ABSTRACT
Conservation of Resources (COR) theory defines psychological stress as the result of a threat or actual loss of resources, or lack of resource gain. Given that disasters present a significant risk for resource loss, the aim of this study was to examine the dynamic relationship between the change in different levels of resources and the change in psychosocial functioning. A random sample of N= 224 community members from a municipality affected by the 2014 Southeast Europe floods were interviewed one and a half and two and a half years post-disaster, using the Connor–Davidson Resilience Scale 10-item version, the Multidimensional Scale of Perceived Social Support, the Community Resources Scale – the Social Capital and Community Engagement subscale, the PTSD Checklist for DSM-5, the Center for Epidemiological Studies Depression Scale-Revised and the Satisfaction with Life Scale. The results of the Latent Difference Scores modelling indicate that the increase in resources was related to a decline in post-traumatic stress (PTS) and depression symptoms and increase in life satisfaction, and vice versa. Interpersonal resources were significantly related to all measured psychosocial outcomes, individual resources to PTS and life satisfaction and community resources to life satisfaction only. The mean level of resources remained the same, but a significant inter-individual variability in resource change was found: for some, they have increased, and for some decreased over time. Furthermore, resources changed independently: an increase in one was not related to an increase in another. These findings highlight the importance of resource gain and loss for psychosocial outcomes and call for targeted post-disaster interventions that can, by increasing the levels of resources in affected communities, decrease the levels of symptoms and increase well-being.
HIGHLIGHTS
• The increase in individual, interpersonal and community resources was related to better psychosocial functioning post-disaster.• On average, there was no change in mean level of resources over a one year period.• Stepwise model of post-disaster psychosocial support focusing on family and community level interventions could be beneficial for long-term psychosocial recovery.
La Teoría de Conservación de Recursos (COR) define el estrés psicosocial como el resultado de una amenaza o efectiva pérdida de recursos, o falta de ganancia de éstos. Dado que los desastres presentan un riesgo significativo de pérdida de recursos, el objetivo de este estudio fue examinar la relación dinámica entre el cambio en diferentes niveles de recursos y el cambio en el funcionamiento psicosocial. Una muestra aleatoria de N=224 miembros de la comunidad de un municipio afectado por las inundaciones de 2014 en el Sudeste de Europa fueron entrevistados uno y medio y dos y medio años post-desastre, usando la versión de 10 ítems de la Escala de Resiliencia de Connor-Davidson, La Escala Multidimensional de Apoyo Social Percibido, la Escala de Recursos Comunitarios – la subescala de Capital Social y Compromiso Social, la Lista de Chequeo para TEPT del DSM-5, la Escala Revisada de Depresión del Centro de Estudios Epidemiológicos y la Escala de Satisfacción con la Vida. Los resultados del modelo de Puntajes de Diferencia Latente indican que el incremento en recursos se relacionó a una disminución de síntomas de estrés postraumático (EPT) y depresión y a un incremento en la satisfacción vital, y viceversa. Los recursos interpersonales se relacionaron significativamente a todos los resultados psicosociales medidos, los recursos individuales a EPT y satisfacción vital, y los recursos comunitarios sólo a satisfacción vital. El nivel promedio de recursos se mantuvo igual, pero se encontró una significativa variabilidad inter-individual en el cambio de recursos: para algunos aumentó y para otros disminuyó en el tiempo. Más aún, los recursos cambiaron independientemente: un incremento en uno no se relacionó a un incremento en otro. Estos hallazgos destacan la importancia de la ganancia y pérdida de recursos para los resultados psicosociales y llaman a realizar intervenciones post-desastre dirigidas que puedan, a través del incremento de los niveles de recursos en las comunidades afectadas, disminuir el nivel de síntomas e incrementar el bienestar.
随机样本由来自一个东南欧城市的 N = 224名社区成员构成,他们受 2014 年洪水影响,在灾后一年半和两年半的时间参加了访谈。使用 Connor-Davidson Resilience Scale 10 题目版本,多维度感知社会支持量表,社区资源量表中的社会资本和社区参与度量表,DSM-5 PCL,流调中心抑郁量表修订版和生活满意度量表。潜在差异分数模型的结果表明,资源的增加与创伤后应激(PTS)症状和抑郁症状的下降以及生活满意度的增加有关,反之亦然。人际关系资源与所有测量的心理社会结果显著相关,个人资源和PTS以及生活满意度相关,社区资源仅与生活满意度显著相关。资源的平均水平保持不变,但发现资源变化的个体间差异显著:对于一些人来说增加,而对有些人来说随着时间的推移而减少。此外,资源呈现独立变化:一类资源的增加与另一类的增加无关。这些研究结果突出了资源获得和丧失对心理社会结果的重要性,并呼吁采取有针对性的灾后干预措施,通过提高受影响社区的资源水平,降低症状水平和增加福祉。
1. Introduction
Unlike cognition-based theories of stress, where stress is considered to be rooted in an individual’s appraisal of an event, a more environmental, resource-based approach has spurred interest in disaster research. This is related to the very nature of the event: surviving a disaster includes a myriad of losses, some tangible, such as the loss of loved ones, physical health and widespread material losses, and many impalpable ones, such as loss of social networks due to relocation, along with loss of optimism, belief in the just world, and hope. These attributes are usually embedded in the definition of the event itself, for example, the United Nations Office for Disaster Risk Reduction (Citation2009) states that a disaster is a ‘serious disruption of the functioning of a community or a society involving widespread human, material, economic or environmental losses and impacts, which exceeds the ability of the affected community or society to cope using its own resources’.
One of the most commonly applied perspectives on stress in studies of disasters stems from the Conservation of Resources (COR) theory (Hobfoll, Citation1989). COR theory claims that people strive to obtain, retain and protect their resources because of their value in itself or their potential in obtaining other valuable resources. Therefore, psychological stress is experienced when there is a threat of loss of resources, actual loss of resources, or lack of resource gain following the investment of resources. Resiliency to stress, or fast recovery after stressful situations, is the result of preventing resource loss or recovering resources, which is related to the previous amount, strength and diversity of one’s resources (Hobfoll, Citation1989, Citation2001). The main resource categories proposed by Hobfoll (Citation1989) are presented in .
Table 1: Types of resources.
There is ample evidence supporting the role of resource loss in post-disaster functioning. Resource loss predicted distress after hurricane, both concurrently (Freedy, Shaw, Jarrell, & Masters, Citation1992) and longitudinally (Benight et al., Citation1999), mediated the relationship between the severity of flood exposure and psychological distress and physical symptoms (Smith & Freedy, Citation2000), and had effects over and beyond traumatic exposure and pre-disaster psychological functioning (Sattler et al., Citation2006; Zwiebach, Rhodes, & Roemer, Citation2010). On the other hand, empirical support for the role of resource gain seems to be inconsistent: some studies indicate that it increases psychological functioning (e.g. Hobfoll, Johnson, Ennis, & Jackson, Citation2003), others found no relationship (e.g. Zwiebach et al., Citation2010), while some even found it related to a decrease in functioning (e.g. Hobfoll, Canetti-Nisim, & Johnson, Citation2006). However, there are several limitations in the approaches used up to date. These include the definition of resources, as well as methodological approaches used to measure them.
