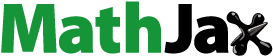
Abstract
This article analyzes determinants of farmers’ adoption decisions for agroforestry practices and the extent of adoption in rural Rwanda. The study also investigates the key constraints hindering smallholder farmers to adopt agroforestry. The study uses the cross-sectional data from a sample of 615 farmers and Cragg’s double-hurdle model is used for the empirical estimation. The findings show that farmers’ land ownership, cooperative membership, farming experience, market orientation, credit access, and distance to the market mainly determine adoption of agroforestry. Policies that enhance adoption of agroforestry should be consider the formation of farmers’ cooperatives and provision of door-to-door education to uneducated farmers. In addition, strategies for supporting liquidity-constrained households to get continuous and increased access to credit should be put forward. Agricultural and resources development policies should direct efforts toward increased access to institutional support services such as better extension to farmers through cooperatives to promote the adoption of agroforestry.
1. Introduction
The motivation for this study is the low level of agricultural productivity and loss of soil fertility in Rwanda. In general, the economy of Rwanda depends heavily on agricultural production, a sector that plays a significant role in food security, employment, poverty reduction, and foreign exchange earnings. The contribution of agriculture to GDP was about 26.3% in 2020 and employed 62.3% of the total labor force in 2019 (World Bank Citation2021). However, compared to other regions of the World, agricultural productivity in Rwanda and most parts of sub-Saharan Africa (SSA) remains low over the past decades (Ngango and Hong Citation2021a). The major factors that cause this low agricultural productivity in SSA countries include land degradation, loss of soil fertility and soil moisture, poor farm management practices, and inadequate use of improved seeds and fertilizers (Beyene and Kassie Citation2015; Khataza et al. Citation2018). In particular, land degradation and low soil fertility are serious constraints faced by smallholder farmers in SSA, posing a major threat to crop productivity, food security, and rural livelihoods (Wawire et al. Citation2021). Previous studies noted that in the face of the growing population, over-cultivation with unsustainable farming practices, overgrazing, deforestation, and clearing woodland resources are the major factors causing land degradation (Coulibaly et al. Citation2017; Jha et al. Citation2021; Wawire et al. Citation2021). Moreover, Wawire et al. (Citation2021) documented that low soil fertility problems are more widespread across SSA, such 75% of the arable land is considered severely depleted due to poor soil management practices on the fragile soils, and thus require costly investments to ensure sustained productivity.
Among the above-mentioned factors, deforestation appears as the most challenging issue to be explored. This is because deforestation accounts for about 60% of global land degradation and approximately 30% of all man-made carbon emissions (Dimobe et al. Citation2015). In Rwanda, the mismanagement of forested lands has resulted in various environmental problems such as soil erosion, frequent floods, and landslides particularly, in the northern and western provinces of Rwanda. It also resulted in dry spells in the eastern province of Rwanda and thus causing soil fertility depletion.
To build the resilience of farmers toward the effects of land degradation for sustainable use of the ecosystem, there is a need for appropriate soil fertility management technologies such as agroforestry. Recently, the development policy agenda in most developing countries has given much attention to agroforestry (i.e. integrating trees on the farm) as a strategy for improving soil fertility. Agroforestry is the practice and science of the interface and interactions between agriculture and forestry, involving farmers, livestock, trees, and forests at multiple scales (Coulibaly et al. Citation2017). Agroforestry practices are widely viewed as potential strategies for improving soil fertility through soil erosion control, enhancement of soil physical properties, increased nitrogen, and nutrient cycling. Agroforestry farming practices were found efficient in the adaptation to climatic variability as well as in improving low crop productivity and ensuring food security (Coulibaly et al. Citation2017). Particularly, agroforestry farming practices such as fertilizer trees can replenish the fertility of soils by transferring atmospheric nitrogen in the soil through their roots and leaf litter (Ajayi et al. Citation2007).
However, the adoption of agroforestry practices continues to be very low in Rwanda although the potentials of agroforestry are evident as highlighted above. A scholarly question that requires further interrogation refers to why this low adoption. Evidence from previous adoption studies suggests that farmers’ adoption of farming practices varies based on a range of socioeconomic, biophysical, and institutional factors. The specific objectives are (i) to examine the key factors determining farmers’ adoption decisions for agroforestry practices and the intensity of adoption of agroforestry in rural Rwanda, and (ii) to investigate the key constraints hindering smallholder farmers in rural Rwanda to adopt the agroforestry.
