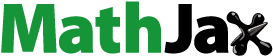
ABSTRACT
Introduction: This paper presents an option for evaluating food control effectiveness by analysing the frequency of non-compliances (FnC).
Material and methods: A food business establishment can have several different types of control areas (i.e. pest control, HACCP), that can be inspected to assess its compliance with regulations in the food sector. From April 2012 to April 2014, 10 736 inspections were performed in Sweden, covering all 15 types of control areas. In these inspections, 2223 non-compliances were found, giving a FnC of 0.21 per control area inspected. Outlying types of control areas, inspection teams and establishments were selected for supervision of the internal audit procedure.
Results and discussion: The key and surprising finding was that types of control area, teams and establishments with high FnC had a higher ratio of false negative non-compliances than those with low FnC. Moreover, false negative non-compliances were more common than false positive non-compliances. Possible explanations include the complexity of legislation affecting food businesses and the complexities of the food business.
Conclusions: The risk of non-compliance going undetected is greatest where many non-compliances have already been detected. These results should inform future food control strategies.
Introduction
For the consumer, efficient food control means safe, honest and unadulterated foods. For the food business operator (FBO), efficient food control should also ensure fairness in terms of implementation of legislation, foreseeability of food control decisions and level competition with other food businesses. FBOs are responsible for the food safety in their establishments. For national food safety authorities (NFSA), ‘effectiveness’ is defined as the ability of the NFSA to ensure high quality of official controls (EU Member States Network on National Audit System [NAS]), [Citation1]. Each NFSA must also ensure the quality, impartiality and consistency of official control processes and good cost efficiency. To achieve these aims, the NFSA must carry out internal and external audits and take corrective measures. Furthermore, according to EU regulation EC No. 882/2004 [Citation2], the NFSA must have audit procedures in place to verify the effectiveness of official controls and to ensure that corrective action is taken when needed and that appropriate and up-to-date documentation is available. However, verification of the effectiveness of official controls is an open question, as EU regulations do not prescribe a specific method. To verify the effectiveness of official controls, the Food and Veterinary Office (FVO) of the European Commission (EC) has audited the controls in seven European Union (EU) Member States, with the focus on the control procedures [Citation3]. FVO defines effectiveness as the ‘extent to which official controls produce an intended effect and/or achieve an objective’ [Citation1]. The objective referred to by FVO is ensuring safe food through official controls that detect non-compliances.
This paper presents one option for evaluating food control effectiveness, namely by analysing the frequency of non-compliances (FnC) with food regulations. The question is whether these non-compliances are false positive or false negative. The efficiency of food control activities is reflected in detection of non-compliances with food safety and quality regulations at food business establishments and subsequent corrective actions. We propose that efficient food control is built on two pillars. The first pillar is that, if complied with, the regulations achieve the objectives of safe food, high food quality, good cost-effectiveness, transparency and absence of fraud, and fair and equitable regulation of food businesses. The second pillar, which is the subject of this paper, is monitoring compliance with the food regulations by different food business establishments. In particular, we examined what FnC actually indicates with regard to food safety regulations. To analyse food control efficiency, the NFSA in Sweden (the National Food Agency, NFA) has established an internal audit procedure referred to as supervision [Citation4,Citation5]. The first step in the supervision procedure is statistical screening to detect outliers in FnC for different determinants, such as categories of establishments, types of control areas and different inspection teams. Thereafter, for a selected sample of these identified outliers (high or low FnC values), a team of skilled inspectors (supervisors) re-examines the findings through examination of written procedures and performance, documentation of control and inspections of the premises.
The present paper focuses on the outliers, i.e. food business establishments with either a high FnC or a low FnC, looking at the type of control area, the category of establishments and the different inspection teams. A high frequency could indicate actual problems with compliance or too strict inspections, while a low frequency could indicate good compliance or lax inspections.
Material and methods
Organisation of official food controls by the NFA
The NFA has four regional food control divisions, each of which is divided into local inspection teams consisting of 6–15 food control inspectors. In 2014, the NFA had 220 full-time employees, allocated to 17 local inspection teams.
