Abstract
The water buffalo is among the most important livestock species of southern Asia, contributing greatly to the ecosystem and rural livelihood of the region. The identification of large-scale single nucleotide polymorphisms in this species would greatly facilitate our understanding of the genetic basis of economically important traits such as milk production, fertility traits and general health traits. The present study investigated the cost-effective method of exome capture and single nucleotide variant (SNV) identification from genomic DNA of Jaffrabadi buffalo using biotin-labelled cDNA as probes. Sequencing of enriched fragments generated 608 Mb of data, which was mapped to a Bos taurus genome assembly followed by variant calling and annotation. Furthermore, 393 coding SNVs were identified, leading to 143 non-synonymous substitutions (nsSNVs) in 75 genes. Of the 75 nsSNV-containing genes, four matched the genes that have previously been reported to be potentially associated with economically important traits such as milk production and meat production. Furthermore, functional annotation using gene ontology (GO) enrichment identified categories such as glutamate receptor activity (GO: 0008066) enriched in the fertility trait samples. These results provide a framework for the application of cost-effective methods of target capture in SNV detection from non-model organisms such as the water buffalo.
Introduction
Water buffalo (Bubalus bubalis) is an important livestock species in many Asian countries and especially in India, where it contributes around 56.85% of total milk production despite its being lower in number than the cattle population (FAOSTAT Citation2010). It also makes a more significant economic contribution in terms of meat, skin-hides and draught power (Roth Citation2004) than cattle in this region. The Jaffrabadi breed of buffalo, the heaviest among all the Indian buffalo breeds, is found and bred around the Gir forest region in Gujarat state, India. This breed yields an appreciable quantity of milk (∼2200 kg/lactation), with an exceptionally high butter-fat content, which varies from 7% to 9% and may be as high as 12–14% in some animals (GLDB Citation2014).
The Human Genome Project initiative has opened the door for the discovery of disease genes and provided a new dimension in medical research. The techniques and approaches applied to the human genome have frequently been applied to livestock genomics and, in turn, livestock genomics has contributed to informing the human genome. In addition to complementing the human genome, livestock genomics, together with agriculture genomics, has a unique responsibility in the quest to feed an expanding world population while minimizing environmental and ecological risks. Clearly, the identification of variation in livestock genomes that predisposes to health and productivity with less reliance on hormones, antibiotics and pesticides will be a major step in meeting this global challenge (Womack Citation2005). In recent times, many such studies (Georges Citation2007; Jiang et al. Citation2010; Bolormaa et al. Citation2011; Utsunomiya et al. Citation2013) have been carried out to identify genetic differences in the form of molecular markers, such as single nucleotide polymorphisms (SNPs) responsible for or associated with variations in phenotypic trait, particularly those of economic importance. Understanding this genetic basis of phenotypes generally requires genotyping thousands of gene-targeted loci, genome wide. With the advent of next generation sequencing (NGS) technologies, the process of identifying genetic variants such as SNPs from whole genome sequencing has geared up substantially (Bardley Citation2012). However, detecting SNPs from a whole genome resequencing approach is still expensive and suitable only for those species for which the reference genome sequences are available. On the other hand, exome sequencing or targeted sequencing of the selective genomic region is a cost-effective approach for identifying functional variants inside approximately 2% of the coding sequences. Exon capture enriches exon DNA by simultaneous hybridization of fragmented genomic DNA from the study individual to many thousands of oligonucleotide probes that are complementary to gene coding (exon) sequences. The captured fragments are then sequenced in parallel on NGS platforms (Gnirke et al. Citation2009; Ng et al. Citation2009). But the non-availability of such commercial capture array/probes for farm animals has restricted the application of exome sequencing, especially for domestic animals such as the buffalo whose draft genome is yet to be made publicly available (Jakhesara et al. Citation2012).
In the present study, targeted capture (Lovett et al. Citation1991) was performed through biotin-labelled cDNA probes prepared from the RNA of various tissues, followed by sequencing of enriched genomic DNA fragments on 454 GS-FLX and Ion Torrent PGM. The resulting sequences were then aligned to the bosTau7 assembly and SNPs were identified. The goal of the present study was to assess and analyse the high-throughput data obtained from sequencing of cDNA-driven hybridization of genomic DNA for the detection of single nucleotide variants (SNVs) following the appropriate bioinformatics procedure.
