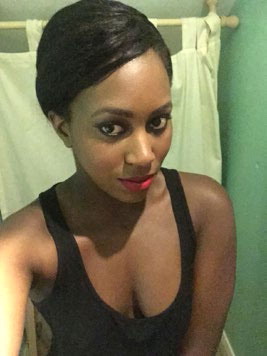
WE ARE ALL BECOMING INCREASINGLY AWARE OF THE VALUE OF OUR DATA, and the desire to share it without the concept of a value exchange is dwindling. A true and widely accepted model for the value exchange has yet to be developed, and as a result the ability for organisations to share data is slowing down data innovation. From an organisation perspective, regulations like GDPR and an increased desire for privacy among consumers are driving this cautionary approach when it comes to data. As a result, these organisations are keen to embrace technological advances that mean they can share data and derive insights whilst maintaining compliance with the demands of both consumers and regulators. There are a number of ways in which this problem is being approached, but the one that I want to discuss in this penultimate article of the data series is Synthetic Data.
As synthetic data is anonymous and exempt from data protection regulations, this opens up a whole range of opportunities for otherwise locked-up data, resulting in faster innovation, less risk and lower costs. This article covers what it is, how it’s generated and the potential applications.
WHAT ARE SYNTHETIC DATA AND HOW ARE THEY GENERATED?
Synthetic datasets are any production data applicable to a given situation that are not obtained through direct measurement, and are generated to meet specific needs or conditions. This is very useful when either privacy needs limit the availability/usage of the data or when the data need for a test environment does not exist.
There are three main types of synthetic data:
Fully synthetic data – contains no original data.
Partially synthetic data – only selected sensitive values are replaced with synthetic data.
Hybrid synthetic data – generated using both synthetic and original data.
There are two primary methods used to generate synthetic data:
Drawing numbers from a distribution – The principle is to observe real-world statistical distributions from the original data and reproduce artificial data according to these distributions. This can also include the creation of generative models.
Agent based modelling (see ) – The principle is to create a model that explains the observed behaviour, then reproduce random data using this model. It is generally agreed that observed features of a complex system can often emerge from a set of simple rules.
Historically synthetic data generation has been mostly developed within academia, however there are now a number of commercial organisations that are bringing the capabilities to market (see Hazy – www.hazy.com and Mostly – www.mostly.ai as two great examples).
SYNTHETIC DATA VS ANONYMISATION: WHAT’S THE DIFFERENCE?
To understand the need for anonymisation (see ) and synthetic data, we must first establish three definitions:
FIGURE 2 THE ODI DATA SPECTRUM (SHOWING HOW DIFFERENT LEVELS OF ANONYMISATION CAN MAKE CLOSED DATA, MEDICAL RECORDS, SHARED AND/OR OPEN DATA)
© The Open Data Institute
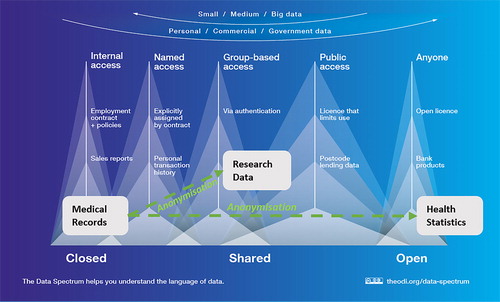
Personal data – defined by GDPR as any information relating to an identified or identifiable natural person (‘data subject’); an identifiable natural person is one who can be identified, either directly or indirectly, in particular by reference to an identifier such as a name, an identification number, location data an online identifier or to one or more factors specific to the physical, physiological, genetic mental, economic, cultural or social identity of that natural person.
Private information – defined by the Office for National Statistics as:
Relating to an identifiable legal or natural person;
Not in the public domain or common knowledge;
Information that if disclosed, would cause the subject damage, harm or distress.
Sensitive information – defined as a sensitive asset that if compromised can cause serious harm to an organisation.
