ABSTRACT
Inhalation of ambient particulate matter (PM) can disrupt the gut microbiome, while exercise independently influences the gut microbiome by promoting beneficial bacteria. In this study, we analyzed changes in gut microbial diversity and composition in response to combined interventions of PM exposure and aerobic exercise, extending up to 12 weeks. This investigation was conducted using mice, categorized into five groups: control group (Con), exercise group (EXE), exercise group followed by 3-day exposure to PM (EXE + 3-day PM), particulate matter exposure (PM), and PM exposure with concurrent treadmill exercise (PME). Notably, the PM group exhibited markedly lower alpha diversity and richness compared to the Con group and our analysis of beta diversity revealed significant variations among the intervention groups. Members of the Lachnospiraceae family showed significant enhancement in the exercise intervention groups (EXE and PME) compared to the Con and PM groups. The biomarker Lactobacillus, Coriobacteraceae, and Anaerofustis were enriched in the EXE group, while Desulfovibrionaceae, Mucispirillum schaedleri, Lactococcus and Anaeroplasma were highly enriched in the PM group. Differential abundance analysis revealed that Paraprevotella, Bacteroides, and Blautia were less abundant in the 12-week PM exposure group than in the 3-day PM exposure group. Moreover, both the 3-day and 12-week PM exposure groups exhibited a reduced relative abundance of Bacteroides uniformis, SMB53, and Staphylococcus compared to non-PM exposure groups. These findings will help delineate the possible roles and associations of altered microbiota resulting from the studied interventions, paving the way for future mechanistic research.
1. Introduction
Particulate matter (PM) is a complex mixture of tiny solid particles and liquid droplets that enter the atmosphere through various means, including vehicle emissions, industrial and agricultural activities, construction, and natural sources such as dust and pollen. PM consists of a blend of organic chemicals, metals, acids, and other particles, making it one of the most significant contributors to air pollution (Anderson et al. Citation2012). This poses serious concerns for both environmental and public health (Arias-Pérez et al. Citation2020). As per the 2019 World Health Organization (WHO) report, a staggering 99% of the global population lived in areas where air quality fell short of WHO standards (Organization Citation2023). PM particles come in various sizes, classified by their aerodynamic diameter as PM 10 (coarse particles, < 10μm), PM 2.5 (fine particles, < 2.5μm), and PM 0.1 (ultrafine particles, < 0.1μm). Coarse and fine particles often originate from different sources and exhibit various chemical compositions. Exposure to elevated levels of PM can result in damage to the lungs and various other organs in the body (Schraufnagel et al. Citation2019). Notably, PM 2.5, a major air pollutant, caused approximately 4 million deaths globally in 2019 (Collaborators and Ärnlöv Citation2020), and was associated with an increased risk of neurodegeneration. Among PM 2.5 components, black carbon and sulfate displayed the strongest associations with dementia and Alzheimer’s disease (Shi et al. Citation2023). PM 10, which is generally found in higher proportions than PM 2.5, primarily stems from forest fires, waste combustion, and industrial and agricultural waste (Tian et al. Citation2021). While PM’s adverse effects on the respiratory (Téllez-Rojo et al. Citation2000; Leikauf et al. Citation2020; Misiukiewicz-Stepien and Paplinska-Goryca Citation2021; Albano et al. Citation2022; Lee et al. Citation2023) and cardiovascular systems (Hamanaka and Mutlu Citation2018; Tschofen et al. Citation2019; Basith et al. Citation2022; Ji Citation2022) have been extensively studied, recent evidence has revealed its detrimental impacts on the gastrointestinal (GI) tract (Vignal et al. Citation2017; Pambianchi et al. Citation2022), including the modulation of the gut microbiome. In addition to direct deposition in the respiratory system, PM can be indirectly deposited in the oropharynx through mucociliary clearance and via the swallowing of mucus and saliva (Mutlu et al. Citation2018; Xie et al. Citation2022).
The gut microbiome encompasses the total genetic material of all microbes in the gastrointestinal tract. Its genetic diversity and biochemical capacity can surpass the functional capability of the human genome (Qin et al. Citation2010). The gut microbiota forms a complex ecosystem of microorganisms, and its stability and resilience are crucial ecological features for human health (Fassarella et al. Citation2021). The gut ecosystem influences various essential physiological functions, including digestion, nutrient absorption, immune system development, and synthesis of certain vitamins and neurotransmitters, among other critical functions (Hillman et al. Citation2017; Lee et al. Citation2023; Yun and Hyun Citation2023). Nonetheless, the diversity and composition of the gut microbiome can be influenced by diet, genetics, age, and environmental exposures (Hasan and Yang Citation2019; Su and Liu Citation2021), which also includes PM exposure and its association with the GI diseases. In relation to diet, the physical characteristics of the dietary components such as substrate form, dietary fiber, and particle size are important determinants in the colonization of gut bacteria. The attachment of gut bacteria to substrates is preliminary for their utilization. Bacteria have variable attachment abilities to the same dietary fiber or particles (Zhang Citation2022). In the gut, PM can serve as a substrate for microbial growth, thereby altering the composition of gut microbiota. Moreover, the inflammatory response triggered by PM in the lungs can have downstream effects on gut microbiota, promoting the growth of specific microorganisms. The indirect consequences of PM exposure, including systemic inflammation and oxidative stress, can impact gut permeability, mucus production, and immune function. These effects can, in turn influence the gut microbial diversity (Salim et al. Citation2014; Gupta et al. Citation2022).
Physical activity and exercise have the potential to enrich the gut microbiota and enhance the Bacteroidetes/Firmicutes ratio. This in turn, may contribute to weight reduction, a lowered risk of obesity-related health issues, and fewer GI complications. Furthermore, exercise can stimulate the proliferation of beneficia bacteria by influencing mucosal immunity and improving barrier functions. This can reduce the incidence of metabolic diseases and promote the growth of short-chain fatty acid (SCFA)-producing bacteria, thereby safeguarding against GI disorders and certain cancers (Monda et al. Citation2017). Exercise can independently impact the composition and functional capacity of gut microbiota (Mailing et al. Citation2019). The connection between the gut microbiome and exercise carries substantial implications for athletes, and potential mechanisms are discussed here (Wegierska et al. Citation2022). Recent research conducted on overweight women unveiled the potential of aerobic exercise to induce metabolic shifts that provide substrates for beneficial gut microbiota, such as Akkermansia (Hintikka et al. Citation2023). Additionally, a recent systematic review concluded that moderate-to high-intensity exercise (30–90 min) performed at least three times per week for more than 8 weeks can effectively alter the gut microbiota in both clinical and healthy individuals (Boytar et al. Citation2023).
The combined effect of PM and exercise has been studied. A study examining all-cause mortality rates in older adults found that moderate physical activity was linked with delayed mortality; however, vigorous physical activity was associated with accelerated mortality in older adults when exposed to high levels of PM 10 (Park et al. Citation2023). Moreover, the combined effect of reduced physical activity and PM 2.5 exposure can accelerate the onset and progression of type 2 diabetes (T2D) and mood disorders (Luo et al. Citation2023). A recent Chinese cohort study revealed that prolonged higher ambient exposure to PM 2.5 can significantly attenuate the effects of increased physical activity-induced cardiovascular benefits. Additionally, farmers exposed to 54 μg/m3 or higher concentrations of PM 2.5 along with increased active farming and commuting were at a higher risk of developing cerebrovascular disease (Sun et al. Citation2023).