The most common criticism of the COR theory is the definition and scope of the key resources that play a role in experiencing stress (Halbesleben, Neveu, Paustian-Underdahl, & Westman, Citation2014; Thompson & Cooper, Citation2001). Acknowledging that almost anything of value may be considered a resource, Hobfoll (Citation2001) proposed 74 key resources broadly representing four resource categories. However, previous studies have been inconsistent in the selection of key resources, both in the number of resources assessed and their focus. For example, the number of resources under study can be found to vary from 14 to 52, focusing on all the four resources categories, material resources or personal and social resources specifically (Benight et al., Citation1999; Freedy et al., Citation1992; Sattler et al., Citation2006; Smith & Freedy, Citation2000). Furthermore, resource loss score is commonly aggregated across different categories of resources, assuming equal impact on post-stressor functioning. For example, Hobfoll, Tracy, and Galea (Citation2006) examined the psychological impact of terrorist attacks using an 11-item scale to assess a mixture of individual and social resources, such as time for sleep, optimism, feeling valuable to others and time with loved ones.
Other studies have examined the role of resources at different levels of ecological systems (e.g. Kimhi, Citation2016; Norris, Stevens, Pfefferbaum, Wyche, & Pfefferbaum, Citation2008). Individual resources can be seen as personal characteristics that affect the ability to cope with threatening events and promote the rate of recovery, such as socio-demographic characteristics, personality traits, sense of control and self-efficacy. Similarly, community and societal resources also contribute to post-disaster functioning but influence a larger number of individuals at the same time. These resources include, among others, community economic resources, social capital, community efficacy or trust in government and public institutions. This view is linked closely to the widely acknowledged approaches that emphasise the ecological perspective of multiple, dynamic and interrelated systems that contribute to development, psychopathology and well-being in general (e.g. Bronfenbrenner’s ecological model, Bronfenbrenner, Citation1977). It may be especially useful in disaster research as it has the potential to guide the pre- and post-event interventions given that disasters affect a multitude of systems at once.
The evidence suggests that resources at differing levels of systems contribute to psychological outcomes post-disaster (for a comprehensive review see, for example, Bonanno, Brewin, Kaniasty, & La Greca, Citation2010; Masten & Narayan, Citation2012). For example, individual characteristics, such as hardiness and persistence were found to be related to lower levels of PTSD, depression and anxiety after natural and human-made disasters (Ahmad et al., Citation2010; Irmansyah, Dharmono, Maramis, & Minas, Citation2010; Ying, Wu, Lin, & Jiang, Citation2014). An important interpersonal resource, social support, has repeatedly been found to contribute to better mental health outcomes after disasters (e.g. Bonanno, Galea, Bucciarelli, & Vlahov, Citation2007; Kaniasty & Norris, Citation2008). Some studies indicate that community resilience, defined as community leadership, collective efficacy, preparedness, place attachment and social trust contribute to mental health and well-being in the context of armed conflict (Braun-Lewensohn & Sagy, Citation2014; Kimhi & Eshel, Citation2009), but more evidence, including in different contexts, is needed. Even though these studies demonstrate the importance of individual and social resources, they do not directly test the role of resource change. Studies following this line of research, despite the advancement in the understanding of the ecology of resources, have yet to contribute to the understanding of the process of resource loss or gain across time (Benight, Cieslak, & Waldrep, Citation2009).
Finally, resource change is usually assessed retrospectively by asking the participants to rate the amount of loss or gain they experienced since the disaster up to a given time point (e.g. Benight et al., Citation1999; Freedy et al., Citation1992; Sattler et al., Citation2006; Smith & Freedy, Citation2000). Yet, studies show that retrospective measurements are burdened with recall bias (Moffitt et al., Citation2010), especially when it comes to psychosocial variables (Henry, Moffitt, Caspi, Langley, & Silva, Citation1994). This may be especially true when measuring change, since change scores tend to be greater in retrospective measurements (Norman, Citation2003). A few authors (e.g. Zwiebach et al., Citation2010) applied a more direct approach to assess changes in resources, namely calculating a difference score by subtracting the scores from two measurement points. However, it has been shown that change scores are burdened with measurement error, impacting significance testing and standardised coefficients (Newsom, Citation2015). Structural equation modelling approaches (SEM) provide a method to analyse longitudinal change and to test change-to-change relationships among two or more processes unburdened by measurement error (Henk & Castro-Schilo, Citation2015; McArdle, Citation2009).
The current study aims to contribute to a growing body of evidence supporting the role of resource loss and gain in post-disaster psychological outcomes. At the same time, it aims to address several of the previously identified gaps. First, it utilises an ecological perspective by identifying resources at individual, interpersonal and community level. Second, resources and outcomes are measured longitudinally, thus directly testing the role of resource loss or gain in psychosocial outcomes across time. Finally, an SEM approach is used to test the dynamic relationship between the change in resources and change in several psychological outcomes, therefore accounting for measurement error associated with change scores. Specifically, it was hypothesised that an increase in hardiness and persistence (individual resources), social support (interpersonal resources) and community social capital and engagement (community resources) would be related to a decrease in the symptoms of post-traumatic stress and depression and an increase in life satisfaction in the long-term period after a natural disaster.
2. Method
2.1. Event
In May 2014 severe flooding struck south-eastern Europe. In Croatia, the event resulted in the first official declaration of a ‘state of catastrophe’ by the National Protection and Rescue Directorate. After several weeks of heavy rainfall, on 17 May around 15:00 hours the river Sava embankment breached in several locations, which led to a rapid water surge to the surrounding communities. As a result of the flooding, two people were killed and more than 13,000 people were evacuated. Due to the suddenness of the event and a number of refusals of early evacuation, a large number of people had to be rescued from their flooded homes.
The flood resulted in devastating material losses: thousands of livestock drowned and around 7,500 damaged buildings, amounting to hundreds of millions EUR worth of damage (National Protection and Rescue Directorate, Citation2015). The reconstruction of homes was an additional source of stress for the affected population. Faced with a choice of receiving monetary reimbursement (up to around 9,500 EUR) to conduct reconstruction or entrusting the reconstruction to the Government, the majority of the affected people chose to take the reimbursement (Bobovec, Mandić, & Pozojević, Citation2016). However, it later became clear that the sum awarded for the reconstruction was insufficient, leading to additional material losses and dissatisfaction and a sense of unfairness.