Categories of establishments and risk classes
The NFA had oversight of around 850 food establishments in 2013, including those approved according to EU regulation EC No. 853/2004 [Citation6]and other registered food production establishments such as wine and spirits producers, and railway and aircraft catering. The approved establishments are categorised according to the European Commission Technical Specification Citation[7] (). Furthermore, the establishments are classified into risk classes based on the volume of products produced, the type of food and the type of processing activity [Citation8]. Establishments with more than one food processing activity are categorised as posing the highest risk. The calculation of inspection hours for establishments in different risk classes has been risk-based since 2007 [Citation8]. Thus, more inspection hours are allocated to food establishments with activities representing a higher risk or with larger production volumes. Furthermore, to comply with legal obligations, the NFA has published a control manual [Citation9] that serves as a guideline for competent food safety authorities in Sweden. The manual points out that the general objective for the competent authorities (safe food and risk-based, legally secure and effective controls) should be achieved by detecting non-compliances at food business establishments. The Food Control Department at the NFA has also issued instructions for the supervision of official inspections, in order to evaluate written guidelines, verify compliance with written guidelines and, through analysis of the results, assess the effectiveness of official food controls [Citation4].
Table 1. Frequency of non-compliances for different categories of food business establishments in Sweden, April 2012-April 2014. Bold text indicates categories outside the 95% confidence interval (CI) of the mean frequency of non-compliances. Highlighted categories* of establishments were selected for the supervision procedure.
Types of food control areas
In Sweden, the official food control inspection of an establishment may include one or more of 15 different types of food control areas. These are: 1) Layout, design, construction of food premises and equipment; 2) raw materials and packaging materials; 3) handling, storage and transport of foods; 4) handling and storage of waste; 5) pest control; 6) cleaning and disinfection; 7) water supply; 8) temperature control; 9) personal hygiene; 10) training; 11) hazard analysis and critical control plan (HACCP); 12) food information and labelling; 13) traceability; 14) microbiological criteria; and 15) other requirements. There are additional, more specific, types of control areas for slaughterhouses and meat cutting plants. However, these specific types of control areas and area 15 (‘other requirements’) were excluded from the present analysis.
Study period and population
During the study period, from April 2012 to April 2014, 10,736 control areas were inspected and 2223 non-compliances were found, giving an overall frequency of non-compliance of 0.21 per control area inspected ().
Reporting official food control inspections
In 2012, the NFA launched a monitoring system for reporting official food control inspections. The results of official inspections are documented using an internet-based application and contain information about the date, attendance, control areas inspected, non-compliances found, etc., together with basic facts about the establishment. During the study period, the system was operational in terms of reporting results from official control inspections. Inspection results relating to approval of establishments, ad hoc inspections, and additional official inspections were excluded.
Performance of supervision
The present study was based on statistical screening of the FnC results followed by supervision for selected outlying official food inspections, as described by Berking et al. [Citation4,Citation5]. In this procedure, supervisors reassess the performance of official inspections and the findings obtained and compare their findings against the initial findings reported by the inspection team. This approach has the advantage of making direct observations and evaluations on the same site and in the same situation as covered in the ordinary food inspection, because the supervisions are carried out concurrently with the official inspections.
Metrics used
In each food establishment, one or more control areas might be inspected. For each of these control areas, zero, one or more non-compliances were recorded in this study. The unit of interest in the study was the frequency of non-compliances (FnC), as defined in Equation 1.
For every supervision made on inspections taking place at establishments, the outcome can be true or false non-compliance and false non-compliance can be positive or negative. During the supervisions selected for this study, the food establishment was inspected and the results were compared with those of the ordinary inspection, using two metrics expressing the relative magnitude of false positive and false negative results (Equations 2 and 3).