Material and methods
Tissue sampling for capture probe preparation
Various tissues (liver, spleen, lymph node, endometrium, skin, follicle, pituitary, ovary and mammary gland) from buffalo with different physiological conditions (e.g. heifer, dry, lactating and pregnant) were collected from the government slaughterhouse. Tissue samples were rinsed twice with sterile RNAse-free phosphate-buffered saline (PBS) and chopped into 1 cm pieces. Finely chopped tissues were transferred to CryoVials® containing RNAlater® and stored at room temperature for 2 h followed by −20°C overnight storage, and finally transferred to liquid nitrogen at −196°C.
Blood sampling for preparation of target libraries
Five millilitre blood samples from three phenotypic trait groups, each comprising eight animals (except for the milk production trait group, which comprised seven animals), were collected in K3EDTA® vacutainers and brought to the laboratory on ice. The animals were grouped on the basis of three economically important traits: general health trait, fertility trait and milk production trait. Within each trait group, samples belonged to one of two phenotypic subgroups: disease resistance (jdr) or disease susceptible (jds) for the general health trait group, infertile (jif) or fertile (jf) for the fertility trait group, and high production (jhp) or low production (jlp) for the milk production trait group. Each phenotypic extreme subgroup consisted of four animals (except for the jhp subgroup, which consisted of three animals) ().
Table 1. Details of samples and their categorization based on condition, under fertility trait, general health trait and milk production based on filed records.
Biotin-labelled cDNA preparation
Total RNA was isolated from various tissues using the standard TRIZOL® protocol (Invitrogen™ Life Technologies [CA, USA]) along with the RNeasy® minikit protocol, following the manufacturer's instructions. Contaminating genomic DNA was removed from total RNA by DNAse treatment using RNAse-free DNAse (Qiagen [GmbH, Germany]). RNA quantity and quality assessment was performed on a Nanodrop ND1000 spectrophotometer (Thermofischer Scientific [CA, USA]) and RNA nano6000 chip (Agilent Technologies [CA, USA]). Once quantified, RNA from all tissues was pooled in equimolar proportion (500 ng of each tissue). Later, biotin-labelled cDNA was synthesized from the pool of RNA using biotin-labelled oligo dT with a Transcriptor First strand cDNA synthesis kit (Roche [Branford, CT USA]).
Genomic library preparation
Genomic DNA was extracted from blood samples collected from Jaffrabadi buffalo using NonidetP-40 and a standard phenol–chloroform extraction method. To prepare a library, 2 µg genomic DNA was fragmented and adapters were ligated following the manufacturer's protocol (GS-FLX Titanium and Ion Torrent PGM).
cDNA-driven target capture
The genomic DNA library was hybridized (1:10) with biotinylated cDNA from pooled tissue along with 2.5 µg of Cot DNA. Here, pooled cDNA was taken in excess so that exons encoding rarely expressed transcripts could also be captured. Hybridization was performed in 2× hybridization buffer (10× SSPE, 10× Denhardt's, 10 mM EDTA and 0.2% SDS). Hybridization was maintained for 24 h at 66°C followed by enrichment of the captured library using streptavidin beads (Qiagen, GmbH Germany). Captured sequences were eluted and purified using Melt solution (100 nM NaOH) and the Mini elute PCR purification kit, following the manufacturer's protocol (Qiagen, GmbH Germany). Enriched or captured libraries were further amplified for eight cycles using primers complementary to the adaptors used for library preparation. Amplified enriched libraries were purified by Ampure XP beads (Beckman Coulter, CA, USA), followed by quantification using the Agilent 2100 Bioanalyzer High Sensitivity chip (Agilent Technologies, CA USA), and subjected to emulsion PCR.
High-throughput sequencing and data analysis
DNA-positive beads from emulsion PCR were recovered, enriched with enrichment beads and subjected to high-throughput sequencing using Roche GS-FLX Titanium and Ion Torrent PGM sequencers.
