterium (p = .0378) characterized the High cluster. For the 1-month-old infant samples, Streptococcus (p = .011), Enterococcus (p = .042), Rothia (p = .038) and Actinomyces (p = .021) were enriched in the High cluster, and Bacteroides (p = .0009), Finegoldia (p = .009) and Gemella (p = .045) were enriched in the Low cluster ().
The Bayesian LCA-based Taxonomic Classification Method for Bifidobacterium spp. showed that, in both time points, Bifidobacterium longum (High: 46.96–50.72%; Low: 46.09–56.24%, for 7 days and 1 month, respectively) was the most abundant species of Bifidobacterium in infants (). Relative abundance of B. dentium (High: 3.95–10.66%; Low: 13.35–14.48%) was significantly more abundant in 1-month-old infants in the low-Bifidobacterium group according to LEfSE analysis (), whereas an unclassified species of Bifidobacterium was enriched in the high-Bifidobacterium group. This species, together with B. breve, appeared in higher amounts in the high-Bifidobacterium cluster of infants in both time points, but without statistical significance. On the other hand, B. adolescentis, B. animalis and B. scardovii were more abundant in low-Bifidobacterium infants of both ages, but no significant differences were found. The phylogenetic tree of Bifidobacterium species confirmed that, in both time points, there were unique zOTUs assigned for specific Bifidobacterium spp. (Supplementary figure S3).
Figure 3. Bifidobacterium spp. relative abundance according to Bifidobacterium relative abundance group. Differences in relative abundance (%) of species of Bifidobacterium identified in fecal microbiota among the (a) first 7 days and (b) 1 month of life. (c) Linear discriminant analysis (LDA) Effect Size (LEfSE) plot of Bifidobacterium species identified in the gut microbiome of 1-month-old infants and their relative abundances. Species bacterial traits found at Bifidobacterium species level according to Bifidobacterium dominance cluster (High and Low coloured in green and orange, respectively). The threshold for the logarithmic discriminant analysis (LDA) score was 3 and p < .05 for Wilcoxon test was considered significant.
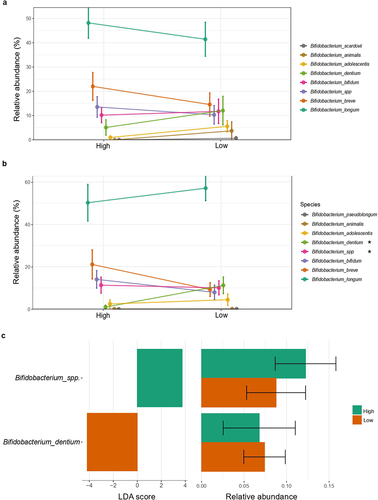
Influence of environmental factors on Bifidobacterium relative abundance and specific microbial associations
Analysis with ‘envfit’ confirmed that the high-low Bifidobacterium relative abundance clustering, based on k-means hierarchical clustering method, is the best approach to explain the dissimilarities in gut microbial composition according to Bifidobacterium relative abundance, as it was the factor that explained the largest amount of variance in both time points (R2 = 0.316, p < .001 at 7 days; R2 = 0.275, p < .001 at 1 month) (). In 7-day-old infants, antibiotic exposure at the moment of birth (R2 = 0.163, p < .001) was the second most important categorical factor, followed by mode of delivery (R2 = 0.161, p < .001). C-section procedures imply the use of antibiotics during delivery, so it is expected than both variables have similar contributions. Accordingly, vaginal-delivery and absence of antibiotic exposure at birth were associated with the high-Bifidobacterium group (). Overall antibiotic exposure during the first 7 days of life (including moment of birth till 7 days of age) was the next most important factor (R2 = 0.117, p < .001), followed by mode of lactation, which was not statistically significant (R2 = 0.045, p > .05), and the use of antibiotics by the mother during pregnancy (R2 = 0.033, p < .05).
Figure 4. Environmental factors contributing to Bifidobacterium-specific microbiota. Significance and explained variance of 6 microbiota covariates modeled by ‘envfit’ across all data types for each time point: for (a) 7 days old and for (c) 1 month old. Horizontal bars show the amount of variance (R2) explained by each covariate in the model as determined by ‘envfit’. The groups within each covariate are detailed in Table 1. Covariates are gradient-colored based on R2. Significant covariates (false discovery rate (FDR) p < .05) are represented in bold. Asterisks (*) denotes the significant covariates at each time point: * p < .05, ** p < .01, *** p < .001. kmeans: hierarchical clusterization based on k-means according to Bifidobacterium relative abundance; AB_birth: antibiotic exposure at birth; AB_pregnancy: antibiotic exposure of the mother during pregnancy; AB_exposure: antibiotic exposure of the infant during his life; Delivery_mode: mode of delivery; Lactation_mode: mode of lactation. Dissimilarities in gut microbiota composition according to Bifidobacterium relative abundance (High or Low) represented by nonmetric multidimensional scaling (NMDS) of zOTU relative abundances for infants (b) 7 days and (d) 1 month of age. The centroids of each group are in blue (Low) and yellow (High). The top contributing categorical variables are displayed as arrows, with a length proportional to the correlation between the variable and the NMDS ordination. (e) Multivariable association between factors and taxonomy in both clusters while accounting for potentially confounding covariates using MaAsLin.
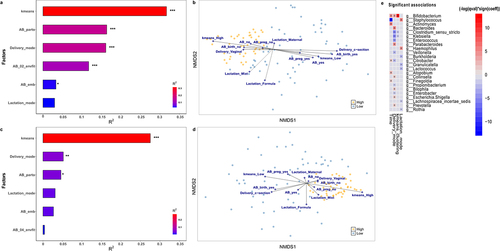
Delivery mode was the second most important environmental factor contributing to the different microbial composition at 1 month of life (R2 = 0.0521, p < .01) (). Vaginal birth was associated with high-Bifidobacterium infants (). Lactation mode played a crucial role in the gut composition (R2 = 0.051, p < .05), even more than antibiotic exposure at birth (R2 = 0.046, p < .05). Breastfeeding (both, exclusive breastfeeding and mixed practises) contributed to the high-Bifidobacterium cluster, while infant formula-feeding was associated with the low-Bifidobacterium group. Antibiotic exposure of the mother during pregnancy and of the infant during the first month of life were the least contributing factors, without statistical significance (R2 = 0.033, p > .05 and R2 = 0.001, p < .05, respectively).
Significant associations between microbial genera and environmental variables were assessed (). The Bifidobacterium genus was most strongly and positively correlated with k-means clustering, followed by time of sampling and delivery mode. Hence, these results support the previous analysis, confirming that the microbial composition of our population differentiates into two groups due mainly to Bifidobacterium relative abundance. Moreover, Clostridium and Klebsiella species correlate negatively to this clustering. The variable time shows a significant positive correlation with Actinomyces, Citrobacter, Atopobium and Finegoldia, while it is negatively correlated with Staphylococcus. Mode of delivery is positively associated with Bacteroides, Parabacteroides, Collinsella, Bilophila, Escherichia/Shigella and Prevotella, and mode of lactation, is positively correlated with Staphylococcus and Haemophilus genus.