2. Rationale and literature review
The rationale for this research is to use empirical approaches to model the adoption decisions and the intensity of adoption of agroforestry farming practices in Rwanda. Then, the study suggests important policy recommendations that can play a significant role in improving the adoption of agroforestry farming practices at a large scale in Rwanda. Consequently, this research builds interaction between agroforestry and other national policy initiatives, i.e. agricultural transformation, food security, environmental sustainability, rural development, and poverty reduction.
2.1. Review of related literature
The theories dealing with technology adoption in the agriculture were reviewed. In general, adoption decisions for a new agricultural technology are influenced by the perceived benefits. Knowing that positive benefits are needed to stimulate technology adoption has led to the theory of induced technology (Chavas and Nauges Citation2020). The basic idea behind the theory of “induced technology” was first used with reference to Hicks (Citation1932). Though, the analysis of agricultural growth by Boserup (Citation1965) was possibly the major influence on the development of induced technology theory. Boserup (Citation1965) argued that a growing population and an increasing demand for agricultural products which result in land pressures largely induce the adoption of agricultural technologies (Chavas and Nauges Citation2020).
The existing literature on the adoption of agroforestry has been extensively reviewed. In particular, numerous previous studies indicated that the adoption of agroforestry practices is mostly attributed to biophysical, plot-level, household, socioeconomic, institutional, and locational characteristics. For example, Jha et al. (Citation2021) argued that the uptake of agroforestry technologies in Tanzania was influenced by a set of household and socio-economic factors as well as farmer perceptions and intentions. Pattanayak et al. (Citation2003) and Edwards-Jones (Citation2006) explored the effect of environmental perspectives and psychological dimensions on farmers’ decisions to adopt agroforestry practices. Coulibaly et al. (Citation2017) investigated the effect of farm/plot-level characteristics and shocks related to climate change on the adoption of agroforestry technologies.
Many of the previous studies of agroforestry in Africa focused on the analysis of the role of agroforestry (as water and soil fertility management) to control soil erosion, carbon offset, and other environmental service markets (König Citation1992; Roose and Ndayizigiye Citation1997; Stainback et al. Citation2012). Other studies in Rwanda investigated the benefits and challenges of agroforestry practices, farmers’ preferences, and perspectives on agroforestry technologies (Ndayambaje and Mohren Citation2011; Bucagu et al. Citation2013; Kiyani et al. Citation2017). Indeed, few of them have attempted to examine the factors determining farmers’ adoption decisions for agroforestry practices and the intensity of adoption of agroforestry. Therefore, this study positions itself in this respect to fill the research gap. This study is also aligned to the Sustainable Development Goals (SDGs), particularly the fifteenth SDG which is about “Protect, restore and promote sustainable use of terrestrial ecosystems, sustainably manage forests, combat desertification, and halt and reverse land degradation and halt biodiversity loss”.
According to Amare et al. (Citation2012), the most common theory that tends to explain farmers’ decision to adopt agricultural technologies in developing countries is the theory of utility maximization. It is assumed that farmers adopt a particular technology only if its use maximizes their utility in terms of net returns (Ngango and Hong Citation2021b). The assumption of random utility theory suggests that rational individuals choose the options that yield them the highest utility given that there is a budget constraint (Owusu et al. Citation2016).
2.2. Overview of Rwandan agriculture and agroforestry farming system
Rwandan agriculture is an important economic sector that contributes about 25% to the country’s gross domestic product and approximately 63% of the workforce is employed in the agriculture sector (World Bank Citation2019). Indeed, agricultural development has recently received considerable devotion as a significant approach to eradicating poverty and ensuring food security and rural development (MINAGRI Citation2020). However, Rwanda’s agricultural production system remains subsistence farming and largely depends on rain-fed farming systems.
Rwanda has favorable altitude, climate, and rainfall conditions for agriculture. Though, the hilly topography with steep slopes has been conducive to depleting soils through rapid runoff of surface water and soil erosion. Rwanda has the highest population density as compared to the rest of the African countries (Ngango and Hong Citation2021c). Furthermore, the country is characterized by a high population growth with tiny land holdings that are roughly 0.75 hectares per household. In some parts of Rwanda, there is a frequency of landlessness (Ansoms et al. Citation2018). The problem of smallholder farming, low crop productivity, and land fragmentation has attracted the attention of policy-makers, research institutions, and non-government organizations.
