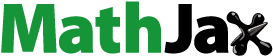
ABSTRACT
The impact of presence of nano-scale particles inside a flow between two flat plates which known as Poiseuille flow is numerically analyzed. Nanofluids have been investigated in the past few years mainly by considering the heat transfer aspect in a fully developed section of internal flows, rather than detailing the hydrodynamic and thermal developing viewpoint of nanofluid. In other words, this research will focus on the fundamental understanding of developing nanoparticles flows in hydrodynamic and thermal boundary layers in the entrance region. Therefore, flow between two flat plates is numerically studied here by injecting and tracking nanoparticles from inlet to outlet of the channel. The flow is kept in the laminar regime with uniform heat flux applied on the plates. ANSYS Fluent 19.3 is used to numerically solve the continuity and momentum equations, as well as discrete phase modeling for particles. The results showed three major stages in vorticity development in the entrance region. It was found that Brownian motion plays an important role in nanoparticles migration, and close to the wall with the involvement of Thermophoresis. Also, nanoparticles can deviate from flow layers after merging boundary layers.
1. Introduction
Using nanoparticles in fluid flows for enhancement of heat transfer has been proposed by many researchers in recent years. Due to higher thermal conductivity (Abadi et al., Citation2020; Ahmadi et al., Citation2020; Alotaibi et al., Citation2020; Aramesh et al., Citation2020; Baghban et al., Citation2019; Loni et al., Citation2018; Maddah et al., Citation2018; Sadeghzadeh et al., Citation2020), nanoparticles are able to manipulate the thermo-physical properties of the base fluid and eventually present a new fluid called nanofluid with the capability of higher rate of heat transfer (Aghayari et al., Citation2020; Ahmadi et al., Citation2019; Ahmadi et al., Citation2019; Ahmadi et al., Citation2019; Aybar et al., Citation2015; Baghban et al., Citation2019; Baghban et al., Citation2019; Ghalandari et al., Citation2019; Ghodsinezhad et al., Citation2016; Ramezanizadeh et al., Citation2019; Ramezanizadeh & Nazari, Citation2019; Sharma et al., Citation2020; Tayebi et al., Citation2019; Tshimanga et al., Citation2016; Williams et al., Citation2008).
Due to modified thermo-physical of the base fluid, it is possible that injection of fine particles in a flow field may change the hydrodynamic aspect of the fluid flow, and importantly the diffusion of boundary layer from a solid wall (M Mahdavi et al., Citation2016). Nonetheless, most of the recent studies have been focused, either experimentally or numerically, on heat transfer parameters or pressure drops in a global scale (M. Mahdavi et al., Citation2017; M. Mahdavi et al., Citation2018; Shoghl et al., Citation2014; Singh et al., Citation2011). There are only a few studies with particulazr attention on the nanoparticles distribution or even their impacts on the parameters inside boundary layer (Avramenko et al., Citation2011; Avramenko et al., Citation2012; Bhushan & Muthu, Citation2019; Bhushan & Walters, Citation2014; Khan & Pop, Citation2010; Pettersson & Rizzi, Citation2009). Baghban et al. (Citation2019) employed similarity solution in combination with symmetry analysis to model turbulent nanofluid with the main focus on major parameters distribution such as temperature, nanoparticles concentration and velocity. They used Buongiorno nanofluid model developed based on mass diffusion of nanoparticles in terms of interaction forces between particles and fluid, with the assumption that nanoparticles can be treated as a continues phase. Due to the strength of turbulent flow compared to the fine size of the particles, they stated that the presence of nanoparticles is negligible or impossible inside the viscous boundary layer. The major source of interaction between nanoparticles and base fluid was considered to be the concentration diffusion due to Brownian and Thermophoretic forces. Mahdavi et al. (2018) conducted a comprehensive study on the impact of nanoparticles by considering a variety of interaction forces. They also stated that it is essential to take into account the possibility of cluster formation inside boundary layer, all well as the presence of attractive/repulsive forces on the surface of the nanoparticles due to arrangement of fluid electrons. They used the Eulerian-Lagrangian approach with Discrete Phase Modeling (DPM) to be able to track parcels of particles from injection surface through the entire computational domain. Their results showed that there will always be nanoparticles concentration distribution inside the boundary layer, induced by interaction forces and particularly Brownian force due to its random nature. In another viewpoint of nanoparticles movement in a fluid flow, Michaelides (Citation2015) attempted to correlate the major forces of Brownian and Thermophoresis to drag force by introducing the equivalent slip velocity. They claimed that the proposed method can be applicable to a variety of nanofluid medium with the high level of being heterogeneous. On the other hand, Ham and Cho (Citation2016) used only the modified thermo-physical of nanofluid as the main effect of using nanoparticles and ignored the possibility of particle-fluid interaction in the flow. However, they mentioned that the improvement of nanofluid properties may not be the only reason heat transfer enhancement. Because of particles sedimentation on the hot surface and inside small cavities, the roughness may change and contribute to the improved heat transfer. This means that nanoparticles may be able to penetrate into boundary layer in some certain circumstances and change the flow field, especially close to the wall. Ding and Wen (Citation2005) used the conception of mass diffusion to connect the link between migration of nanoparticles and other flow field parameters. They stated that particles can diffuse into flow field similar to continues phase by defining three mass flux induced by viscosity gradient, non-uniform shear rate and Brownian motion. Because of dependency of flow parameters, the concentration distribution may be inevitable, as well as nanofluid mixture viscosity with being a function of nanoparticle volume fraction. Subsequently, their results presented a noticeable variation of fluid viscosity inside the boundary layer from the pipe wall.