Bifidobacterium relative abundance modulates antibiotic resistant gene load
Antibiotic resistance load and presence diverged depending on Bifidobacterium relative abundance (Supplementary table S7). The multivariate redundant discriminant analysis (RDA) () showed that, for 7 days infant gut samples, tetO, ermB, and tetM were the ARGs associated with the Low-Bifidobacterium cluster, and further analysis suggest that their sources are likely Escherichia/Shigella, Phocaeicola, Blautia, Veillonella, Raoutella, and Staphylococcus, whereas Streptococcus, Enterococcus, and Klebsiella were associated to the high-Bifidobacterium cluster.
Figure 5. Redundancy Discriminant Analysis (RDA) biplot depicting the relationship between the bacterial communities and copies of ARGs. Coloured arrows represent differential bacteria and copies ARGs, colored dots represent samples from different groups. Angles between the arrows represent correlations; acute angles represent positive correlations and obtuse angles represent negative correlations. The black arrows are the ARGs that best explain the differences between the sample groups. The orange arrows are the taxa that best explain the differences between the sample groups (n = 9 taxa).
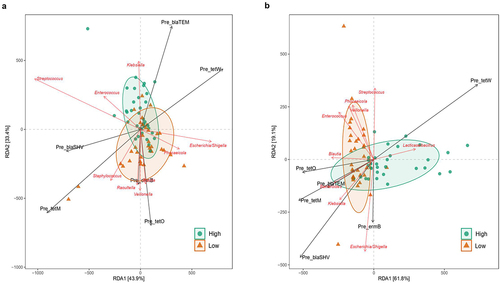
In 1-month-old infants, the Low cluster was positively associated with Blautia, Enterococcus, Veillonella, Phocaelcola, Streptococcus, Escherichia/Shigella, Klebsiella, and Bacteroides, and the last three genera were highly correlated with copies of tetO, tetM, blaTEM, blaSHV (p = .016), and ermB resistant genes. On the contrary, tetW is negatively correlated with the other ARGs and taxa and is associated with infants from the high-Bifidobacterium cluster. This gene (tetW) is more prevalent (p = .02) in 1-month-old infants in the high-Bifidobacterium (73.08% of presence) group compared to the low-Bifidobacterium (42.50%).
Spearman correlations between microbiota at the genus level and copies of ARGs were performed (). In 7-day-old infants with higher abundance of Bifidobacterium, a high abundance of the Lachnospira genus was associated with a higher abundance of blaTEM, and tetW gene (p < .05), while in the low-Bifidobacterium infants, a higher abundance of blaTEM gene was associated to higher abundance of Escherichia/Shigella (p < .05). In addition, in the low-Bifidobacterium cluster, tetM, and ermB genes appear negatively correlated with Blautia, Clostridium, Klebsiella, and Pseudomonas (p < .01).
Figure 6. Spearman correlations between genera and copies of ARGs. Heat map to show Spearman correlations between copies of ARGs and microbiota composition at the genus level, divided by Bifidobacterium relative abundance clusters (High and Low) for infants of 7 days of age (a) and 1 month of age (b). Statistical differences are marked as follows: * p < .05, ** p < .01.
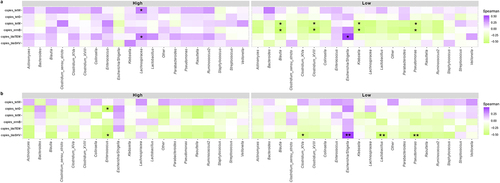
On the other hand, in the High cluster of 1-month-old infants, there is a positive correlation between blaSHV,and tetO genes and Enterococcus (p < .01). For the Low cluster, blaSHV is negatively correlated with Clostridium (p < .05), Lactobacillus (p < .001), and Pseudomonas (p < .001), and positively correlated with Escherichia/Shigella (p < .001).
Discussion
We demonstrated the association of Bifidobacterium spp. with the modulation of early-life microbiota and the antibiotic resistance gene load, and determined which perinatal factors are involved in these complex interactions.
Our primary findings showed that the presence of ARGs in infant’s gut correlated with a low-Bifidobacterium abundance, so we focused on how the abundance of Bifidobacterium could shape the infant resistome in the early stages of life. Results from this resistance epidemiology study align with previous studiesCitation6: the microbiota of low-Bifidobacterium dominated infants is associated with a higher abundance of antibiotic resistant genes, due to a differential microbial composition responsible for carrying these ARGs, as well as the external factors to which the infant is exposed, such as mode of delivery, antibiotic exposure, and breastfeeding. Overall, our results provide insight into the very early acquisition and development of ARGs in a general population cohort of healthy infants.
Bifidobacterium relative abundance is associated with a specific gut microbiota
The infant gut resistome changes primarily in relation to the microbiome as ARGs are carried by the microorganisms in the gut and transferred between them through horizontal gene transfer. As far as we know, this is the first study encompassing the influence of Bifidobacterium on antibiotic resistance load in infants of 7 days and 1 month of age. These two time points are of high interest as the gut microbiota at these ages is very diverse and still in the early stages of development. Thus, it is still largely shaped by the initial colonizers that the baby is exposed to during delivery and by feeding mode. This is the reason why the specific composition of the gut microbiota can vary widely between individuals.
To achieve our objective, we first studied the microbial profile of our study population. The application of k-means clustering algorithms elucidated that the infants in the study clustered into two different groups according to Bifidobacterium spp. abundance. Bacteroidetes appeared more abundant in those samples with low abundance of Bifidobacterium, enhancing a potential interaction between Bacteroides spp. and Bifidobacterium spp. Both are generalist glycan degraders capable of using a wide range of substrates: Bacteroides spp. are specialized as primary degraders in the metabolism of complex carbohydrates, whereas Bifidobacterium spp. more commonly metabolize smaller glycans, such as oligosaccharides, sometimes through syntrophic interactions with Bacteroides spp., in which they act as secondary degraders.Citation38,Citation39 Some bifidobacteria can produce exopolysaccharides (EPS), which can be metabolized by Bacteroides.Citation40 It has been described that cocultures of Bifidobacterium and Bacteroides behave differently against fermentable carbohydrates as a function of the specific characteristics of the strains from each species.Citation41
It is also known that Bifidobacterium strains have antibacterial activity against various pathogens, including Escherichia coli, Streptococcus spp., Salmonella spp., Listeria spp. and Cutibacterium spp,Citation42 as well as against multidrug-resistant pathogens,Citation43 by nutrient competition,Citation44 biofilm removal or inhibition,Citation45,Citation46 production of antimicrobial substances,Citation47,Citation48 acidification, and enhancement of mucus barrier function.Citation49 In addition, Bifidobacterium can influence the overall composition and diversity of the gut microbiota, which helps to maintain a diverse and stable microbial community in the gut.Citation50 In our study we have observed that Escherichia/Shigella and Streptococcus are more abundant than Bifidobacterium in the low-Bifidobacterium cluster of infants at 7 days of life, which reverts at 1 month of life. This may be explained by the impact of breastfeeding on the infant microbiome, as it is well-known that breastfeeding enriches the Bifidobacterium dominance,Citation51,Citation52 and most of our study population is breastfed. Moreover, at both time points, low-Bifidobacterium infants had higher abundances of Staphylococcus, Klebsiella, and Clostridium than high-Bifidobacterium infants. These genera have been previously found to carry ARGs,Citation2 therefore, suggesting that the correlation between Bifidobacterium and antibiotic resistance load can be a result of the genera that predominate when Bifidobacterium are less dominant. All these results highlight the importance of considering specific species and strain interactions in the relationship among intestinal microbial populations and its dynamics over time.
