Abstract
Effective management of plant health is fundamental for food and income security to meet the growing demands of local and global markets. This however requires farmers’ adequate access to quality planting materials under the prevailing contextual and psycho-social factors. This study, anchored in the Unified Theory of Acceptance and Use of Technologies, unravels technology-acceptance factors that influence farmers’ intentions to use banana tissue culture planting materials in the control of Banana Xanthomonas Wilt. Data were collected from 248 randomly sampled banana farmers using a structured questionnaire and analyzed using structural equation modelling to examine hypothesized paths in the uptake of banana tissue culture planting materials. Results show that farmer intentions to use tissue culture planting materials are dependent on two constructs: social influence and farmer innovativeness. However, social influence is the main predictor of intentions to use tissue culture planting materials. In particular, farmer innovativeness mediates facilitating conditions and social influence in predicting intentions to use tissue culture planting materials. Thus, this study reveals two factors that influence farmer intentions to use tissue culture planting materials: social influence and farmer innovativeness. The findings imply that social influence and farmer innovativeness are critical in disseminating novel agricultural technologies in Uganda and elsewhere.
Introduction
Good plant health is fundamental to ensure food and income security among smallholder farmers, so as to meet the demands of local and global markets (Danielsen et al. Citation2013). Likewise, effective plant healthcare is essential for producing healthy crops for both human and animal consumption (Karubanga, Matsiko, and Danielsen Citation2017), as well as for non-food purposes (Danielsen et al. Citation2012). However, substantial amounts of crop produce are lost every year due to plant health problems (Karubanga, Matsiko, and Danielsen Citation2017).
In sub-Saharan Africa, most of these losses are mainly due to pests and diseases associated with seed saved from previous harvests, that smallholder farmers rely on for planting material. In rural farming communities, the informal seed systemFootnote1 is usually preferred to the formal seed system.Footnote2 Even when seed is available from the market, farmers often prefer using their own seed to save money and avert risks associated with the crops they grow (e.g. changes in weather, pests and erratic market prices). For example, in informal systems mostly in developing countries, the seed from vegetatively propagated crops such as banana is mainly produced locally. More than 90% of farmers in the banana farming systems in East and Central Africa rely on suckers sourced from friends, neighbours, relatives and/or their own fields to establish new banana gardens (Smith et al. Citation2008; Lwandasa et al. Citation2014). However, the banana planting material they use is seldom certifiably disease free, which poses a high risk of transmitting pests and diseases across farms (Jogo et al. Citation2013). Seed-borne pests and diseasesFootnote3 continue to be a serious threat to banana production, more so in areas where the planting material is of poor quality and/or scarce.
Banana Xanthomonas Wilt (BXW) caused by the bacterium Xanthomonas campestrisn pv. musacearum is a major threat to food and income security among smallholder farmers in Uganda, especially in the areas where banana is an important staple and cash crop. If uncontrolled, BXW can eventually cause an estimated production loss of the banana crop of about 53% over a 10-year period (Tripathi et al. Citation2009), equivalent to a reduction from 10.5 to 5.6 million metric tonnes per year (FAOSTAT Citation2013). The disease can potentially decimate the entire crop where highly susceptible cultivars are grown (Kubiriba et al. Citation2012). Between 2002–2005, it is estimated that the disease caused a total loss equivalent to 61.1 million dollars to the country, mainly associated with the East African Highland Banana (EAHB) ‘Matooke’ (AAA-EAHB genome group) and the ‘Kayinja’ beer banana (ABB genome group) (Tushemereirwe et al. Citation2009). To avert the situation, use of tissue culture (TC) banana (Musa spp.) planting materials is one of the strategies that has been promoted for controlling the BXW disease. Using TC banana planting material is an effective method of providing pest- and disease-free plants (Dubois et al. Citation2013), guaranteed by high standards in the production process. It is therefore argued that as a control measure in the spread of BXW, TC banana planting materialsFootnote4 can be of high quality (Wambugu et al. Citation2000) and consequently of utmost relevance for boosting banana productivity since they aid in the establishment of clean banana plantations – void of BXW.
Campaigns against BXW have since focused on encouraging farmers to adopt TC seedlings as a technology. However, the uptake of TC seedlings remains low (Jogo et al. Citation2013), at less than 7% of the total banana coverage in the country (Dubois et al. Citation2013). Previous research assessing adoption of TC banana planting materials has mainly focused on economic factors such as high cost of seedlings, and higher labour and input requirements (Muyanga Citation2009; Njau et al. Citation2011; Kabunga, Dubois, and Qaim Citation2012). Little attention has been paid to studying how farmers’ beliefs and attitudes towards TC banana planting materials affects their intentions to use them to control BXW. Specifically, two objectives are addressed in this study: first, to identify and describe the contextual and psycho-social technology-acceptance factors that influence the use of TC banana planting materials in Uganda; and second, to explore their effects on the uptake of TC banana planting material. To our knowledge, there are no studies that have explicitly analyzed and explained how farmers’ beliefs and attitudes predict acceptance and use of TC banana planting materials in combatting BXW. Our contribution to the literature is twofold: First, the study applies the Unified Theory of Acceptance and Use of Technology (UTAUT) model to an agricultural technology-adoption context in a developing country. Second, we extend the UTAUT model by including two additional variables that influence uptake of new technologies: perceived quality and farmer innovativeness.
Theoretical framework
The technology-acceptance factors can negatively affect farmers’ decisions to use TC banana planting materials. In this study, we use the Unified Theory of Acceptance and Use of Technology (UTAUT) to examine the effect of technology-acceptance factors on TC banana planting materials uptake in Central Uganda. We expect that understanding these factors could help increase uptake of TC banana planting material. Recent applications of UTAUT for assessing uptake of a new technology such as TC banana include: Wu, Tao, and Yang (Citation2007); Maldonado et al. (Citation2009); Islam and Grönlund (Citation2011); and Taiwo and Downe (Citation2013). Specifically, UTAUT has been applied to examine rural farmers’ adoption of agricultural information technology services (Wu Citation2012); farmers’ uptake of solar water pump technology in Northern Pakistan (Zhou and Abdullah Citation2017), and farmers’ acceptance of pressurized irrigation technology (Nejadrezaei et al. Citation2018).