Literature review shows that the focus of nanofluid studies has been mainly to investigate the thermal and hydrodynamic features of nanoparticles in terms of heat transfer enhancement or pressure drop, both experimentally and numerically. There are only a few reports with the proper attention to the possibility of particles diffusion and altering the global boundary layer. In this study, the impact of nanoparticles on changing of developing boundary layer in a flow between two plates known as Poiseuille flow is numerically analyzed. The reason behind choosing such a geometry is for the purpose of easier comparison of developing section and boundary layers. Other complicated geometries lead to three-dimensional boundary layer growth which makes it difficult to compare for various volume fractions. Because of the application of nanoparticles in thermal systems, a uniform heat flux boundary condition is applied on the plates. Nanoparticles are injected at the inlet and tracked in the Lagrangian frame by using the discrete model.
2. Physical model and mathematical formulation
2.1. Geometry description and working fluid
For the purpose of this research, a 2D model of flow between two parallel plates is numerically studied with constant uniform heat flux on the wall. The schematic of the computational domain is shown in Figure . Nanoparticles are injected through inlet boundary and travel the entire domain and leave from the outlet. Uniform constant heat flux is applied on both plates at the top and bottom. The space between two plates is 4 mm, with a total length of 6 m to ensure fully developed flow is achieved far before reaching the outlet. Reynolds number is kept below 1800 to maintain laminar forced convection all over the domain.
Figure 1. Schematic of the flow domain between two flat parallel plates exposed to uniform heat flux t the top and bottom.
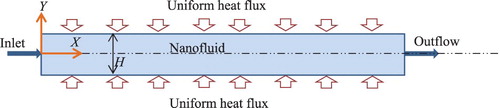
Since the main purpose of this study is to investigate the impact of nanoparticles on boundary layers, up to 6% vol. alumina nanofluid is studied here. Alumina nanofluid is one of the most common types of nanofluids being used by researchers, and appropriate empirical properties are available in the literature. Heat transfer fluid is entered into the domain at temperature 22°C. Mass flow rate is kept between 0.2 and 0.9 kg/s, and the comparison among different volume fractions of nanofluid is made through similar Reynolds number. The properties of working base fluid and alumina nanoparticles are presented in Table .
Table 1. Thermo-physical properties of water and nanoparticles at 22°C (M. Mahdavi et al., Citation2015).
During the process of nanofluid preparation, thermo-physical properties of the mixture are changed, and therefore empirical correlations are required to be implemented in the simulations. Nanofluid density is calculated by the correlation proposed by Sharifpur et al. (Citation2016) to consider the impact of thin nanolayer formed around nanoparticles, and thermal conductivity and viscosity by Corcione (Citation2011) and Khanafer and Vafai (Citation2011), as follows:
(1)
(1)
(2)
(2)
(3)
(3)
(4)
(4)
(5)
(5)
2.2. Mathematical formulation and discrete phase modeling
Laminar forced convective flow is numerically solved in this research by using ANSYS-Fluent 19.3. Fluid governing equations consist of continuity, momentum and energy (M. Mahdavi, Sharifpur, and Meyer 2018; Michaelides & Feng, Citation1994):
(6)
(6)
(7)
(7)
(8)
(8) where i and j are index notation representing both X and Y directions. Sm and Se are the momentum and energy source terms due to interaction between fluid and particles. To obtain these source terms, Discrete Phase Modelling (DPM) is used to track the nanoparticles inside computational domain in the Lagrangian frame. The other terms are only concerned with fluid variables and properties in these equations. Both momentum and energy source terms in these equations are simply based on the force and energy transported by the mass flow rate of the nanoparticles, as further details explained by Mahdavi et al. (2018). The general force balance form of accelerated particles with various interactions is given as (Michaelides & Feng, Citation1994):
(9)
(9)
The term on the left-hand side is the acceleration of particles in Lagrangian time. The terms on the right-hand side are drag, gravitational force, lift, magnus, thermophoresis and Brownian random motion, respectively. They are defined as follows:
Due to nano-size of the particles, drag force will be as follows to be properly combined with Brownian motion (Li & Ahmadi, Citation1992):
(10)
(10)
(11)
(11)
Lift Force (Zheng and Silber-li Citation2009):
(12)
(12) where
is the shear rate.