The application of Bayesian LCA-based method in our microbial population showed a distinct Bifidobacterium species profile in the two groups. Specifically, 1-month-old infants with low-Bifidobacterium abundance were enriched with Bifidobacterium dentium, which is found primarily in the oral cavity and possesses various enzymes that enable it to break down complex carbohydrates and degrade protective glycans on mucin proteins.Citation53,Citation54 Moreover, B. dentium is capable to assimilate type 1 and type 2 HMOs.Citation55,Citation56 By fermenting these carbohydrates, B. dentium produces SCFAs and other metabolites that provide energy to both itself and other gut bacteria.Citation57 This metabolic activity may contribute to the overall functioning and health of the gut ecosystem, enhancing the potential beneficial role of this species in the gut of low-Bifidobacterium infants. A non-classified Bifidobacterium spp. was enriched in the high-Bifidobacterium group of infants, highlighting the importance of species-specific identification in microbial studies to understand the role of each species in the early gut establishment and maturation.
Antibiotic resistance genes in the infant gut
Antibiotic-resistant bacteria carry a set of antibiotic resistance genes, and this set in a population of bacteria is known as the resistome. Most predominant resistance genes encode for proteins that confer resistance to beta-lactams, tetracyclines, macrolides, aminoglycosides, and quinolones.Citation17 Beta-lactamases have been prescribed for many decades, and are commonly used during pregnancy and labor, so it is understandable that resistance appears in infant fecal samples.Citation2 Although tetracycline antibiotics are no longer used in pregnancy, tet genes have been reported to be the most abundant among infant fecal samples and the most representative resistance gene in the infant resistome.Citation58,Citation59 Klassert et. alCitation2 determined that genes conferring resistance to macrolides (mefA, ermB, and ermC) showed the highest dissemination in a sample set of mother-infant pairs and also present the highest likelihood of vertical transmission.Citation60
Our primary results showed a lower number of tetM copies in the guts of those infants clustered in the high-Bifidobacterium group, although, as mentioned, these antibiotics are not used during pregnancy. The prevalence of tetracycline resistance genes in various bacteria species, coupled with the common utilization of tetracycline to treat infections, may explain the acquisition of tet genes by women. Although this antibiotic is not used during pregnancy and early life due to potential dental staining effects, it is still extensively used in animals and, therefore, the environment and the diet may be sources of tet genes without direct exposure to the antibiotic.Citation59 Hence, the vertical transfer of antibiotic resistances from the mother to their offspring, together with the high prevalence of this resistance in the environment, may be the principal mechanism of spread of tetracycline resistance in early life.
To complete the study, other ARGs conferring resistance to antibiotics that are used during pregnancy and labor (beta-lactams and macrolides) were measured and the relationship with specific bacterial communities was assessed. We quantified of blaTEM, blaSHV, and ermB genes in infant fecal samples, and similarly to tet genes, we showed that their presence has been associated with a low abundance of Bifidobacterium.
Antibiotic resistance load is affected by the specific microbiota associated with Bifidobacterium relative abundance
Some studies have shown that Bifidobacterium can help to reduce the risk of antibiotic resistance by competing with pathogenic bacteria for nutrients and space in the gut and by producing antimicrobial compounds that inhibit the growth of other bacteria.Citation61,Citation62 A study conducted in 2019 described a change in the gut microbiome and a reduction in ARG abundance in infants supplemented with Bifidobacterium infantis EVC001.Citation63 In some studies, the negative correlation between Bifidobacterium relative abundance and ARG load has been described in infants from 6 weeks of age.Citation6 Moreover, it has been found that Bifidobacterium themselves are less likely to possess ARGs than other taxa such as Enterococcus, Streptococcus, Staphylococcus, and Bacteroides.Citation6,Citation7 Pseudomonadota, more specifically, Gammaproteobacteria, have been demonstrated to be the major carriers for ARGs in studies either employing culture-based or metagenomics methods.Citation64 Members of the family Enterobacteriaceae, such as Escherichia, Klebsiella, Citrobacter, and Enterobacter, which are responsible for a large range of infections, contribute the most abundant and unique ARGs in the infant’s gut.Citation2
Comparably, our results showed that at 7 days of age, tetO, ermB, and tetM are positively correlated with the bacterial species predominate in the Low cluster of infants, namely Escherichia/Shigella, Phocaeicola, Blautia, Veillonella, Raoutella, and Staphylococcus. Streptococcus, Enterococcus, and Klebsiella were associated with the high-Bifidobacterium cluster at 7 days old gut microbiota and with the low-Bifidobacterium cluster at 1-month old. This result shows the gain of stability of gut microbiota at 1 month of age compared to 7 days, as it has been previously described.Citation65
Moreover, at 1 month of age, low-Bifidobacterium infants are associated with Blautia, Veilonella, Phocaelcola, Escherichia/Shigella, Klebsiella, and Bacteroides, the last three of which highly correlated with copies of tetO, tetM, blaTEM, blaSHV, and ermB resistant genes.
It is important to recall that tetW was negatively correlated with the other ARGs and associated with infants from the high-Bifidobacterium cluster at this time point, which matches with previous findings that indicate that tetW gene is an ARG from the Bifidobacterium genus.Citation66,Citation67 Spearman correlations also highlighted the influence of Escherichia/Shigella on antibiotic resistance load: in low-Bifidobacterium infants, this genus was positively correlated with the blaTEM resistance gene.