Preprocessing of next generation sequencing raw data
Sff files obtained from the Roche GS-FLX 454 next generation sequencer were converted into fastq format using Biopython (Cock et al. Citation2009). Later, fastq files containing NGS data were used for quality filtering using PRINSEQ (Schmieder & Edwards Citation2011). Quality criteria used in PRINSEQ were a filter sequence shorter than 40 bp and a filter sequence with a mean quality score below 20. Sequences satisfying the quality criteria were retained and later used for mapping against the Bos taurus genome.
Reference-guided assembly
Individual samples were mapped on to the reference B. taurus genome using CLC Genomics Workbench (version 4.9) with the following parameters: (i) mismatch cost set as 2; (ii) insertion cost set as 3; (iii) deletion cost set as 3; and (iv) length fraction matches to the reference set as 0.5, with minimum similarity of 0.8. After mapping, picard ‘MarkDuplicate’ was used to remove duplicate reads from the alignment files.
Single nucleotide variant calling and annotation
SAMtools mpileup was used for consensus calling from individual samples in relation to the reference (Li et al. Citation2009). Consensus calling was performed only for high-quality bases (Q > 20). Later, Bcftools was used for SNV calling. SNV annotation was performed using SnpEff (Cingolani et al. Citation2012).
Gene enrichment analysis
The Database for Annotation, Visualization and Integrated Discovery (DAVID) was used to identify enriched GO categories (Huang da et al. Citation2009), with the B. taurus gene set as the background. Files containing changed genes were used as input for enrichment analysis. Categories with a false discovery rate (FDR) value < 0.05 were considered significantly enriched in this study.
Results
Assessment and mapping of next generation sequencing data
A total of 608 Mb of data comprising approximately 4 million reads was generated by sequencing 23 samples on GS-FLX and Ion Torrent PGM. After stringent quality filtering, approximately 425 Mb of data with around 2 million reads was retained for downstream analysis (). After quality filtering, 92% of filtered reads were mapped to the cattle genome with approximately 8× coverage. Post-mapping, around 25,000 reads were discarded owing to PCR duplication.
Table 2. Number of reads obtained after quality filtering and mapping under different trait groups.
Table 3. Number of single nucleotide polymorphisms (SNPs) detected in different samples of various groups.
Single nucleotide variant calling, annotation and probable variant detection
In SAMtools (Li et al. Citation2009), variant calling detected 2838 SNVs from the general health trait group, 36,743 SNVs from the fertility trait group and 56,996 SNVs from the production trait group (). Of the identified SNVs, 72,465 were located in off-target regions and 3974 in flaking regions. The remaining 16,998 SNVs were located within the gene regions. The SNVs in the gene region annotated using SnpEff were identified as 16,615 SNVs in the intronic region, 199 in the untranslated region (UTR), one variant in the splice site and 393 exonic SNVs leading to 143 non-synonymous nucleotide substitutions. Non-synonymous substitutions (nsSNVs) were distributed among 75 genes () (see Supplementary Table S1).
Figure 1. Distribution of annotated single nucleotide polymorphisms (SNPs). The highest number of SNPs was detected in the intergenic region, followed by the intron; the secondary pie chart shows the distribution of 393 SNPs detected in the exons of the genes.
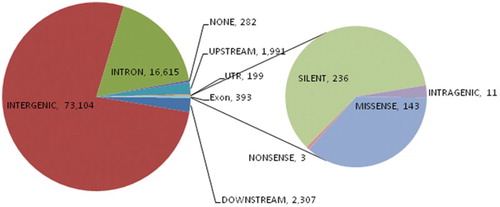
Figure 2. Distribution of single nucleotide polymorphisms (SNPs) annotated in various genic regions of the 23 individuals under study: colour formatting indicates high and low values. Lower numbers are presented in dark green to light green colours, whereas yellow to red colours indicate higher numbers of SNPs.