Magnus force induced by rotation of the particle around its axis (Oesterle & Bui Dinh, Citation1998; Turkyilmazoglu & Altundag, Citation2020) can change the trajectory and final destination of the particles in the fluid, as mentioned by Mahdavi et al. (2018):
(13)
(13)
(14)
(14)
(15)
(15)
(16)
(16)
Particle angular velocity is obtained by solving the angular momentum equation:
(17)
(17)
(18)
(18)
(19)
(19)
Thermophoretic force is caused by the higher kinetic energy of the fluid particles compared to heaver nanoparticles. This force appears in opposite direction of the temperature gradient of the particles (McNab & Meisen, Citation1973);
(20)
(20)
(21)
(21)
Brownian random force due to random motion of particles suspended in a fluid (Li & Ahmadi, Citation1992):
(22)
(22)
The randomness of Brownian induced by fluid temperature can be reflected in white Gaussian random function of , which basically can change between −2–2.
Heat transfer equation of a particle will be simply the energy balance between fluid due to convection and moving particles from one computational cell to other, as here:
(23)
(23) where h and Ap are heat transfer coefficient and heat transfer area on the particles. Mass flow rate is set up as an inlet boundary condition and constant heat flux on the plates. Particles are injected at the inlet with similar conditions of the flow field.
Two types of boundary conditions are applied here regarding the Eulerian for fluid flow and Lagrangian for nanoparticles. Mass flow rate is adjusted for the fluid flow at the inlet with the assumption of fully developed at the outlet, known as outflow. The inlet temperature is kept constant for both flow and nanoparticles, with uniform constant heat flux on the top and bottom plates. For DPM particles, the temperature and velocity are adjusted according to inlet flow. Various nanofluid volume fractions can be obtained by changing the nanoparticles mass flow rate.
The simulation is done in laminar regime by using the Coupled scheme for pressure-velocity coupling. PRESTO! is used to discretize the pressure gradient terms and second-order upwind for momentum and energy terms. Then the modified properties of nanofluid are added to the equations, and the simulation is performed until the solution is converged. The flow is solved in laminar steady regime for incompressible fluid flow. To ensure the convergence is reached, temperature on the walls, pressure drop along the channel, heat and mass balanced are monitored to reach their asymptotic or minimum values. Afterwards, nanoparticles are injected from inlet with considering all the essential interaction terms and are tracked until they leave the domain.
3. Results and discussion
3.2. Model validation
A structured mesh is generated in the computational domain with fine cells at the entrance region. Since the flow is laminar, even a coarse mesh can provide the correct results for velocity and temperature. However, to ensure the accuracy of the discrete model is also preserved with appropriate small Lagrangian time step, a finer mesh of 40 × 1200 cells was generated to produce average time step of 0.001 s. It is noted that particles time step is generated according to the sizes of the cell.
To validate the numerical calculations, the analytical velocity profile in X direction for the flow between two parallel plates available from many fluid and heat transfer textbooks (Bejan, Citation2013; Cengel, Citation2014) is used. The x velocity profile correlation with considering the variation of y coordinate between will be as follows:
(24)
(24)
The results of numerical calculations and analytical solution are compared in Figure . To confirm the accuracy of the results for the case of nanofluid, simulation was also conducted for alumina nanofluid 1% vol. as shown in Figure . There is a perfect agreement between correlation and simulation, in both water and nanofluid flows. It means that the generated mesh and solution method are appropriate for further modeling.
3.2. Simulation results – hydrodynamic and thermal analysis
For the sake of comparison, it is important to use one of the non-dimensional numbers regarding hydrodynamic or heat transfer feathers. In this case, a fixed Reynolds number can be employed to find the impacts of nanoparticles compared to pure water. It means that with modified properties, the velocity has to be adjusted to obtain the same Reynolds number. Otherwise, the comparison will be wrong. In other words, the advantages of using nanofluid can be revealed when the same flow non-dimensional numbers are employed, in this case Reynolds number.
The results of velocity and temperature on the central line of the channel from inlet to outlet are presented in Figure for various nanoparticles volume fraction. Since Reynolds number represents the friction factor in internal and laminar flows as , and similar Reynolds number leads to same friction factors, volume faction indicates no negative effects on friction factor compared to pure water in Figure (a). While, the core velocity increases by higher volume fraction, that can result in a higher level of heat transfer and lowering the core temperature in Figure (b). It is also observed that the rate of the temperature dropping in connection with nanoparticles concentration changes in downstream. This can be proved by checking the gradient of velocity and temperature in the flow direction, as shown in Figure . Although velocity gradient is not largely affected by the presence of nanoparticles, however, a higher rate of heat transfer is observed along the channel for higher concentration. In other words, a smaller temperature gradient can be caused by better heat diffusion (because of nanofluid high thermal conductivity) from the wall to the core of the fluid, leading to temperature drop faster.