For data to be shared between organisations it must be modified; for sensitive data in general this is done by redaction and for personal data this is done by anonymisation. Anonymisation is a process that alters a dataset to reduce the risk of re-identification as much as possible. Modern anonymisation techniques tend to fall into three categories:
Suppression – removing identifiers or pieces of information that may lead to re-identification;
Generalisation – aggregating data points into a coarser granularity, or otherwise removing details to obfuscate data about people on an individual basis;
Disruption – adding noise and changing values to the extent that it is increasingly difficult to know how, or whether, information about specific individuals can be recovered or inferred.
Synthetic data methodology can be best described as a subset of anonymisation created by an automated process such that it holds similar statistical patterns as the original dataset. Each individual record may have no relation to reality but, when viewed in aggregate, the dataset is still useful for certain analyses and for testing software. If done correctly, synthetic datasets can contain no personal data, which eliminates the risk of or re-identification.
POTENTIAL USE CASES
The applications and potential use cases for synthetic are vast, including analytics, machine learning, and data modelling. Fraud detection and healthcare are described in more detail here as being prominent areas where considerable work is taking place, but these are just two examples. The important thing to remember is that being able to share granular level statistically representative synthetic data with external organisations (including start-ups and researchers) can lead to truly disruptive innovations in a much shorter time frame.
Financial services and fraud detection
Standard anonymisation is often insufficient for financial transaction data; it has been demonstrated that 80% of credit card owners could be re-identified by only three transactions, even when just the merchant and date of the transaction are revealed. Synthetic data maintains the majority of the valuable information and statistical integrity of the original data but eliminates the risk of re-identification.
The ability to generate synthetic fraud data is of great benefit to the financial services sector because it allows for collaboration amongst organisations. This collaboration, which is inherent between fraudulent actors, can give organisations a valuable speed advantage which allows them close fraudulent loopholes more quickly. Patterns of fraudulent behaviour can not only be recreated in synthetic data, but the signal can also be amplified, allowing machine learning models to be trained more quickly and comprehensively. The PaySim mobile money simulator (https://www.kaggle.com/ntnu-testimon/paysim1) is an example of using aggregate data from a private dataset to generate a synthetic equivalent that resembles the normal operation of transactions. Malicious behaviour was injected into the synthetic dataset to evaluate the performance of fraud detection models.
Healthcare
There has been considerable interest in using synthetic data within the healthcare sector to help researchers answer important questions about diseases, and to improve the efficiency and effectiveness of services in the sector. The Simulacrum is a synthetic database that imitates some of the cancer data held securely by the National Cancer Registration and Analysis Service (NCRAS). Access to this data can give analysts and researchers the ability to answer questions that will broaden their understanding of cancer, whilst protecting the confidentiality of the patient. Because this data is entirely synthetic, it removed the need for many of the essential controls that are required to access the real data held by Public Health England (PHE).
Although the data is synthetic, the Simulacrum maintains most of the properties of the original data with a high degree of accuracy. It is important to remember that the more complex the data query the more approximate the results, which is a limitation of using synthetic data. However, because the data model (but not the data) is the same as the real model in the Cancer Analysis System in PHE, researchers can use the Simulacrum to plan and test their hypotheses before making a formal request to PHE to analyse the real data. This database was developed by Health Data Insight in partnership with IQVIA and AstraZeneca.
The Open Data Institute are also currently looking to release a synthetic dataset of A&E admissions.
THE FUTURE OF SYNTHETIC DATA
Sample-free population synthetic population generation – It is possible to generate synthetic population in all geographies as long as there is widely available public data. Using sample-free synthetic reconstruction methods gives us the capability to generate synthetic populations without any sample data to use as a basis.
Research has shown that the sample-free method of synthetic reconstruction can give globally better results than the sample-based methods. However, it should be noted that although the sample-free method is much less data demanding, the data requires much more pre-processing before use. This method of population generation is currently being used in academia and there is definite commercial opportunity that could take advantage of this technology.
Additional information
Notes on contributors
Louise Maynard-Atem
Louise Maynard-Atem is an innovation specialist in the Data Exchange team at Experian. She is the co-founder of the Corporate Innovation Forum and is an advocate for STEM activities, volunteering with the STEMettes and The Access Project.