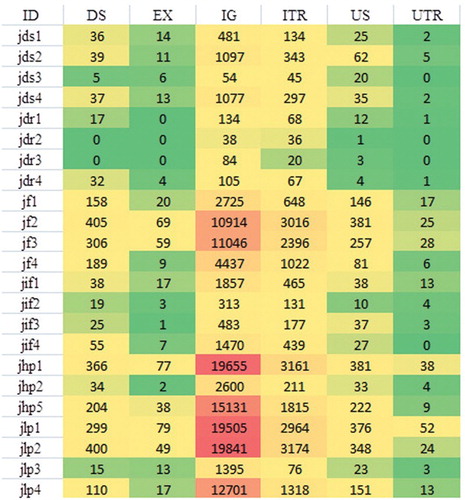
The distribution of the SNPs, with annotation for each sample, is shown in and Supplementary Tables S2–S4. Of the total 24 SNVs identified in the exons of the general health trait samples, 20 SNVs were exclusively present in the disease-susceptible (jds) subgroup while four SNPs were exclusively present in the disease resistance (jdr) subgroup. All four SNPs in the jdr subgroup were located in the same gene: SLC9A3R2 (one mis-sense and three silent mutations). Out of the 20 SNVs identified in jds samples, 12 SNVs were detected in the LRRC32 gene (five non-synonymous and seven silent changes). Of the 146 SNVs identified in the fertility trait group, 123 SNVs were exclusively present in the fertility (jf) subgroup while 15 SNVs were exclusively present in the infertile (jif) subgroup. Of the 15 exonic SNVs in the infertile subgroup samples, four SNVs were present in the ZNF165 (one missense and three silent mutations) gene alone. In the production trait group, of the 105 SNVs exclusively detected in high-production samples, 37 were non-synonymous substitutions, whereas, of the 144 SNVs in low-production samples, 44 were non-synonymous substitutions.
The variants present in all the samples of one phenotype of a particular trait (e.g. low milk production in milk production trait), but absent in all the samples of another phenotype of the same trait (e.g. high milk production in milk production trait), and vice versa, were also extracted. All the samples of the disease-susceptible (jds) group harboured three variants in the exon of the LRRC32 gene, which were absent in all the samples of the disease resistance subgroup; no such SNP was detected when the condition was reversed. In the fertility trait group, all the samples of the fertility subgroup harboured 104 SNVs, which were absent in all the samples of the infertility subgroup; again, no such SNP was detected when the condition was reversed. All the samples of the high milk production subgroup carried one intergenic SNV, which was not present in any of the low milk production samples; two such intergenic SNVs were detected when the condition was reversed.
Functional annotation of genes harbouring single nucleotide variants
Functional analysis of the SNVs identified in the genes was performed using DAVID (Huang da et al. Citation2009). Initially, genes harbouring nsSNVs exclusively found in each of the phenotypic subgroups of the traits were selected to discover the pathway or categories of genes enriched. But no significant enriched category (p < 0.05 after FDR multiple correction) was found, indicating that these genes were affected at random. Thus, all the genes harbouring SNVs, irrespective of their position (intron, UTR, exon), were selected for each trait group separately. The analysis showed that genes from the general disease trait group did not show enrichment of any GO category, while the fertile and infertile subgroups showed enrichment of genes under GO categories mostly associated with molecular functions such as ion binding and transporter channel activity (), although none of the categories was common in both. Genes in the high milk production subgroup showed enrichment of 13 GO categories (), of which six GO categories, associated mainly with metabolic processes and ion transport, related to biological processes; another six, associated with nucleoside binding and calcium ion binding, related to molecular functions; and one, associated with the plasma membrane, related to cellular components. Genes in the low milk production subgroup showed enrichment of 20 GO categories (), of which six GO categories were associated with biological processes such as calcium and metal ion transport, while 12 GO categories were associated with molecular functions such as calcium ion binding, ATP binding and channel activity.
Table 4. List of gene ontology (GO) categories with their false discovery rate (FDR) values enriched in fertility and infertility trait samples.
Table 5. List of top gene ontology (GO) categories with their false discovery rate (FDR) values enriched in high milk production trait samples.
Table 6. List of gene ontology (GO) categories with their false discovery rate (FDR) values enriched in low milk production trait samples.