UTAUT is one of the new models in the domain of technology acceptance and compared to other widely-used theories such as the Technology Acceptance Model (TAM), Innovation Diffusion Theory (IDT) and Theory of Planned Behavior (TPB), it utilizes a more integrative approach, combining variables from existing theories as predictors of technology acceptance and usage (Nejadrezaei et al. Citation2018). The Unified Theory of Acceptance and Use of Technology integrates constructs across eight models that include: the Technology Acceptance Model (TAM) (Davis Citation1989), the Theory of Reasoned Action (TRA) (Fishbein and Ajzen Citation1975), the theory of Planned Behavior (TPB) (Ajzen Citation1991), the Combined TAM and TPB (Taylor and Todd Citation1995), the Innovation Diffusion Theory (IDT) (Rogers Citation2003), the Social Cognitive Theory (SCT) (Bandura Citation1989), the Motivational Model (MM) (Davis, Bagozzi, and Warshaw Citation1992), and the Model of PC Utilization (MPCU) (Thompson, Higgins, and Howell Citation1991). Consequently, it has a better predictive value than any one individual theory (San Martín and Herrero Citation2012; Okumus et al. Citation2018).
In explaining intentions to use technologies, UTAUT uses the concepts of social influence, facilitating conditions, effort expectancy and performance expectancy. The promotion of TC planting materials amongst smallholder farmers for adoption mirrors this structural set of the UTAUT model in the sense that the focus is on farmers’ acceptance of TC as a technology. UTAUT explains that people accept new ideas, such as using banana TC planting materials, following a series of complex mental processes in which intentions precede behaviour (Venkatesh et al. Citation2003; Islam and Grönlund Citation2011). However, the concept of effort expectancy, which recounts the extent to which a farmer believes that using TC planting material is free of effort, was dropped in the analytical framework because it is a notion farmers in the current study communities are ardently aware of, having interfaced with this technology for over a decade now. Subsequently, we integrate farmer innovativeness and perceived quality of the technology as additional determinants of farmer acceptance and use of the TC banana technology. Previous studies have shown higher innovativeness in individuals to be associated with positive beliefs and perceptions towards technology uptake (Ali, Nair, and Hussain Citation2016; Okumus et al. Citation2018). Similarly, farmer perceptions of the quality of proposed planting materials have also been shown to be of significance for the acceptance and eventual use of proposed technologies (Andrade-Piedra et al. Citation2016). Thus, farmer innovativeness and perceived quality of the planting materials may be essential determinants of farmers’ acceptance of the TC technology.
Previous research (Venkatesh, Thong, and Xu Citation2012; Ali, Nair, and Hussain Citation2016; Okumus et al. Citation2018) showed that intention to use a technology is the most significant predictor for uptake behaviour and actual usage. Ajzen (Citation1991) asserted that individuals’ intentions capture the motivational aspects that affect their behaviour and indicate any individual's willingness to develop an action. A common construct that explains intentions is social influence. For this study, social influence is defined as the degree to which a farmer perceives that relevant people believe that he or she should use a technology (Taylor and Todd Citation1995). El-Gayar, Moran, and Hawkes (Citation2011) argue that social influence triggers individuals’ behavioural intentions to use new technologies and various scholars (Abushanab and Pearson Citation2007; Eckhardt, Laumer, and Weitzel Citation2009; Ali, Nair, and Hussain Citation2016; Okumus et al. Citation2018) attest to this. Since banana cultivation is embedded in the cultural values of exchange of planting materials and traditional practices (Mulugo Citation2019), the pressures created by social interactions and norms may motivate farmers in the uptake of TC as banana planting materials in the control of BXW. In the context of this study, social influence is operationalized as being persuaded (informed) by faith based organizations, members of the community and farmer groups to use TC planting materials in growing bananas. It can thus be assumed that social influence on the use of TC banana planting materials will influence farmer uptake intentions.
Facilitating conditions as a construct describes the degree to which a farmer believes that organizational and technical infrastructure exist to support his or her use of TC as banana planting material. A number of studies attest and confirm the effect of facilitating conditions on acceptance and usage of technologies (Venkatesh, Thong, and Xu Citation2012; Ali, Nair, and Hussain Citation2016; Okumus et al. Citation2018). Increasing levels of facilitating conditions are expected to reduce levels of uncertainty or ambiguity with the TC banana technology (Al-Gahtani, Hubona, and Wang Citation2007). For instance, Alawadhi and Morris (Citation2008) tested and confirmed the significant effect of facilitating conditions on students’ use of e-government services. In another study, Lakhal, Khechine, and Pascot (Citation2013) verified facilitating conditions as a significant determinant of students’ intentions to use desktop video conferencing in a distance learning course. In the context of this study, ‘facilitating conditions’ are operationalized as TC nurseries having demonstration gardens and the TC nursery operators being willing to provide information to intending users on how to plant and manage the TC planting materials. It can therefore be assumed that facilitating conditions will influence uptake intentions for TC banana planting materials. Perceived seed quality on the other hand is described as the degree to which a farmer believes that TC planting materials are free of pests and diseases (particularly BXW) and are of high genetic purity (RTB Citation2016). Studies (Abay, Waters-Bayer, and Bjørnstad Citation2008; Kraft, de Jesús Luna-Ruíz, and Gepts Citation2010; Andrade-Piedra et al. Citation2016) attest and confirm the importance of seed quality in farmer acceptance and use of technologies. High perception levels of the quality of TC planting materials are expected to motivate farmers in their use of TC banana planting materials since their uncertainty/risk as a potential means of spreading BXW in farmers’ banana crop is reduced. In the perspective of this research, seed quality is operationalized as TC planting materials being perceived as pest and BXW free and void of mutants. It is, as such, assumed that farmers’ perceived quality of TC banana planting materials will influence their intentions to use this technology.
Performance expectancy is contextualized in this study as the extent to which a banana farmer believes that the use of TC as banana planting material enhances the performance and output from his or her banana plantation(s) (Compeau and Higgins Citation1995). Previous studies have shown that intention to use a technology or system is significantly predicted by performance expectancy (Slade et al. Citation2015; Ali, Nair, and Hussain Citation2016; Okumus et al. Citation2018). Biemans et al. (Citation2005) revealed that performance expectancy is a high predictor of a nurse's behavioural intention to use medical teleconferencing applications. Similarly, Fang, Li, and Liu (Citation2008) showed that performance expectancy significantly predicts managers’ intentions to engage in knowledge sharing using web2.0. We therefore presume that performance expectancy has a direct effect on TC technology uptake intentions. In light of this study, performance expectancy was operationalized as TC planting materials yielding marketable large sized banana bunches and many suckers. Thus, we further postulate that farmers’ perceived performance expectancy of TC banana planting materials will positively influence uptake intentions of the TC banana technology.