In particular, approximately 80% of the Rwandan population live in rural areas and around 36% of the households own an average farm size of roughly 0.11 ha, which is 6% of the farmlands. Also, 30% of the households own an average farm size of roughly 0.6 ha, that is 24% of the farmlands, whereas 24% of households own an average farm size of about 2 ha (i.e. 70% of the farmlands). Besides, about 10% of the households are landless. The national average holding of 0.76 ha is generally divided over 4–5 small plots, often in multiple locations (Ngango and Hong Citation2021c).
Crop productivity in Rwanda as well as in many sub-Saharan African countries is mostly constrained by various challenges such as population pressure, soil degradation, utilization of poor farming practices, and the inadequate application of improved agricultural inputs, i.e. hybrid seeds and chemical fertilizers (Liliane et al. Citation2020). According to Coulibaly et al. (Citation2017), population pressure, soil erosion, land degradation, and declining soil fertility in terms of nitrogen, phosphorus, and potassium are the key constraints contributing to low crop productivity, hunger, and malnutrition.
The uptake of sustainable land management farming systems such as crop rotation and integration of trees and crops and/or animals is at a limited level in most parts of Rwanda (Kiyani et al. Citation2017). The significant efforts to promote the widespread adoption of agroforestry farming systems have been implemented by the World Agroforestry (ICRAF) in partnership with Rwanda Agriculture and Animal Resources Development Board (RAB) and the Ministry of Agriculture and Animal Resources (MINAGRI). These efforts were made with the main purpose of addressing the issue of soil erosion and restoring soil fertility on farmlands, as well as improving agricultural productivity and food security. Particularly, several dominant tree species such as Alnus acuminata, Persea americana, Calliandra calothyrsus, Erythrina abyssinica, Gliricidia sepium, Grevillea robusta, Leucaena leucocephala, Mangifera indica, Markhamia lutea, and Acacia polyacantha have been introduced and disseminated among farmers in both the Semi-arid and Sub-humid regions (MINAGRI Citation2020).
3. Methodology
3.1. Study location
Due to the limited resources to conduct a nationwide survey, this study was conducted in the Huye, Gisagara, and Nyaruguru districts of the southern province of Rwanda. The topography of this agroecological zone is characterized by fragmented, hilly terrain with steep slopes and deep-water valleys (Csorba et al. Citation2019). Annual rainfall varies from 1300 to 1450 mm with a mean annual temperature of 20 °C (Mukuralinda et al. Citation2010; Kiyani et al. Citation2017). The rainfall is distributed over two cropping seasons, i.e. Season A starts from September to January, and Season B starts from February to May (Mukuralinda et al. Citation2010). In particular, Huye, Gisagara, and Nyaruguru districts are predominantly rural and residents depend on subsistence agriculture for their livelihood. The agroforestry system of farming has been promoted in these districts but not all farmers have adopted it (Kiyani et al. Citation2017). Statistics from the fifth Rwanda population and housing census conducted by the National Institute of Statistics of Rwanda (NISR) in 2022 indicate that the total population of the districts of Huye, Gisagara, and Nyaruguru is about 381,900; 397,051; and 318,126, respectively (NISR Citation2023). Huye, Gisagara, and Nyaruguru districts have total households of about 96,037; 101,145; and 73,805, respectively (NISR Citation2023). In particular, 78% of the total households in Huye district are mainly farmers while in Gisagara and Nyaruguru districts, the proportion of agricultural households is roughly 86.4% and 88.9%, respectively (NISR Citation2023).
3.2. Survey design and data collection
The target population for this research project consists of farmers who have adopted agroforestry practices and those who have not yet adopted agroforestry (i.e. still practicing conventional farming) in Rwanda. However, due to the limited resources to conduct a nationwide survey, data for this research project came from a household survey conducted in the Huye, Gisagara, and Nyaruguru districts of the southern province of Rwanda. A multi-stage sampling technique was used to select respondents. In the first stage, three districts (i.e. Huye, Gisagara, and Nyaruguru) were purposively selected because they are among the regions of Rwanda with a dominance of productive agroforestry practices. The second stage involved the selection of villages in each district. This was done with the help of sector agronomists and social economic development officers (SEDOs) at the cell level, to identify potential villages with high dominance of agroforestry practices. Approximately 24 villages were selected in each district giving a total of 72 villages.