Several studies have elaborated on the role of physical exercise and exposure to PM in combination with the gut microbiota or on the combination of the effects of PM exposure and physical activity in the context of the risk of developing diseases. However, the combined effects of aerobic exercise and exposure to PM on the alteration of gut microbiota and the protective effects of exercise in relation to PM exposure and gut microbiota outcomes are limited. We aimed to determine the changes in the composition and diversity of the gut microbiota over a period of 12 weeks with treadmill exercise and PM exposure (simultaneous, non-simultaneous) interventions in a mouse model.
2. Materials and methods
2.1. Animal care
Four-week-old female C57BL/6J mice were randomly separated into five groups: Con, control group (n = 5); PM, particulate matter exposure (n = 5); PME, PM exposure with concurrent treadmill exercise (n = 5); EXE, the treadmill exercise group (n = 5); EXE + 3-day PM, 5 exercise group followed by 3-day exposure to PM (n = 5). The mice were housed in sterile cages at a controlled temperature (22 ± 2 °C), relative humidity (50 ± 10%), and standard light–dark cycle (12:12), with free access to chow food (fat 4%; rodent NIH-31 open formula auto diet; Zeigler Feed) and water. Mice were supplied by Koatech (South Korea). Authorization for performing animal experiments was granted by the Inha University Ethics Committee (approval number: INHA 220203-811).
2.2. Treadmill exercise and PM exposure
The treadmill exercise protocol spanned 12 weeks, and PM exposure was performed for either 3 or 12 weeks. – A custom-made PM treadmill chamber (Korea patent registration No. 10-2529955 and 10-2529956) consisting of air circulation, PM supply, mouse treadmill, and a central control unit (So et al. Citation2021) was utilized for PM exposure and treadmill exercise (). The PM used in this study was ISO 12103-1 (Powder Technology Inc., U.S.), and the concentration was maintained above 100 μg/m3 (So et al. Citation2021). The PM concentration in the chamber was maintained by continuous monitoring and measurement using an AeroTrak 9306-V2 particle counter (TSI Inc., MN, U.S.). The EXE, EXE + 3-day PM, and PME groups underwent 1 h of treadmill running per day at an intensity of 20 m/min with a 5-degree uphill incline, following 1 week of acclimatization to treadmill exercise.
2.3. Fecal collection and DNA extraction
Feces were collected after 12 weeks of intervention. The animals were restrained in sterile containers to collect fecal pellets, which were snap-frozen and stored at −80 °C, until further processing. DNA was extracted from ∼100 mg of thawed fecal material and homogenized in a Fast-Prep 24 (MP Biomedicals, Irvine, CA, USA) using the SPINeasy DNA Kit for Feces (MP Biomedicals). The quality of the extracted DNA was analyzed by electrophoresis using a 1% agarose gel and visualizing it using a ChemiDoc imaging system (Bio-Rad, Hercules, CA, USA). Further, quality metrics were analyzed using a SpectraMax iD3 spectrophotometer (Molecular Devices, San Jose, CA, USA), and DNA was quantified using Qubit 4 (Thermo Fisher Scientific, Waltham, MA, USA).
2.4. 16S rDNA sequencing and library preparation
The bacterial 16S rRNA gene was amplified by a two-step PCR amplification protocol. Briefly, in the first PCR run, the V4 region was amplified using 515F and 806R primers (F-primer: 5′-TCG TCG GCA GCG TCA GAT GTG TAT AAG AGA CAG GTG CCA GCM GCC GCG GTA A-3′ and R-primer:5′- GTC TCG TGG GCT CGG AGA TGT GTA TAA GAG ACA G-3′) and 2X KAPA Hifi HotStart ReadyMix (KAPA Biosystems, UK). The second PCR resulted in the attachment of dual indices and sequencing adapters, and the Nextera XT Index Kit v2 (Illumina, San Diego, CA, USA) was used to generate libraries. AMPure XP beads (Beckman Coulter, USA) were used for PCR product clean-up. PCR thermal cycling conditions were as described in our previous study (Yun et al. Citation2022). The purified libraries were pooled in equimolar concentrations, followed by paired-end sequencing on an iSeq 100 Illumina platform (150 cycles) at a targeted depth of 1.2 Gb.
2.5. Bioinformatics analysis
Raw reads were imported into QIIME 2 v2022.02.01 (Bolyen et al. Citation2019) using the paired-end format and inspected for quality control by generating quality plots in FastQC. Primers were trimmed using Cutadapt v4.0 (Martin Citation2011). The joining of paired-end reads yielded insufficient merged reads; therefore, further analysis was carried out with forward reads. Denoising of reads was performed using DADA2 to extract the abundances and representative sequences. The evolutionary relationship was depicted by a SATé-enabled phylogenetic placement (SEPP) (Janssen et al. Citation2018) -based phylogenetic tree via the fragment-insertion method against the Greengenes 13.8 reference database (McDonald et al. Citation2012). The Naive Bayes classifier (Pedregosa et al. Citation2011) was trained using the Greengenes database to extract V4 region sequences to assess the bacterial taxonomy of representative reads. The samples were rarified to a minimum library size (27450). The QIIME 2 output files were exported and modified to match the input criteria for use with MicrobiomeAnalyst (Chong et al. Citation2020) for data visualization and further analysis. Alpha diversity was estimated using the observed features and ACE, Shannon, and Fisher metrics at the feature level using filtered reads. Comparisons of intervention groups were performed using the Kruskal–Wallis post-hoc test with false discovery rate (FDR)-corrected p-values. Beta diversity was analyzed at the feature level using the Bray–Curtis metric and confirmed via analysis of similarities (ANOSIM). The composition of gut microbial samples was analyzed at the phylum, family, genus, and species levels to highlight the biomarker taxa, and statistical significance was calculated using two-way analysis of variance (ANOVA) and Tukey’s multiple comparison tests. Differentially abundant taxa were examined using the linear discriminant analysis (LDA) effect size (LEfSe) algorithm. A multiple linear regression model was employed for the association of PM exposure duration with microbial taxa after adjusting for covariates. The significance threshold was set at p < 0.05.
3. Results
The bacterial structure and composition of the mouse gut microbiota were characterized via 16S rRNA gene sequencing analysis of samples exposed to PM and exercise interventions.
3.1. Bacterial diversity and community structure
Alpha diversity was measured using metrics of richness by considering the unique features (observed) and low-abundance features (ACE), whereas the diversity within samples was estimated using the Shannon and Fisher metrics, which account for both richness and evenness. shows that the richness of samples in the EXE + 3-day PM, PM, and PME intervention groups was significantly decreased compared to that in the Con group (p = 0.02) when estimated using the observed feature and ACE metrics. However, alpha diversity was higher in the PME group than in the Con and EXE groups (p = 0.03), as estimated using the Shannon metric. The diversity derived from the Fisher metric was similar to the richness estimates, where the Con group showed greater diversity than the EXE + 3-day PM, PM, and PME groups (p = 0.02) ().