2.2. Participants and procedure
The sample consisted of 224 community members from the most severely struck municipality in Croatia. The sample size was large enough to ensure that parameter estimates are within the acceptable margin of error of 6%, with the confidence level of 95%. Participants were eligible for this study if they were between 25 and 65 years old, have lived in the community at least 5 years prior to the flooding and have been in the community at the day of the flood incident. They were recruited using random sampling of households based on the register provided by the State Geodetic Administration. If several adults in one household were eligible, one adult whose birthday was closest to the interview date was selected. Up to three attempts were made to conduct the interview. Interviews were conducted by trained interviewers using instruments that have been translated and back-translated by experts fluent in English and Croatian. The average time for completing a single interview was approximately 1 h. Several community leaders supported and announced the study to community members, including the school principal, religious leaders and the local radio station.
Interviews were conducted at two time points: in November 2015 (T1) and in September 2016 (T2). T1 was selected based on the almost completed rebuilding efforts in the community and the return of the majority of the community members, to ensure the feasibility of assessing community resources. The 10-month interval between the measurement points was deemed adequate in order to capture change in resources and psychosocial outcomes. Response rate at T1 was 71.3% and the most common reasons for refusal were lack of time or not wanting to be reminded of the floods. Dropout rate at T2 was 30.5% and was mostly due to moving out of the community or working seasonal jobs. Only 19 participants (8.5% of the original sample) refused to participate at T2, due to low interest or perceiving no benefits from participating. No significant differences were found in socio-demographic variables, exposure, mental health-care utilization and resource and outcome measures at T1 between the participants who dropped-out and those who remained in the study; therefore no evidence was found for systematic drop-out. Descriptive information about the sample and dropout analysis is provided in .
Table 2: Sample descriptive information and drop-out analysis.
The study was approved by the University of Zagreb Department of Psychology ethical committee. Written informed consent was obtained from all participants at T1. Data collection guaranteed confidentiality since an individual code assigned to the household was used to match questionnaires from two time points. In case a participant reported symptoms of distress, he/she was provided with information on where to seek help and a flyer with information on stress and coping.
2.3. Measures
Individual resources were assessed with Connor–Davidson Resilience Scale 10-item version (CD-RISC 10; Campbell-Sills & Stein, Citation2007). This self-report scale captures two facets of individual resources, hardiness and persistence. Participants rated their responses on a 5-point scale (0 = not true at all, 1 = rarely true, 2 = sometimes true, 3 = often true, 4 = true nearly all the time) referring to the previous month. Internal consistency of the scale was very good at both time points (Cronbach’s α(T1) = .89, Cronbach’s α(T2) = .91).
Interpersonal resources were assessed using the Multidimensional Scale of Perceived Social Support (MSPSS; Zimet, Dahlem, Zimet, & Farley, Citation1988). The 12-item scale assesses the perception of support from three different sources (family, friends and a significant other) on a 7-point rating scale (1 = strongly disagree, 2 = disagree, 3 = slightly disagree, 4 = neither agree nor disagree, 5 = slightly agree, 6 = agree, 7 = strongly agree). Internal consistency was excellent at both time points (Cronbach’s α(T1) = .91, Cronbach’s α(T2) = .93).
Community resources were assessed using a 6-item Social Capital and Community Engagement subscale of the Community Resources Scale (Bakic, Citation2017). The scale was developed for the purpose of this study and pretested in a pilot. The Social Capital and Community Engagement subscale taps into social relationships at the community level, namely connectedness, trust and mutual helping as well as collective efficacy (e.g. There is a feeling of trust between community members; Community members work together to solve problems). Participants responded on a 5-point rating scale (0 = not at all, 1 = to a small extent, 2 = somewhat, 3 = to a large extent, 4 = to a full extent). Internal consistency was good at both time points (Cronbach’s α(T1) = .81, Cronbach’s α(T2) = .79).
Post-traumatic stress (PTS) symptoms were measured using the PTSD Checklist for DSM-V (PCL-5; Weathers et al., Citation2013), a self-report measure based on the DSM-5 classification. Participants rated to what extent they have been bothered in the past month by 20 problems across 4 clusters of symptoms (re-experiencing, avoidance, negative alterations in mood or cognitions, increased arousal) on a 5-point scale (0 = not at all, 1 = a little bit, 2 = moderately, 3 = quite a bit, 4 = extremely). Internal consistency of the scale was excellent at both time points (Cronbach’s α(T1) = Cronbach’s α(T2) = .93). A cut-off score of 33 is recommended for diagnosing PTSD when the score is calculated as a sum of all responses (Bovin et al., Citation2016).
Depression symptoms were measured using The Center for Epidemiologic Studies Depression Scale Revised (CESD-R; Eaton, Smith, Ybarra, Muntaner, & Tien, Citation2004). The scale measures symptoms of depression in nine clusters as defined by DSM-5 (sadness, loss of interest, appetite, and sleep, thinking/concentration, guilt, fatigue, movement/agitation, suicidal ideation). Participants rated the number of days the problem bothered them in the past week/past two weeks on a 5-point scale (0 = not at all or less than 1 day last week, 1 = one or 2 days last week, 2 = three to 4 days last week, 3 = five to 7 days last week, 4 = nearly every day for two weeks). Internal consistency of the scale was excellent at both time points (Cronbach’s α(T1) = .94, Cronbach’s α(T2) = .93). A cut-off score indicating ‘significant’ depressive symptomatology is 16 when the score is calculated as a sum of all responses (Eaton et al., Citation2004).
Life satisfaction was measured by the Satisfaction with Life Scale (SWLS; Diener, Emmons, Larsen, & Griffin, Citation1985). The 5-item scale measures global life satisfaction. Participants rated their responses on a 7-point scale (1 = strongly disagree, 2 = disagree, 3 = slightly disagree, 4 = neither agree nor disagree, 5 = slightly agree, 6 = agree, 7 = strongly agree). Internal consistency of the scale was very good at both time points (Cronbach’s α(T1) = Cronbach’s α(T2) = .88).
2.4. Data analysis
To analyse the relationship between the change in resources and change in psychosocial outcomes, latent difference score (LDS) models were specified (McArdle, Citation2009). These models allow assessing the change directly, as a difference between the latent scores from two measurement points; the approach which has previously been described as particularly useful to examine the mean change in scores and predicting change across time (Henk & Castro-Schilo, Citation2015; McArdle, Citation2009). In addition, they enable parcelling out the part of variance pertaining to the error, resulting in perfectly reliable change variables; a property especially important when measuring inherently unreliable difference scores (McArdle, Citation2009).