The third stage involved a random selection of household farmers (including both adopters and non-adopters of agroforestry) in each village. About 8 households were randomly selected from each village, giving a total sample size of 615 households. Prior to conducting the formal survey, the questionnaire was pre-tested to a few numbers of households in the study area, to inspect the adequacy of the survey instrument. Before the survey, enumerators who speak the local language (Kinyarwanda) were trained to understand the questionnaire and the data collection using a digitalized questionnaire in Kobo toolbox.
3.3. Analytical framework
3.3.1. Specification of Cragg’s double-hurdle model
The adoption of agricultural technologies in most developing countries tends to be constrained by limited financial resources, market imperfections, poor rural infrastructures, and a lack of information (Zeweld et al. Citation2015). Despite these constraints, Asfaw et al. (Citation2012) argued that farmers are supposed to adopt a given farm technology only if its use maximizes its utility in terms of net returns. Thus, following Asfaw et al. (Citation2012), we apply the random utility framework to model the adoption of agroforestry. We assume that farmers are risk neutral and opt for the agricultural technology that maximizes their utility function subject to input costs and other constraints. More formally, let us consider as the utility that a farmer
derives from the adoption of agroforestry and
to be the utility from non-adoption. Under such assumptions, the farmer can adopt a farming practice, if the utility derived from the adoption is greater than the utility of non-adoption, that is,
(Abdulai and Huffman Citation2014).
Since households choose whether to adopt agroforestry or not to adopt it (a dichotomous choice) and, if so, to what extent they adopt (i.e. a continuous variable – the intensity of adoption), a double-hurdle model is suitable. This model was proposed by Cragg (Citation1971) and it is considered a generalized and improved form of the Tobit regression model in estimating adoption relationships by expounding the extent or rate of adopting a technology (Adewale et al. Citation2020). The double-hurdle model is appropriate because it allows two autonomous scenarios into the analytical framework. According to Weatherspoon et al. (Citation2021), this model has experienced considerable success in agricultural applications where households faced two hurdles in decision-making processes. The first hurdle is the decision made on whether to adopt or not a farming practice; while the second hurdle has to do with the rate of adopting a farming practice that can be in the form of an index or continuous variable. Again, the model distinguishes between the factors determining the adoption of technology, and the rate or intensity of adopting or utilizing the technology as two separate stages (Adewale et al. Citation2020; Weatherspoon et al. Citation2021; Zeleke et al. Citation2021).
In Cragg’s double-hurdle model, the first hurdle is the decision of whether or not farmers have adopted agroforestry. The second hurdle is the extent of usage once the adoption decision has been made, i.e. the amount of land under cultivation that the farmer dedicated to agroforestry farming practices in square meters (m2). In estimating the model, the first hurdle which represents the adoption decision employs the binary regression model (probit) and it is specified as follows:
(1)
(1)
where
is a binary latent variable that takes the value of 1 for farmers who adopt the agroforestry farming practices, and zero otherwise.
denotes a vector of household characteristics and agroforestry attributes;
is a vector of parameters to be estimated; and
is the error term, with
The second hurdle which refers to the intensity of adoption for agroforestry farming practices, is given in a Tobit model and it is specified as follows:
(2)
(2)
where
is the observed response for the acreage allocated to agroforestry farming practices,
is a vector of the observed explanatory variables and
is a vector of parameters to be estimated.
is associated with
which is the error term for the Tobit model.
According to Martínez-Espiñeira (Citation2006), if the two decisions of whether or not to adopt agroforestry and how much farmland to allocate to agroforestry farming practices are independently made by individual farmers, the error terms are assumed to be independently and normally distributed as follows: and
this implied that there is no correlation between the two error terms.
Allowing for heteroscedasticity and a non-normal error structure across equations, the log-likelihood function for the double-hurdle model can be written as follows:
(3)
(3)
Hence, in this study, we employ Cragg’s double-hurdle model to examine the decision to adopt or not and the extent of adoption as a function of the same set of independent variables. Those explanatory variables include age, gender, marital status, education level, household size, land ownership, cooperative membership, farming experience, market orientation, credit access, distance to market, and location fixed effects.
4. Results and discussion
4.1. Descriptive analysis
The summary statistics of variables presented in indicate that about 41% of farmers in the study area have adopted agroforestry practices while 49% are non-adopters. In addition, the average land allotted for agroforestry is approximately 2270.5 m2. The average age of farmers in the sample area is roughly 47 years, and about 31% have formal education. also shows that on average, the household size is approximately 5 persons and about 57% privately own the land. The average farming experience is roughly 24 years and the access to credit is estimated to be 26% in the sample area.