Discussion
In the present study, NGS runs generated altogether 608 Mb of data which, after quality filtering, was reduced to 425 Mb. The reference assembly of B. taurus (Eck et al. Citation2009) was used for mapping purposes, as the most recent common ancestor of the domestic cow and the river buffalo is estimated to have existed recently, approximately 5–10 million years ago (MacEachern et al. Citation2009). Mapping using CLC Genomics (version 4.9) resulted in a mapping percentage of more than 92% of total buffalo reads. The high mapping percentage obtained in the present study (∼92%) may be due to the study design, which targeted the coding region of the genome, which is highly conserved across genomes. The approach, type of data and sequencing platform used in other studies may also be reasons for their lower mapping rate (le Roex et al. Citation2012) compared to that of targeted sequencing data. Duplicate reads were removed, as they sometimes inappropriately increase the vertical depth in SNV calling. As the proportion of off-target capture was higher than in other studies, the capture protocol could be further improved using more stringent two-step hybridization protocols along with Cot DNA (Barbazuk et al. Citation2005). Moreover, the hybridization temperature can be optimized and the library fragment size reduced (Fu et al. Citation2010), which will help in the further reduction of off-target sequences. More than 97% of SNP detection in off-target regions also points towards the importance of SNPs located in the non-coding region for association studies, as suggested by Guo et al. (Citation2012). The present authors examined whether the 75 genes containing non-synonymous mutations were reportedly associated with economically important traits such as milk production, meat production and growth rate in other breeds of cattle. The current results were compared with the list of genes containing nsSNPs associated with economically important traits obtained in the study by Kawahara-Miki et al. (Citation2011). Of the 75 genes containing nsSNPs, four (Supplementary Table S5) matched the genes that are potentially associated with economically important traits such as milk production and meat production. LRRC32, also known as GARP, harboured variants present in all the disease-susceptible samples, indicating the role it plays in immunity. GARP is well documented as a surface receptor of expanded T-regulatory cells and platelets, and is a member of the leucine-rich repeat family that exhibits evolutionary similarity to Toll-like receptors (Böttcher et al. Citation2003). GARP has been shown to be expressed in placenta, lung, kidney, heart, liver, skeletal muscle and pancreas, but not brain (Ollendorff et al. Citation1994). Therefore, the present findings provide additional evidence of its association with general disease immunity.
Genes associated with the GO category of glutamate receptor activity (GO: 0008066), such as GRIA1 and GRIN2B, are reported to be associated with ovulation and fertility in females (Sugimoto et al. Citation2010; Schuh-Huerta et al. Citation2012). The present study provides evidence of its role in fertility as it was exclusively enriched in fertility subgroup samples. In infertility subgroup samples the majority of the GO categories enriched were associated with molecular functions such as ion, cation and gated channel activity, which surprisingly were reported to be associated with infertility in males (Shukla et al. Citation2012). Furthermore, GO categories enriched in the high milk production sample were associated with biological processes such as metabolic and ion transport processes. The results corroborate the study conducted by Bionaz et al. (Citation2012) on bovine mammary transcriptome during the lactation cycle, which indicated increased overall metabolism, e.g. large increases in carbohydrate, lipid and metabolism of other secondary molecules (e.g. glycans), and phosphorylation during lactation in the mammary gland on examination of KEGG pathway categories and subcategories. No reported work was found on GO categories associated with milk production and fertility traits in cattle or buffalo using exome sequencing.
Conclusion
The present study demonstrates that sampling a small but genome-wide subset of the exome can be an effective approach for the discovery of thousands of putative SNVs. This investigation of the Jaffrabadi buffalo, a species without a reference genome, has yielded important candidate genes which may be targeted by researchers in future association studies involving water buffalo, unlike studies based on microarray and transcriptome analysis, in which prior references are required. This study also reveals that SNPs in the off-target region should not be ignored in data analysis, as today's researchers are also identifying important SNVs even in the non-coding region. In the present study, based on a targeted sequencing approach, candidate genes were identified which could be further validated by traditional methods and used for breeding programmes.
Authors’ contributions
MRU prepared the manuscript and performed the bioinformatics data analysis. ABP, VDB and TMS carried out cDNA capture and performed sequencing. PGK, SJJ and ABP performed sample collection. RBS, DNR and CGJ guided in drafting the manuscript. CGJ conceived the whole experiment and monitored the progress. All authors read and approved the final manuscript.
Supplemental data
Supplemental data for this article can be accessed at here.
Supplemental Tables
Download MS Word (22.8 KB)Acknowledgement
We are thankful to officials of the Government Slaughter House, Ahmedabad, India, for providing samples.