Farmer innovativeness in the perspective of this study is the degree to which a farmer is receptive to new agricultural related ideas and makes innovative decisions independently of the communicated experience of others (Midgley and Dowling Citation1978). Previous studies have discussed the influence of innovativeness on individuals’ perceptions and behaviours and its role in determining users’ acceptance of technologies (Lu et al. Citation2003; Lu, Yao, and Yu Citation2005; Yi and Probst Citation2006; Turan, Tunç, and Zehir Citation2015). For instance, Okumus et al. (Citation2018) showed individual innovativeness to be a significant predictor of restaurant customers’ intentions to use smartphone diet apps while ordering food. Additionally, Liu, Li, and Carlsson (Citation2010) revealed that innovativeness was a significant predictor of Chinese students’ intentions to use mobile learning. In the field of self-service technologies, Chen (Citation2008) also found that an individual’s innovativeness directly influenced intentions to purchase and use these technologies. For this study, farmer innovativeness was operationalized as farmer willingness to creatively integrate the use of TC planting materials with their local knowledge and other available materials in the control and management of BXW. We expect farmers’ innovativeness to influence TC planting materials uptake intentions. Moreover, highly innovative farmers may have better perceptions of TC planting materials in terms of facilitating conditions, influencing their uptake intentions compared to less innovative farmers (Okumus et al. Citation2018). Likewise, San Martín and Herrero (Citation2012) argued that individuals with higher innovativeness are less influenced by others’ opinions about technological innovations. Thus, higher innovativeness may result in weaker social influence on adoption and uptake of the TC technology. Consequently, for highly innovative users, facilitating conditions and social influence may be less influential when adopting a technology.
Methodology
In this section, we first describe the research design, with some a priori expectations. Next, we describe the selection of participants and collection of data, including how items were measured. Finally, we discuss the different components of the data collected for SEM analysis and present some descriptive statistics.
Research design
This study employs a structural equation modelling (SEM) approach to develop a research model that represents the relationships among six variables as adapted from UTAUT (Venkatesh et al. Citation2003). The six variables include: intention to use TC planting materials, social influence, facilitating conditions, perceived quality of TC technology, performance expectancy, and farmer innovativeness, as shown in .
Figure 1: Research model illustrating the hypothetical influence of technology acceptance factors on intentions to use TC banana planting materials.
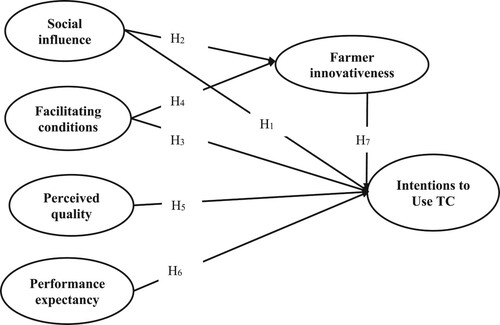
Based on the research model above, nine hypotheses were formulated:
H1: Social influence has a positive and significant influence on farmer intentions to use banana TC planting materials.
H2: Social influence has a positive and significant influence on farmer innovativeness in the use of banana TC planting materials.
H3: Facilitating conditions have a positive and significant influence on farmer intentions to use banana TC planting materials.
H4: Facilitating conditions have a positive and significant influence on farmer innovativeness to use banana TC planting materials.
H5: Farmers’ perceived quality of banana TC planting materials has a positive and significant influence on their intentions to use banana TC planting materials.
H6: Performance expectancy has a positive and significant influence on farmer intentions to use banana TC planting materials.
H7: Farmer innovativeness has a positive and significant influence on farmer intentions to use banana TC planting materials.
H8: Social influence is mediated by farmer innovativeness in predicting farmer intentions to use banana TC planting materials.
H9: Facilitating conditions is mediated by farmer innovativeness in predicting farmer intentions to use banana TC planting materials.
Research participants and data collection methods
A total of 248 banana farmers were randomly sampled from two farming communities () that hosted community-based banana TC nurseries in Luweero and Mukono districts in Central Uganda using a village listing. The selected sample represented about 24% of the 1060 banana farmers in the study communities (). Data were collected using a structured questionnaire through face-to-face interviews. Data elicited included demographic information and farmers’ responses to multiple items measuring each construct reflected in the research model of . Notably, informed consent from the participating farmers was obtained prior to the individual interviews.
Table 1: Number of farmers selected for the study.
Measures
To measure the constructs in this study, 21 statements were used. Social influence (SI) was measured with 3 items (e.g. ‘If I am informed about TC seed by a faith-based leader in my church/mosque, then I can use it as banana seed’). Facilitating conditions (FC) was measured with 3 items (e.g. ‘The TC nursery operator is welcoming and willingly provides information on how to plant TC seed’), and perceived quality (PQ) had 3 items (e.g. ‘TC seed is BXW free’) as proposed by RTB (Citation2016). The performance expectancy (PE) variable had 4 items (e.g. ‘TC seed yields bunches with large banana fingers’) as well as farmer innovativeness (FI) variable (e.g. ‘I apply wood ash around TC banana mats that have exhibited BXW symptoms to prevent further infection’). Finally, the intention for uptake of TC technology (IU) had 4 items (e.g. Given the chance, I intend to use TC seed when expanding my banana plantation) as adapted from Okumus et al. (Citation2018). Each item was measured on a five-point Likert scale with 1 = strongly disagree, 2 = disagree, 3 = neutral, 4 = agree and 5 = strongly agree.
Assessment of assumptions for SEM analysis
Preceding path analysis, four assumptions of SEM were assessed, namely: Normality, measurement validity, convergent validity, discriminant validity and multicolinearity. First, the normality of the data was examined. Skewness and kurtosis stretched from −1.89–1.25 and −0.57–5.04 respectively (). Following Kline’s (Citation2005) recommendations that the skewness and kurtosis indices should be within 3 and 10 respectively, the data in this study are regarded as normal.
Table 2: Descriptive statistics of the study constructs.