Figure 3. Evolution of velocity and temperature on the centerline of the flow between two parallel plates from inlet to outlet for different nanofluid concentration.
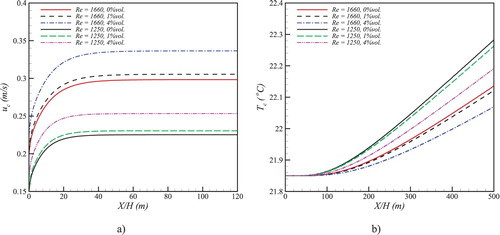
Figure 4. Evolution of velocity and temperature gradient in flow direction on the centerline of the flow between two parallel plates from inlet to outlet for different nanofluid concentration.
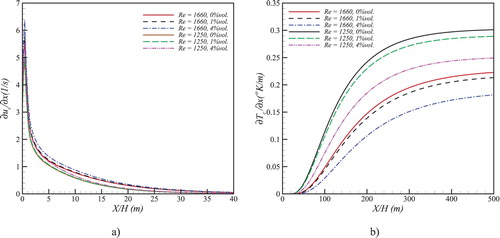
One of the important impacts of shear flow between two parallel plates is concerned about the rotational impact of the fluid element on nanoparticles. Vorticity is the representative of rotational flow, as presented in Figure for three different volume fractions, and defined:
(25)
(25)
Figure 5. Evolution of vorticity magnitude from inlet in flow direction close to the centerline between two parallel plates with different nanofluid concentration.
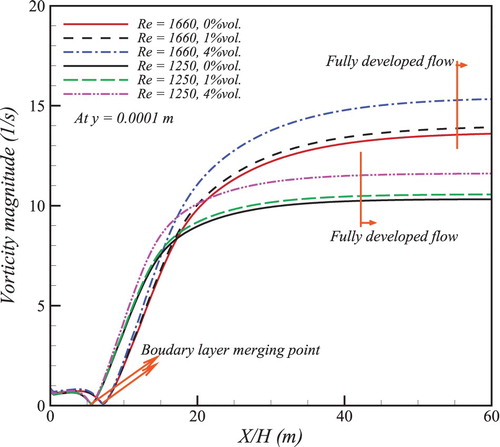
This definition of vorticity can imply that the fluid element can start rotating around its axis. This can happen when a fluid element enters the viscous boundary layer. This conception can be used to interpret the trend of vorticity magnitude in Figure . The first part of the graph is flat, meaning the fluid elements are inside the inviscid region with the parallel flow. There is a sharp drop to zero when the element enters the boundary layer, followed by a quick rise. It is observed that the presence of nanoparticles has a negligible impact of fluid vorticity up to a point where the rate of vorticity magnitude growth starts decreasing. It can also be said that the merging point of boundary layers from top and bottom is highly dependent on Reynolds number, the lower Reynolds number the merging point closer to the inlet. Therefore, three areas can be identified for the evolution of vorticity as the initial flat part, high and low growth rate of vorticity. There is no doubt that the mixing of the boundary layer occurs in the second area.
The significant factor influencing the velocity gradient and vorticity is shear stress produced by the contact between fluid and wall, shown in Figure . There is a consistent trend for the wall shear stress, and as the nanoparticles concentration increases, shear stress is shifted by a factor. Although the jump between 1% to 4%vol. is noticeable. This is caused by the prominent adverse effect of viscosity, especially for higher Reynolds number.
To understand the behavior of heat transfer from the wall to fluid, the gradient of temperature in the y-direction is provided in Figure . Two aspects of this graph are of importance. Firstly, the gradient of temperature is adversely affected by nanoparticles, and higher volume fraction can lower the temperature gradient by increasing the flow resistance and delaying the flow transfer. Secondly, it was shown that higher nanoparticles concentration could improve heat transfer, but in Figure is proven that diffusion of heat in the y-direction is the main reason for higher heat transfer. In other words, from , it can be concluded that smaller temperature gradient does not necessarily lead to lower heat transfer, and in the case of nanofluid, the thermal conductivity can improve the heat transfer through diffusion.
Figure 7. Temperature gradient in y-direction for different nanoparticles concentration close to the centerline of the flow between two parallel plates.