Conceptually, a difference score calculated using an LDS model is analogous to an observed difference score calculated by subtracting the values of two measurements but is defined at a latent level. This is achieved by specifying a second-order latent variable with no observed indicators and forcing a decomposition of the T2 latent construct by a series of constraints. To specify a LDS model, we (1) specified a latent construct at T1 and T2; (2) regressed the T2 latent construct on both the T1 latent construct and a second-order latent construct, with the two regression weights set to 1.0; and (3) freely estimated the covariance between the T1 and the second-order latent construct. Mathematically, these constraints define the T2 latent construct as a sum of a T1 latent construct and a second-order latent construct, meaning that the latter represents the difference between a T2 and a T1 latent construct and can be interpreted as a latent difference score variable (for detailed discussion on specifying LDS models see Henk & Castro-Schilo, Citation2015; McArdle, Citation2009).
To analyse the independent contribution of a single resource, LDS models were specified by regressing latent difference scores of psychosocial outcome variables on the latent difference scores of the three levels of resources separately. Upon identifying the significant independent contributions, a full LDS model was tested by regressing psychosocial outcome variables on all the three levels of resources at the same time, thus allowing to identify the relative contribution of a resource with others held constant. Prior to fitting LDS models, longitudinal invariance was tested for all latent constructs in order to ascertain latent construct comparability across two measurement points (see Little, Citation2013).
Latent constructs were identified by same observed indicators at each time point using parcels. When the key study question pertains to relationships among latent variables, parcels have several advantages over single items: superior psychometric properties (higher reliability and communality, better distributional properties) as well as more favourable intervals between scale points (Little, Cunningham, Shahar, & Widaman, Citation2002). In addition, they reduce the number of parameters in the model; a property that is especially beneficial with relatively small samples. Three parcels per scale were constructed using recommendations from Little, Rhemtulla, Gibson, and Schoemann (Citation2013). The parcel score was calculated as an average response across all items assigned to it. Latent constructs were scaled by constraining the indicator loadings to average 1.0 and indicator intercepts sum to zero for each construct (effects coding) (Little, Slegers, & Card, Citation2006). Latent variances are thus estimated as the average of indicators’ variances, and latent means as the optimally weighted average; resulting in latent variances and means estimation in the original response scale (Little, Citation2013; Little et al., Citation2006).
Although skew (SI) and kurtosis (KI) indices did not point to an extreme deviation from normality (for all variables in the analysis SI < |3| and KI <|10|) (Kline, Citation2011), multivariate skew and kurtosis tested by Mardia’s multivariate test were significantly different from normal (g1p = 38.4, p < .001, g2p = 206.22, p < .001). Therefore, robust standard errors and corrected model test statistics were used to assess models (see Kline, Citation2011). Models were considered to fit the data well when: the model χ2was non-significant (p< .01), the Root Mean Square Error of Approximation (RMSEA) was ≤.05, the Bentler Comparative Fit Index (CFI) was ≥ .95 and the Standardised Root Mean Square Residual (SRMR) was ≤ .08 (Hu & Bentler, Citation1999).
Multiple imputations (MI) were used to address participant dropout between the two time points of the study. MI were shown to work well with sample sizes as low as N = 50, multiple regressions up to 18 predictors and as much as 50% missing data in the dependent variable (Graham, Citation2009). One hundred multiple imputations were calculated and parameters and model fit indices are shown as pooled values (Enders & Mansolf, Citation2016).
Analyses were conducted in R (v 3.2.1; R Core Team, Citation2015), using the following packages: MVN (v 4.0; Korkmaz, Goksuluk, & Zararsiz, Citation2014), lavaan (v 0.5–22; Rosseel, Citation2012), semTools (v 0.4–11; semTools Contributors, Citation2016) and Amelia (v 1.7.4; Honaker, King, & Blackwell, Citation2011).
3. Results
Bivariate correlations of all variables prior to multiple imputations are reported in . At the first assessment point (T1), 32.7% (n= 73) of community members met the cut-off criteria for probable PTSD, while at the second assessment point (T2) the prevalence declined to 17.9%. (n = 40). Similarly, 35.4% (n= 79) of participants met the criteria for probable depression at T1, which declined to 18.4% (n = 41) at T2.
Table 3. Zero-order correlation of variables in the model.
In order to meaningfully compare latent constructs over time, longitudinal measurement invariance was tested for all six constructs in the analysis. The scalar model of invariance fitted the data well for all six constructs in the model (). allowing for a meaningful comparison of means across time. For all models, indicators represented the latent variables significantly (all at p< .001), while standardised coefficients ranged from .69 to .95.
3.1. Latent difference score models
Prior to fitting regression models with latent difference constructs, separate LDS models were analysed in order to estimate the mean and the variance of change scores (). The fit of these models is the same as the fit of intercept invariant model (). The means of all the latent difference scores for different types of resources (individual, interpersonal and community resources) as well as life satisfaction were non-significant, indicating no change in sample mean between the two time points. For post-traumatic stress symptoms, the mean change was significant (p< .001) and it indicated a small to medium sample level decrease in symptoms (M = −0.27, Cohen’s d = 0.43). For depression symptoms, the mean change was also significant (p< .001), indicating a small decrease in sample mean level of symptoms (M = −0.26, Cohen’s d = 0.36). The latent difference score variances were significant for all constructs (all p< .001), indicating significant between-person differences in a within-person change: for some participants, the score increased while for others it decreased over time.
Table 4. Means and variances of latent differences constructs.
3.2. Regression analysis
Single multivariate regression models were tested in order to analyse the independent contribution of different levels of resources (). Regressing psychosocial outcomes on individual resources resulted in a good model fit (χ2 (237) = 281.5, p= .04; CFI = .99; RMSEA (90% CI) = .02 (.00 – .03); SRMR = .07). The increase (decrease) in individual resources was related to a decrease (increase) in PTS symptoms and to an increase (decrease) in life satisfaction. Individual resources accounted for 6.8% of the change in PTS symptoms and 14.8% of the change in life satisfaction. Difference scores in individual resources were not related to difference scores in depression symptoms. The regression model with interpersonal resources also fitted the data well (χ2 (237) = 280.63, p= .04; CFI = .99; RMSEA (90% CI) = .03 (.01 – .04); SRMR = .07). The increase (decrease) in interpersonal resources was related to a decrease (increase) in PTS and depression symptoms, and an increase (decrease) in life satisfaction. Interpersonal resources predicted 11.2% of the variance of change in PTS symptoms, 5.1% in depression symptoms, and 23.1% in life satisfaction. Finally, specifying community resources as a predictor resulted in an acceptable model fit (χ2 (237) = 320.95, p= .001; CFI = .97; RMSEA (90% CI) = .04 (.02 – .05); SRMR = .08). The increase (decrease) in community resources was related to an increase (decrease) in life satisfaction, accounting for 6.2% of the variance. The change in community resources was not related to a change in PTS and depression symptoms.
Table 5. Standardised regression coefficients in single multivariate regression models predicting latent difference scores in PTS and depression symptoms and life satisfaction with latent difference scores in resources.