Overall, these findings suggest that low levels of Bifidobacterium may lead specific ARG-carrying genera to dominate the infant’s gut, thus increasing the antibiotic resistance load. But how does this Bifidobacterium variation occur? Resistome composition is strongly influenced by the phylogenetic profile of the bacterial population. Considering that there are still numerous bacteria possessing unidentified ARGs, the known ARGs may represent only a small part of the true resistome. In this vein, it would be interesting to include genes conferring resistance to other classes of antibiotics, such as aminoglycosides or quinolones, that, together with a metagenomic approach, would provide a wider view of the infant resistome. Aminoglycosides are widely used for neonatal sepsis and are usually combined with beta-lactams,Citation68 and resistance to quinolones also emerged with clinical use and has become common in some bacterial pathogens. Although quinolones are in limited in the pediatric population, the presence of ciprofloxacin-resistant E. coli in the infant’s fecal microbiota has been described.Citation69
Factors influencing infant’s gut microbiota
High Bifidobacterium dominance in infants’ guts has been directly related to the mode of delivery: vaginal or c-section. C-section-born infants have a decreased abundance of Bifidobacterium compared to vaginally born infants.Citation70,Citation71 For this reason, we assessed whether our microbial clusters may be a result of the procedure of delivery, but we again obtained two different clusters according to Bifidobacterium relative abundance, independent of the mode of delivery. All infants born vaginally had a significantly different microbiota depending on if they had high or low Bifidobacterium relative abundance, at both 7 days and 1 month of age, so we could discard that mode of delivery was shaping our microbiota clusters. Indeed, k-means hierarchical clustering was the variable with the highest contribution to the separation of the microbial composition of our population, as expected. However, we observed role of delivery in the modulation of gut microbiota, as it was associated with genera such as Enterococcus, Klebsiella, and Clostridium, which have been previously related to the microbiota of c-section born infants.Citation72
In addition, it has been seen that early-life antibiotic treatment disrupts the proper and natural development of gut microbiota with a potential negative influence on later health.Citation73 It has also been previously described that intrapartum antibiotic prophylaxis (IAP) has a negative effect on the later bifidobacterial establishment in the neonatal gut.Citation7,Citation74 To assess this effect, antibiotic exposure of our cohort was recorded during pregnancy and also during the first month of life of the infants. During a c-section procedure, antibiotics are commonly administered as a preventive measure to reduce the risk of infection (e.g., cefazolin, vancomycin, clindamycin and erythromycin).Citation75 On the other hand, there is the possibility that vaginal-born infants have been exposed to antibiotics at birth too, and this has been recorded and considered in our study. Interestingly, the antibiotic exposure at delivery and how childbirth occurred had a similar contribution to the microbial composition of infants of 7 days of age, while this interaction dissociated at 1 month of age, with the antibiotic exposure losing importance, indicating a possible mitigation of its effects over time. Nevertheless, in both time points, c-section procedures and exposure to antibiotics contributed to a gut microbiota with low Bifidobacterium spp. Therefore, our results confirm previous knowledge on the effect of antibiotics on the early microbial composition but highlight the importance of Bifidobacterium abundance in the infant’s gut.Citation76
The feeding mode showed a significant contribution to 1-month-old infants’ microbiota but not to that at 7 days old, maybe because of the short period infants consumed milk or because a reduced number of infants that where mixed or formula-fed. Formula-feeding contributed to the low-Bifidobacterium microbiota profile, while mixed and exclusive breastfeeding contributed to the high-Bifidobacterium infants, highlighting the widely known benefits of breastfeeding practises for infant health ,Citation77 , .Citation78,Citation79 Nevertheless, breastmilk plays a crucial role on the acquisition of antibiotic resistance in early life.Citation80 Human milk has been found to contain antibiotic-resistant bacteria, such as Staphylococcus, Streptococcus, Acinetobacter, Enterococcus, and Corynebacterium, resistant to antibiotics and with multidrug-resistant profiles.Citation81 Moreover, metagenomic analyses have highlighted the high levels of ARGs and MGEs in breastmilk and the similarity of the breastmilk and infant gut resistomes.Citation3 Overall, more understanding on mother’s gut and breastmilk resistomes may shed light to decipher how neonates acquire antibiotic resistance genes in early life and the role that environmental factors may play, so new approaches and decisions can be made to reduce antibiotic resistance. The interplay between early microbial colonization, environmental factors, and dietary changes might have lasting effects that extend beyond infancy. Understanding the persistence of these early exposures and their potential link to ARGs could offer valuable insights into strategies for promoting healthy gut microbiota development and combating antibiotic resistance.
Our results support the notion that microbiota plays a crucial role in early-life antibiotic resistance gene acquisition, focusing on infants of 7 days and 1 month of age. Bifidobacterium dominance has been found to be associated with the load of ARGs: low-Bifidobacterium abundance is associated with a higher presence of ARGs in infant’s gut, which is also associated with a specific microbial profile. Moreover, we assessed that antibiotic exposure and mode of delivery are two of the key factors influencing microbial composition, and thus, antibiotic resistance load, and we suggest the potential impact of new or current antibiotic prescription practices during pregnancy, at birth, or during the first month of life on the infant gut microbiome and resistome. Our findings highlight the public health concern of antibiotic resistance and elucidate that more studies are needed to gain further insights into how prevalent antibiotic resistance can be in the population and to develop new strategies to ameliorate the ARG load.
Contribution statement
Conceptualization, RC, and MC; sample collection and clinical data, CM-C; data curation, AS and RC; bioinformatic analysis, AS and RC; statistical analysis, AS and RC; writing-original draft preparation, AS; writing-review, all authors. All authors have read and agreed to the published version of the manuscript.
Abbreviations
ARG | = | Antibiotic Resistant Genes |
HMO | = | Human Milk Oligosaccharides |
Supplemental Material
Download Zip (1.4 MB)Acknowledgments
We would like to thank all the families who were involved in the study as well as whole MAMI team, which includes neonataologist, paediatrisians, midwifes, nurses, research scientists and computer/laboratory technicians and also, we would like to thank the support from Biobank (Biobanco para la Investigación Biomédica y en Salud Pública de la Comunidad Valenciana, IBSP-CV). We also acknowledge GVA-European Social Fund (ACIF/2021) for the predoctoral fellowship grant to Anna Samarra and Plan GenT project (CDEIGENT 2020) to Raul Cabrera-Rubio and also, Spanish Ministry of Science and Innovation (MCIN) research grant (ref. PID2022-139475OB-I00) . IATA-CSIC is a Centre of Excellence Severo Ochoa (CEX2021-001189-S MCIN/AEI/10.13039/501100011033).
Disclosure statement
No potential conflict of interest was reported by the author(s).
Data availability statement
The dataset supporting the conclusions of this article is included in the NCBI’s Sequence Read Archive (SRA) repository in the MAMI BioProject ID PRJNA614975 (http://www.ncbi.nlm.nih.gov/bioproject/61497).
Supplementary material
Supplemental data for this article can be accessed online at https://doi.org/10.1080/19490976.2024.2357176
Additional information
Funding
References
- Patangia DV, Ryan CA, Dempsey E, Stanton C, Ross RP. Vertical transfer of antibiotics and antibiotic resistant strains across the mother/baby axis. Trends Microbiol. 2022;30(1):47–20. doi:10.1016/j.tim.2021.05.006.
