ABSTRACT
Although we live in an era of unprecedented quantities and access to data, deriving actionable information from raw data is a hard problem. Earth observation systems (EOS) have experienced rapid growth and uptake in recent decades, and the rate at which we obtain remotely sensed images is increasing. While significant effort and attention has been devoted to designing systems that deliver analytics ready imagery faster, less attention has been devoted to developing analytical frameworks that enable EOS to be seamlessly integrated with other data for quantitative analysis. Discrete global grid systems (DGGS) have been proposed as one potential solution that addresses the challenge of geospatial data integration and interoperability. Here, we propose the systematic extension of EASE-Grid in order to provide DGGS-like characteristics for EOS data sets. We describe the extensions as well as present implementation as an application programming interface (API), which forms part of the University of Minnesota’s GEMS (Genetic x Environment x Management x Socioeconomic) Informatics Center’s API portfolio.
Disclosure statement
No potential conflict of interest was reported by the author(s).
Data availability statement
There are no data associated with this manuscript.
Supplementary Material
Supplemental data for this article can be accessed online at https://doi.org/10.1080/20964471.2021.2017539.
Additional information
Funding
Notes on contributors
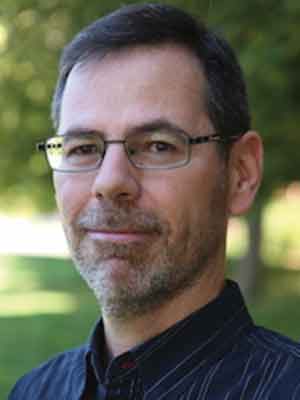
Jeffery A. Thompson
Jeffery A. Thompson is a High-Performance Computing Geospatial Scientist within the Minnesota Supercomputing Institute (MSI) at the University of Minnesota. He obtained a masters degree in Geographic Information Sciences and a second masters in Resource Science from the University of New England, Australia. He also holds a PhD in Geography from the University of New South Wales, Australia. He has more than a fifteen year of experience applying remote sensing and geospatial data within Earth and environmental sciences. His primary research interest is in applying remotely sensed image time-series to phenological studies, and he is particularly interested in im- proving our understanding of the interactions between vegetation and eco-hydrological dynamics. Having worked on projects in agriculture, mining, resource management and environmental issues, his geospatial experience is wide ranging.
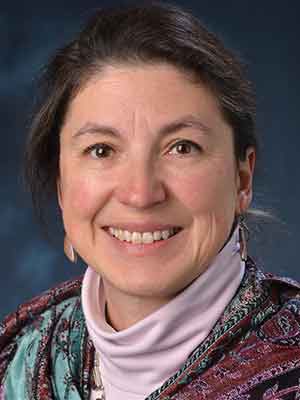
Mary J. Brodzik
Mary J. Brodzik received the B.A. degree summa cum laude in mathematics from Fordham University, Bronx, NY, USA, in 1987. She is a Senior Associate Scientist at the National Snow & Ice Data Center (NSIDC), Cooperative Institute for Research in Environmental Sciences (CIRES), University of Colorado Boulder, Boulder, Co, USA. Since coming to NSIDC in 1993, she has implemented software systems to de- sign, produce, and analyze snow and ice data products from satellite-based visible and passive microwave imagery. She has contributed to the NSIDC data management and software development teams for the NASA Cold Lands Processes Experiment and Operation IceBridge. As a Principal Investigator of the NASA Making Earth Science Data Records for Use in Research Environments Calibrated Enhanced-Resolution Brightness Temperature Project, she managed the operational production of a 40+ year EASE- Grid 2.0 Earth Science Data Record of satellite passive microwave data, and related enhanced-resolution SMAP images. She is using MODIS data products to derive the first systematically derived global map of the world’s glaciers. For the U.S. Agency for International Development, she developed snow and glacier ice melt models to better understand the contribution of glacier ice melt to major rivers with headwaters in High Asia. Prior to her work at NSIDC, she worked in software development, validation and verification on Defense Department satellite command and control and satellite track- ing systems. Her research interests include optical and passive microwave sensing of snow, remote sensing data gridding schemes, and effective ways to visualize science data.
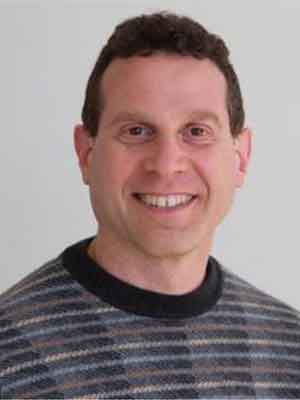
Kevin A. T. Silverstein
Kevin Silverstein is Scientific Lead for the Research Informatics Solutions (RIS) group at the Minnesota Supercomputing Institute (MSI) and Operations Manager of the GEMS Agroinformatics Initiative. He has spent decades performing large-scale bioinformatics analyses involving cutting-edge high-throughput data from bacteria, fungi, plants, mammals and complex communities. He has performed detailed investigations of plant-microbe systems in continued research since 2001. Additionally, in 2010–2012, he led an effort to identify mutations in clinical patients with Fairview Hospital which has been expanded in partnership with MSI and continues to be used today. The knowledge gained from handling protected patient data has been brought over to the GEMS platform to protect farmer and corporate data.
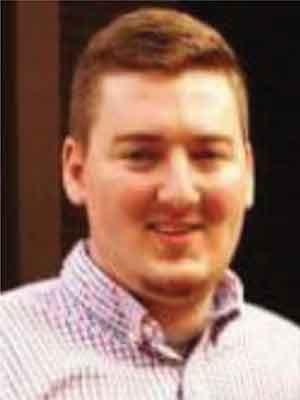
Mason A. Hurley
Mason Hurley is a Data Engineer within the GEMS Agroinformatics Center (GEMS). He graduated from the University of Minnesota with an M.S. in Applied Economics. He spent several years as a Data Scientist at the InSTePP research center where he worked on analytical workflow design and automation while continuing his graduate research on statistical theory and the spatial/temporal dynamics of farm size distributions. He is currently working on expanding GEMS’ geospatial functionality as well as the design and implementation of high-performance data analysis pipelines.
Nathan L. Carlson
Nathan Carlson is a Systems Operations Engineer at the University of Minnesota’s Supercomputing Institute. He graduated from the University of North Dakota with degrees in Computer Science and Physics. His work with large-scale computing has always been in support of some spatial-temporal analysis including near-ground weather data to support departments of transportation and farmers, climate model data made accessible by the Earth System Grid Federation for the CMIPs at Lawrence Livermore National Lab and now with GEMS at the University of Minnesota.