Funding
This study was supported by a grant from the Department of Biotechnology, Government of India.
Disclosure statement
The authors declare that they have no competing interests.
References
- Barbazuk WB., Bedell JA, Rabinowicz PD. 2005. Reduced representation sequencing: a success in maize and a promise for other plant genomes. Bioessays. 27:839–848. doi: 10.1002/bies.20262
- Bardley DG. 2012. Novel SNP Discovery in African Buffalo, Syncerus caffer, Using High-Throughput Sequencing.
- Bionaz M, Periasamy K, Rodriguez-Zas SL, Everts RE, Lewin HA, Hurley WL, Loor JJ. 2012. Old and new stories: revelations from functional analysis of the bovine mammary transcriptome during the lactation cycle. PloS one. 7(3):e33268. doi: 10.1371/journal.pone.0033268
- Bolormaa S, Hayes B, Savin K, Hawken R, Barendse W, Arthur P, Herd R, Goddard M. 2011. Genome-wide association studies for feedlot and growth traits in cattle. J Anim Sci. 89:1684–1697. doi: 10.2527/jas.2010-3079
- Böttcher T, von Mering M, Ebert S, Meyding-Lamadé U, Kuhnt U, Gerber J, Nau R. 2003. Differential regulation of Toll-like receptor mRNAs in experimental murine central nervous system infections. Neurosci Lett. 344:17–20. doi: 10.1016/S0304-3940(03)00404-X
- Cingolani P, Platts A, Wang le L, Coon M, Nguyen T, Wang L, Land SJ, Lu X, Ruden DM. 2012. A program for annotating and predicting the effects of single nucleotide polymorphisms, SnpEff: SNPs in the genome of Drosophila melanogaster strain w1118; iso-2; iso-3. Fly. 6:80–92. doi: 10.4161/fly.19695
- Cock PJ, Antao T, Chang JT, Chapman BA, Cox CJ, Dalke A, Friedberg I, Hamelryck T, Kauff F, Wilczynski B. 2009. Biopython: freely available Python tools for computational molecular biology and bioinformatics. Bioinformatics. 25:1422–1423. doi: 10.1093/bioinformatics/btp163
- Eck SH, Benet-Pages A, Flisikowski K, Meitinger T, Fries R, Strom TM. 2009. Whole genome sequencing of a single Bos taurus animal for single nucleotide polymorphism discovery. Genome Bio. 10(8):R82. doi: 10.1186/gb-2009-10-8-r82
- FAOSTAT (2010). Food and Agricultural Organization Statistical Database.
- Fu Y, Springer NM, Gerhardt DJ, Ying K, Yeh CT, Wu W, Swanson-Wagner R, D'Ascenzo M, Millard T, Freeberg L. 2010. Repeat subtraction-mediated sequence capture from a complex genome. Plant J. 62:898–909. doi: 10.1111/j.1365-313X.2010.04196.x
- Georges M. 2007. Mapping, fine mapping, and molecular dissection of quantitative trait loci in domestic animals. Annu Rev Genomics Hum Genet. 8:131–162. doi: 10.1146/annurev.genom.8.080706.092408
- GLDB (2014). Gujarat Livestock Development Board.