Selection procedure for supervision
The selection of categories of establishments, teams and types of control areas for supervision was based on the following four-step procedure:
Selection of categories of establishments
The first step was aimed at detecting outliers or categories of establishments with FnC outside the 95% confidence interval (CI) of the mean. These outliers are shown in as bold text. The second step was to scrutinise the selected categories of establishments with regard to their importance, defined as number of control areas inspected per category. Thus slaughterhouses and meat product plants were identified as establishments with high FnC, while egg packing plants and factory fishing vessels were identified as establishments with low FnC.
Selection of inspection teams
The third step was selection of inspection teams from amongst those used in inspecting the selected categories of establishments. For egg packing plants, slaughterhouses and meat product plants, the mean and 95% CI of the FnC were calculated for each inspection team, and teams with FnC values outside the 95% CI were selected for each previously selected category of establishment (–). In the study period (April 2012-April 2014), this comprised two teams with high FnC and five teams with low FnC.
Table 2. Egg packing plants. Frequency of non-compliances for different food control inspection teams. Bold text indicates teams outside the 95% confidence interval (CI) of the mean frequency of non-compliances. Highlighted* teams were selected for the supervision procedure.
Table 3. Slaughterhouses. Frequency of non-compliances for different food control inspection teams. Bold text indicates teams outside the 95% confidence interval (CI) of the mean frequency of non-compliances. Highlighted* teams were selected for the supervision procedure.
Table 4. Meat product plants. Frequency of non-compliances for different food control inspection teams. Bold text indicates teams outside the 95% confidence interval (CI) of the mean frequency of non-compliances. Highlighted* teams were selected for the supervision procedure.
Selection of the type of control areas
The fourth step was selection of types of control areas amongst the selected category of establishments and the selected inspection teams. For slaughterhouses and meat product plants, mean FnC and its 95% CI were calculated for each type of control area. The types of control areas with FnC values outside the 95% CI were identified (, ). For practical reasons, the selection for supervision was limited to the types of control areas planned to be inspected during 2015. For slaughterhouses, raw materials and packaging materials were the type of control areas selected for supervision. For meat product plants, HACCP, food information and labelling, personal hygiene and training were selected. For the categories of establishments with low frequency of non-compliances (egg packing plants and factory fishing vessels), there were insufficient data to select types of control areas. For these establishments, all planned control areas were selected for supervision. The screening process identified 22 official inspections, including at least 45 control areas that should be supervised.
Table 5. Slaughterhouses. Frequency of non-compliances for control areas. Bold text indicates control areas outside the 95% confidence interval (CI) of the mean frequency of non-compliances. Highlighted* control areas were selected for the supervision process.
Table 6. Meat product plants. Frequency of non-compliances for different control areas. Bold text indicates control areas outside the 95% confidence interval (CI) of the mean frequency of non-compliances. Highlighted* control areas were selected in the screening process.
On-the-spot supervision
On-the-spot supervision was performed on the teams, categories of establishments and types of control areas selected according to the statistical selection process and selection criteria described above. The supervisor informed the inspection teams to be supervised about the establishments and types of control areas that had been chosen for the supervision. The team manager appointed members of the team to attend the supervision. The supervision was made as one inspection visit. During the inspection, one or more control areas were supervised. The supervisor participated as a passive observer during the official inspection procedure and examined the documentation for the actual food control inspection. The supervisor also evaluated the performance of the food control inspection procedure and subsequent documentation. False negative non-compliance frequencies (Eq. 2) were recorded by the supervisor, as they indicated that the initial inspection did not detect non-compliance, did not further investigate non-compliance or detected non-compliance but assessed it as being in compliance. False positive non-compliance frequencies (Eq. 3) were also recorded by the supervisor, as they indicated that the initial inspection had wrongly assessed observations during inspection as being in non-compliance or that the assessment had insufficient legal support. These supervision findings were discussed with the inspection team members before being presented to the group of supervisors. The supervisions were then documented in a separate report addressed to the team manager and the head of division. Of the 22 selected official food control inspections, 21 were subject to on-the-spot supervisions during 2015. One supervision could not be carried out due to unavailability of a supervisor. Data collected from the on-the-spot supervisions and preparation process were analysed for differences between teams, categories of establishments and types of control areas.