Secondly, measurement validity was assessed using construct reliability (CR), instead of Cronbach's alpha because the latter understates reliability (Hair et al. Citation2006). The precondition is that CR = 0.70 and above to satisfy measurement validity (Nunnally and Bernstein Citation1994). Convergent validity, concerned with whether observable variables (items) share adequate variance in the construct/latent variable was assessed using two indicators; namely factor loadings and AVE. This study adopted the criteria of Hair et al. (Citation1998) on assessing factor loadings. They specify that a minimum threshold value of 0.4 for a sample size of 200 is adequate for confirming convergent validity. The AVE minimum threshold value adopted for this study in assessing convergent validity was 0.5 as suggested by Segars (Citation1977). Discriminant validity was assessed by comparing the values of correlates to square root of AVE values. The preconditions are that the correlates must be smaller than the square root of AVE to satisfy the condition of discriminant validity (Fornell and Larcker Citation1981). Correlation analysis was conducted following Hamilton (Citation2006) to evaluate whether there was no more than one correlate exceeding 0.600 and so ruling out the risk of multicollinearity.
Measurement validity, based on composite reliability (CR) values for all constructs, ranged from 0.70 to 0.99 () which were all above the minimum threshold of 0.70. Accordingly, measurement validity was confirmed in this study. Similarly, convergent validity was confirmed, since all factor loading had values above 0.4 (Hair et al. Citation1998) and AVE values were also all above 0.5. Regarding the discriminant validity, the values of correlates were less than the square root of values of AVE (). Since inter-construct variance was less than intra-construct variance, it meant that all constructs exhibited distinctness from each other. Accordingly, discriminant validity was confirmed. In testing for multicollinearity, the values of correlates ranged between 0. 034 and 0.538 and were all below the minimum threshold value of 0.60 as suggested by Hamilton (Citation2006). This ruled out the risk of multicollinearity.
Table 3: Validity and reliability for constructs.
Table 4: Testing for multicollinearity and discriminant validity.
Structural model assessment
Using Analysis of Moment Structure (AMOS) version 21 programme, the research model for this study was tested. As recommended by Hair et al. (Citation2006), a variety of indices were used to test for model fit, namely the χ2 statistic, the adjusted goodness of fit index (AGFI), the root mean square error of approximation (RMSEA), comparative fit index (CFI) and Tucker-Lewis index (TLI) ().
Table 5: Fit indices for the measurement model.
Further analysis was done using bootstrapping to assess mediation relationships as well as determining effect sizes of prediction relationships. A model-based bootstrapping simulation of up to two thousand repetitions was performed as suggested by Byrne (Citation2010). Notably, Byrne (Citation2010) explained that this procedure is important for ascertaining that the overall fit is not inflated because of the small sample size relative to the degrees of freedom of the model. On effect sizes, Cohen (Citation1988) reasoned that effects with values less than 0.1 are considered small, those with less than 0.3 medium and values with 0.5 or more are considered large. In this study, the Cohen (Citation1988) criterion of evaluating effect sizes was adopted to assess the extent of influence the independent variables had on the dependent variable.
Results
Sample characteristics
Results in show that the sample of respondents comprised more females (55%) than males (45%). Most were married (67%) and were between the ages of 30 and 49 years (55%). Forty-six percent of the respondents earn between UGX 1,000,000–4,999,900 annually, with most of them (77%) having attained primary and secondary school education. The Baganda ethnic group is the most dominant (92%) in the study communities and other minor groups comprise the Basoga (2%) Bakiga (1%) and others. ()
Table 6: Demographic characteristics of study participants.
Structural equation model results
shows the results of the structural equation model predicting the intention to use banana TC planting materials in the control of BXW. The overall goodness-of-fit of the illustrated model, as measured by the fit indices, indicated a good fit to the data. Furthermore, all independent and mediating variables explain 28% of the variance in intentions to use the TC banana technology (). The R2 value of 0.28 is higher than the 0.26 value that Cohen (Citation1988) suggested would indicate a substantial model.
Results presented in show that social influence (β = 0.432; t = 7.651; p < 0.01) had a positive and significant influence on farmers’ intentions to use TC planting materials. This finding supports hypothesis H1 implying that social influence is a significant predictor of farmer intentions to use banana TC planting materials. Furthermore, social influence (β = 0.240; t = 2.720; p < 0.01) and facilitating conditions (β = 0.340; t = 5.062; p < 0.01) indicated a positive and significant influence on farmer innovativeness, confirming hypotheses H2 and H4. Similarly, farmer innovativeness had a positive and significant influence on intentions to use TC planting materials (β = 0.095; t = 2.396; p < 0.05), thus supporting hypothesis H7 that farmer innovativeness significantly predicts farmer intentions to use banana TC planting materials.
Table 7: Path analysis results for testing hypotheses.
However, none of facilitating conditions (β = −0.044; t = −1.043; p > 0.05), perceived quality of the TC planting materials (β = 0.031; t = 0.574; p > 0.05) and performance expectancy (β = 0.175; t = 1.913; p > 0.05) were significant predictors of farmer intentions to use TC planting materials. Hence, hypotheses H3, H5 and H6 were not supported in this study, implying that facilitating conditions, perceived quality and performance expectancy are not perceived as important constructs in predicting farmer intentions to use TC banana planting materials.
Extent of influence of technology-acceptance factors
Bootstrapping results () show that the indirect standardized effects for farmers’ innovativeness (β = 0.025; 95% CI = 0.003 ∼ 0.054) in mediating social influence to predict intention for uptake of TC planting materials was significant. Thus, the hypothesis (H8) that farmer innovativeness mediates social influence in predicting intentions for uptake of TC was confirmed. Similarly, the indirect standardized effects for farmers’ innovativeness (β = 0.046; 95% CI = 0.008 ∼ 0.098) in mediating facilitating conditions to predict intention to use TC planting materials was significant. Likewise, hypothesis (H9), that farmer innovativeness mediates facilitating conditions in predicting intentions to use TC, was confirmed.
Table 8: Boot strapping for mediation and effect sizes.
Results further show that social influence is the most dominant predictor of intentions to use TC planting materials with the largest total effect of 0.492. Additionally, this finding is statistically significant (β = 0.492; 95% CI = 0.308 ∼ 0.647) and close to the large effect size criterion of Cohen (Citation1988). The finding implies that social influence predicted about 49.2% of the variance in farmer willingness to use banana TC planting materials. Furthermore, facilitating conditions (β = −0.016; 95% CI = −0.159 ∼ 0.133), perceived quality of the TC planting materials (β = 0.040; 95% CI = −0.126 ∼ 0.203), performance expectancy (β = 0.141; 95% CI = −0.068 ∼ 0.332) and farmer innovativeness (β = 0.148; 95% CI = 0.029 ∼ 0.279) respectively predict 1.6%, 4%, 14.1% and 14.8% of the variation in the farmers’ intentions to use TC planting materials. Much as all these findings were significant, they fell short of meeting the medium size criterion of effect sizes as suggested by Cohen (Citation1988).