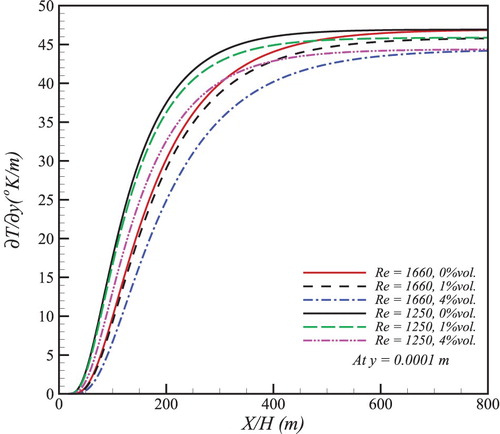
One of the main advantages of using DPM is that nanoparticles can be tracked in the flow field until final fate. Y position and velocity magnitude of both nanoparticles and fluid elements are illustrated in Figures and . Nanoparticles and fluid are released at two major locations at the inlet, close to the wall and center. Since nanoparticles are assumed as the dispersed phase, the continues phase surrounds each individual parcel, and all the important forces on small particles become noticeable. At first, it is seen that there is a clear oscillation in nanoparticles results of tracking. The only force with a random parameter in the correlation is Brownian, caused by the random vibration of particles at that temperature. In other words, the oscillation is the result of Gaussian random parameter in Brownian force. The results show that the dominant effect of Brownian force is unavoidable and particularly close to the wall with high-temperature gradient. Also, in spite of nano-size particles, it is revealed that nanoparticles are able to slightly deviate from initial fluid element and travel to other flow layers under the influence of shear forces.
Figure 8. Tracking of nanoparticle and fluid particle y position from inlet to outlet according to Lagrangian time.
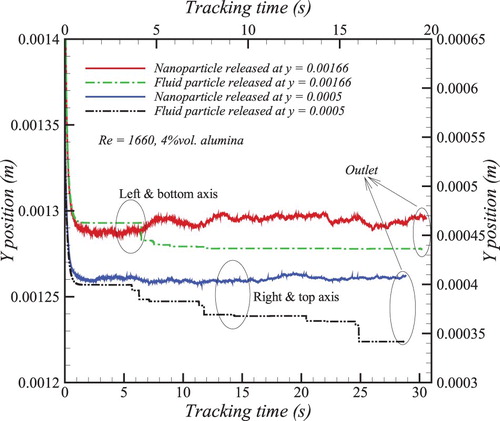
Figure 9. Tracking velocity magnitude of nanoparticles and fluid particles from inlet to outlet according to Lagrangian time.
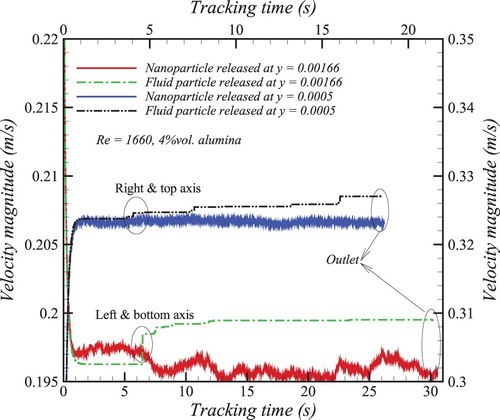
Nanoparticles angular velocity is mainly induced by the shear stress of viscous boundary layer and gradient of temperature a thermophoretic force. Angular velocity can also have a similar trend to vorticity, shown in Figure . Both conceptions represent rotation around particles or element axis. It is observed that the location of the initial rotation of nanoparticles can slightly differ according to the volume fraction of injection. It is noted that higher viscosity of larger volume fraction can result in shorter merging boundary layer, and closer location to inlet for initial particles rotation. Stronger angular velocity is expected for higher volume fraction due to stronger interaction forces.
4. Conclusion
Heat and fluid flow in the entrance region between two parallel plates with the presence of nanoparticles was numerically investigated. The flow was assumed forced laminar convection, and the plates were exposed to constant uniform heat flux. Continuity, momentum and energy equations were applied to continues phase in Eulerian and discrete phase modeling for nanoparticles injection in the Lagrangian frame. The findings can be listed as follows:
The initial results were validated against analytical solutions and found in excellent agreement. Velocity and temperature profiles were drastically affected by nanoparticles concentration, as well as temperature gradient.
Velocity gradient in flow direction showed only slight deviation for various nanoparticles volume fraction. Evaluation of vorticity magnitude revealed three stages for flow rotational development. A similar trend was also found with nanoparticles angular velocity.
It was proved that adding to the volume fraction can in fact expedite merging hydrodynamic boundary layer, caused by improved fluid viscosity.
The DPM results on Lagrangian tracking time showed that Brownian motion had a dominant effect impact on particle migration, and mainly close to the wall due to higher interference of thermophoresis at the vicinity of the plates.