Data analysis
For statistical analyses, Fisher´s exact test was used.
Results
The results are presented in – and –.
Figure 1. Frequency of observations judged false negative (neg) and false positive (pos) per supervision in control areas with a high and low frequency of non-compliances (FnC). The results do not include categories of establishments with a low frequency of non-compliances.
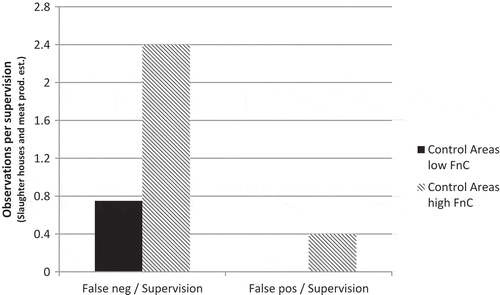
In 11 out of 21 supervisions, false non-compliances were found. In total, there were 25 false non-compliances, of which 22 (88%) were false negatives and only three (12%) were false positives. In most cases, only 1–2 false non-compliances per supervision were found (nine of 11 supervisions), while in two supervisions there were six and seven false non-compliances, respectively.
The frequency of false negative non-compliances was higher for types of control areas with high FnC (2.4) than for control areas with low FnC (0.75) (), but there was no significant difference between types of control areas. In slaughterhouses and meat product plants (high-risk establishments) 20 false non-compliances were found, of which 18 were false negative and two were false positive. For control areas with high FnC, the actual number of false negatives and false positives was 12 and 2, respectively. For control areas with low FnC, the corresponding values were 6 and 0, respectively.
The frequency of false negative non-compliance was higher for categories of establishments with high FnC (1.38) than for establishments with low FnC (0.50) (), but there was no significant difference between categories of establishments. The number of false positives was 1 and 2 for categories of establishments with low and high FnC, respectively, while the number of false negatives was 4 and 18 for categories of establishments with low and high FnC, respectively.
Figure 2. Frequency of observations judged false negative and false positive per supervision in categories of establishments (est.) with a high and low frequency of non-compliances (FnC).
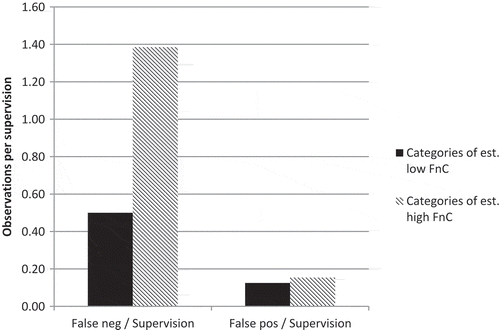
The frequency of false negative non-compliances was higher for teams with high FnC (1.33) than for teams with low FnC (0.93) (). The absolute number of false positive non-compliances was small, one for teams with low FnC and two for teams with high FnC.
Discussion
The key finding
The key and somewhat surprising finding was that types of control areas, teams and establishments with high FnC had a higher ratio of false negative non-compliances than teams, establishments and control areas with low FnC. Moreover, false negative non-compliances were much more common than false positive non-compliances. This means that the current food control inspection system is failing to detect all non-compliances, in particular in problem settings. There is thus a need to analyse the determinants of these false non-compliances. We interpret these findings as supporting the claim that deficiencies in food control effectiveness can arise more often in types of control areas and establishments with high FnC. The results also illustrate that FnC can be used as one of several indicators for concerns or higher risks in terms of food safety. A high FnC for a type of control area or establishment indicates that the FBO has not succeeded in meeting the statutory requirements for that control area or establishment. A high FnC can also indicate a greater underlying problem, since supervision of official inspections found a high frequency of non-compliances not identified by the official inspections.