The effect size in the relationship between facilitating conditions and farmer innovativeness (β = 0.310; 95% CI = 0.192 ∼ 0.423) was significant and fell within the criterion of medium size. This finding implies that facilitating conditions predicted about 31% of the variation in farmer innovativeness to use the TC planting materials in controlling BXW. On the other hand, the effect size in the relationship between social influence and farmer innovativeness (β = 0.167; 95% CI = 0.040 ∼ 0.289) was significant but it was only meeting small size criterion. This finding implies that facilitating conditions predicted about 16.7% of the variation in farmer innovativeness to use the TC planting materials in controlling BXW.
Discussion
Considering the importance of agricultural technology in the livelihoods of farmers, it is necessary to have a deeper understanding of the factors that are likely to influence easy uptake of new technologies such as a banana tissue culture. This study aimed to identify and examine technology-acceptance factors that reportedly influence the uptake of banana TC planting materials, this technology being part of the strategy of the Government of Uganda to control the prevalence of BXW. Based on UTAUT, the study aimed at investigating hypotheses on factors that were presumed to influence farmers’ adoption and use of banana TC planting materials including; social influence, facilitating conditions and performance. Owing to the relevance of individuals’ innovativeness towards acceptance of new agricultural technologies and farmers’ perceptions of the quality of proposed planting materials, this study also examined the role of farmer innovativeness and farmers’ perceived quality of the planting materials and how these influence farmer intentions to use the TC technology. The proposed model consists of explanatory variables from UTAUT, and is extended by two additional variables, viz; perceived quality and farmer innovativeness. Results showed that social influence and farmer innovativeness are significant predictors of uptake of banana TC technology and that social influence was the most important among all predictors for intentions to use the TC technology. Results point to the fact that farmers will have positive intentions to use TC as planting material if there is influence from a farmer's social circle or if a farmer is innovative and creatively integrates the use of TC planting materials with local knowledge and other available materials in the control and management of BXW. This is consistent with several previous studies (Eckhardt, Laumer, and Weitzel Citation2009; Amoroso and Lim Citation2015; Okumus et al. Citation2018). The study findings further reveal that social influence and facilitating conditions influence farmer innovativeness. This indicates that a farmer's social circle and enabling conditions in the use of TC are essential in stimulating farmer innovativeness, an important predictor in TC planting materials uptake. This infers that social interactions and facilitating conditions are crucial in creating an enabling environment for farmers to be innovative.
Another important finding is the non-significant influence of facilitating conditions in predicting farmers’ intentions for use of banana TC technology. This finding may be due to the fact that TC planting materials have been promoted in the study area for over a decade, and even campaigns against BXW have mainly focused on encouraging farmers to use TC seedlings. In addition, TC nurseries have been established in the study communities and, as such, farmers are familiar with TC planting materials and facilitating conditions, which explains why TC may not be of relevance to them in deciding whether or not to use them. Interestingly, this finding contrasts with previous literature supporting the significant effect of facilitating conditions on an individual’s acceptance and uptake of technologies (Venkatesh et al. Citation2003; Alawadhi and Morris Citation2008; Eckhardt, Laumer, and Weitzel Citation2009; Lakhal, Khechine, and Pascot Citation2013; Ali, Nair, and Hussain Citation2016). For example, Alawadhi and Morris (Citation2008) tested and confirmed the significant effect of facilitating conditions on students’ use of e-government services and Lakhal, Khechine, and Pascot (Citation2013) verified facilitating conditions as a significant determinant of students’ intentions to use desktop video conferencing in a distance learning course.
Likewise, performance expectancy is not a significant predictor of farmer adoption of TC. This is perhaps explained by the fact that farmers have for the past decades interfaced with TC technology and have observed its performance on their respective farmsteads. Thus, they are keenly aware of the benefits that they can derive from the technology and possibly rather than buy TC banana seed, they use suckers from their TC planted mats as planting material to expand or fill gaps in their banana plantations. Our findings however contrast with previous literature supporting the significant effect of performance expectancy on intended users’ acceptance and use of technologies (Biemans et al. Citation2005; Fang, Li, and Liu Citation2008; Eckhardt, Laumer, and Weitzel Citation2009; Ali, Nair, and Hussain Citation2016; Okumus et al. Citation2018). For example, Biemans et al. (Citation2005) examined nurses’ behavioural intentions to use medical teleconferencing applications and their findings reveal that performance expectancy is a high predictor of behavioural intention. Similarly, empirical evidence from a study by Fang, Li, and Liu (Citation2008) shows that performance expectancy significantly predicts managers’ intentions to engage in knowledge sharing using web2.0.
Conversely, the perceived quality of TC planting materials was also found to be a non-significant predictor of farmer use of TC. This is probably because for the past decade, farmers in the study communities have been intensively sensitized to and trained in the importance of TC as clean planting materials in attempts to control BXW. The finding implies that the farmers are undoubtedly aware of the quality of the TC planting materials, efforts that can be attributed to the role played by agricultural extension programmes through the Government of Uganda (Barungi, Guloba, and Adong Citation2015). Lastly, our findings further reveal that the mediating role of farmer innovativeness is significant in enhancing the potential of social influence and facilitating conditions in predicting the intentions of farmers to use the TC planting materials. This means however that farmers’ innovativeness is a critical construct in intervening social influence and facilitating conditions if proper prediction for use of TC planting materials is to be made by the farmers (see also Turan, Tunç, and Zehir Citation2015).
Conclusion
This study applied the UTAUT model to investigate factors influencing the use of TC banana planting materials as a strategy to control BXW. In attempts to achieve this overall objective, this study further used the constructs of farmer innovativeness and perceived quality of planting materials to extend the theoretical model of UTAUT. Our results have revealed that farmer innovativeness is an important and critical factor in influencing the adoption of any agricultural technologies; in this case the TC banana planting materials. Farmer innovativeness also serves as a cardinal influence in mediating other technological-acceptance factors, such as social influence, and facilitating conditions for more meaningful and accurate prediction of farmers’ intentions to use the available quality TC planting materials with the purpose of curbing BXW to enhance food and income security of smallholder farmers. This study reveals critical factors and thus expands the UTAUT framework in the context of farmers’ use of TC planting materials by proposing two additional constructs that are ideal in predicting the technological acceptance behaviour among the farming communities – i.e. farmer innovativeness and perceived quality of TC planting materials. It can be concluded that these two are direct determinants of farmers’ intentions to use agricultural technologies, such as banana TC planting materials. This study provides strong empirical insights to propose and test a model for assessing technology-acceptance related factors that influence farmers’ intentions to use promoted and approved agricultural technologies for enhancing rural livelihoods. This implies that the results provide a strong foundation for application in different contexts and theoretical assessments in other research fields.