Table 1. Description of variables and summary statistics.
presents the mean differences in observed characteristics between adopters and non-adopters of agroforestry in terms of socioeconomic characteristics. The results indicate that the statistical t-tests revealed significant differences in the observed characteristics between adopters and non-adopters for variables such as age, marital status, household size, land ownership, cooperative membership, farming experience, market orientation, credit access, and the distance to market.
Table 2. Differences in household characteristics by adoption category (sample mean).
The results in further indicate that the major constraints that hinder farmers to adopt agroforestry practices are the lack of extension services, high cost of implementation, lack of seedlings, small pieces of land, insecurity of land tenure, and termites.
4.2. Econometric analysis
The results for the two-step double-hurdle model using the same set of explanatory variables are reported in . The Wald chi-square of 32.72 estimated through the maximum likelihood and significance at 1% level indicate that the model fits the variable properly. The sigma is significant at a 1% level, indicating that heteroskedasticity was found and correct in the model.
Table 3. Results of the double hurdle model on the adoption of agroforestry in rural Rwanda.
4.2.1. Determinants of the likelihood of adoption decisions for agroforestry
The first-hurdle estimates of determinants of the adoption of agroforestry in rural Rwanda. The parameter estimates for the first hurdle indicate that the variables such as land ownership, cooperative membership, farming experience, market orientation, credit access, and distance to market significantly influence the adoption decisions for agroforestry. In particular, land ownership, cooperative membership, farming experience, market orientation, and credit access have a positive influence on the adoption of agroforestry. While on the other hand, the variable distance to the market negatively influences the adoption of agroforestry.
As expected, land ownership was found to have affected adoption decisions positively at the 1% significance level. The marginal effect indicates that land tenure increases the likelihood of adoption by 37 percentage points. This finding is consistent with the results of Zerihun et al. (Citation2014) who found that private ownership of land was associated with an increase in the adoption of agroforestry practices in Eastern Cape Province, South Africa. Another interesting significant (p < 0.01) variable is access to credit. The marginal effect for this variable shows that households who had more access to credit were more likely to adopt agroforestry practices as compared to farmers who had low access to credit. A plausible explanation for this finding is that credit access assists rural households to accumulate enough capital to invest in expensive technologies (Abdulai and Huffman Citation2014). This result is supported by previous findings of Zerihun et al. (Citation2014) and Beyene et al. (Citation2019), who acknowledged the positive effect of credit access on the adoption of agroforestry. The results reported in also indicate that cooperative membership has a significant (p < 0.1) and positive effect on the adoption of agroforestry farming practices. A similar result was also reported by Jha et al. (Citation2021) in their study on the factors influencing the adoption of agroforestry by smallholder farmer households in Tanzania.
Farming experience was also found to have a positive and significant (p < 0.1) influence on farmers’ adoption of agroforestry. This result is consistent with the findings of Coulibaly et al. (Citation2017) who revealed that farmer’s experience influences their adoption of agroforestry practices in Malawi. Furthermore, the marginal effect for market orientation which is a proxy of the amount of harvest sold to the market indicates that market orientation enhances the likelihood of adoption of agroforestry by 1 percentage point. On the other hand, the marginal effect for the distance to market variable shows that farmers who are far from the market are less likely to adopt agroforestry as compared to farmers close to the market.
4.2.2. Determinants of the intensity of adoption of agroforestry
The second hurdle estimates the intensity or extent of the adoption of agroforestry in rural Rwanda, conditional on the decision to adopt. The results from the truncated regression model which are reported in show that the level of education, cooperative membership, market orientation, credit access, and distance to market were found to significantly determine the intensity of adoption of agroforestry. Particularly, the level of education, cooperative membership, and market orientation variables positively and significantly influence the intensity of adoption of agroforestry. While credit access and distance to the market negatively and significantly determine the extent of adoption of agroforestry. shows that although the level of education has no significant effect on the likelihood of adoption of agroforestry, the education level significantly increases the rate of adoption of agroforestry. This result corroborates the findings of Adewale et al. (Citation2020) who found that farmers’ education positively influences the rate of adoption of agricultural technologies in Nigeria.