In the multiple structural regression model shown in , only significant regression paths from previous analysis were specified. The model fitted the data well (χ2 (567) = 653.92, p= .03; CFI = .98; RMSEA (90% CI) = .02 (.01 – .03); SRMR = .07). While controlling for other resources, an increase (decrease) in individual resources significantly predicted an increase (decrease) in life satisfaction, while the effect on PTS symptoms was close to statistical significance (p = .054). The change in interpersonal resources significantly predicted the change in all three psychosocial outcomes: an increase (decrease) in those resources was related to a decrease (increase) in PTS and depression symptoms and to an increase (decrease) in life satisfaction. The relationship between community resources and life satisfaction was no longer significant while controlling for other predictors. In total, resources accounted for 10% of the change in PTS symptoms, 3.5% in depression symptoms and 25.9% in life satisfaction.
Figure 1. Standardised coefficients in multiple multivariate regression model predicting latent difference scores in PTS and depression symptoms and life satisfaction with latent difference scores in resources.
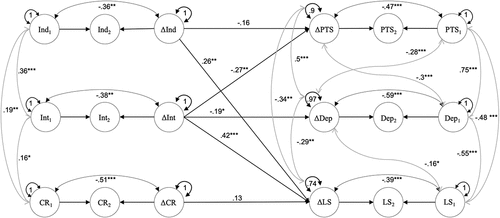
Covariances between all other latent constructs in the model were initially estimated freely; however, only significant covariances were included in the final model. All latent constructs at T1 correlated significantly, with the correlations ranging from r= .19 to r = .75 (). In addition, latent differences in the psychosocial outcomes correlated significantly ranging from r = −.29 to .5, as well as T1 level of PTS and depression symptoms with the change in depression and PTS symptoms, respectively, and T1 life satisfaction with the change in depression symptoms (). Latent differences in the resource variables did not correlate significantly (for individual and interpersonal resources r= .17, p= .095; for individual and community resources r = .11, p= .263; for interpersonal resources and community resources r = .18; p = .146).
4. Discussion
The current study aimed to contribute to a growing body of evidence supporting the role of resources in post-disaster psychosocial outcomes. It analysed the independent and relative contribution of change in individual, interpersonal and community resources to change in PTS and depression symptoms and life satisfaction after a flooding based on a dynamic process hypothesis derived from the Conservation of Resources (COR) theory. The change was observed directly, rather than retrospectively, over a time period between one and a half and two and a half years post-disaster, and modelled using the Latent Difference Score models (McArdle, Citation2009) thus addressing several of the gaps in previous studies.
One and a half years after a severe flooding, about 30% of the participants met the cut-off criteria for probable PTSD and depression. Although this percentage declined two and a half years post-disaster, the rates of potential mental health disorders remain high. Independently, the increase (decrease) in individual resources was related to a decrease (increase) in PTS symptoms (β = −.26; p= .01) and an increase (decrease) in life satisfaction (β = .39; p< .001). The increase (decrease) in interpersonal resources was related to a decrease (increase) in PTS (β = −.34; p< .001) and depression symptoms (β = −.23; p= .001) and an increase (decrease) in life satisfaction (β = .48; p< .001). The increase (decrease) in community resources was related to an increase (decrease) in life satisfaction (β = .25; p= .009). In the full LDS model, while controlling for other resources, community resources were no longer significantly related to life satisfaction (β = .13; p = .144).
The prevalence of probable PTSD and depression is comparable to the mid-range found in previous studies. The prevalence of disorders among direct disaster survivors ranges between 3.7% and 60% for PTSD (Neria, Nandi, & Galea, Citation2007) and 5.8% and 54% for depression (Tang, Liu, Liu, Xue, & Zhang, Citation2014), and declines over time (Goldmann & Galea, Citation2014; McFarlane, Citation1988; Norris, Murphy, Baker, & Perilla, Citation2004). In addition, the overall pattern of relationships in estimated models supports the role of resources in COR theory: the more the resources changed over the time period of the study, the greater was the change in psychosocial recovery. Importantly, resources at different levels of the ecological system predicted different psychosocial outcomes, with interpersonal resources as the strongest predictor of recovery.
That interpersonal resources were related to all of the measured outcomes came as no surprise: the support from a significant other, family and friends has consistently been found as a protective factor, contributing to good post-disaster outcomes (Bonanno et al., Citation2010, Citation2007; Kaniasty & Norris, Citation2008). Deterioration of social support was previously found to negatively impact psychological distress even in the mid- to long-term period after a disaster (Norris & Kaniasty, Citation1996). Disasters are characterised by numerous factors that can cause deterioration in social support: they disrupt social networks, increase expectations for support, interfere with social activities and can lead to conflicts related to aid distribution; all of which impact mental health and general well-being (Kaniasty & Norris, Citation2004). In other words, interpersonal resources can serve as a mesosystem, by fostering availability of other resources closer to the individual (Bronfenbrenner, Citation1977). They can be used to obtain different types of support, such as emotional, instrumental or informational, therefore contributing to mobilizing multiple resources and influencing several psychosocial outcomes at the same time.
Individual resources defined as relatively stable traits of hardiness and persistence reflect the ability to adapt to change, problems, illness, pressure, failure and painful feelings (Campbell-Sills & Stein, Citation2007). Consistently with the results of the current study, they have previously been found to be related to a range of mental health outcomes after disaster (Ahmad et al., Citation2010; Irmansyah et al., Citation2010; Ying et al., Citation2014), as well as personality traits, such as neuroticism, positive and negative affectivity, optimism and hardiness, all of which are important factors in post-disaster adaptation (Campbell-Sills, Cohan, & Stein, Citation2006; Connor & Davidson, Citation2003; Karaırmak, Citation2010). It has also been found that individual resources can increase following an intervention (e.g. Connor & Davidson, 2003); however to the authors’ knowledge, no previous study has examined whether they change in the aftermath of a disaster or the relationship of that change to psychosocial outcomes. That individual resources were not related to depression symptoms is an interesting finding, as they are conceptually related to the concept of mastery, the belief that one can solve one’s problems and respond effectively in times of stress; a construct that has been found to be related to a less depressed mood and should prevent feelings of helplessness often found in mood disorders (Yehuda, Flory, Southwick, & Charney, Citation2006). Our findings may reflect the higher importance of contextual resources in post-disaster settings and the crucial role of accessing support across broader networks (Bonanno et al., Citation2010), rather than the role of individual resources.