Figure 2. Alpha-diversity boxplots illustrating the feature richness (observed features and ACE metrics) and diversity (Shannon and Fisher metrics) using filtered data. Post-hoc pairwise comparisons were made using the Kruskal–Wallis test. p-values were adjusted based on the Benjamini–Hochberg procedure or false discovery rate (FDR) correction.
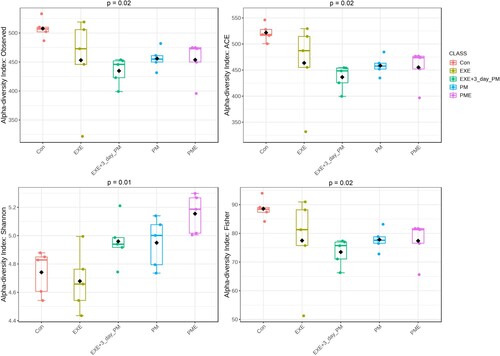
Microbial communities were compared using the Bray–Curtis dissimilarity distance method. The feature-level taxonomic variations among the intervention groups showed significant separation along the axes, where axis 1 accounted for 22.6% of the total variance, as revealed via ANOSIM (). Each group clustered significantly differently than the other groups, explaining the dissimilarity between them.
Figure 3. Principal coordinate analysis (PCoA) of the intervention groups using the Bray–Curtis distance metric based on the feature level. Individual samples are represented by the data points. The values along the axes show the percentage of total variance explained by each axis. Statistical significance was determined using analysis of similarities (ANOSIM) (R: 0.71696; p < 0.001).
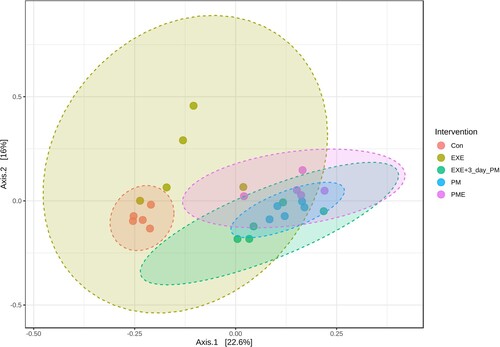
3.2. Gut bacterial abundance profiling
Nine bacterial phyla, including two dominant inhabitant phyla (Bacteroidetes and Firmicutes), were identified in the mouse gut (). The relative proportion of Bacteroidetes significantly decreased by 1.3-fold in all the intervention groups, including EXE, PM, and PME groups (p < 0.0001), and by 1.1-fold in the EXE + 3 d PM group (p = 0.03), compared to that in the Con group. Alternatively, the abundance of Bacteroidetes was relatively higher in the EXE + 3 d PM group than in the EXE (p = 0.008), PM (p = 0.004), and PME (p = 0.0009) groups (). The compositional variation in Bacteroidetes was compensated by the change in the relative abundance of Firmicutes. The relative proportion of Firmicutes was higher in all intervention groups (EXE, PM, and PME; p < 0.0001, EXE + 3-day PM; p = 0.008) than in the Con group. However, the EXE + 3-day PM group had a lower percentage of Firmicutes than the EXE (p = 0.0004), PM (p = 0.006), and PME (p = 0.0001) groups (). No differences were observed in the phylum-level taxonomy among the remaining phyla.
Figure 4. Relative taxonomic abundance profiling of mouse gut microbiota across the experimental groups. The graph illustrates phylum level taxonomic profiling (top) and family level relative abundance (bottom). Statistical significances were calculated using two-way ANOVA and Tukey’s multiple comparison tests.
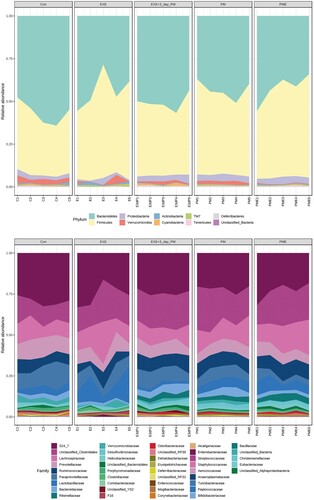
Taxonomic abundance at the family level was also analyzed. Lachnospiraceae family (Firmicutes) abundance was significantly higher in the EXE group than in the Con group (p = 0.003; ). Moreover, the members of this family also expanded in the PME group compared to that in the PM (p = 0.04) and Con (p < 0.0001) groups. However, EXE + 3-day PM had significantly lower proportions of Lachnospiraceae than did EXE (p < 0.0001), PM (p = 0.01), and PME (p < 0.0001). No differences were observed between the Con, PM, and EXE + 3-day PM groups. Lactobacillaceae family (Firmicutes) abundance was not significantly different among the Con, EXE, and PM intervention groups. However, the relative abundance of Lactobacillaceae was higher in EXE + 3-day PM than in Con (p = 0.004). Similarly, compared to that in the Con group, the PME group showed an increased abundance of this family (p = 0.04; ).
Paraprevotellaceae family (Bacteroidetes) members were the most abundant in the Con group compared to that in the remaining groups—EXE (p = 0.01), EXE + 3-day PM (p = 0.001), PM, and PME (p < 0.0001). Its proportion was significantly lower in the PME group than in the EXE (p < 0.0001) and EXE + 3-day PM (p = 0.0004) groups. Prevotellaceae family (Bacteroidetes) members showed a similar trend to Paraprevotellaceae, where EXE (p = 0.01), EXE + 3-day PM (p = 0.009), and PM and PME (p = 0.001) showed shrinkage of the taxa compared to that in Con. Furthermore, members of the Rikenellaceae family (Bacteroidetes) were less abundant in PME than in Con (p = 0.002) and EXE (p = 0.04). The predominant family of mouse gut microbiota, S24_7, now called Muribaculaceae, belongs to the phylum Bacteroidetes. Its abundance was decreased in all experimental groups—EXE (p = 0.001), EXE + 3-day PM, PM, and PME (p < 0.0001)—compared to that in the Con group. Moreover, the relative proportion of S24_7 was lower in the PM group than in the EXE group (p = 0.001; ).