Types of control areas
Most false negative non-compliances were found in types of control areas with high FnC. Complexity could be the main reason for this, as control areas with high FnC (HACCP and food information and labelling) are more complicated to inspect than those with low FnC (personal hygiene and training). HACCP is a broad type of control area and covers both the food production process and process-specific hazards, which vary widely depending on what is produced. Food information and labelling are covered by a broad range of legislation and involve activities such as prevention of food fraud, mandatory food information and protection of designations of origin, nutrition and health claims, weights and volumes. During the supervision performed in the present study, most false non-compliances were found in control areas with high FnC, i.e. the inspectors did not identify all non-compliances in the more complex types of control areas.
According to the supervisors, lack of sufficient experience among food control inspectors is also a determinant for false negative non-compliances. A possible explanation is that inspectors need broader knowledge about production processes and process-specific hazards and more experience in order to perform accurate food control inspections in complex settings.
Teams
The findings were similar for all teams studied. A possible explanation for the relatively small difference in ratios between the teams is that the composition of the teams changed over time, thereby levelling out any differences, while the categories of establishments and the control areas remained the same.
According to Läikkö-Roto et al. [Citation10], introduction of new inspection staff may impair the quality and efficacy of official food controls. Our results supported that finding, as results from two particular supervisions contributed nearly 60% (13 of 22) of the observed false non-compliances. In both cases, the controls were performed by inspectors with little experience of the actual type of control area or establishment. One of these supervisions was performed at a team with high FnC (seven false non-compliances) and the other at a team with low FnC (six false non-compliances). These findings support the hypothesis that the assessment of observations made during official food control inspections is dependent on the individual inspector’s knowledge and experience [Citation11]. Official controls at establishments with complex types of control areas, i.e. high FnC, require highly experienced and trained inspectors. The first author has also experienced that inspectors tend to report less non-compliances if they already found non-compliances. Especially minor non-compliances can be under-reported. In future inspections, one priority should be to ensure that the teams are well-balanced in terms of sufficient experience and knowledge.
Establishments
Establishments with high FnC (slaughterhouses and meat product plants) have more complex production processes than establishments with low FnC (egg packing plants and factory fishing vessels). Slaughter and meat processing both involve intensive manipulation and handling of meat and, since many foodborne pathogens can be harboured in the gastrointestinal tract of food-producing animals, there are significant risks of cross-contaminations during handling. Egg packing plants in Sweden only handle eggs from hens and there are few hazards during processing, so eggs are eaten whole have a limited risk of cross-contamination. Factory fishing vessels in Sweden only handle shrimps, which are boiled after capture at sea.
There is also a difference in complexity in the regulations between high FnC and low FnC establishments, in that it is more difficult for a slaughterhouse or meat product plant to ensure that its complex food production processes comply with the food regulations. Complex food business establishments often have to follow complex regulations, so there is a correlation with control areas. Furthermore, since high FnC establishments have higher risks in their production, they are categorised as high-risk establishments, which results in more inspection hours and consequently more frequent and longer inspections than for low-risk establishments. In future assessments, the importance of considering the complexities in establishments and in regulations when interpreting the results of FnC should be borne in mind. Comparisons of FnC between different categories of establishments can be biased due to differences in complexity, while comparisons within a category of establishments should be easier to interpret.
What is effectiveness?
We could not find any published information on to measure the effectiveness of official food controls using the definition of effectiveness defined by NAS, i.e. the extent to which official controls produce an intended effect and/or achieve an objective such as consumer food safety. A study in Finland [Citation12] approached the subject by evaluating official food controls and found that the use of checklists and templates for inspection reports enhanced the consistency and efficacy of inspections. The templates also reduced the time required and increased the quality of reporting. Time limits for correcting non-compliances at food business establishments had a significant effect on the efficacy of controls [Citation12]. Those authors concluded that food control efficacy is determined by the extent of correcting non-compliances.