As a priori expectation, the extent of adoption of agroforestry is positively and significantly correlated with cooperative membership. Besides, the results reported in indicate that market orientation significantly increases the extent of adoption of agroforestry. However, access to credit is associated with a decrease in the extent of adoption of agroforestry even though this variable has a positive effect on the adoption decision. This implies that access to credit increases the likelihood of adopting the agroforestry but if farmers adopt, they tend to have a low rate of adoption than farmers without access to credit. This does not follow a priori expectation and the probable reason is that the credit access might assist farmers to make a decision whether to adopt a farming practice or not but the credit may be used for other agricultural investments (Kassa et al. Citation2021). Moreover, the distance to the market is negatively associated with the intensity of the adoption of agroforestry.
5. Conclusion
The present study has examined the determinants of the adoption of agroforestry farming practices and the intensity of the adoption of agroforestry in rural Rwanda. The study also investigated the key constraints hindering smallholder farmers in rural Rwanda to adopt agroforestry. The results reveal that adoption decisions for agroforestry are significantly influenced by factors such as land ownership, cooperative membership, farming experience, market orientation, and credit access have a positive influence on the adoption of agroforestry. While the variable distance to the market negatively and significantly influences the adoption of agroforestry. These findings imply that government interventions and stakeholder strategies should focus on sensitizing farmers to form and join agricultural cooperatives. Moreover, strategies for supporting liquidity-constrained households to get easy access to credit should be put forward, so that they can uptake agroforestry. The marketing of agricultural produce as well as the marketing facilities should be efficient to allow an accelerated uptake of agroforestry. In addition, agricultural markets should be built in the proximity of rural villages to reduce the higher distance to the market that prevents farmers to uptake agroforestry.
The results also reveal that the intensity of adoption of agroforestry is significantly and positively determined by the level of education, cooperative membership, and market orientation variables. The credit access and distance to the market negatively and significantly determine the extent of adoption of agroforestry. These results suggest that the Government of Rwanda through the Ministry of Agriculture and Animal Resources and development partners should encourage agricultural cooperatives to accelerate the rate of agroforestry adoption. Additionally, continuous and increased support from government and partners for providing door-to-door education to uneducated farmers is needed. Lastly, the study revealed that the major constraints that hinder farmers to adopt agroforestry farming practices are the lack of extension services, high cost of implementation, lack of seedlings, small pieces of land, insecurity of land tenure, and termites. Therefore, increased access to institutional support services such as better extension to farmers through cooperatives is recommended to promote the adoption of agroforestry. Moreover, the study calls for continuous and increased access to credit available to rural farmers and financing support from the government, development agencies, and private sector partners.
Acknowledgements
The authors gratefully acknowledge the financial support of the University of Rwanda through UR – Sweden Programme. We would also like to thank the enumerators and the data manager for their valuable cooperation during data collection.
Disclosure statement
No potential conflict of interest was reported by the author(s).
References
- Abdulai A, Huffman W. 2014. The adoption and impact of soil and water conservation technology: an endogenous switching regression application. Land Economics. 90(1):26–43. doi: 10.3368/le.90.1.26.
- Adewale IO, Ibidun Comfort A, Amos Igbekele A, Taiwo Timothy A. 2020. Adoption of improved technologies and profitability of the catfish processors in Ondo State, Nigeria: a Cragg’s double-hurdle model approach. Sci Afr. 10:e00576. doi: 10.1016/j.sciaf.2020.e00576.
- Ajayi OC, Akinnifesi FK, Sileshi G, Chakeredza S. 2007. Adoption of renewable soil fertility replenishment technologies in the southern African region: lessons learnt and the way forward. Natural Resources Forum. 31(4):306–317. doi: 10.1111/j.1477-8947.2007.00163.x.
- Amare M, Asfaw S, Shiferaw B. 2012. Welfare impacts of maize–pigeonpea intensification in Tanzania. Agricultural Economics. 43(1):27–43. doi: 10.1111/j.1574-0862.2011.00563.x.
- Ansoms A, Cioffo G, Dawson N, Desiere S, Huggins C, Leegwater M, Murison J, Nyenyezi Bisoka A, Treidl J, Van Damme J. 2018. The Rwandan agrarian and land sector modernisation: confronting macro performance with lived experiences on the ground. Review of African Political Economy. 45(157):408–431. doi: 10.1080/03056244.2018.1497590.