Nomenclature
= | particle projected area [m2] | |
= | Cunningham correction factor | |
= | drag coefficient | |
= | rotational coefficient | |
= | specific heat [J/kg.K] | |
D | = | disk diameter [m] |
DT | = | Thermophoresis coefficient |
= | particle diameter [m] | |
dc | = | water molecule diameter [m] |
= | thermophoresis diffusion coefficient [m2/s] | |
Fdrag | = | drag force [N] |
Flift | = | lift force [N] |
FMagnus | = | Magnus force [N] |
Fthermo | = | thermophoresis force [N] |
FB | = | Brownian force [N] |
h | = | heat transfer coefficient [W/m2.K] |
H | = | plate distance (m) |
Ip | = | moment of inertia [kg.m2] |
= | Thermal conductivity [W/m.K] | |
= | Boltzmann constant [m2.kg/oK.s2] | |
= | particle mass [kg] | |
P | = | pressure [Pa] |
q | = | laminar and turbulent heat flux [W/m2] |
Re | = | Reynolds number |
Rep | = | particle Reynolds number |
= | particle angular Reynolds number | |
Sm | = | momentum source term [Pa/m] |
Se | = | energy source term for nanoparticles [kg/s.oK] |
tv | = | nano-layer thickness [nm] |
= | particle time step [s] | |
Tfr | = | reference temperature [ok] |
V, u | = | velocity [m/s] |
Greek letters
= | particle volume fraction | |
= | shear rate [1/s] | |
= | viscosity [Pa.s] | |
= | particle angular velocity [1/s] | |
= | relative particle-liquid angular velocity [1/s] | |
= | density [kg/m3] | |
= | Particle relaxation time [s] | |
= | Gaussian white noise random number |
Subscript
W, l, c | = | Water, continues phase |
m | = | Nanofluid, mixture |
p | = | particle |
Disclosure statement
No potential conflict of interest was reported by the author(s).
References
- Abadi, E., Mohammad, A., et al., Sadi, M., Farzaneh-Gord, M., Ahmadi, M.H., Kumar, R., Chau, K. (2020). A numerical and experimental study on the energy efficiency of a regenerative heat and mass exchanger utilizing the counter-flow Maisotsenko cycle. Engineering Applications of Computational Fluid Mechanics, 14(1), 1–12. https://doi.org/10.1080/19942060.2019.1617193
- Aghayari, R., et al. (2020). Theoretical and experimental studies of heat transfer in a double-pipe heat exchanger equipped with twisted tape and nanofluid. The European Physical Journal Plus, 135(2), 1–26. https://doi.org/10.1140/epjp/s13360-020-00252-8
- Ahmadi, M. H., et al. (2020). Evolving connectionist approaches to compute thermal conductivity of TiO2/water nanofluid. Physica A: Statistical Mechanics and its Applications, 540, 122489. https://doi.org/10.1016/j.physa.2019.122489
- Ahmadi, M. H., Ghahremannezhad, A., et al. (2019). Development of simple-to-use predictive models to determine thermal properties of Fe2O3/water-Ethylene Glycol nanofluid. Computation, 7(1), 18. https://doi.org/10.3390/computation7010018
- Ahmadi, M. H., Mohseni-Gharyehsafa, B., et al. (2019). Applicability of connectionist methods to predict dynamic viscosity of silver/water nanofluid by using ANN-MLP, MARS and MPR Algorithms. Engineering Applications of Computational Fluid Mechanics, 13(1), 220–228. https://doi.org/10.1080/19942060.2019.1571442
- Ahmadi, M. H., Sadeghzadeh, M., et al. (2019). Precise smart model for estimating dynamic viscosity of SiO2/ethylene Glycol–water nanofluid. Engineering Applications of Computational Fluid Mechanics, 13(1), 1095–1105. https://doi.org/10.1080/19942060.2019.1668303
- Alotaibi, S., et al. (2020). Modeling thermal conductivity of Ethylene Glycol-based nanofluids using multivariate adaptive regression splines and group method of data handling artificial neural network. Engineering Applications of Computational Fluid Mechanics, 14(1), 379–390. https://doi.org/10.1080/19942060.2020.1715843
- Aramesh, M., et al. (2020). Investigating the effect of using nanofluids on the performance of a double-effect absorption refrigeration cycle combined with a solar collector. Proceedings of the Institution of Mechanical Engineers, Part A: Journal of Power and Energy, 234(7), 981–993. https://doi.org/10.1177/0957650919889811
- Avramenko, A. A., Blinov, D. G., & Shevchuk, I. V. (2011). Self-Similar analysis of fluid flow and heat-mass transfer of nanofluids in boundary layer. Physics of Fluids, 23(8), 082002. https://doi.org/10.1063/1.3623432
- Avramenko, A. A., Blinov, D. G., Shevchuk, I. V., & Kuznetsov, A. V. (2012). Symmetry analysis and Self-similar forms of fluid flow and heat-mass transfer in turbulent boundary layer flow of a nanofluid. Physics of Fluids, 24(9), 092003. https://doi.org/10.1063/1.4753945
- Aybar, H. Ş., et al. (2015). A review of thermal conductivity models for nanofluids. Heat Transfer Engineering, 36(13), 1085–1110. https://doi.org/10.1080/01457632.2015.987586
- Baghban, A., Jalali, A., et al. (2019). Developing an ANFIS-based swarm concept model for estimating the relative viscosity of nanofluids. Engineering Applications of Computational Fluid Mechanics, 13(1), 26–39. https://doi.org/10.1080/19942060.2018.1542345
- Baghban, A., Sasanipour, J., et al. (2019). Towards experimental and modeling study of heat transfer performance of water-SiO2 nanofluid in quadrangular cross-section channels. Engineering Applications of Computational Fluid Mechanics, 13(1), 453–469. https://doi.org/10.1080/19942060.2019.1599428
- Bejan, A. (2013). Convection heat transfer. John wiley & sons.