- Li X, Stokholm J, Brejnrod A, Vestergaard GA, Russel J, Trivedi U, Thorsen J, Gupta S, Hjelmsø MH, Shah SA. et al. The infant gut resistome associates with E. coli, environmental exposures, gut microbiome maturity, and asthma-associated bacterial composition. Cell Host Microbe [Internet]. 2021;29(6):975–987.e4. [accessed 2022 Feb 10]. http://www.cell.com/article/S1931312821001451/fulltext.
- Pärnänen K, Karkman A, Hultman J, Lyra C, Bengtsson-Palme J, Larsson DGJ, Rautava S, Isolauri E, Salminen S, Kumar HSatokari R. et al. Maternal gut and breast milk microbiota affect infant gut antibiotic resistome and mobile genetic elements. Nat Commun [Internet]. 2018;9(1):3891. [accessed 2023 Mar 26]. doi:10.1038/s41467-018-06393-w.
- Lawson MAE, O’Neill IJ, Kujawska M, Gowrinadh Javvadi S, Wijeyesekera A, Flegg Z, Chalklen L, Hall LJ. Breast milk-derived human milk oligosaccharides promote Bifidobacterium interactions within a single ecosystem. Isme J. 2019 [accessed 2023 Apr 3]. 14(2):635–648. https://www.nature.com/articles/s41396-019-0553-2.
- Walsh C, Lane JA, van Sinderen D, Hickey RM. Human milk oligosaccharides: Shaping the infant gut microbiota and supporting health. J Funct Foods. 2020;72:104074. doi:10.1016/j.jff.2020.104074.
- Taft DH, Liu J, Maldonado-Gomez MX, Akre S, Huda MN, Ahmad SM, Stephensen CB, Mills DA, Suen G. Bifidobacterial dominance of the gut in early life and acquisition of antimicrobial resistance. mSphere. 2018;3(5):10–128. [accessed 2022 Dec 20]. https:/pmc/articles/PMC6158511/.
- Saturio S, Suárez M, Mancabelli L, Fernández N, Mantecón L, de Los Reyes-Gavilán CG, Ventura M, Gueimonde M, Arboleya S, Solís G. Effect of intrapartum antibiotics prophylaxis on the bifidobacterial establishment within the neonatal gut. Microorganisms. 2021;9(9):1867. [accessed 2023 Mar 26]. https:/pmc/articles/PMC8471514/.
- van Schaik W. The human gut resistome. Phil Trans R Soc B. 2015;370(1670):20140087. [accessed 2024 Mar 28]. doi:10.1098/rstb.2014.0087.
- Duranti S, Lugli GA, Mancabelli L, Turroni F, Milani C, Mangifesta M, Ferrario C, Anzalone R, Viappiani A, van Sinderen D. et al. Prevalence of antibiotic resistance genes among human gut-derived bifidobacteria. Appl Environ Microbiol [Internet]. 2017;83(3):e02894–16. [accessed 2024 Mar 28]. https://pubmed.ncbi.nlm.nih.gov/27864179/.
- Michaelis C, Grohmann E. Horizontal gene transfer of antibiotic resistance genes in biofilms. Antibiotics [Internet]. 2023;12(2):328. [accessed 2024 Mar 28]. https:/pmc/articles/PMC9952180/.
- Ellabaan MMH, Munck C, Porse A, Imamovic L, Sommer MOA. Forecasting the dissemination of antibiotic resistance genes across bacterial genomes. Nat Commun [Internet]. 2021;12(1):1–10. [accessed 2024 Mar 28]. https://www.nature.com/articles/s41467-021-22757-1.
- Hill CJ, Lynch DB, Murphy K, Ulaszewska M, Jeffery IB, O’Shea CA, Watkins C, Dempsey E, Mattivi F, Tuohy K. et al. Evolution of gut microbiota composition from birth to 24 weeks in the INFANTMET Cohort. Microbiome [Internet]. 2017;5(1):1–18. [accessed 2022 Dec 20]. doi:10.1186/s40168-016-0213-y.
- García-Mantrana I, Alcántara C, Selma-Royo M, Boix-Amorós A, Dzidic M, Gimeno-Alcañiz J, Úbeda-Sansano I, Sorribes-Monrabal I, Escuriet R, Gil-Raga F. et al. MAMI: A birth cohort focused on maternal-infant microbiota during early life. BMC Pediatr [Internet]. 2019;19(1):1–8. [accessed 2022 Dec 20]. doi:10.1186/s12887-019-1502-y.
- Selma-Royo M, Calatayud Arroyo M, García-Mantrana I, Parra-Llorca A, Escuriet R, Martínez-Costa C, Collado MC. Perinatal environment shapes microbiota colonization and infant growth: impact on host response and intestinal function. Microbiome [Internet]. 2020;8(1):1–19. [accessed 2023 Jul 20]. doi:10.1186/s40168-020-00940-8.
- Zhang K, Jin M, Yang D, Shen Z, Liu W, Yin J, Yang Z, Wang H, Shi D, Yang J. et al. Antibiotic resistance genes in gut of breast-fed neonates born by caesarean section originate from breast milk and hospital ward air. BMC Microbiol [Internet]. 2022 [accessed 2023 Mar 26]. 22(1):1–11. doi:10.1186/s12866-022-02447-8.
- Carvalho MJ, Sands K, Thomson K, Portal E, Mathias J, Milton R, Gillespie D, Dyer C, Akpulu C, Boostrom I. et al. Antibiotic resistance genes in the gut microbiota of mothers and linked neonates with or without sepsis from low- and middle-income countries. Nat Microbiol [Internet]. 2022;7(9):1337. [accessed 2023 Mar 26]. https:/pmc/articles/PMC9417982/.
- Klassert TE, Zubiria-Barrera C, Kankel S, Stock M, Neubert R, Lorenzo-Diaz F, Doehring N, Driesch D, Fischer D, Slevogt H. Early bacterial colonization and antibiotic resistance gene acquisition in Newborns. Front Cell Infect Microbiol. 2020;10:332. doi:10.3389/fcimb.2020.00332.
- Loo EXL, Zain A, Yap GC, Purbojati RW, Drautz-Moses DI, Koh YQ, Chong YS, Tan KH, Gluckman PD, Yap F. et al. Longitudinal assessment of antibiotic resistance gene profiles in gut microbiomes of infants at risk of eczema. BMC Infect Dis [Internet]. 2020;20(1):1–12. [accessed 2023 Mar 26]. doi:10.1186/s12879-020-05000-y.
- Stedtfeld RD, Guo X, Stedtfeld TM, Sheng H, Williams MR, Hauschild K, Gunturu S, Tift L, Wang F, Howe A. et al. Primer set 2.0 for highly parallel qPCR array targeting antibiotic resistance genes and mobile genetic elements. FEMS Microbiol Ecol [Internet]. 2018;94(9):fiy130. [accessed 2022 Dec 20]. https://academic.oup.com/femsec/article/94/9/fiy130/5057470.