In another study in Finland [Citation10], the prerequisites for effective official food control were investigated. This revealed that the competent authorities invest in creating adequate working conditions through the provision of guidance papers, pro forma templates and possibilities for staff to collectively hold discussions. However, poor orientation, tacit knowledge and incomplete commitment among staff to quality systems are persistent challenges in food control units. Insufficient human resources and the inability of heads of food control units to recognise problems in the workplace setting may further impair the functional capacity of these units [Citation10]. One example of the importance of setting relevant objectives is the finding that the publicly accessible rankings (smileys) do not represent the microbiological content, and consequently the risks to consumers, of the products originating from sushi bars [Citation13].
In order to measure the effectiveness of food controls, the objectives of these controls should be set to focus on safe food and reliable detection of non-compliances with food safety regulations at food business establishments. Guidance for effectiveness criteria should be derived from the food safety legislation, which aims to (a) ensure safe food production, but also to (b) combat food fraud, (c) ensure fair competition between food business operators and (d) confirm that food information is reliable. Different parts of the regulations have differing importance for the production of safe food and fair information practices. Hence, supervision should validate the effectiveness of future official controls.
Acknowledgments
This study was funded by the Swedish National Food Agency, the Swedish University of Agricultural Sciences and the National Veterinary Institute.
Disclosure statement
No potential conflict of interest was reported by the authors.
Additional information
Funding
Notes on contributors
Christian Berking
Christian Berking graduated as a veterinarian from the Swedish University of Agricultural Sciences in Uppsala in 1996. He became a Swedish Specialist in Food Hygiene in 2005 and is currently working at the National Food Agency in Sweden. His main work topics are supervision and quality development of official food controls concerning animal welfare at slaughterhouses and foods of animal origin.
Ivar Vågsholm
Ivar Vågsholm graduated as a veterinarian in 1984 and obtained his PhD from the University of California in 1989. He has been Professor of Food Safety at Swedish University of Agricultural Sciences since 2009 and Head of the Department of Biomedicine and Veterinary Public Health since 2015. He has worked with European Union questions relating to animal health and food safety for the past 30 years. A key contribution has been as a member of the EFSA scientific panels on Animal Health and Welfare 2012-2015, Biological Hazards 2003-2012 and the EU Commission’s Scientific Committee of Veterinary Measures relating to Public Health 1997-2003. He has participated in two WHO consultations on campylobacter control and environmental health. Revisions of meat inspection procedures, food safety legislation, organising food control systems and control of TSE have been major research topics. Currently his main interests are the food safety of leafy greens, circular food production systems and novel foods. The challenge is to avoid cycles of nutrients becoming cycles of pathogens. Another interest is the organisation of control and inspection with regard to animal welfare, animal health and food safety.
Lisa-Marie Hedberg
Lisa-Marie Hedberg has a background as a food technologist. She is currently working as a Government Inspector at the National Food Authority in Sweden, with the focus on official inspections of foods of animal origin, supervisions, quality of food controls and training.
Sölvi Sörgjerd
Sölvi Sörgjerd graduated as a veterinarian in 1996 and is currently Deputy Head of the Department of Microbiology at the National Veterinary Institute.
Rauni Niskanen
Rauni Niskanen is currently Head of the Department of Microbiology at the National Veterinary Institute (SVA) in Uppsala, Sweden. SVA is an expert authority under the Ministry of Enterprise and Innovation, and is the nation’s leading knowledge centre for infectious diseases in veterinary medicine. Rauni Niskanen graduated in veterinary medicine from the Swedish University of Agricultural Sciences in Uppsala in 1982. She obtained a PhD degree from the same university in 1995, based on studies on diagnostic methods and epidemiological investigations of bovine diarrhoea virus infection in Swedish dairy herds. In the following years, she worked as Lecturer and as Senior Lecturer in Ruminant Medicine. In 2002, she was appointed Associate Professor in Ruminant Medicine. She had acted as Head of the Department of Ruminant Medicine and the Department of Clinical Science, in total for about six years. Between 2007 and 2015, she worked as Head of the Food Control Department at National Food Agency in Uppsala and held the post of Deputy Director General between 2012 and 2014.