3.2.1. Genus or species level composition
Approximately 40% of bacterial taxa remained unclassified at the genus or species level. Akkermansia muciniphila abundance was not significantly different between the groups, except for Con and EXE + 3-day PM (p = 0.01), and Con and PME (p < 0.0001), although the proportion of A. muciniphila was higher in the Con group (). Lactobacillus salivarius was more abundant in the EXE (p = 0.0004) and PM (p = 0.01) groups than in the Con group. However, its proportion was lower in the EXE + 3-day PM group than in the EXE (p = 0.0002) and PM (p = 0.008) groups. Another Lactobacillus sp. was significantly expanded in the EXE group compared to that in all other intervention groups (p < 0.0001). By contrast, the EXE + 3-day PM group had the lowest abundance among all groups: Con (p = 0.03), PM (p = 0.02), and PME (p < 0.0001). Bacteroides abundance was higher in the PM (p = 0.009) and EXE + 3-day PM (p < 0.0001) groups than in the EXE group. Moreover, EXE + 3-day PM had a higher relative proportion of Bacteroides than did the Con (p = 0.005) and PME (p = 0.001) groups. Oscillospira proportions were similar in the PM, PME, and EXE + 3-day PM groups. In addition, its proportion was similar in the control and EXE groups. However, the PM, PME, and EXE + 3-day PM intervention groups had a higher proportion of Oscillospira than did the Con (p < 0.007) and EXE (p < 0.0003) groups (). Paraprevotella abundance was higher in the Con group than in all the other groups (p < 0.0001). However, its relative abundance was lowest in the PME group (p < 0.0001). Notably, the EXE + 3-day PM group had a higher percentage of Paraprevotella than did the PM group (p = 0.02), similar to the EXE group. Prevotella was found to have a greater relative abundance in the Con group than in the other intervention groups (P < 0.0001; ).
3.2.2. Biomarker bacterial taxa
The statistically differential bacterial taxa abundance was analyzed by performing LEfSe, demonstrating 26 bacterial taxa that significantly surpassed the LDA score of > 2 (). Paraprevotella, A. muciniphila, Corynebacterium, RF32, Parabacteroides, Butyricimonas, Turibacter, Facklamia, Aerococcaceae, SMB53, Bacillaceae, and Sporosarcina were enriched in the control group. Lactobacillus, Coriobacteraceae, and Anaerofustis were enriched in the EXE group. The EXE + 3-day PM group showed enrichment for Bacteroides, Bacteroidales, Clostridiaceae, and Dorea. Desulfovibrionaceae, Mucispirillum schaedleri, Lactococcus, and Anaeroplasma were highly enriched in the PM group, whereas Rikenellaceae, Ruminococcus flavefaciens, and AF12 were enriched in the PME group ().
Figure 6. Differentially abundant taxa across intervention groups estimated using the LEfSe algorithm. The bacterial taxa illustrated an LDA > 2 and a significance threshold of 0.05.
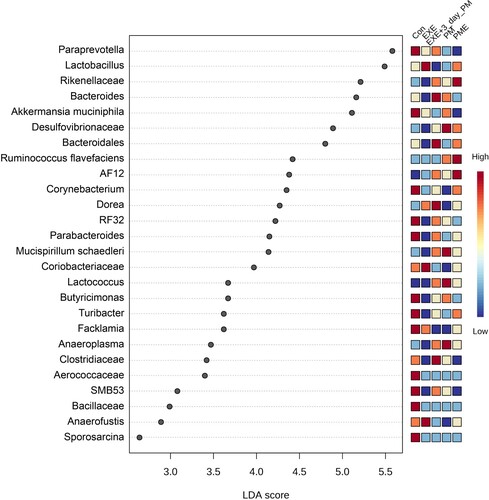
The bacterial taxa significantly associated with PM exposure duration are presented in . A multiple linear regression model was used to determine the association between gut bacteria and PM exposure duration (12 weeks, 3 days, non-PM). The confounding variable exercise was controlled to identify significant bacterial taxa. Paraprevotella was significantly reduced in the 12-week PM group, whereas Bacteroides abundance was significantly increased in the 3-day PM (p = 0.02) and 12-week PM groups (p = 0.01) compared with that in the non-PM group. Moreover, the 12-week PM group had a lower abundance of Paraprevotella (p = 0.04) and Bacteroides (p = 0.01) than that in the 3-day PM group (). Bacteroides uniformis (p = 0.03), SMB53 (p = 0.02), and Staphylococcus (p = 0.04) decreased in the PM exposure groups (3-day and 12-week) compared with that in the non-PM exposure groups. Lactobacillus reuteri (p = 0.02), L. salivarius (p = 0.03), Lactobacillus sp. (p = 0.004), and Ruminococcus gnavus (p = 0.03) were present in lower proportions in the 3-day PM exposure group than that in the non-PM exposure group (). Flexispira (p = 0.04) and Lactococcus were significantly more abundant in the PM exposure groups (3-day and 12-week PM) than that in the control non-PM exposure group. Blautia was higher in the 3-day PM exposure group than that in the 12-week PM exposure group (p = 0.03), and the 12-week PM exposure group had a lower abundance than that in the non-PM group (p = 0.03). Butyricimonas abundance was associated with PM exposure, whereas the 12-week PM exposure showed reduced proportions compared with that in the 3-day PM exposure (p = 0.03). Parabacteroides distasonis was significantly reduced after 12-week PM exposure compared with that in the 3-day PM exposure group (p = 0.04) ().
Figure 7. Association of gut microbial species with PM exposure duration following adjustment of exercise covariate using multiple linear regression model. The log-transformed counts of adjusted significantly altered bacterial genera/species are displayed on the Y-axis, with a p-value cut-off of 0.05 (FDR-corrected).
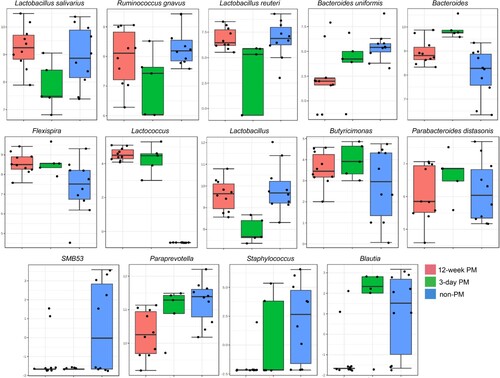
4. Discussion
This study aimed to identify alterations in the gut microbiota composition and diversity during a 12-week period of treadmill exercise and PM exposure. Physical activity and cardiorespiratory fitness are positively linked to increased alpha diversity in fecal bacteria (Ortiz-Alvarez et al. Citation2020). Moderate endurance exercise can contribute to human health by reducing inflammation and improving body composition as well as gut microbial diversity. Elite athletes possess high gut microbial diversity and are enriched in amino acid biosynthesis and carbohydrate and fiber metabolism-related bacterial species, consequently producing key SCFAs (Clauss et al. Citation2021; Imdad et al. Citation2022). Exercise intervention did not influence the alpha and beta diversities of the human gut microbiota but had an impact on SCFA producers (Motiani et al. Citation2020; Bycura et al. Citation2021; Moitinho-Silva et al. Citation2021). Our findings did not reveal significant differences in alpha diversity between the control and exercise intervention groups. However, the beta-diversity (Bray–Curtis) of the gut microbiota significantly differed between the Con and EXE groups, as evidenced by separate clustering (). PM, a study variable that can modulate the gut microbial diversity. Its exposure can cause gut microbial dysbiosis (Ran et al. Citation2021) and promote cardiovascular disease (CVD) (Chen et al. Citation2019). A recent study reported an alteration of microbial profiles in the small intestine by exposure to diesel exhaust particles, which can lead to systemic inflammation and early CVD biomarkers (Phillippi et al. Citation2022). Our findings revealed that PM exposure decreased the alpha diversity (Fisher) and richness (observed features and ACE) of the gut microbiota in mice, irrespective of exposure duration (3 days or 12 weeks) and exercise intervention (). Beta-diversity analysis of the gut microbiota indicated that the intervention groups were significantly dissimilar from each other, as estimated using the Bray–Curtis metric ().