- Aronesty E. Comparison of sequencing utility programs. Open Bioinforma J. 2013;7(1):1–8. doi:10.2174/1875036201307010001.
- Edgar RC. Search and clustering orders of magnitude faster than BLAST. Bioinformatics [Internet]. 2010;26(19):2460–2461. [accessed 2023 Feb 13]. https://pubmed.ncbi.nlm.nih.gov/20709691/.
- Edgar RC. UNOISE2: improved error-correction for Illumina 16S and ITS amplicon sequencing. bioRxiv. 2016;081257. [accessed 2023 Feb 13]. doi:10.1101/081257v1.
- Caporaso JG, Kuczynski J, Stombaugh J, Bittinger K, Bushman FD, Costello EK, Fierer N, Pẽa AG, Goodrich JK, Gordon JI. et al. QIIME allows analysis of high-throughput community sequencing data. Nat Methods [Internet]. 2010;7(5):335–336. [accessed 2023 Feb 13]. https://pubmed.ncbi.nlm.nih.gov/20383131/.
- Bokulich NA, Kaehler BD, Rideout JR, Dillon M, Bolyen E, Knight R, Huttley GA, Gregory Caporaso J. Optimizing taxonomic classification of marker-gene amplicon sequences with QIIME 2’s q2-feature-classifier plugin. Microbiome [Internet]. 2018;6:1–17. [accessed 2023 Feb 13]. doi:10.1186/s40168-018-0470-z.
- Cole JR, Wang Q, Fish JA, Chai B, McGarrell DM, Sun Y, Brown CT, Porras-Alfaro A, Kuske CR, Tiedje JM. Ribosomal database project: data and tools for high throughput rRNA analysis. Nucleic Acids Res [Internet]. 2014;42(D1):D633. [accessed 2023 Feb 13]. https:/pmc/articles/PMC3965039/.
- Katoh K, Standley DM. MAFFT multiple sequence alignment software version 7: improvements in performance and usability. Mol Biol Evol [Internet]. 2013;30(4):772–780. [accessed 2023 Feb 13]. https://pubmed.ncbi.nlm.nih.gov/23329690/.
- Price MN, Dehal PS, Arkin AP. FastTree 2 – approximately maximum-likelihood trees for large alignments. PLoS One [Internet]. 2010;5(3):e9490. [accessed 2023 Feb 13]. https:/pmc/articles/PMC2835736/.
- Gao X, Lin H, Revanna K, Dong Q. A Bayesian taxonomic classification method for 16S rRNA gene sequences with improved species-level accuracy. BMC Bioinf [Internet]. 2017;18(1):1–10. [accessed 2023 June 29]. https://pubmed.ncbi.nlm.nih.gov/28486927/.
- Pruesse E, Quast C, Knittel K, Fuchs BM, Ludwig W, Peplies J, Glöckner FO. SILVA: a comprehensive online resource for quality checked and aligned ribosomal RNA sequence data compatible with ARB. Nucleic Acids Res [Internet]. 2007;35(21):7188–7196. [accessed 2023 June 29]. https://pubmed.ncbi.nlm.nih.gov/17947321/.
- Wickham H. ggplot2. ggplot2; 2009.
- Kassambara A, Mundt F. Extract and visualize the results of multivariate data analyses [R package factoextra version 1.0.7]; 2020.
- Chen H, Boutros PC. VennDiagram: A package for the generation of highly-customizable Venn and Euler diagrams in R. BMC Bioinf [Internet]. 2011 [accessed 2023 Feb 7]. 12(1):1–7. doi:10.1186/1471-2105-12-35.
- McMurdie PJ, Holmes S, Watson M. phyloseq: An R package for reproducible interactive analysis and graphics of microbiome census data. PLOS ONE [Internet]. 2013 [accessed 2023 Feb 7]. 8(4):e61217. doi:10.1371/journal.pone.0061217.
- Community Ecology Package [R package vegan version 2.6-4]. 2022 [accessed 2023 Feb 6]. https://CRAN.R-project.org/package=vegan.
- Kassambara A. Pipe-friendly framework for basic statistical tests [R package rstatix version 0.6.0]. 2020.
- Mallick H, Rahnavard A, McIver LJ, Ma S, Zhang Y, Nguyen LH, Tickle TL, Weingart G, Ren B, Schwager EH. et al. Multivariable association discovery in population-scale meta-omics studies. PLOS Comput Biol [Internet]. 2021 [accessed 2023 June 29]. 17(11):e1009442. doi:10.1371/journal.pcbi.1009442.
- Benjamini Y, Hochberg Y. Controlling the false discovery rate: a practical and powerful approach to multiple testing. J R Stat Soc B (Methodol) [Internet]. 1995;57(1):289–300. [accessed 2023 Feb 7]. doi:10.1111/j.2517-6161.1995.tb02031.x.
- Fernandez-Julia PJ, Munoz-Munoz J, van Sinderen D. A comprehensive review on the impact of β-glucan metabolism by Bacteroides and Bifidobacterium species as members of the gut microbiota. Int J Biol Macromol. 2021;181:877–889. doi:10.1016/j.ijbiomac.2021.04.069.
- Nishiyama K, Yokoi T, Sugiyama M, Osawa R, Mukai T, Okada N. Roles of the cell surface architecture of bacteroides and Bifidobacterium in the gut colonization. Front Microbiol. 2021;12:754819.
- Rios-Covian D, Cuesta I, Alvarez-Buylla JR, Ruas-Madiedo P, Gueimonde M, De Los Reyes-Gavilán CG. Bacteroides fragilis metabolises exopolysaccharides produced by bifidobacteria. BMC Microbiol [Internet]. 2016;16(1):1–8. [accessed 2023 Jul 11]. doi:10.1186/s12866-016-0773-9.
- Rios-Covian D, Arboleya S, Hernandez-Barranco AM, Alvarez-Buylla JR, Ruas-Madiedo P, Gueimonde M, De Los Reyes-Gavilan CG. Interactions between Bifidobacterium and bacteroides species in cofermentations are affected by carbon sources, including exopolysaccharides produced by Bifidobacteria. Appl Environ Microbiol [Internet]. 2013;79:7518. [accessed 2023 Jul 11]. https:/pmc/articles/PMC3837738/.
- Lim HJ, Shin HS. Antimicrobial and immunomodulatory effects of Bifidobacterium strains: a review. J Microbiol Biotechnol [Internet]. 2020;30(12):1793. [accessed 2024 Mar 28]. https:/pmc/articles/PMC9728261/.
- Choi YJ, Shin HS. Antibacterial effect of eight probiotic strains of Bifidobacterium against pathogenic staphylococcus aureus and Pseudomonas aeruginosa. J Bacteriol Virol [Internet]. 2021;51(3):128–137. [accessed 2024 Mar 28]. https:/journal-jbv.apub.kr/articles/xml/z4AG/.