Furthermore, this study offers practical implications in relation to banana farmers and supporting institutions (e.g. TC Laboratories and research institutions) that help in breeding and propagating banana TC planting materials. Concerning rural-based farmers, the research findings also suggest that social influence needs to be taken as the most significant predictor in assessing their intentions to use the new TC planting materials. This is a key implication for research and other technology promoting institutions and extension efforts for the success of future related technologies meant for the marginalized rural farming communities. Results recommend that new interventions, such as the banana TC planting materials, need to be promoted through locally institutionalized mechanisms, like faith-based leaders, political and community leaders and their respective members. Thus, fostering individual and group-based forms of interactive learning (e.g. through farmer groups, experimental gardens and extension) can act as systems for more enhanced encouragement purposely to trigger the acceptance and use of agricultural technologies, such as the TC banana planting materials.
However, it should be noted that this research was basically designed to investigate perceptions of farmers towards TC planting materials with limited attention paid to the actual measurement of farmer use of the TC planting materials in study area. Thus, this calls for future research efforts to focus on investigating the actual uptake of the TC banana technology in Uganda and elsewhere in the world. Additionally, prospective studies may also propose comparative models for TC technology users and non-users to establish the extent to which the intentions to use the technology differ among the two independent groups. The study sample was limited to Central Uganda and future studies should collect empirical data from other banana growing regions to ascertain whether use of technologies like TC banana planting materials are influenced by different ecological zones and/or regions.
Disclosure statement
No potential conflict of interest was reported by the authors.
Additional information
Funding
Notes
1 An informal seed system is managed by the farmers without the public sector (Thiele Citation1999).
2 A formal seed system is regulated by the public sector, usually by an inspection process known as ‘certification’ and controls variety release to ensure that available seed is of a recognized variety with a low incidence of disease (RTB Citation2016).
3 Nematodes, weevils, bacterial and fungal wilts (incl. BXW) and viral diseases.
4 TC banana planting materials and TC seedlings are used interchangeably throughout the manuscript.
References
- Abay, F., A. Waters-Bayer, and Å Bjørnstad. 2008. “Farmers’ Seed Management and Innovation in Varietal Selection: Implications for Barley Breeding in Tigray, Northern Ethiopia.” AMBIO: A Journal of the Human Environment 37: 312–320.
- Abushanab, E., and J. M. Pearson. 2007. “Internet Banking in Jordan: the Unified Theory of Acceptance and Use of Technology (UTAUT) Perspective.” Journal of Systems and Information Technology 9 (1): 78–97.
- Ajzen, I. 1991. “The Theory of Planned Behaviour.” Organizational Behavior and Human Decision Processes 50: 179–211.
- Al-Gahtani, S. S., G. S. Hubona, and J. Wang. 2007. “Information Technology (IT) in Saudi Arabia: Culture and the Acceptance and Use of IT.” Information & Management 44 (8): 681–691.
- Alawadhi, A., and A. Morris. 2008. “The Use of the UTAUT Model in the Adoption of E-Government Services in Kuwait.” Proceedings of the 41st Hawaii International Conference on System Sciences, Hawaii.
- Ali, F., P. K. Nair, and K. Hussain. 2016. “An Assessment of Students’ Acceptance and Usage of Computer Supported Collaborative Classrooms in Hospitality and Tourism Schools.” Journal of Hospitality, Leisure, Sport & Tourism Education 18: 51–60.
- Amoroso, D., and R. Lim. 2015. “Exploring the Personal Innovativeness Construct: The Roles of Ease of Use, Satisfaction and Attitudes.” Asian Pacific Journal for Information Systems 25 (4): 662–685.
- Andrade-Piedra, J. L., J. Bentley, C. Almekinders, K. Jacobsen, S. Walsh, and G. Thiele. 2016. Case Studies of Roots, Tubers and Bananas seed systems. Lima: RTB Working Papers.
- Bandura, A. 1989. “Human Agency in Social Cognitive Theory.” American Psychologist 44: 1175–1184.
- Barungi, M., M. Guloba, and A. Adong. 2015. Uganda’s Agricultural Extension Systems: How Appropriate is the Single Spine Structure? (Vol. 16). EPRC Research Report.
- Biemans, M., J. Swaak, M. Hettinga, and J. G. Schuurman. 2005. “Involvement Matters: The Proper Involvement of Users and Behavioural Theories in the Design of a Medical Teleconferencing Application.” Proceeds of GROUP’05, Sanibel Island, Florida, USA.
- Byrne, B. M. 2010. Structural Equation Modeling with AMOS. Basic Concepts, Applications and Programming. New York, US: Routledge – Taylor & Francis Group.
- Chau, P. Y. K. 1997. “Reexamining a Model for Evaluating Information Center Success Using a Structural Equation Modeling Approach.” Decision Sciences 28 (2): 309–334.
- Chen, L. 2008. “A Model of Consumer Acceptance of Mobile Payment.” International Journal of Mobile Communications 6 (1): 32–52.
- Chen, L.-S., O. M. Kwok, and P. Goodson. 2008. “U.S. Health Educators’ Likelihood of Adopting Genomic Competencies Into Health Promotion.” American Journal of Public Health 98 (9): 1651–1657.
- Cheng, E. W. L. 2007. “SEM Being More Effective than Multiple Regression in Parsimonious Model Testing for Management Development Research.” Journal of Management Development 20 (7): 650–667.
- Cleveland, M., M. Laroche, and N. Papadopoulos. 2009. “Cosmopolitanism, Consumer Ethnocentrism, and Materialism: An Eight-Country Study of Antecedents and Outcomes.” Journal of International Marketing 17 (1): 116–146.
- Cohen, J. 1988. Statistical Power Analysis for the Behavioral Sciences. 2nd ed. New Jersey city: Lawrence Erlbaum.
- Compeau, D. R., and C. A. Higgins. 1995. “Computer Self-Efficacy: Development of a Measure and Initial Test.” MIS Quarterly 19 (2): 189–211.