Finally, community resources, namely social capital and community engagement, exhibited a positive contribution through general life satisfaction. Studies on these resources are scarce; however, available evidence suggest that they are related to lower levels of anxiety and distress in the context of war (Braun-Lewensohn & Sagy, Citation2014; Kimhi & Eshel, Citation2009) and have a buffering effect against psychological distress after a series of natural disasters (Benight, Citation2004). In addition, a closely related resource, sense of community, was found to be related to subjective well‐being and life satisfaction in community-based samples (Davidson & Cotter, Citation1991; Prezza, Amici, Roberti, & Tedeschi, Citation2001). All of these studies examined related, but not identical aspects of community resources, in a wide variety of contexts and communities; therefore, the extent of the effect of community resources on psychosocial adaptation is yet to be determined. Although not directly beneficial for psychological health recovery in the current study, connectedness among members of the community, levels of trust, mutual helping and community efficacy enhance the quality of life and may contribute to the efforts to revitalise and rebuild the community after a disaster (Norris et al., Citation2008). Furthermore, the relationship between distal systems, risks or resources (those that are further away in relation to the individual in an ecological system) to individual-level outcomes is expected to be weaker, since they arise from the broader community or societal context and exhibit their influence through more proximal processes. This is also evidenced in the current study, where community resources were no longer a significant predictor of psychological adaptation after controlling for other resources. However, resources at distal levels of systems, such as community social capital and engagement, impact a large number of individuals at the same time and thus have an important value as a strategy for disaster preparedness and response (Norris et al., Citation2008).
It is important to emphasise that the mean level of resources during the course of the study remained the same. In addition, there were no significant relationships between changes in different resources, meaning that for any individual they changed independently: increase in one resource was not necessarily followed by an increase in the other. These findings call for targeted post-disaster interventions that can, by increasing the mean levels of resources in affected communities, decrease the levels of symptoms and increase well-being. Following the stepwise model of psychosocial support (Inter-Agency Standing Committee [IASC], Citation2007) and essential elements of mass trauma interventions (Hobfoll et al., Citation2007), the present study provides further evidence that such interventions should start by securing basic needs and safety, followed by family and community level interventions focused on fostering social support and connectedness. Interventions focused on recuperating individual resources may be needed later during the post-disaster recovery, and should target individuals who experience higher mental health risks. However, the research interests up to date do not reflect the relative need for better understanding of the role of different levels of interventions. There is a disproportional amount of evidence of successful interventions at the individual level compared to the community level. This gap is largely due to major challenges of doing research at the community level in the immediate aftermath of disasters; nevertheless, such studies are essential to determine potential benefits and pitfalls of community-level interventions (Bonanno et al., Citation2010).
There are several limitations to this study. It should be noted that the sample size relative to the number of estimated parameters in the models is relatively small. However, the requirement of a large sample size in SEM models is most often related to specific distributional assumptions, namely multivariate normality. Since robust estimators are used in this study, this limitation is somewhat addressed. In addition, new developments in the field of structural equation modelling point to relatively small gains in mean and variance confidence intervals above the sample size of 150 (Little, Citation2013), arguing that much smaller samples are required compared to earlier recommendations. Other limitations pertain to methodological and theoretical considerations. The first time point of measurement in this study was one and a half years after the disaster, which might have resulted in lower means and variances of change and reduced the size of regression coefficients. In addition, refusals at T1 due to not wanting to be reminded of the floods could have further reduced the estimated psychosocial effects of the disaster. However, since the means of PTS and depression symptoms were still changing between two time points, this indicates that the community was still recovering from the disaster. Next, community resilience in this study is measured through individual-level perceptions, and can more precisely be understood as the availability of those resources to that individual. Although these perceptions are likely to be embedded in the actual resources available in the community, this cannot be ascertained without a cross-community examination of both community-level estimates and individual perceptions. The emerging literature on the role of community resources in post-disaster outcomes continues to rely on aggregating individual-level perceptions, and even though measures of community resources for archival, population-level data have been developed (e.g. Sherrieb, Norris, & Galea, Citation2010), they have not been applied yet to studies of psychological outcomes after adversity. In addition, given the correlational nature of the study, it is possible that individual characteristics as well as current levels of mental health and well-being affected estimates of resources. However, previous studies found that resource loss is an important predictor of post-disaster outcomes over and beyond pre-disaster psychological functioning (e.g. Zwiebach et al., Citation2010), suggesting the resources-to-outcomes relationship. Finally, the percentages of the explained change in the psychosocial outcomes, especially for depression symptoms, are relatively small. This indicates that there are numerous resources that contribute to post-disaster recovery, and further research is needed to establish their relative importance.
In conclusion, this study emphasises the role of dynamic change of different levels of resources in psychosocial outcomes in communities after disasters. It supports the position that understanding resources, their trajectories and change, is one of the most important challenges for future research (Norris et al., Citation2008). Identifying resources at individual, but especially at community and society level that can be easily mobilised, that are robust to disaster impact and can be increased by interventions, is of paramount importance to disaster preparedness and response. Future studies would benefit from including more time points in both the immediate aftermath of disasters as well in the long-term period and examining the factors that affect resource change.
Disclosure statement
No potential conflict of interest was reported by the authors.
Additional information
Funding
References
- Ahmad, S., Feder, A., Lee, E. J., Wang, Y., Southwick, S. M., Schlackman, E., … Charney, D. S. (2010). Earthquake impact in a remote South Asian population: Psychosocial factors and posttraumatic symptoms. Journal of Traumatic Stress, 23(3), 408–13.
- Bakic, H. (2017, May). Community resilience: Measurement and implications. Paper presented at the ERFCON 2017 Preconference Growing Resilience: Researching Beyond Academia, Zagreb, Croatia.
- Benight, C. C. (2004). Collective efficacy following a series of natural disasters. Anxiety, Stress and Coping, 17(4), 401–420.
- Benight, C. C., Cieslak, R., & Waldrep, E. (2009). Social and cognitive frameworks for understanding the mental health consequences of disasters. In Y. Neria, S. Galea, & F. H. Norris (Eds.), Mental health and disasters (pp. 161–174). New York: Cambridge University Press.
- Benight, C. C., Ironson, G., Klebe, K., Carver, C. S., Wynings, C., Burnett, K., … Schneiderman, N. (1999). Conservation of resources and coping self-efficacy predicting distress following a natural disaster: A causal model analysis where the environment meets the mind. Anxiety, Stress and Coping, 12(2), 107–126.
- Bobovec, B., Mandić, R., & Pozojević, A. (2016). Results of the programme of reconstruction of buildings and remediation of flood consequences in the territory of Vukovar-Srijem County. Polytechnic and Design, 4(1), 37–43.
- Bonanno, G. A., Brewin, C. R., Kaniasty, K., & La Greca, A. M. (2010). Weighing the costs of disaster. Psychological Science in the Public Interest, 11(1), 1–49.
- Bonanno, G. A., Galea, S., Bucciarelli, A., & Vlahov, D. (2007). What predicts psychological resilience after disaster? The role of demographics, resources, and life stress. Journal of Consulting and Clinical Psychology, 75(5), 671–682.