- Horrocks V, King OG, Yip AYG, Marques IM, McDonald JAK. Role of the gut microbiota in nutrient competition and protection against intestinal pathogen colonization. Microbiology (N Y) [Internet]. 2023;169(8):1377. [accessed 2024 Mar 28]. https:/pmc/articles/PMC10482380/.
- Abdelhamid AG, Esaam A, Hazaa MM. Cell free preparations of probiotics exerted antibacterial and antibiofilm activities against multidrug resistant E. coli. Saudi Pharm J [Internet]. 2018;26(5):603–607. [accessed 2024 Mar 28]. https://pubmed.ncbi.nlm.nih.gov/29991904/.
- Tomé AR, Carvalho FM, Teixeira-Santos R, Burmølle M, Mergulhão FJM, Gomes LC. Use of probiotics to control biofilm formation in food industries. Antibiotics [Internet]. 2023;12(4):754. [accessed 2024 Mar 28]. https:/pmc/articles/PMC10135146/.
- Javvadi SG, Kujawska M, Papp D, Gontarczyk AM, Jordan A, Lawson MAE, O’Neill IJ, Alcon-Giner C, Kiu R, Clarke P. et al. A novel bacteriocin produced by Bifidobacterium longum subsp. infantis has dual antimicrobial and immunomodulatory activity. bioRxiv. 2022;2022–01. [accessed 2024 Mar 28]. doi:10.1101/2022.01.27.477972v2.
- Touré R, Kheadr E, Lacroix C, Moroni O, Fliss I. Production of antibacterial substances by bifidobacterial isolates from infant stool active against Listeria monocytogenes. J Appl Microbiol [Internet]. 2003;95:1058–1069. [accessed 2024 Mar 28]. doi:10.1046/j.1365-2672.2003.02085.x.
- Gutierrez A, Pucket B, Engevik MA. Bifidobacterium and the intestinal mucus layer. Microbiome Res Rep [Internet]. 2023;2(4). [accessed 2024 Mar 28]. https:/pmc/articles/PMC10688832/.
- O’Callaghan A, van Sinderen D. Bifidobacteria and their role as members of the human gut microbiota. Front Microbiol [Internet]. 2016;7:925. [accessed 2024 Mar 28]. https:/pmc/articles/PMC4908950/.
- Yatsunenko T, Rey FE, Manary MJ, Trehan I, Dominguez-Bello MG, Contreras M, Magris M, Hidalgo G, Baldassano RN, Anokhin AP. et al. Human gut microbiome viewed across age and geography. Nature [Internet]. 2012;486(7402):222. [accessed 2023 Apr 3]. https:/pmc/articles/PMC3376388/.
- Penders J, Thijs C, Vink C, Stelma FF, Snijders B, Kummeling I, Van Den Brandt PA, Stobberingh EE. Factors influencing the composition of the intestinal microbiota in early infancy. Pediatrics [Internet]. 2006;118(2):511–521. [accessed 2023 Apr 3]. https:/pediatrics/article/118/2/511/68933/Factors-Influencing-the-Composition-of-the.
- Engevik MA, Luk B, Chang-Graham AL, Hall A, Herrmann B, Ruan W, Endres BT, Shi Z, Garey KW, Hyser JM. et al. Bifidobacterium dentium fortifies the intestinal mucus layer via autophagy and calcium signaling pathways. MBio [Internet]. 2019;10(3):10–128. [accessed 2023 June 29]. https:/pmc/articles/PMC6581858/.
- Ghoddusi HB, Tamime AY. MICROFLORA of the INTESTINE | Biology of Bifidobacteria. Encycl Food Microbiol: Second Ed. 2014;2:639–645.
- Moya-Gonzálvez EM, Rubio-Del-Campo A, Rodríguez-Díaz J, Yebra MJ. Infant-gut associated Bifidobacterium dentium strains utilize the galactose moiety and release lacto-N-triose from the human milk oligosaccharides lacto-N-tetraose and lacto-N-neotetraose. Sci Rep [Internet]. 2021;11(1):23328. [accessed 2023 June 29]. https:/pmc/articles/PMC8639736/.
- Sakanaka M, Gotoh A, Yoshida K, Odamaki T, Koguchi H, Xiao JZ, Kitaoka M, Katayama T. Varied pathways of infant gut-associated Bifidobacterium to assimilate human milk oligosaccharides: prevalence of the gene set and its correlation with Bifidobacteria-Rich Microbiota formation. Nutr. 2020 2019;12(1):71. [accessed 2023 June 29]. https://www.mdpi.com/2072-6643/12/1/71/htm.
- Engevik MA, Danhof HA, Hall A, Engevik KA, Horvath TD, Haidacher SJ, Hoch KM, Endres BT, Bajaj M, Garey KW. et al. The metabolic profile of Bifidobacterium dentium reflects its status as a human gut commensal. BMC Microbiol [Internet]. 2021;21(1):154. [accessed 2023 June 29]. https:/pmc/articles/PMC8145834/.
- Haddad EN, Comstock SS. Archive for research in child health (ARCH) and baby gut: Study protocol for a remote, prospective, longitudinal pregnancy and birth cohort to address microbiota development and child health. Methods Protoc [Internet]. 2021;4(3):52. [accessed 2022 Feb 10]. https:/pmc/articles/PMC8395764/.
- Karami N, Nowrouzian F, Adlerberth I, Wold AE. Tetracycline resistance in Escherichia coli and persistence in the infantile colonic microbiota. Antimicrob Agents Chemother. 2006;50(1):156–161. [accessed 2022 Feb 10]. https://pubmed.ncbi.nlm.nih.gov/16377681/.
- Severgnini M, Camboni T, Ceccarani C, Morselli S, Cantiani A, Zagonari S, Patuelli G, Pedna MF, Sambri V, Foschi C. et al. Distribution of ermb, ermf, tet(W), and tet(m) resistance genes in the vaginal ecosystem of women during pregnancy and puerperium. Pathogens [Internet]. 2021;10(12):1546. [accessed 2022 May 14]. https:/pmc/articles/PMC8705968/.
- Merenstein D, Fraser CM, Roberts RF, Liu T, Grant-Beurmann S, Tan TP, Smith KH, Cronin T, Martin OA, Sanders ME. et al. Bifidobacterium animalis subsp. lactis BB-12 protects against antibiotic-induced functional and compositional changes in human fecal microbiome. Nutr. 2021;13(8):2814. [accessed 2023 Feb 13]. https://pubmed.ncbi.nlm.nih.gov/34444974/.
- Pathak P, Trilligan C, Rapose A. Case Report: Bifidobacterium—friend or foe? A case of urinary tract infection with Bifidobacterium species. BMJ Case Rep [Internet]. 2014;2014(sep24 1):bcr2014205122–bcr2014205122. [accessed 2023 Feb 13]. https:/pmc/articles/PMC4173150/.