- Asfaw S, Shiferaw B, Simtowe F, Lipper L. 2012. Impact of modern agricultural technologies on smallholder welfare: evidence from Tanzania and Ethiopia. Food Policy. 37(3):283–295. doi: 10.1016/j.foodpol.2012.02.013.
- Beyene AD, Kassie M. 2015. Speed of adoption of improved maize varieties in Tanzania: an application of duration analysis. Technol Forecasting Social Change. 96:298–307. doi: 10.1016/j.techfore.2015.04.007.
- Beyene AD, Mekonnen A, Randall B, Deribe R. 2019. Household level determinants of agroforestry practices adoption in rural Ethiopia. Forest, Tree Livelihood. 28(3):194–213. doi: 10.1080/14728028.2019.1620137.
- Boserup E. 1965. The conditions of agricultural growth: the economics of agrarian change under population pressure. London: Allen and Unwin.
- Bucagu C, Vanlauwe B, Van Wijk MT, Giller KE. 2013. Assessing farmers’ interest in agroforestry in two contrasting agro-ecological zones of Rwanda. Agroforest Syst. 87(1):141–158. doi: 10.1007/s10457-012-9531-7.
- Chavas J-P, Nauges C. 2020. Uncertainty, learning, and technology adoption in agriculture. Applied Econ Perspect Pol. 42(1):42–53. doi: 10.1002/aepp.13003.
- Coulibaly JY, Chiputwa B, Nakelse T, Kundhlande G. 2017. Adoption of agroforestry and the impact on household food security among farmers in Malawi. Agric Syst. 155:52–69. doi: 10.1016/j.agsy.2017.03.017.
- Cragg JG. 1971. Some statistical models for limited dependent variables with application to the demand for durable goods. Econometrica. 39(5):829–844. doi: 10.2307/1909582.
- Csorba A, Szegi T, Fodor H, Bukombe B, Uwiragiye Y, Naramabuye FX, Michéli E. 2019. Characterization of rice agriculture in the Southern Province of Rwanda by means of microwave remote sensing. Phys Chem Earth, Parts A/B/C. 112:58–65. doi: 10.1016/j.pce.2019.02.002.
- Dimobe K, Ouédraogo A, Soma S, Goetze D, Porembski S, Thiombiano A. 2015. Identification of driving factors of land degradation and deforestation in the Wildlife Reserve of Bontioli (Burkina Faso, West Africa). Global Ecol Conserv. 4:559–571. doi: 10.1016/j.gecco.2015.10.006.
- Edwards-Jones G. 2006. Modelling farmer decision-making: concepts, progress and challenges. Anim Sci. 82(6):783–790. doi: 10.1017/ASC2006112.
- Hicks JR. 1932. The theory of wages. London-Melbourne-Toronto: Macmillan, St Martin’s Press New York.
- Jha S, Kaechele H, Sieber S. 2021. Factors influencing the adoption of agroforestry by smallholder farmer households in Tanzania: case studies from Morogoro and Dodoma. Land Use Policy. 103:105308. doi: 10.1016/j.landusepol.2021.105308.
- Kassa Y, Giziew A, Ayalew D. 2021. Determinants of adoption and intensity of improved faba bean cultivars in the central highlands of Ethiopia: a double-hurdle approach. CABI Agric Biosci. 2(1):1–12. doi: 10.1186/s43170-021-00045-8.
- Khataza RRB, Doole GJ, Kragt ME, Hailu A. 2018. Information acquisition, learning and the adoption of conservation agriculture in Malawi: a discrete-time duration analysis. Technol Forecast Soc Change. 132:299–307. doi: 10.1016/j.techfore.2018.02.015.
- Kiyani P, Andoh J, Lee Y, Lee DK. 2017. Benefits and challenges of agroforestry adoption: a case of Musebeya sector, Nyamagabe District in southern province of Rwanda. Forest Science and Technology. 13(4):174–180. doi: 10.1080/21580103.2017.1392367.
- König D. 1992. The potential of agroforestry methods for erosion control in Rwanda. Soil Technology. 5(2):167–176. doi: 10.1016/0933-3630(92)90017-U.
- Liliane M, Ezekiel N, Gathuru G. 2020. Socio-economic and institutional factors affecting smallholders farmers to adopt agroforestry practices in southern province of Rwanda. Int J Agric Sc Food Technol. 6(1):068–074. doi: 10.17352/2455-815X.000057.