- Danielsen, S., J. Centeno, J. López, L. Lezama, G. Varela, P. Castillo, C. Narváez, I. Zeledón, F. Pavón, and E. Boa. 2013. “Innovations in Plant Health Services in Nicaragua: From Grassroots Experiment to a Systems Approach.” Journal of International Development 25 (7): 968–986.
- Danielsen, S., F. B. Matsiko, E. Mutebi, and G. Karubanga. 2012. “Second Generation Plant Health Clinics in Uganda: Measuring Clinic Performance from a Plant Health System Perspective 2010-2011.” Working paper 2. Centre for Health Research and Development, Faculty of Health and Medical Sciences, University of Copenhagen, Denmark.
- Davis, F. D. 1989. “Perceived Usefulness, Perceived Ease of Use, and User Acceptance of Information Technology.” MIS Quarterly 13: 319–340.
- Davis, F. D., R. P. Bagozzi, and P. R. Warshaw. 1992. “Extrinsic and Intrinsic Motivation to Use Computers in the Workplace.” Journal of Applied Social Psychology 22 (14): 1111–1132.
- Dubois, T., Y. Dusabe, M. Lule, P. van Asten, D. Coyne, J. C. Hobayo, S. Nkurunziza, et al. 2013. “Tissue Culture Banana (Musa spp.) for Smallholder Farmers: Lessons Learnt from East Africa.” Acta Horticulturae 986: 51–59.
- Eckhardt, A., S. Laumer, and T. Weitzel. 2009. “Who Influences Whom? Analyzing Workplace Referents’ Social Influence on IT Adoption and Non-Adoption.” Journal of Information Technology 24 (1): 11–24.
- El-Gayar, O. F., M. Moran, and M. Hawkes. 2011. “Students’ Acceptance of Tablet PCs and Implications for Educational Institutions.” Journal of Educational Technology & Society 14 (2): 58–70.
- Fang, W. C., M. W. Li, and C. W. Liu. 2008. “Measurement of the Knowledge-Sharing Efficacy of Web2.0 Site Constructed on the Basis of Knowledge-based Systems by Applying the Model of UTAUT: Evidence of the early adopters”. Proceeds of the 3rd International Conference on Innovative Computing Information and Control (ICICIC’08).
- FAOSTAT Agriculture Data. 2013.
- Fishbein, M., and I. Ajzen. 1975. Belief, Attitude, Intention, and Behavior: An Introduction to Theory and Research. Reading, MA: Addison-Wesley.
- Fornell, C., and D. F. Larcker. 1981. “Evaluating Structural Equation Models with Unobservable Variables and Measurement Error.” Journal of Marketing Research 18 (1): 39–50.
- Hair Jr., J. F., W. C. Black, B. J. Babin, R. E. Anderson, and R. L. Tatham. 2006. Multivariate Data Analysis. 6th ed. Upper saddle River, NJ: Prentice-Hall International.
- Hair, J. F., R. L. Tatham, R. E. Anderson, and W. Black. 1998. Multivariate Data Analysis. 5th ed. London: Prentice-Hall.
- Hamilton, L. C. 2006. Statistics with Stata. Belmont, CA: Brooks/Cole.
- Islam, M. S., and Å Grönlund. 2011. “Factors Influencing the Adoption of Mobile Phones among the Farmers in Bangladesh: Theories and Practices.” ICTer 4 (1): 4–14.
- Jogo, W., E. Karamura, W. Tinzaara, J. Kubiriba, and A. Rietveld. 2013. “Determinants of Farm-Level Adoption of Cultural Practices for Banana Xanthomonas Wilt Control in Uganda.” Journal of Agricultural Science 5 (7): 70–81.
- Kabunga, N. S., T. Dubois, and M. Qaim. 2012. “Heterogenous Information Exposure and Technology Adoption: The Case of Tissue Culture Bananas in Kenya.” Agricultural Economics 43: 473–486.
- Karubanga, G., F. Matsiko, and S. Danielsen. 2017. “Access and Coverage: Which Farmers do Plant Clinics Reach in Uganda?” Development in Practice 27 (8): 1091–1102.
- Kline, R. B. 2005. Principles and Practice of Structural Equation Modeling. 2nd ed. New York: Guildford Press.
- Kraft, K. H., J. de Jesús Luna-Ruíz, and P. Gepts. 2010. “Different Seed Selection and Conservation Practices for Fresh Market and Dried Chile Farmers in Aguascalientes, Mexico.” Economic Botany 64 (4): 318–328.
- Kubiriba, J., E. B. Karamura, W. Jogo, W. K. Tushemereirwe, and W. Tinzaara. 2012. “Community Mobilization: A Key to Effective Control of Banana Xanthomonas Wilt.” Journal of Development and Agricultural Economics 4 (5): 125–131.
- Lakhal, S., H. Khechine, and D. Pascot. 2013. “Student Behavioural Intentions to Use Desktop Video Conferencing in a Distance Course: Integration of Autonomy to the UTAUT Model.” Journal of Computing in Higher Education 25 (2): 93–121.
- Liu, Y., H. Li, and C. Carlsson. 2010. “Factors Driving the Adoption of m-Learning: an Empirical Study.” Computers & Education 55 (3): 1211–1219.
- Loibl, C., S. H. Cho, F. Diekmann, and M. T. Batte. 2009. “Consumer Self-Confidence in Searching for Information.” Journal of Consumer Affairs 43 (1): 26–55.
- Lu, J., Y. E. Yao, and C. S. Yu. 2005. “Personal Innovativeness, Social Influences and Adoption of Wireless Internet Services via Mobile Technology.” Journal of Strategic Information Systems 14: 245–268.
- Lu, J., C. S. Yu, C. Liu, and J. E. Yao. 2003. “Technology Acceptance Model for Wireless Internet.” Internet Research 13 (3): 206–222.
- Lwandasa, H., G. H. Kagezi, A. M. Akol, J. W. Mulumba, R. Nankya, C. Fadda, and D. J. Jarvis. 2014. “Assessment of Farmers’ Knowledge and Preferences for Planting Materials to Fill-Gaps in Banana Plantations in Southwestern Uganda.” Uganda Journal of Agricultural Sciences 15 (2): 165–178.
- MacCallum, R. C., M. W. Browne, and H. M. Sugawara. 1996. “Power Analysis and Determination of Sample Size for Covariance Structure Modeling.” Psychological Methods 1 (2): 130–149.