Numerous investigations have identified adverse links between exposure to air pollution and indices related to the diversity, evenness, and richness of the intestinal microbiome. PM exposure can elevate colonic immune cell infiltration, triggering heightened inflammatory responses through intestinal tissue damage, and concurrently inducing alterations in the gut microbial community through the initiation of oxidative stress. Bacteroidetes to Firmicutes ratio and the richness of the gut microflora were significantly reduced after PM 2.5 exposure, according to a recent study using mice (Dai et al. Citation2022). Across various studies, exposure to air pollution exhibited negative association with taxa from Acidobacteria, Cyanobacteria, Deferribacteres, Fibrobacteres, Gemmatimonodota, Lentisphaerae, Spirochaetes, Synergistetes Thermodesulfobacteriota, and Verrucomicrobia, while showing positive associations with taxa from Bdellovibrionales, Deltaproteobacteria, Elusimicrobiota, and Fusobacteria. Similarly, our results revealed a reduction in the abundance of Bacteroidetes in the PM exposure groups and increased abundance of Firmicutes compared with that in the Con group (). A recent cohort study from China corroborates our data, confirming the association of reduced Bacteroidetes abundance with PM 2.5 constituents revealed by weighted correlation analysis. The authors demonstrated that long-term PM 2.5 exposure, specifically exposure to black carbon, is associated with modulation in gut microbiota; therefore, this should be monitored to mitigate its adverse effects (Li et al. Citation2023) including inflammation, oxidative stress, and gut damage and permeability, on gut microbiota (Van Pee et al. Citation2023).
The Lachnospiraceae family was highly abundant in the exercise (EXE) group, compared to the case for the Con group, and in the PME group, compared to the case for the PM alone group (). A recent report demonstrated that aerobic exercise (3 months) together with a Mediterranean diet (low glycemic index) led to an increased abundance of species from Lachnospiraceae family and some other species in subjects with nonalcoholic fatty liver disease (NAFLD), in comparison to the diet and exercise alone groups (Calabrese et al. Citation2022). The present study highlights the role of aerobic exercise with a stronger covariate diet (Yun et al. Citation2022) in the expansion of Lachnospiraceae. However, our data were gathered from healthy mice; therefore, exercise alone with a normal chow diet showed the same effects even in the presence of PM exposure. The bacterial families Lachnospiraceae and Ruminococcaceae (from the phylum Firmicutes) are known for their butyrate-producing bacteria, the abundances of which are significantly reduced in patients with T2D, according to clinical evidence (James et al. Citation2022). A recent study demonstrated that voluntary and endurance exercise capacity is driven by members of Lachnospiraceae family, and these intestinal microbes appear to act via the gut–brain axis (Thaiss Citation2023). Intense physical activity stimulates a dopamine release surge in the brain striatum in the presence of the microbiome (Friend et al. Citation2017). This can be explained by the crosstalk between the microbiome and the brain through microbiome-derived molecules in systemic circulation or via direct neurotransmitter-based connections between the gut and the brain (Agirman et al. Citation2021).
Bacteroides, a genus from the phylum Bacteroidetes, was found to be less abundant in male C57BL/6 mice after 14 weeks of aerobic exercise compared to the case in the control mice (Yang et al. Citation2021). In agreement with a previous study, our analysis showed a reduction in the abundance of Bacteroides in the EXE group compared to that in the Con group. Moreover, the effects of exercise were sustained in the PME group, which showed a lower abundance of members from Bacteroides than the PM group ( and ). Cigarette smoking has been associated with increased abundances of members from Proteobacteria and Bacteroides spp. in the gut; however, smoking cessation has been shown to decrease its abundance (Biedermann et al. Citation2014). Moreover, an increased abundance of members from Bacteroides is independently associated with higher levels of lipopolysaccharides (LPSs) and lower insulin sensitivity in T2D patients than those in non-T2D controls; this might be linked to gut barrier function (Wang et al. Citation2020). A study showed that strength training exercise for four weeks was associated with a higher abundance of members from Dorea and other bacterial spp. than that in healthy controls (Chen et al. Citation2021). The genus Dorea, classified within the family Lachnospiraceae, possesses the capacity to ferment glucose and other sugars, resulting in the production of ethanol, formic acid, and acetic acid. The functional role of this genus can exhibit either pro-inflammatory or anti-inflammatory characteristics, contingent upon the specific composition of the surrounding gut bacteria (Shahi et al. Citation2017). Furthermore, Lactobacilli protect the intestinal barrier from infections by promoting mucus production and synthesizing barrier-related proteins. They release antimicrobial substances such as short-chain fatty acids (SCFAs), bacteriocins, and hydrogen peroxide to inhibit the growth or eliminate pathogens. Moreover, Lactobacilli modulate the host's immune response to pathogens (Dempsey and Corr Citation2022). Our data showed that Lactobacillus salivarius, another species from the genus Lactobacillus, and members from the genus Dorea were enriched in the EXE group compared to the case for the Con group; further, 12 weeks of PM exposure in combination with exercise (PME) was associated with a higher abundance of members from Dorea and Lactobacillus sp. than PM exposure alone ( and ).
The abundance of members from the genus Butyricimonas was lower in the EXE and PME groups than in the Con and PM groups, respectively (), showing an association of Butyricimonas with PM exposure (). A study reported that Microplastics (MPs) can adsorb and transport toxic phthalate esters (PAEs) into the mouse gut, which can increase intestinal permeability and induce inflammation, compared to the case for exposure to MPs and phthalate esters alone. The same study revealed the relative abundance of members from Butyricimonas in the mouse gut after exposure to MPs and phthalate-contaminated MPs (Deng et al. Citation2020), corroborating our data. Members from the Butyricimonas produce butyrate, a short-chain fatty acid with anti-inflammatory and anti-oxidative properties (Fu et al. Citation2019). We hypothesized that the abundance of Butyricimonas after exposure to either PM or MPs was able to cope with increasing stress. PM is known to induce the expression of biomarkers of oxidative stress. A metabolomics analysis revealed that higher exposure to PM (2.5 µm) led to a significant elevation in the levels of stress hormones, such as cortisol, cortisone, epinephrine, and norepinephrine (Li et al. Citation2017), which may indirectly contribute to oxidative stress.
4.1. Conclusions
Alpha and beta diversity are influenced by the interventions compared to the case for the control group (no interventions). However, exercise did not yield significant alpha diversity variations in the gut microbiota. Interestingly, members from Lachnospiraceae were associated with exercise intervention in the absence and presence of PM exposure. The biomarker taxa Lactobacillus, Coriobacteriaceae, and Anaerofustis were enriched in the EXE group, while Desulfovibrionaceae, Mucispirillum schaedleri, Lactococcus, and Anaeroplasma were highly enriched in the PM group. The PM exposure (3-day and 12-week) groups showed a reduced relative abundance of Bacteroides uniformis, SMB53, and Staphylococcus compared to the non-PM exposure groups. The gut microbiota can interact with the host system, particularly by means of microbiota-derived metabolites. However, many of these interactions and the mechanisms underlying these interactions remain unknown. Filling this knowledge gap can advance our understanding of the etiological properties of gut microbial taxa with regard to certain diseases associated with PM exposure. Furthermore, our findings underscore the beneficial effects of exercise, which not only enhances the athletic abilities of the body but also boosts physiological functions for overall human health.