- Martínez-Espiñeira R. 2006. A Box-Cox double-hurdle model of wildlife valuation: the citizen’s perspective. Ecol Econ. 58(1):192–208. doi: 10.1016/j.ecolecon.2005.07.006.
- MINAGRI. 2020. Ministry of Agriculture and Animal Resources (MINAGRI) annual report 2019–2020, Kigali, Rwanda.
- Mukuralinda A, Tenywa JS, Verchot L, Obua J, Nabahungu NL, Chianu JN. 2010. Phosphorus uptake and maize response to organic and inorganic fertilizer inputs in Rubona, Southern Province of Rwanda. Agroforest Syst. 80(2):211–221. doi: 10.1007/s10457-010-9324-9.
- Ndayambaje JD, Mohren GMJ. 2011. Fuelwood demand and supply in Rwanda and the role of agroforestry. Agroforest Syst. 83(3):303–320. doi: 10.1007/s10457-011-9391-6.
- Ngango J, Hong S-J. 2021a. Speed of adoption of intensive agricultural practices in Rwanda: a duration analysis. Agrekon. 60(1):43–56. doi: 10.1080/03031853.2021.1883448.
- Ngango J, Hong S. 2021b. Adoption of small-scale irrigation technologies and its impact on land productivity: evidence from Rwanda. J Integrat Agric. 20(8):2302–2312. doi: 10.1016/s2095-3119(20)63417-7.
- Ngango J, Hong S. 2021c. Improving farm productivity through the reduction of managerial and technology gaps among farmers in Rwanda. Agric Food Secur. 10(1):11. doi: 10.1186/s40066-020-00284-4.
- NISR. 2023. The Fifth Rwanda population and housing census, main indicators report, by the National Institute of Statistics of Rwanda (NISR), Kigali, Rwanda.
- Owusu CR, Burton MP, Gibson FL, Hailu A. 2016. Choice of rice production practices in Ghana: a comparison of willingness to pay and preference space estimates. J Agric Econ. 67(3):799–819. doi: 10.1111/1477-9552.12180.
- Pattanayak SK, Evan Mercer D, Sills E, Yang J-C. 2003. Taking stock of agroforestry adoption studies. Agroforest Syst. 57(3):173–186. doi: 10.1023/A:1024809108210.
- Roose E, Ndayizigiye F. 1997. Agroforestry, water and soil fertility management to fight erosion in tropical mountains of Rwanda. Soil Technology. 11(1):109–119. doi: 10.1016/S0933-3630(96)00119-5.
- Stainback GA, Masozera M, Mukuralinda A, Dwivedi P. 2012. Smallholder agroforestry in Rwanda: A SWOT-AHP analysis. Small Scale Forest. 11(3):285–300. doi: 10.1007/s11842-011-9184-9.
- Wawire AW, Csorba Á, Tóth JA, Michéli E, Szalai M, Mutuma E, Kovács E. 2021. Soil fertility management among smallholder farmers in Mount Kenya East region. Heliyon. 7(3):e06488. doi: 10.1016/j.heliyon.2021.e06488.
- Weatherspoon DD, Miller SR, Niyitanga F, Weatherspoon LJ, Oehmke JF. 2021. Rwanda’s commercialization of smallholder agriculture: implications for rural food production and household food choices. J Agric Food Indust Organ. 19(1):51–62. doi: 10.1515/jafio-2021-0011.
- World Bank. 2019. World development indicators. http://databank.worldbank.org/data/reports.aspx?source=world-development-indicators.
- World Bank. 2021. World development indicators. https://databank.worldbank.org/source/world-development-indicators.
- Zeleke BD, Geleto AK, Komicha HH, Asefa S. 2021. Determinants of adopting improved bread wheat varieties in Arsi Highland, Oromia Region, Ethiopia: A double-hurdle approach. Cogent Economics Finance. 9(1):1932040. doi: 10.1080/23322039.2021.1932040.
- Zerihun MF, Muchie M, Worku Z. 2014. Determinants of agroforestry technology adoption in Eastern Cape Province, South Africa. Development Studies Research. 1(1):382–394. doi: 10.1080/21665095.2014.977454.
- Zeweld W, Huylenbroeck GV, Hidgot A, Chandrakanth MG, Speelman S. 2015. Adoption of Small-scale irrigation and its livelihood impacts in Northern Ethiopia. Irrig and Drain. 64(5):655–668. doi: 10.1002/ird.1938.