- Maldonado, U. P. T., G. F. Khan, J. Moon, and J. J. Rho. 2009. “E-learning Motivation, Students’ Acceptance/Use of Educational Portal in Developing Countries.” Proceeds of the 4th International Conference on Computer Sciences and Convergence Information Technology.
- McDonald, R. P., and M. R. Ho. 2002. “Principles and Practice in Reporting Structural Equation Analyses.” Psychological Methods 7 (1): 64–82.
- Midgley, D. F., and G. R. Dowling. 1978. “Innovativeness: the Concept and its Measurement.” Journal of Consumer Research 4 (4): 229–242.
- Mulugo, Lucy. 2019. “Socio-Cultural Factors Influencing Uptake of Banana Tissue Culture Planting Materials in Central Uganda.” PhD diss., Makerere University.
- Muyanga, M. 2009. “Smallholder Adoption and Economic Impacts of Tissue Culture Banana in Kenya.” African Journal of Biotechnology 8: 6548–6555.
- Nejadrezaei, N., M. S. Allahyari, M. Sadeghzadeh, A. Michailidis, and H. El Bilali. 2018. “Factors Affecting Adoption of Pressurized Irrigation Technology among Olive Farmers in Northern Iran.” Applied Water Science 8 (6): 190.
- Njau, N., M. Mwangi, R. Kahuthia-Gathu, R. Muasya, and J. Mbaka. 2011. “Macropropagation Technique for Production of Healthy Banana Seedlings.” African Crop Science Conference Proceedings 10: 469–472.
- Nunnally, J. C., and I. H. Bernstein. 1994. Psychometric Theory. New York: McGraw- Hill.
- Okumus, B., F. Ali, A. Bilgihan, and A. B. Ozturk. 2018. “Psychological Factors Influencing Customers’ Acceptance of Smartphone Diet Apps When Ordering Food at Restaurants.” International Journal of Hospitality Management 72: 67–77.
- Rogers, E. M. 2003. Diffusion of Innovations. 5th ed. New York, NY: Free Press.
- RTB (Research Program in Roots, Tubers and Bananas). 2016. User’s Guide to the Multistakeholder Framework for Intervening in RTB Seed Systems. Lima: RTB.
- San Martín, H., and Á Herrero. 2012. “Influence of the User’s Psychological Factors on the Online Purchase Intention in Rural Tourism: Integrating Innovativeness to the UTAUT Framework.” Tourism Management 33 (2): 341–350.
- Segars, A. H. 1997. “Assessing the Uni-Dimensionality of Measurement: A Paradigm and Illustration Within the Context of Information Systems Research.” Omega International Journal of Management Science 25 (1): 107–121.
- Slade, E. L., Y. K. Dwivedi, N. C. Piercy, and M. D. Williams. 2015. “Modeling Consumers’ Adoption Intentions of Remote Mobile Payments in the United Kingdom: Extending UTAUT with Innovativeness, Risk, and Trust.” Psychology & Marketing 32 (8): 860–873.
- Smith, J. J., D. R. Jones, E. Karamura, G. Blomme, and F. L. Turyagyenda. 2008. An Analysis of the Risk from Xanthomonas campestris pv. musacearum to Banana Cultivation in Eastern, Central and Southern Africa. Bioversity International, Montpellier, France. ISBN, 978–2.
- Taiwo, A. A., and A. G. Downe. 2013. “The Theory of User Acceptance and use of Technology (UTAUT): A Meta-Analytic Review of Empirical Findings.” Journal of Theoretical & Applied Information Technology 49 (1): 48–58.
- Taylor, S., and P. Todd. 1995. “Decomposition and Crossover Effects in the Theory of Planned Behaviour: A Study of Consumer Adoption Intentions.” International Journal of Research in Marketing 12 (2): 137–155.
- Thiele, G. 1999. “Informal Potato Seed Systems in the Andes: Why are They Important and What Should We Do with Them?” World Development 27 (1): 83–99.
- Thompson, R., C. Higgins, and J. Howell. 1991. “Personal Computing: Toward a Conceptual Model of Utilization.” MIS Quarterly 15 (1): 125–143.
- Tripathi, L., M. Mwangi, S. Abele, V. Aritua, W. K. Tushemereirwe, and R. Bandyopadhyay. 2009. “Xanthomonas Wilt: A Threat to Banana Production in East and Central Africa.” Plant Disease 93: 440–451.
- Turan, A., AÖ Tunç, and C. Zehir. 2015. “A Theoretical Model Proposal: Personal Innovativeness and User Involvement as Antecedents of Unified Theory of Acceptance and Use of Technology.” Procedia-Social and Behavioral Sciences 210: 43–51.
- Tushemereirwe, W. K., J. Kubiriba, C. Nankinga, M. Masanza, J. Muhangi, F. Ssekiwoko, and E. Karamura. 2009. Proceedings of the Workshop on Review of the Strategy for the Management of Banana Xanthomonas Wilt. Kampala, Uganda, 25–35.
- Venkatesh, V., M. G. Morris, G. B. Davis, and F. D. Davis. 2003. “User Acceptance of Information Technology: Toward a Unified View.” MIS Quarterly 27 (3): 425–478.
- Venkatesh, V., J. Y. Thong, and X. Xu. 2012. “Consumer Acceptance and Use of Information Technology: Extending the Unified Theory of Acceptance and use of Technology.” MIS Quarterly 36 (1): 157–178.
- Wambugu, F., M. Karembu, M. Njuguna, and S. W. Wanyangu. 2000. Biotechnology to Benefit Small-Scale Banana Producers in Kenya. Global Development Network, New Delhi Google Scholar.
- Wu, L. 2012. “An Empirical Research on Poor Rural Agricultural Information Technology Services to Adopt.” Procedia Engineering 29: 1578–1583.
- Wu, Y., Y. Tao, and P. Yang. 2007. “Using UTAUT to Explore the Behavior of 3G Mobile Communication Users.” In Proceedings of The International Conference on Industrial Engineering and Engineering Management (IEEM).
- Yi, Jackson, and Park Probst. 2006. “Understanding Information Technology Acceptance by Individual Professionals: Toward an Integrative View.” Information and Management 43: 350–363.
- Zhou, D., and Abdullah. 2017. “The Acceptance of Solar Water Pump Technology among Rural Farmers of Northern Pakistan: A Structural Equation Model.” Cogent Food & Agriculture 3 (1): 1280882.