Additional information
Disclosure statement
No potential conflict of interest was reported by the author(s).
Additional information
Funding
References
- Agirman G, Yu KB, Hsiao EY. 2021. Signaling inflammation across the gut-brain axis. Science (New York, NY). 374:1087–1092. doi: 10.1126/science.abi6087.
- Albano GD, Montalbano AM, Gagliardo R, Anzalone G, Profita M. 2022. Impact of air pollution in airway diseases: role of the epithelial cells (Cell models and biomarkers). Int J Mol Sci. 3:23. doi: 10.3390/ijms23052799.
- Anderson JO, Thundiyil JG, Stolbach A. 2012. Clearing the air: a review of the effects of particulate matter air pollution on human health. J Med Toxicol. 8:166–175. doi: 10.1007/s13181-011-0203-1.
- Arias-Pérez RD, Taborda NA, Gómez DM, Narvaez JF, Porras J, Hernandez JC. 2020. Inflammatory effects of particulate matter air pollution. Environ Sci Pollut Res Int. 27:42390–42404. doi: 10.1007/s11356-020-10574-w.
- Basith S, Manavalan B, Shin TH, Park CB, Lee WS, Kim J, Lee G. 2022. The impact of fine particulate matter 2.5 on the cardiovascular system: a review of the invisible killer. Nanomaterials. 12:2656. doi: 10.3390/nano12152656.
- Biedermann L, Brülisauer K, Zeitz J, Frei P, Scharl M, Vavricka SR, Fried M, Loessner MJ, Rogler G, Schuppler M. 2014. Smoking cessation alters intestinal microbiota: insights from quantitative investigations on human fecal samples using FISH. Inflamm Bowel Dis. 20:1496–1501.doi: 10.1097/MIB.0000000000000129.
- Bolyen E, Rideout JR, Dillon MR, Bokulich NA, Abnet CC, Al-Ghalith GA, Alexander H, Alm EJ, Arumugam M, Asnicar F, et al. 2019. Reproducible, interactive, scalable and extensible microbiome data science using QIIME 2. Nat Biotechnol. 37:852–857. doi: 10.1038/s41587-019-0209-9.
- Boytar AN, Skinner TL, Wallen RE, Jenkins DG, Dekker Nitert M. 2023. The effect of exercise prescription on the human gut microbiota and comparison between clinical and apparently healthy populations: A systematic review. Nutrients. 15:1534. doi: 10.3390/nu15061534.
- Bycura D, Santos AC, Shiffer A, Kyman S, Winfree K, Sutliffe J, Pearson T, Sonderegger D, Cope E, Caporaso JG. 2021. Impact of different exercise modalities on the human Gut microbiome. Medicine & Science in Sports & Exercise. 53:230. doi: 10.3390/sports9020014.
- Calabrese FM, Disciglio V, Franco I, Sorino P, Bonfiglio C, Bianco A, Campanella A, Lippolis T, Pesole PL, Polignano M, et al. 2022. A Low glycemic index Mediterranean diet combined with aerobic physical activity rearranges the Gut microbiota signature in NAFLD patients. Nutrients. 14:1773. doi: 10.3390/nu14091773.
- Chen D, Xiao C, Jin H, Yang B, Niu J, Yan S, Sun Y, Zhou Y, Wang X. 2019. Exposure to atmospheric pollutants is associated with alterations of gut microbiota in spontaneously hypertensive rats. Exp Ther Med. 18:3484–3492. doi: 10.3892/etm.2019.7934.
- Chen H, Shen L, Liu Y, Ma X, Long L, Ma X, Ma L, Chen Z, Lin X, Si L, et al. 2021. Strength exercise confers protection in central nervous system autoimmunity by altering the Gut microbiota. Front Immunol. 12:628629. doi: 10.3389/fimmu.2021.628629.
- Chong J, Liu P, Zhou G, Xia J. 2020. Using microbiomeanalyst for comprehensive statistical, functional, and meta-analysis of microbiome data. Nature Protocols. 15:799–821. doi: 10.1038/s41596-019-0264-1.
- Clauss M, Gérard P, Mosca A, Leclerc M. 2021. Interplay between exercise and gut microbiome in the context of human health and performance. Front Nutr. 8:637010. doi: 10.3389/fnut.2021.637010.
- Collaborators G, Ärnlöv J. 2020. Global burden of 87 risk factors in 204 countries and territories, 1990–2019: a systematic analysis for the global burden of disease study 2019. The Lancet. 396:1223–1249. doi: 10.1016/S0140-6736(20)30752-2.
- Dai S, Wang Z, Yang Y, Du P, Li X. 2022. PM2.5 induced weight loss of mice through altering the intestinal microenvironment: mucus barrier, gut microbiota, and metabolic profiling. J Hazard Mater. 431:128653. doi: 10.1016/j.jhazmat.2022.128653.
- Dempsey E, Corr SC. 2022. Lactobacillus spp. for gastrointestinal health: current and future perspectives. Front Immunol. 13:840245. doi: 10.3389/fimmu.2022.840245.
- Deng Y, Yan Z, Shen R, Wang M, Huang Y, Ren H, Zhang Y, Lemos B. 2020. Microplastics release phthalate esters and cause aggravated adverse effects in the mouse gut. Environ Int. 143:105916. doi: 10.1016/j.envint.2020.105916.
- Fassarella M, Blaak EE, Penders J, Nauta A, Smidt H, Zoetendal EG. 2021. Gut microbiome stability and resilience: elucidating the response to perturbations in order to modulate gut health. Gut. 70:595–605. doi: 10.1136/gutjnl-2020-321747.
- Friend DM, Devarakonda K, O'Neal TJ, Skirzewski M, Papazoglou I, Kaplan AR, Liow JS, Guo J, Rane SG, Rubinstein M, et al. 2017. Basal Ganglia dysfunction contributes to physical inactivity in obesity. Cell Metabolism. 25:312–321. doi: 10.1016/j.cmet.2016.12.001.
- Fu X, Liu Z, Zhu C, Mou H, Kong Q. 2019. Nondigestible carbohydrates, butyrate, and butyrate-producing bacteria. Crit Rev Food Sci Nutr. 59:S130–s152. doi: 10.1080/10408398.2018.1542587.
- Gupta N, Yadav VK, Gacem A, Al-Dossari M, Yadav KK, Abd El-Gawaad NS, Ben Khedher N, Choudhary N, Kumar P, Cavalu S. 2022. Deleterious effect of Air pollution on human microbial community and bacterial flora: A short review. Int J Environ Res Public Health. 19:15494. doi: 10.3390/ijerph192315494.
