ABSTRACT
The rapid development of intelligent navigation drives the rapid accumulation of ocean data, and the ocean science has entered the era of big data. However, the complexity and variability of the ocean environments make some data unavailable. It makes ocean target detection and the unmanned surface vehicle (USV) intelligent control process in ocean scenarios face various challenges, such as the lack of training data and training environment. Traditional ocean image data collection method used to capture images of complex ocean environments is costly, and it leads to a serious shortage of ocean scene image data. In addition, the construction of an autonomous learning environment is crucial but time-consuming. In order to solve the above problems, we propose a data collection method using virtual ocean scenes and the USV intelligent training process. Based on virtual ocean scenes, we obtain rare images of ocean scenes under complex weather conditions and implement the USV intelligent control training process. Experimental results show that the accuracy of ocean target detection and the success rate of obstacle avoidance of the USV are improved based on the virtual ocean scenes.
Disclosure statement
No potential conflict of interest was reported by the authors.
Data availability statement
The data referred to in this paper is not publicly available at the current time.
Additional information
Funding
Notes on contributors
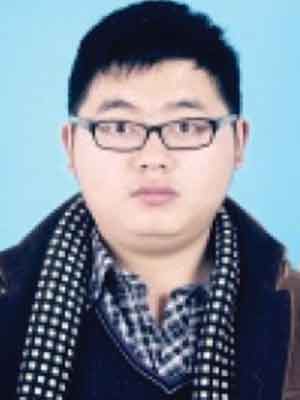
Wei Wang
Wei Wang received the B.Eng. degrees in the School of Computer Science and Technology, Fuyang Normal University, China, in 2012. Received the M.Eng degree in the School of Information Engineering and Automation, Kunming University of Science and Technology, in 2016. He is currently working toward the Ph.D. degree at the School of Computers, Shanghai University, China. His research interests include deep reinforcement learning, Intelligent unmanned system.
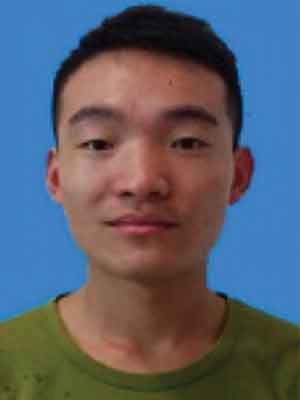
Yang Li
Yang Li received the B.Eng. degrees from the School of Computer Science and Technology, Anhui University, China, in 2017. He is currently working toward the Ph.D. degree at the School of Computers, Shanghai University, China. His research interests include deep reinforcement learning, multi-agent reinforcement learning, and multi-agent system.
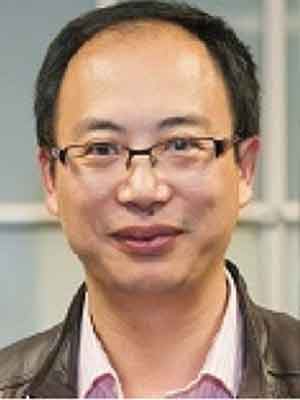
Xiangfeng Luo
Xiangfeng Luo is a professor in the School of Computers, Shanghai University, China. Currently, he is a visiting professor at Purdue University, USA. His main research interests include Web Wisdom, Cognitive Informatics, and Text Understanding. He has authored or co-authored more than 100 publications and his publications have appeared in IEEE Trans. on Systems, Man, and Cybernetics-Part C, IEEE Trans. on Automation Science and Engineering, IEEE Trans. on Learning Technology, etc. He has served as the Guest Editor of ACM Transactions on Intelligent Systems and Technology. Dr. Luo has also served on the committees of a number of conferences/workshops, including Program Co-chair of ICWL 2010 (Shanghai), WISM 2012 (Chengdu), CTUW2011 (Sydney) and PC member for more than 40 conferences and workshops.
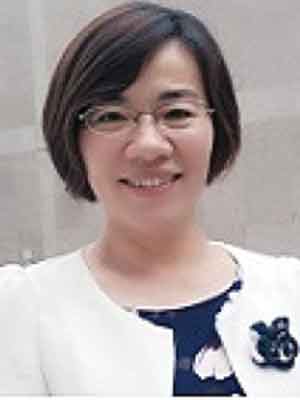
Shaorong Xie
Shaorong Xie received the B.Eng. and M.Eng. degrees in mechanical engineering from Tianjin Polytechnic University, Tianjin, China, in 1995 and 1998, respectively, and the Dr. Eng. degree in mechanical engineering from the Institute of Intelligent Machines at Tianjin University and the Institute of Robotics and Automatic Information System, Nankai University, Tianjin, China, in 2001. She is a Professor with the School of Mechatronic Engineering and Automation, Shanghai University, Shanghai, China. Her research areas include advanced robotics technologies, bionic control mechanisms of eye movements, and image monitoring systems.