- Bhushan, S., & Muthu, S. (2019). Parallel performance assessment of a pseudo-spectral solver for transition and turbulent boundary layer flows. Engineering Applications of Computational Fluid Mechanics, 13(1), 763–781. https://doi.org/10.1080/19942060.2019.1645736
- Bhushan, S., & Walters, D. K. (2014). Development of parallel pseudo-spectral solver using influence matrix method and application to boundary layer transition. Engineering Applications of Computational Fluid Mechanics, 8(1), 158–177. https://doi.org/10.1080/19942060.2014.11015505
- Cengel, Y. (2014). Heat and mass transfer: Fundamentals and applications. McGraw-Hill Higher Education.
- Corcione, M. (2011). Empirical correlating equations for predicting the effective thermal conductivity and dynamic viscosity of nanofluids. Energy Conversion and Management, 52(1), 789–793. https://doi.org/10.1016/j.enconman.2010.06.072 doi:10.1016/j.enconman.2010.06.072
- Ding, Y., & Wen, D. (2005). Particle migration in a flow of nanoparticle suspensions. Powder Technology, 149(2-3), 84–92. https://doi.org/10.1016/j.powtec.2004.11.012
- Ghalandari, M., et al. (2019). Numerical simulation of nanofluid flow inside a root canal. Engineering Applications of Computational Fluid Mechanics, 13(1), 254–264. https://doi.org/10.1080/19942060.2019.1578696
- Ghodsinezhad, H., Sharifpur, M., & Josua Petrus Meyer, J. P. (2016). Experimental investigation on cavity flow natural convection of Al2O3–water nanofluids. International Communications in Heat and Mass Transfer, 76, 316–324. http://linkinghub.elsevier.com/retrieve/pii/S0735193316301865. https://doi.org/10.1016/j.icheatmasstransfer.2016.06.005
- Ham, J., & Cho, H. (2016). Theoretical analysis of pool boiling characteristics of Al2O3 nanofluid according to volume concentration and nanoparticle size. Applied Thermal Engineering, 108, 158–171. https://doi.org/10.1016/j.applthermaleng.2016.07.058
- Khan, W. A., & Pop, I. (2010). Boundary-Layer flow of a nanofluid past a stretching sheet. International Journal of Heat and Mass Transfer, 53(11–12), 2477–2483. https://doi.org/10.1016/j.ijheatmasstransfer.2010.01.032
- Khanafer, K., & Vafai, K. (2011). A critical synthesis of thermophysical characteristics of nanofluids. International Journal of Heat and Mass Transfer, 54(19), 4410–4428. https://doi.org/10.1016/j.ijheatmasstransfer.2011.04.048
- Li, A., & Ahmadi, G. (1992). Dispersion and deposition of spherical particles from point sources in a turbulent channel flow. Aerosol Science and Technology, 16(4), 209–226. https://doi.org/10.1080/02786829208959550
- Loni, R., et al. (2018). GMDH modeling and experimental investigation of thermal performance enhancement of hemispherical cavity receiver using MWCNT/Oil nanofluid. Solar Energy, 171, 790–803. https://doi.org/10.1016/j.solener.2018.07.003
- Maddah, H., et al. (2018). Factorial experimental design for the thermal performance of a double pipe heat exchanger using Al2O3-TiO2 hybrid nanofluid. International Communications in Heat and Mass Transfer, 97, 92–102. https://doi.org/10.1016/j.icheatmasstransfer.2018.07.002
- Mahdavi, M., Sharifpur, M., Ahmadi, M. H., & Meyer, J. P. (2018). Aggregation study of Brownian nanoparticles in convective phenomena. Journal of Thermal Analysis and Calorimetry Online, https://doi.org/10.1007/s10973-018-7283-y
- Mahdavi, M., Sharifpur, M., Ghodsinezhad, H., & Meyer, J. P. (2017). A new combination of nanoparticles mass diffusion flux and slip mechanism approaches with electrostatic forces in a natural convective cavity flow. International Journal of Heat and Mass Transfer, 106, 980–988. https://doi.org/10.1016/j.ijheatmasstransfer.2016.10.065
- Mahdavi, M., Sharifpur, M., & Meyer, J. P. (2015). CFD modelling of heat transfer and pressure drops for nanofluids through vertical tubes in laminar flow by Lagrangian and Eulerian approaches. International Journal of Heat and Mass Transfer, 88, 803–813. https://doi.org/10.1016/j.ijheatmasstransfer.2015.04.112 doi:10.1016/j.ijheatmasstransfer.2015.04.112
- Mahdavi, M., Sharifpur, M., & Meyer, J. P. (2016). Natural convection study of brownian nano-size particles inside a water-filled cavity by Lagrangian-Eulerian tracking approach. In Heat Transfer, International Conference on Heat Transfer, Fluid Mechanics and Thermodynamics (HEFAT2016), Costa del Sol, Malaga, Spain, 17.