- Hamanaka RB, Mutlu GM. 2018. Particulate matter Air pollution: effects on the cardiovascular system. Front Endocrinol (Lausanne). 9:680. doi: 10.3389/fendo.2018.00680.
- Hasan N, Yang H. 2019. Factors affecting the composition of the gut microbiota, and its modulation. PeerJ. 7:e7502. doi: 10.7717/peerj.7502.
- Hillman ET, Lu H, Yao T, Nakatsu CH. 2017. Microbial ecology along the gastrointestinal tract. Microbes Environ. 32:300–313. doi: 10.1264/jsme2.ME17017.
- Hintikka JE, Ahtiainen JP, Permi P, Jalkanen S, Lehtonen M, Pekkala S. 2023. Aerobic exercise training and gut microbiome-associated metabolic shifts in women with overweight: a multi-omic study. Sci Rep. 13:11228. doi: 10.1038/s41598-023-38357-6.
- Imdad S, Lim W, Kim JH, Kang C. 2022. Intertwined relationship of mitochondrial metabolism, gut microbiome and exercise potential. Int J Mol Sci. 23:2679. doi: 10.3390/ijms23052679.
- James MM, Pal N, Sharma P, Kumawat M, Shubham S, Verma V, Tiwari RR, Singh B, Nagpal R, Sarma DK, et al. 2022. Role of butyrogenic firmicutes in type-2 diabetes. J Diabetes Metab Disord. 21:1873–1882. doi: 10.1007/s40200-022-01081-5.
- Janssen S, McDonald D, Gonzalez A, Navas-Molina JA, Jiang L, Xu ZZ, Winker K, Kado DM, Orwoll E, Manary M, et al. 2018. Phylogenetic placement of exact amplicon sequences improves associations with clinical information. mSystems. 3:10. doi: 10.1128/mSystems.00021-18.
- Ji JS. 2022. Air pollution and cardiovascular disease onset: hours, days, or years? Lancet Public Health. e890–e891. doi: 10.1016/S2468-2667(22)00257-2.
- Lee J, Song X, Hyun B, Jeon CO, Hyun S. 2023. Drosophila gut immune pathway suppresses host development-promoting effects of acetic acid bacteria. Mol Cells. 46:637. doi: 10.14348/molcells.2023.0141.
- Lee JY, Lee SM, Lee WK, Park JY, Kim DS. 2023. NAA10 hypomethylation is associated with particulate matter exposure and worse prognosis for patients with non-small cell lung cancer. Anim Cells Syst (Seoul). 27:72–82. doi: 10.1080/19768354.2023.2189934.
- Leikauf GD, Kim SH, Jang AS. 2020. Mechanisms of ultrafine particle-induced respiratory health effects. Exp Mol Med. 52:329–337. doi: 10.1038/s12276-020-0394-0.
- Li H, Cai J, Chen R, Zhao Z, Ying Z, Wang L, Chen J, Hao K, Kinney PL, Chen H, et al. 2017. Particulate matter exposure and stress hormone levels: a randomized, double-blind, crossover trial of Air purification. Circulation. 136:618–627. doi: 10.1161/CIRCULATIONAHA.116.026796.
- Li S, Guo B, Dong K, Huang S, Wu J, Zhou H, Wu K, Han X, Liang X, Pei X, et al. 2023. Association of long-term exposure to ambient PM2.5 and its constituents with gut microbiota: evidence from a China cohort. The Sci Total Environ. 884:163577. doi: 10.1016/j.scitotenv.2023.163577.
- Luo H, Huang Y, Zhang Q, Yu K, Xie Y, Meng X, Kan H, Chen R. 2023. Impacts of physical activity and particulate air pollution on the onset, progression and mortality for the comorbidity of type 2 diabetes and mood disorders. The Sci Total Environ. 890:164315. doi: 10.1016/j.scitotenv.2023.164315.
- Mailing LJ, Allen JM, Buford TW, Fields CJ, Woods JA. 2019. Exercise and the gut microbiome: a review of the evidence, potential mechanisms, and implications for human health. Exerc Sport Sci Rev. 47:75–85. doi: 10.1249/JES.0000000000000183.
- Martin M. 2011. Cutadapt removes adapter sequences from high-throughput sequencing reads. EMBnet Journal. 17:10–12. doi: 10.14806/ej.17.1.200.
- McDonald D, Price MN, Goodrich J, Nawrocki EP, DeSantis TZ, Probst A, Andersen GL, Knight R, Hugenholtz P. 2012. An improved greengenes taxonomy with explicit ranks for ecological and evolutionary analyses of bacteria and archaea. ISME J. 6:610–618. doi: 10.1038/ismej.2011.139.
- Misiukiewicz-Stepien P, Paplinska-Goryca M. 2021. Biological effect of PM10 on airway epithelium-focus on obstructive lung diseases. Clin Immunol. 227:108754. doi: 10.1016/j.clim.2021.108754.
- Moitinho-Silva L, Wegener M, May S, Schrinner F, Akhtar A, Boysen TJ, Schaeffer E, Hansen C, Schmidt T, Rühlemann MC, et al. 2021. Short-term physical exercise impacts on the human holobiont obtained by a randomised intervention study. BMC Microbiol. 21:162. doi: 10.1186/s12866-021-02214-1.
- Monda V, Villano I, Messina A, Valenzano A, Esposito T, Moscatelli F, Viggiano A, Cibelli G, Chieffi S, Monda M, et al. 2017. Exercise modifies the gut microbiota with positive health effects. Oxid Med Cell Longevity. 2017:3831972. doi: 10.1155/2017/3831972.
- Motiani KK, Collado MC, Eskelinen JJ, Virtanen KA, Löyttyniemi E, Salminen S, Nuutila P, Kalliokoski KK, Hannukainen JC. 2020. Exercise training modulates Gut microbiota profile and improves Endotoxemia. Med Sci Sports Exercise. 52:94–104. doi: 10.1249/MSS.0000000000002112.
- Mutlu EA, Comba IY, Cho T, Engen PA, Yazıcı C, Soberanes S, Hamanaka RB, Niğdelioğlu R, Meliton AY, Ghio AJ, et al. 2018. Inhalational exposure to particulate matter air pollution alters the composition of the gut microbiome. Environ Pollut. 240:817–830. doi: 10.1016/j.envpol.2018.04.130.
- Organization WH. 2023. WHO ambient air quality database, 2022 update: status report: World Health Organization.
- Ortiz-Alvarez L, Xu H, Martinez-Tellez B. 2020. Influence of exercise on the human gut microbiota of healthy adults: a systematic review. Clin Transl Gastroenterol. 11:e00126. doi: 10.14309/ctg.0000000000000126.
- Pambianchi E, Pecorelli A, Valacchi G. 2022. Gastrointestinal tissue as a “new” target of pollution exposure. IUBMB Life. 74:62–73. doi: 10.1002/iub.2530.
- Park H, Yang PS, Sung JH, Jin MN, Jang E, Yu HT, Kim TH, Pak HN, Lee MH, Joung B. 2023. Association between the combined effects of physical activity intensity and particulate matter and All-cause mortality in older adults. Mayo Clin Proc. 98:1153–1163. doi: 10.1016/j.mayocp.2023.04.017.