References
- National Audit Systems Network (NAS). Auditing effectiveness of official control systems. 2014. p. 1. [cited 2016 May 11]. Available from: https://www.livsmedelsverket.se/globalassets/produktion-handel-kontroll/vagledningar-kontrollhandbocker/auditing-effectiveness-of-official-control-systems.pdf.
- EC No 882/2004. Regulation of the European Parliament and of the Council, on official controls performed to ensure the verification of compliance with feed and food law, animal health and animal welfare rules. Off J Eur Union. 2004 April 30;L165. [cited 2016 Mar 03] Available from: http://eur-lex.europa.eu/legal-content/EN/TXT/HTML/?uri=CELEX:02004R0882-20140630&rid=1
- Food and Veterinary Office. Overview report of a series of FVO fact-finding missions and audits carried out in 2012 and 2013 in order to evaluate the systems put in place to give effect to article 8 (3)of regulation (ec) no 882/2004 of The European Parliament and of the Council. FINAL Overview report (DG)SANCO 2014-7263. Ref. Ares(2014)4257848-17/12/2014. 2014 [cited 2016 Mar 03] Available from: http://ec.europa.eu/food/fvo/overview_reports/act_getPDF.cfm?PDF_ID=146
- Berking C, Johansson Ö, Karlsson T, et al. (2015) Instruktion för supervision av offentlig kontroll vid Område Livsmedelskontroll. Revised 20. 02.15.National Food Agency (NFA). 5 pp. [cited 2016 Jun 01]. (in Swedish).
- Berking C, Johansson Ö, Karlsson T, et al. (2016) Instruktionsbilaga för supervisionsprojekt avvikelsefrekvens, Bilaga 4. Revised 01. 01.16.National Food Agency (NFA). 5 pp. (in Swedish).
- EC No 853/2004. 29 April 2004. Regulation of the European Parliament and of the Council, laying down specific hygiene rules for food of animal origin. Off J Eur Union. L226. [cited 2016 May 09] Available from: http://eur-lex.europa.eu/legal-content/EN/TXT/PDF/?uri=CELEX:32004R0853R[01]&from=EN
- European Commission. Technical specifications in relation to the master list and the lists of EU approved food establishments and certain other specified food establishments. SANCO/2179/2005 Revision 2014. 2014. [cited 2016 Mar 03]. Available from: http://ec.europa.eu/food/food/biosafety/establishments/techspecs_en.pdf
- National Food Agency (NFA). Vägledning till kontrollmyndigheter m.fl. Riskklassning av livsmedels-anläggningar och beräkning av kontrolltid. 2013. 50 pp. [cited 2016 May 11] (in Swedish). Available from: http://www.livsmedelsverket.se/globalassets/produktion-handel-kontroll/vagledningar-kontrollhandbocker/vagledning-riskklassning-livsmedelsanlaggningar-berakning-kontrolltid.pdf
- National Food Agency (NFA). Kontrollhandbok - planera, leda och följa upp offentlig livsmedelskontroll. Fördjupning - Verifiering av den offentliga kontrollens effekt. 2015. 18 pp. [cited 2016 May 11] (in Swedish). Available from: http://www.livsmedelsverket.se/globalassets/produktion-handel-kontroll/vagledningar-kontrollhandbocker/fordjupning-verifiering-av-kontrollens-effekt—kontrollhandbok.pdf
- Läikkö-Roto T, Lundén J, Heikkilä J, et al. Prerequisites for effective official food control. Food Control. 2016;61:172–9.
- Lee J-E, Nelson DC, Almanza BA. Health inspection reports as predictors of specific training needs. Int J Hosp Manag. 2012;31(2): 522–528.
- Läikkö-Roto T, Mäkelä S, Lundén J, et al. Consistency in inspection processes of food control officials and efficacy of official controls in restaurants in Finland. Food Control. 2015;57:341–350.
- Leisner JJ, Lund TB, Frandsen EA, et al. What consumers expect from food control and what they get – A case study of the microbial quality of sushi bars in Denmark. Food Control. 2014;45:76–80.