- Bovin, M. J., Marx, B. P., Weathers, F. W., Gallagher, M. W., Rodriguez, P., Schnurr, P. P., & Keane, T. M. (2016). Psychometric properties of the PTSD checklist for diagnostic and statistical manual of mental disorders–fifth edition (PCL-5) in veterans. Psychological Assessment, 28(11), 1379–1391. doi:10.1037/pas0000254
- Braun-Lewensohn, O., & Sagy, S. (2014). Community resilience and sense of coherence as protective factors in explaining stress reactions: Comparing cities and rural communities during missiles attacks. Community Mental Health Journal, 50(2), 229–234.
- Bronfenbrenner, U. (1977). Toward an experimental ecology of human development. American Psychologist, 32(7), 513–531.
- Campbell-Sills, L., Cohan, S. L., & Stein, M. B. (2006). Relationship of resilience to personality, coping, and psychiatric symptoms in young adults. Behaviour Research and Therapy, 44(4), 585–599.
- Campbell-Sills, L., & Stein, M. B. (2007). Psychometric analysis and refinement of the Connor–Davidson resilience scale (CD-RISC): Validation of a 10-item measure of resilience. Journal of Traumatic Stress, 20(6), 1019–1028.
- Connor, K. M., & Davidson, J. R. T. (2003). Development of a new resilience scale: The connor-davidson resilience scale (CD-RISC). Depression and Anxiety, 18(2), 76–82. doi:10.1002/da.10113
- Davidson, W. B., & Cotter, P. R. (1991). The relationship between sense of community and subjective wellbeing: A first look. Journal of Community Psychology, 19(3), 246–253.
- Diener, E., Emmons, R. A., Larsen, R. J., & Griffin, S. (1985). The satisfaction with life scale. Journal of Personality Assessment, 49(1), 71–75.
- Eaton, W. W., Smith, C., Ybarra, M., Muntaner, C., & Tien, A. (2004). Center for Epidemiologic Studies Depression Scale: Review and revision (CESD and CESD-R). In M. E. Maruish (Ed.), The use of psychological testing for treatment planning and outcomes assessment (3rd ed., pp. 363–379). Mahwah, NJ: Lawrence Erlbaum.
- Enders, C. K., & Mansolf, M. (2016). Assessing the fit of structural equation models with multiply imputed data. Psychological Methods, 23(1), 76–93.
- Freedy, J. R., Shaw, D. L., Jarrell, M. P., & Masters, C. R. (1992). Towards an understanding of the psychological impact of natural disasters: An application of the conservation resources stress model. Journal of Traumatic Stress, 5(3), 441–454.
- Goldmann, E., & Galea, S. (2014). Mental health consequences of disasters. Annual Review of Public Health, 35(1), 169–183.
- Graham, J. W. (2009). Missing data analysis: Making it work in the real world. Annual Review of Psychology, 60(1), 549–576.
- Halbesleben, J. R. B., Neveu, J.-P., Paustian-Underdahl, S. C., & Westman, M. (2014). Getting to the “COR”. Journal of Management, 40(5), 1334–1364.
- Henk, C. M., & Castro-Schilo, L. (2015). Preliminary detection of relations among dynamic processes with two-occasion data. Structural Equation Modeling: A Multidisciplinary Journal, 23(2), 180–193.
- Henry, B., Moffitt, T. E., Caspi, A., Langley, J., & Silva, P. A. (1994). On the “remembrance of things past”: A longitudinal evaluation of the retrospective method. Psychological Assessment, 6(2), 92–101.
- Hobfoll, S. E. (1989). Conservation of resources: A new attempt at conceptualizing stress. American Psychologist, 44(3), 513–524.
- Hobfoll, S. E. (2001). The influence of culture, community, and the nested-self in the stress process: Advancing conservation of resources theory. Applied Psychology, 50(3), 337–421.
- Hobfoll, S. E., Canetti-Nisim, D., & Johnson, R. J. (2006). Exposure to terrorism, stress-related mental health symptoms, and defensive coping among Jews and Arabs in Israel. Journal of Consulting and Clinical Psychology, 74(2), 207–218.
- Hobfoll, S. E., Johnson, R. J., Ennis, N., & Jackson, A. P. (2003). Resource loss, resource gain, and emotional outcomes among inner city women. Journal of Personality and Social Psychology, 84(3), 632–643.
- Hobfoll, S. E., Tracy, M., & Galea, S. (2006). The impact of resource loss and traumatic growth on probable PTSD and depression following terrorist attacks. Journal of Traumatic Stress, 19(6), 867–878.
- Hobfoll, S. E., Watson, P., Bell, C. C., Bryant, R. A., Brymer, M. J., Friedman, M. J., … Ursano, R. J. (2007). Five essential elements of immediate and mid-term mass trauma intervention: Empirical evidence. Psychiatry, 70(4), 283–315.
- Honaker, J., King, G., & Blackwell, M. (2011). Amelia II: A program for missing data. Journal of Statistical Software, 45(7). doi:10.18637/jss.v045.i07
- Hu, L., & Bentler, P. M. (1999). Cutoff criteria for fit indexes in covariance structure analysis: Conventional criteria versus new alternatives. Structural Equation Modeling: A Multidisciplinary Journal, 6(1), 1–55.
- Inter-Agency Standing Committee (IASC). (2007). IASC guidelines on mental health and psychosocial support in emergency settings. Retrieved from https://interagencystandingcommittee.org/mental-health-and-psychosocial-support-emergency-settings-0/documents-public/iasc-guidelines-mental
- Irmansyah, I., Dharmono, S., Maramis, A., & Minas, H. (2010). Determinants of psychological morbidity in survivors of the earthquake and tsunami in Aceh and Nias. International Journal of Mental Health Systems, 4(1), 8.
- Kaniasty, K., & Norris, F. H. (2004). Social support in the aftermath of disasters, catastrophes, and acts of terrorism: Altruistic, overwhelmed, uncertain, antagonistic, and patriotic communities. In R. Ursano, A. Norwood, & C. Fullerton (Eds.), Bioterrorism: Psychological and public health interventions (pp. 200–229). Cambridge: Cambridge University Press.
- Kaniasty, K., & Norris, F. H. (2008). Longitudinal linkages between perceived social support and posttraumatic stress symptoms: Sequential roles of social causation and social selection. Journal of Traumatic Stress, 21(3), 274–281.
- Karaırmak, Ö. (2010). Establishing the psychometric qualities of the Connor–Davidson Resilience Scale (CD-RISC) using exploratory and confirmatory factor analysis in a trauma survivor sample. Psychiatry Research, 179(3), 350–356.
- Kimhi, S. (2016). Levels of resilience: Associations among individual, community, and national resilience. Journal of Health Psychology, 21(2), 164–170.