- Pedregosa F, Varoquaux G, Gramfort A, Michel V, Thirion B, Grisel O, Blondel M, Prettenhofer P, Weiss R, Dubourg V. 2011. Scikit-learn: machine learning in python. J Mach Learn Res. 12:2825–2830. In.
- Phillippi DT, Daniel S, Pusadkar V, Youngblood VL, Nguyen KN, Azad RK, McFarlin BK, Lund AK. 2022. Inhaled diesel exhaust particles result in microbiome-related systemic inflammation and altered cardiovascular disease biomarkers in C57Bl/6 male mice. Part Fibre Toxicol. 19:10. doi: 10.1186/s12989-022-00452-3.
- Qin J, Li R, Raes J, Arumugam M, Burgdorf KS, Manichanh C, Nielsen T, Pons N, Levenez F, Yamada T, et al. 2010. A human gut microbial gene catalogue established by metagenomic sequencing. Nature. 464:59–65. doi: 10.1038/nature08821.
- Ran Z, An Y, Zhou J, Yang J, Zhang Y, Yang J, Wang L, Li X, Lu D, Zhong J, et al. 2021. Subchronic exposure to concentrated ambient PM2.5 perturbs gut and lung microbiota as well as metabolic profiles in mice. Environ Pollut. 272:115987. doi: 10.1016/j.envpol.2020.115987.
- Salim SY, Kaplan GG, Madsen KL. 2014. Air pollution effects on the gut microbiota: a link between exposure and inflammatory disease. Gut Microbes. 5:215–219. doi: 10.4161/gmic.27251.
- Schraufnagel DE, Balmes JR, Cowl CT, De Matteis S, Jung SH, Mortimer K, Perez-Padilla R, Rice MB, Riojas-Rodriguez H, Sood A, et al. 2019. Air pollution and noncommunicable diseases: a review by the forum of international respiratory societies’ environmental committee, Part 2: air pollution and organ systems. Chest. 155:417–426. doi: 10.1016/j.chest.2018.10.041.
- Shahi SK, Freedman SN, Mangalam AK. 2017. Gut microbiome in multiple sclerosis: the players involved and the roles they play. Gut Microbes. 8:607–615. doi: 10.1080/19490976.2017.1349041.
- Shi L, Zhu Q, Wang Y, Hao H, Zhang H, Schwartz J, Amini H, van Donkelaar A, Martin RV, Steenland K, et al. 2023. Incident dementia and long-term exposure to constituents of fine particle air pollution: a national cohort study in the United States. Proc Natl Acad Sci U S A. 120:e2211282119. doi: 10.1073/pnas.2211282119.
- So B, Park J, Jang J, Lim W, Imdad S, Kang C. 2021. Effect of aerobic exercise on oxidative stress and inflammatory response during particulate matter exposure in mouse lungs. Front Physiol. 12:773539. doi: 10.3389/fphys.2021.773539.
- Su Q, Liu Q. 2021. Factors affecting gut microbiome in daily diet. Front Nutr. 8:644138. doi: 10.3389/fnut.2021.644138.
- Sun D, Liu C, Ding Y, Yu C, Guo Y, Sun D, Pang Y, Pei P, Du H, Yang L, et al. 2023. Long-term exposure to ambient PM2·5, active commuting, and farming activity and cardiovascular disease risk in adults in China: a prospective cohort study. Lancet Planet Health. 7:e304–e312. doi: 10.1016/S2542-5196(23)00047-5.
- Téllez-Rojo MM, Romieu I, Ruiz-Velasco S, Lezana MA, Hernández-Avila MM. 2000. Daily respiratory mortality and PM10 pollution in Mexico city: importance of considering place of death. Eur Respir J. 16:391–396. doi: 10.1034/j.1399-3003.2000.016003391.x.
- Thaiss CA. 2023. A microbiome exercise. Science (New York, NY). 381:38. doi: 10.1126/science.adi6329.
- Tian Y, Zhang L, Wang Y, Song J, Sun H. 2021. Temporal and spatial trends in particulate matter and the responses to meteorological conditions and environmental management in Xi’an, China. Atmosphere. 12:1112. doi: 10.3390/atmos12091112.
- Tschofen P, Azevedo IL, Muller NZ. 2019. Fine particulate matter damages and value added in the US economy. Proc Natl Acad Sci U S A. 116:19857–19862. doi: 10.1073/pnas.1905030116.
- Van Pee T, Nawrot TS, van Leeuwen R, Hogervorst J. 2023. Ambient particulate air pollution and the intestinal microbiome; a systematic review of epidemiological, in vivo and, in vitro studies. The Sci Total Environ. 878:162769. doi: 10.1016/j.scitotenv.2023.162769.
- Vignal C, Pichavant M, Alleman LY, Djouina M, Dingreville F, Perdrix E, Waxin C, Ouali Alami A, Gower-Rousseau C, Desreumaux P, et al. 2017. Effects of urban coarse particles inhalation on oxidative and inflammatory parameters in the mouse lung and colon. Part Fibre Toxicol. 14:46. doi: 10.1186/s12989-017-0227-z.
- Wang J, Li W, Wang C, Wang L, He T, Hu H, Song J, Cui C, Qiao J, Qing L, et al. 2020. Enterotype Bacteroides Is associated with a high risk in patients with diabetes: a pilot study. J Diabetes Res. 2020:6047145. doi: 10.1155/2020/6047145.
- Wegierska AE, Charitos IA, Topi S, Potenza MA, Montagnani M, Santacroce L. 2022. The connection between physical exercise and gut microbiota: implications for competitive sports athletes. Sports Medicine. 52:2355–2369. doi: 10.1007/s40279-022-01696-x.
- Xie S, Zhang C, Zhao J, Li D, Chen J. 2022. Exposure to concentrated ambient PM2.5 (CAPM) induces intestinal disturbance via inflammation and alternation of gut microbiome. Environ Int. 161:107138. doi: 10.1016/j.envint.2022.107138.
- Yang W, Liu Y, Yang G, Meng B, Yi Z, Yang G, Chen M, Hou P, Wang H, Xu X. 2021. Moderate-Intensity physical exercise affects the exercise performance and gut microbiota of mice. Front Cell Infect Microbiol. 11:712381. doi: 10.3389/fcimb.2021.712381.
- Yun E-J, Imdad S, Jang J, Park J, So B, Kim J-H, Kang C. 2022. Diet is a stronger covariate than exercise in determining gut microbial richness and diversity. Nutrients. 14:2507. doi: 10.3390/nu14122507.
- Yun HM, Hyun S. 2023. Role of gut commensal bacteria in juvenile developmental growth of the host: insights from Drosophila studies. Anim Cells Syst (Seoul). 27:329–339. doi: 10.1080/19768354.2023.2282726.
- Zhang P. 2022. Influence of foods and nutrition on the gut microbiome and implications for intestinal health. Int J Mol Sci. 23:9588. doi: 10.3390/ijms23179588.