ABSTRACT
A Discrete Global Grid System (DGGS) is a type of spatial reference system that tessellates the globe into many individual, evenly spaced, and well-aligned cells to encode location and, thus, can serve as a basis for data cube construction. This facilitates integration and aggregation of multi-resolution data from various sources to rapidly calculate spatial statistics. We calculated normalized area and compactness for cell geometries from 5 open-source DGGS implementations - Uber H3, Google S2, RiskAware OpenEAGGR, rHEALPix by Landcare Research New Zealand, and DGGRID by Southern Oregon University - to evaluate their suitability for a global-level statistical data cube. We conclude that the rHEALPix and OpenEAGGR and DGGRID ISEA-based DGGS definitions are most suitable for global statistics because they have the strongest guarantee of equal area preservation - where each cell covers almost exactly the same area on the globe. Uber H3 has the smallest shape distortions, but Uber H3 and Google S2 have the largest variations in cell area. However, they provide more mature software library functionalities. DGGRID provides excellent functionality to construct grids with desired geometric properties but as the only implementation does not provide functions for traversal and navigation within a grid after its construction.
Acknowledgement(s)
The authors would like to thank the reviewers and the editor for the constructive feedback and the insightful comments on the manuscript which greatly improved the work.
Disclosure statement
No potential conflict of interest was reported by the author(s).
Data availability statement
The data that support the findings of this study are openly available on “Zenodo” at https://doi.org/10.1080/20964471.2022.2094926, reference number 6634479 Version v1.0.2.
Supplementary data
Supplemental data for this article can be accessed online at https://doi.org/10.1080/20964471.2022.2094926.
Additional information
Funding
Notes on contributors
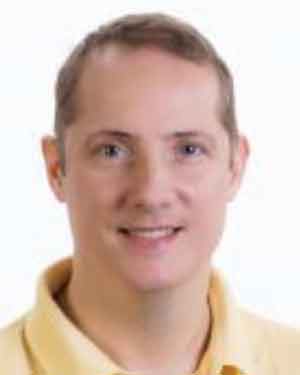
Alexander Kmoch
Alexander Kmoch, PhD, is a Research Fellow in Geoinformatics at the University of Tartu. He works on Distributed Spatial Systems and has many years of international experience in geospatial data management and web- and cloud-based geoprocessing with a particular focus on land use, soils, hydrology, and water quality. His interests include OGC standards and web-services for location-based data sharing, modeling workflows, machine learning, and interactive geo-visualisation.