- Gnirke A, Melnikov A, Maguire J, Rogov P, LeProust EM, Brockman W, Fennell T, Giannoukos G, Fisher S, Russ C. 2009. Solution hybrid selection with ultra-long oligonucleotides for massively parallel targeted sequencing. Nature Biotechnol. 27:182–189. doi: 10.1038/nbt.1523
- Guo Y, Long J, He J, Li CI, Cai Q, Shu XO, Zheng W, Li C. 2012. Exome sequencing generates high quality data in non-target regions. BMC Genomics. 13(1):194. doi: 10.1186/1471-2164-13-194
- Huang da W, Sherman BT, Lempicki RA. 2009. Systematic and integrative analysis of large gene lists using DAVID bioinformatics resources. Nature Protocol. 4:44–57. doi: 10.1038/nprot.2008.211
- Jakhesara SJ, Ahir VB, Padiya KB, Koringa PG, Rank DN, Joshi CG. 2012. Tissue-specific temporal exome capture revealed muscle-specific genes and SNPs in Indian Buffalo (Bubalus bubalis). Genomics, Proteomics & Bioinformatics. 10:107–113. doi: 10.1016/j.gpb.2012.05.005
- Jiang L, Liu J, Sun D, Ma P, Ding X, Yu Y, Zhang Q. 2010. Genome wide association studies for milk production traits in Chinese Holstein population. PloS One. 5(10):e13661. doi: 10.1371/journal.pone.0013661
- Kawahara-Miki R, Tsuda K, Shiwa Y, Arai-Kichise Y, Matsumoto T, Kanesaki Y, Oda S, Ebihara S, Yajima S, Yoshikawa H, et al. 2011. Whole-genome resequencing shows numerous genes with nonsynonymous SNPs in the Japanese native cattle Kuchinoshima-Ushi. BMC Genomics. 12(1):103. doi: 10.1186/1471-2164-12-103
- le Roex N, Noyes H, Brass A, Bradley DG, Kemp SJ, Kay S, van Helden PD, Hoal EG. 2012. Novel SNP Discovery in African Buffalo, Syncerus caffer, using high-throughput Sequencing. PloS One. 7(11):e48792. doi: 10.1371/journal.pone.0048792
- Li H, Handsaker B, Wysoker A, Fennell T, Ruan J, Homer N, Marth G, Abecasis G, Durbin R. 2009. The sequence alignment/map format and SAMtools. Bioinformatics. 25:2078–2079. doi: 10.1093/bioinformatics/btp352
- Lovett M, Kere J, Hinton LM. 1991. Direct selection: a method for the isolation of cDNAs encoded by large genomic regions. Proc Natl Acad Sci. 88:9628–9632. doi: 10.1073/pnas.88.21.9628
- MacEachern S, McEwan J, Goddard M. 2009. Phylogenetic reconstruction and the identification of ancient polymorphism in the Bovini tribe (Bovidae, Bovinae). BMC Genomics. 10:177–88. doi: 10.1186/1471-2164-10-177
- Ng SB, Turner EH, Robertson PD, Flygare SD, Bigham AW, Lee C, Shaffer T, Wong M, Bhattacharjee A, Eichler EE. 2009. Targeted capture and massively parallel sequencing of 12 human exomes. Nature. 461:272–276. doi: 10.1038/nature08250
- Ollendorff V, Noguchi T, Delapeyriere O, Birnbaum D. 1994. The GARP gene encodes a new member of the family of leucine-rich repeat-containing proteins. Cell Growth Differ-Pub Am Assoc Cancer Res. 5:213–220.
- Roth J. 2004. “Bubalus bubalis” (Online), Animal Diversity Web.
- Schmieder R, Edwards R. 2011. Quality control and preprocessing of metagenomic datasets. Bioinformatics. 27:863–864. doi: 10.1093/bioinformatics/btr026
- Schuh-Huerta SM, Johnson NA, Rosen MP, Sternfeld B, Cedars MI, Pera RAR. 2012. Genetic variants and environmental factors associated with hormonal markers of ovarian reserve in Caucasian and African American women. Human Reprod. 27:594–608. doi: 10.1093/humrep/der391
- Shukla KK, Mahdi AA, Rajender S. 2012. Ion channels in sperm physiology and male fertility and infertility. J Andrology. 33:777–788. doi: 10.2164/jandrol.111.015552
- Sugimoto M, Sasaki S, Watanabe T, Nishimura S, Ideta A, Yamazaki M, Matsuda K, Yuzaki M, Sakimura K, Aoyagi K, et al. 2010. Ionotropic glutamate receptor AMPA 1 is associated with ovulation rate. PloS One. 5(11):e13817. doi: 10.1371/journal.pone.0013817
- Utsunomiya YT, Do Carmo AS, Carvalheiro R, Neves HH, Matos MC, Zavarez LB, O'Brien AMP, Sölkner J, McEwan JC, Cole JB, et al. 2013. Genome-wide association study for birth weight in Nellore cattle points to previously described orthologous genes affecting human and bovine height. BMC Genetics. 14(1):52. doi: 10.1186/1471-2156-14-52
- Womack JE. 2005. Advances in livestock genomics: opening the barn door. Genome Res. 15:1699–1705. doi: 10.1101/gr.3809105