- McNab, G. S., & Meisen, A. (1973). Thermophoresis in liquids. Journal of Colloid And Interface Science, 44(2), 339–346. https://doi.org/10.1016/0021-9797(73)90225-7
- Michaelides, E. E. (2015). Brownian movement and thermophoresis of nanoparticles in liquids. International Journal of Heat and Mass Transfer, 81, 179–187. https://doi.org/10.1016/j.ijheatmasstransfer.2014.10.019
- Michaelides, E. E., & Feng, Z. (1994). Heat transfer from a rigid sphere in a nonuniform flow and temperature field. International Journal of Heat and Mass Transfer, 37(14), 2069–2076. https://doi.org/10.1016/0017-9310(94)90308-5
- Oesterle, B., & Bui Dinh, T. (1998). Experiments on the lift of a spinning sphere in a range of intermediate Reynolds numbers. Experiments in Fluids, 25(1), 16–22. https://doi.org/10.1007/s003480050203
- Pettersson, K., & Rizzi, A. (2009). Comparing different CFD Methods accuracy in computing local boundary layer properties. Engineering Applications of Computational Fluid Mechanics, 3(1), 98–108. https://doi.org/10.1080/19942060.2009.11015257
- Ramezanizadeh, M., Nazari, M. A., Ahmadi, M. H., & Chau, K.-w. (2019). Experimental and numerical analysis of a nanofluidic thermosyphon heat exchanger. Engineering Applications of Computational Fluid Mechanics, 13(1), 40–47. https://doi.org/10.1080/19942060.2018.1518272
- Ramezanizadeh, M., & Nazari, M. A. (2019). Modeling thermal conductivity of Ag/water nanofluid by applying a mathematical correlation and artificial neural network. International Journal of Low-Carbon Technologies, 14(4), 468–474. https://doi.org/10.1093/ijlct/ctz030
- Sadeghzadeh, M., et al. (2020). Prediction of thermo-physical properties of TiO2-Al2O3/water nanoparticles by using artificial neural network. Nanomaterials, 10(4), 697. https://doi.org/10.3390/nano10040697
- Sharifpur, M., Yousefi, S., & Meyer, J. P. (2016). A new model for density of nanofluids Including nanolayer. International Communications in Heat and Mass Transfer, 78, 168–174. https://doi.org/10.1016/j.icheatmasstransfer.2016.09.010
- Sharma, J. P., et al. (2020). A study on thermohydraulic characteristics of fluid flow through microchannels. Journal of Thermal Analysis and Calorimetry, 140(1), 1–32. https://doi.org/10.1007/s10973-019-08741-4
- Shoghl, S. N., Bahrami, M., & Moraveji, M. K. (2014). Experimental investigation and CFD modeling of the dynamics of bubbles in nanofluid pool boiling. International Communications in Heat and Mass Transfer, 58, 12–24. https://doi.org/10.1016/j.icheatmasstransfer.2014.07.027
- Singh, P. K., P. V. Harikrishna, T. Sundararajan, & S. K. Das. 2011. Experimental and numerical Investigation into the heat transfer study of nanofluids in Microchannel. J. Heat Transfer. 133(12): 121701, https://doi.org/10.1115/1.4004430.
- Tayebi, T., Chamkha, A. J., & Djezzar, M. (2019). Natural convection of CNT-water nanofluid in an Annular space between confocal elliptic cylinders with constant heat flux on Inner wall. Scientia Iranica. Transaction B, Mechanical Engineering, 26(5), 2770–2783.
- Tshimanga, N., Sharifpur, M., & Meyer, J. P. (2016). Experimental Investigation and model development for thermal conductivity of Glycerol–MgO nanofluids. Heat Transfer Engineering, 37(18), 1538–1553. https://doi.org/10.1080/01457632.2016.1151297
- Turkyilmazoglu, M., & Altundag, T. (2020). Exact and approximate solutions to projectile motion in air incorporating magnus effect. The European Physical Journal Plus, 135(7), 1–12. https://doi.org/10.1140/epjp/s13360-020-00593-4
- Williams, W., Buongiorno, J., & Hu, L.-W. (2008). Experimental investigation of turbulent convective heat transfer and pressure loss of alumina/water and Zirconia/water nanoparticle colloids (nanofluids) in horizontal tubes. Journal of Heat Transfer, 130(4), 42412. https://doi.org/10.1115/1.2818775
- Zheng, X., & Silber-li, Z. (2009). The influence of Saffman lift force on nanoparticle concentration distribution near a wall. Applied Physics Letters, 95(12), 124105. https://doi.org/10.1063/1.3237159