- Casaburi G, Duar RM, Vance DP, Mitchell R, Contreras L, Frese SA, Smilowitz JT, Underwood MA. Early-life gut microbiome modulation reduces the abundance of antibiotic-resistant bacteria. Antimicrob Resist Infect Control [Internet]. 2019;8(1):1–8. [accessed 2022 Dec 20]. https://pubmed.ncbi.nlm.nih.gov/31423298/.
- Khan I, Yasir M, Farman M, Kumosani T, Albasri SF, Bajouh OS, Azhar EI. Evaluation of gut bacterial community composition and antimicrobial resistome in pregnant and non-pregnant women from Saudi population. Infect Drug Resist [Internet]. 2019;12:1749. [accessed 2022 Feb 10]. https:/pmc/articles/PMC6593780/.
- Bäckhed F, Roswall J, Peng Y, Feng Q, Jia H, Kovatcheva-Datchary P, Li Y, Xia Y, Xie H, Zhong H. et al. Dynamics and stabilization of the human gut microbiome during the first year of life. Cell Host Microbe [Internet]. 2015;17(5):690–703. [accessed 2023 Jul 11]. http://www.cell.com/article/S1931312815001626/fulltext.
- Nøhr-Meldgaard K, Struve C, Ingmer H, Agersø Y. The tetracycline resistance gene, tet(W) in Bifidobacterium animalis subsp. lactis follows phylogeny and differs from tet(W) in other species. Front Microbiol. 2021;12:1623. doi:10.3389/fmicb.2021.658943.
- Cao L, Chen H, Wang Q, Li B, Hu Y, Zhao C, Hu Y, Yin Y. Literature-based phenotype survey and in silico genotype investigation of antibiotic resistance in the genus Bifidobacterium. Curr Microbiol. 2020;77(12):4104–4113. doi:10.1007/s00284-020-02230-w.
- Fouhy F, Ogilvie LA, Jones BV, Ross RP, Ryan AC, Dempsey EM, Fitzgerald GF, Stanton C, Cotter PD, Abdo Z. Identification of aminoglycoside and β-lactam resistance genes from within an infant gut functional metagenomic library. PLoS One [Internet]. 2014;9(9):e108016. [accessed 2022 May 14]. https://pubmed.ncbi.nlm.nih.gov/25247417/.
- Gurnee EA, Ndao IM, Johnson JR, Johnston BD, Gonzalez MD, Burnham CAD, Hall-Moore CM, McGhee JE, Mellmann A, Warner BB. et al. Gut colonization of healthy children and their mothers with pathogenic ciprofloxacin-resistant Escherichia coli. J Infect Dis [Internet]. 2015;212(12):1862–1868. [accessed 2022 Oct 18]. https://pubmed.ncbi.nlm.nih.gov/25969564/.
- Selma-Royo M, Calatayud Arroyo M, García-Mantrana I, Parra-Llorca A, Escuriet R, Martínez-Costa C, Collado MC. Perinatal environment shapes microbiota colonization and infant growth: impact on host response and intestinal function. Microbiome [Internet]. 2020;8(1):1–9. [accessed 2023 Apr 3]. https://pubmed.ncbi.nlm.nih.gov/33228771/.
- Pan K, Zhang C, Tian J. The effects of different modes of delivery on the structure and predicted function of intestinal microbiota in neonates and early infants. Pol J Microbiol [Internet]. 2021;70:45. [accessed 2023 Mar 26]. https:/pmc/articles/PMC8008759/.
- Selma-Royo M, Calatayud Arroyo M, García-Mantrana I, Parra-Llorca A, Escuriet R, Martínez-Costa C, Collado MC. Perinatal environment shapes microbiota colonization and infant growth: impact on host response and intestinal function. Microbiome [Internet]. 2020;8(1):1–9. [accessed 2023 June 28]. https:/pmc/articles/PMC7685601/.
- Gibson MK, Crofts TS, Dantas G. Antibiotics and the developing infant gut microbiota and resistome. Curr Opin Microbiol [Internet]. 2015;27:51. [accessed 2023 Mar 26]. https:/pmc/articles/PMC4659777/.
- Mazzola G, Murphy K, Ross RP, Di Gioia D, Biavati B, Corvaglia LT, Faldella G, Stanton C, Wilson BA. Early gut microbiota perturbations following intrapartum antibiotic prophylaxis to prevent group B streptococcal disease. PLOS One [Internet]. 2016:11(6):e0157527. [accessed 2023 Mar 26]. https://pubmed.ncbi.nlm.nih.gov/27332552/.
- ACOG Guidance: Antibiotic Prophylaxis during Labor and Delivery - The ObG Project [Internet]. [accessed 2023 June 28]. https://www.obgproject.com/2018/08/29/acog-guidance-antibiotic-prophylaxis-during-labor-and-delivery/.
- Reyman M, van Houten MA, Watson RL, Chu MLJN, Arp K, de Waal WJ, Schiering I, Plötz FB, Willems RJL, van Schaik W. et al. Effects of early-life antibiotics on the developing infant gut microbiome and resistome: a randomized trial. Nat Commun [Internet]. 2022;13(1):1–12. [accessed 2023 Mar 26]. https://www.nature.com/articles/s41467-022-28525-z.
- Nadimpalli ML, Bourke CD, Robertson RC, Delarocque-Astagneau E, Manges AR, Pickering AJ. Can breastfeeding protect against antimicrobial resistance? BMC Med [Internet]. 2020;18(1):1–11. [accessed 2022 Feb 14]. doi:10.1186/s12916-020-01862-w.
- World Health Organization. Exclusive breastfeeding for six months best for babies everywhere [Internet]. Exclusive breastfeeding for six months best for babies everywhere. 2011 [accessed 2022 Oct 24]. https://www.who.int/news/item/15-01-2011-exclusive-breastfeeding-for-six-months-best-for-babies-everywhere.
- Liu Y, Qin S, Song Y, Feng Y, Lv N, Xue Y, Liu F, Wang S, Zhu B, Ma J. et al. The perturbation of infant gut microbiota caused by cesarean delivery is partially restored by exclusive breastfeeding. Front Microbiol [Internet]. 2019;10:427987. [accessed 2022 Oct 5]. https://pubmed.ncbi.nlm.nih.gov/30972048/.
- Samarra A, Esteban-Torres M, Cabrera-Rubio R, Bernabeu M, Arboleya S, Gueimonde M, Collado MC. Maternal-infant antibiotic resistance genes transference: what do we know? Gut Microbes [Internet]. 2023;15:2194797. [accessed 2023 June 28]. https://pubmed.ncbi.nlm.nih.gov/37020319/.
- Das L, Virmani R, Sharma V, Rawat D, Singh Y. Human milk microbiota: transferring the antibiotic resistome to infants. Indian J Microbiol [Internet]. 2019;59:410–416. [accessed 2022 Jan 20]. https://pubmed.ncbi.nlm.nih.gov/31762502/.