- Kimhi, S., & Eshel, Y. (2009). Individual and public resilience and coping with long-term outcomes of war. Journal of Applied Biobehavioral Research, 14(2), 70–89.
- Kline, R. B. (2011). Principles and practice of structural equation modeling (3rd ed). Methodology in the social sciences. New York: Guilford Press.
- Korkmaz, S., Goksuluk, D., & Zararsiz, G. (2014). MVN: An R package for assessing multivariate normality. The R Journal, 6(2), 151–162.
- Little, T. D. (2013). Longitudinal structural equation modeling. Methodology in the social sciences. New York: Guildford.
- Little, T. D., Cunningham, W. A., Shahar, G., & Widaman, K. F. (2002). To parcel or not to parcel: Exploring the question, weighing the merits. Structural Equation Modeling: A Multidisciplinary Journal, 9(2), 151–173.
- Little, T. D., Rhemtulla, M., Gibson, K., & Schoemann, A. M. (2013). Why the items versus parcels controversy needn’t be one. Psychological Methods, 18(3), 285–300.
- Little, T. D., Slegers, D. W., & Card, N. A. (2006). A non-arbitrary method of identifying and scaling latent variables in SEM and MACS models. Structural Equation Modeling: A Multidisciplinary Journal, 13(1), 59–72.
- Masten, A. S., & Narayan, A. J. (2012). Child development in the context of disaster, war, and terrorism: Pathways of risk and resilience. Annual Review of Psychology, 63(1), 227–257.
- McArdle, J. J. (2009). Latent variable modeling of differences and changes with longitudinal data. Annual Review of Psychology, 60(1), 577–605.
- McFarlane, A. C. (1988). The longitudinal course of posttraumatic morbidity the range of outcomes and their predictors. The Journal of Nervous and Mental Disease, 176(1), 30–39.
- Moffitt, T. E., Caspi, A., Taylor, A., Kokaua, J., Milne, B. J., Polanczyk, G., & Poulton, R. (2010). How common are common mental disorders? Evidence that lifetime prevalence rates are doubled by prospective versus retrospective ascertainment. Psychological Medicine, 40(6), 899–909.
- National Protection and Rescue Directorate. (2015). Poplave u Vukovarsko-srijemskoj županiji u svibnju 2014 [Flooding in Vukovar-Syrmia County in May 2014]. Retrieved from http://stari.duzs.hr/news.aspx?newsID=22140&pageID=677
- Neria, Y., Nandi, A., & Galea, S. (2007). Post-traumatic stress disorder following disasters: A systematic review. Psychological Medicine, 38(04). doi:10.1017/s0033291707001353
- Newsom, J. T. (2015). Longitudinal structural equation modeling: A comprehensive introduction. Multivariate applications series. New York, NY: Routledge.
- Norman, G. (2003). Hi! How are you? Response shift, implicit theories and differing epistemologies. Quality of Life Research, 12(3), 239–249.
- Norris, F. H., & Kaniasty, K. (1996). Received and perceived social support in times of stress: A test of the social support deterioration deterrence model. Journal of Personality and Social Psychology, 71(3), 498–511.
- Norris, F. H., Murphy, A. D., Baker, C. K., & Perilla, J. L. (2004). Postdisaster PTSD over four waves of a panel study of Mexico’s 1999 flood. Journal of Traumatic Stress, 17(4), 283–292.
- Norris, F. H., Stevens, S. P., Pfefferbaum, B., Wyche, K. F., & Pfefferbaum, R. L. (2008). Community resilience as a metaphor, theory, set of capacities, and strategy for disaster readiness. American Journal of Community Psychology, 41(1–2), 127–150.
- Prezza, M., Amici, M., Roberti, T., & Tedeschi, G. (2001). Sense of community referred to the whole town: Its relations with neighboring, loneliness, life satisfaction, and area of residence. Journal of Community Psychology, 29(1), 29–52.
- R Core Team. (2015). R: A language and environment for statistical computing. Retrieved from http://www.R-project.org/
- Rosseel, Y. (2012). lavaan: An R package for structural equation modeling. Journal of Statistical Software, 48(2). doi:10.18637/jss.v048.i02
- Sattler, D. N., de Alvarado, A. M. G., de Castro, N. B., van Male, R., Zetino, A. M., & Vega, R. (2006). El Salvador earthquakes: Relationships among acute stress disorder symptoms, depression, traumatic event exposure, and resource loss. Journal of Traumatic Stress, 19(6), 879–893.
- semTools Contributors. (2016). semTools: Useful tools for structural equation modeling. R package version 0.4-11. Retrieved from http://cran.r-project.org/package=semTools
- Sherrieb, K., Norris, F. H., & Galea, S. (2010). Measuring capacities for community resilience. Social Indicators Research, 99(2), 227–247.
- Smith, B. W., & Freedy, J. R. (2000). Psychosocial resource loss as a mediator of the effects of flood exposure on psychological distress and physical symptoms. Journal of Traumatic Stress, 13(2), 349–357.
- Tang, B., Liu, X., Liu, Y., Xue, C., & Zhang, L. (2014). A meta-analysis of risk factors for depression in adults and children after natural disasters. BMC Public Health, 14(1). doi:10.1186/1471-2458-14-623
- Thompson, M. S., & Cooper, C. L. (2001). A rose by any other name: A commentary on Hobfoll’s conservation of resources theory. Applied Psychology: An International Review, 50(3), 408–418.
- United Nations Office for Disaster Risk Reduction. (2009). UNISDR Terminology on disaster risk reduction. Retrieved from http://www.unisdr.org/we/inform/publications/7817
- Weathers, F. W., Litz, B. T., Keane, T. M., Palmieri, P. A., Marx, B. P., & Schnurr, P. P. (2013). The PTSD Checklist for DSM-5 (PCL-5). Scale available from the National Center for PTSD. Retrieved from www.ptsd.va.gov
- Yehuda, R., Flory, J. D., Southwick, S., & Charney, D. S. (2006). Developing an agenda for translational studies of resilience and vulnerability following trauma exposure. Annals of the New York Academy of Sciences, 1071, 379–396.
- Ying, L., Wu, X., Lin, C., & Jiang, L. (2014). Traumatic severity and trait resilience as predictors of posttraumatic stress disorder and depressive symptoms among adolescent survivors of the Wenchuan earthquake. PLoS One, 9(2), e89401.
- Zimet, G. D., Dahlem, N. W., Zimet, S. G., & Farley, G. K. (1988). The multidimensional scale of perceived social support. Journal of Personality Assessment, 52(1), 30–41.
- Zwiebach, L., Rhodes, J., & Roemer, L. (2010). Resource loss, resource gain, and mental health among survivors of Hurricane Katrina. Journal of Traumatic Stress, 23(6), 751–758.
Appendix
Table A1. Model fit results for testing measurement invariance across measurement points for constructs in the model.