ABSTRACT
Several scholars and policy-makers have claimed that Europe, and Western Europe in particular, has managed to ‘decouple’ economic growth from material use. We identify and address one major limitation in the existing literature – failure to take the endogeneity of economic growth into account. Based on a panel data-set of 32 European countries from 2000 to 2014, we estimate the causal impact of gross domestic product (GDP) on domestic material consumption (DMC) applying an instrumental variable approach. We use the number of storm occurrences as an instrument for GDP, which we show is both relevant and valid. Our results provide new evidence that increasing the GDP growth rate causes the DMC growth rate to increase for Western Europe, whereas the effect is insignificant for the Eastern European economies and Europe as a whole. As our results partly question current wisdom on the achievements of ‘decoupling’, especially among European policy-makers, we offer two explanations that are consistent with these results.
1. Introduction
There have been extensive discussions among scholars and policy-makers on how economic growth can be achieved while staying within the planetary boundaries (e.g. Arrow et al. Citation1995; Rockström et al. Citation2009). This has triggered a large literature investigating the effects of economic growth on various types of environmental pressures, in particular carbon dioxide (CO2) emissions (Selden and Song Citation1994; Schmalensee, Stoker, and Judson Citation1998; Galeotti, Lanza, and Pauli Citation2006; Vollebergh, Melenberg, and Dijkgraaf Citation2009; Wagner Citation2015).
Interdisciplinary scholars have argued that CO2 emissions and other single indicators of environmental pressures often used in economic studies fail to comprehensively capture (1) the variety of pressures arising at different stages of contemporary supply chains and (2) pressures that occur with a delay. These scholars have proposed material flow and footprint indicators as a more comprehensive measure for environmental pressures (Alfsen and Sæbø Citation1993; Bringezu, Schütz, and Moll Citation2003; Ibenholt Citation2003; van der Voet, van Oers, and Moll Citation2005b; Hertwich Citation2010; Bleischwitz Citation2010; Hoang and Alauddin Citation2012; Bouwmeester and Oosterhaven Citation2013; Wiedmann et al. Citation2006; Teixidó-Figueras and Duro Citation2015).Footnote1 The advantage of considering materials as a proxy for environmental pressures is that they reflect apparent and not final consumption (EC Citation2015a), i.e. the actual use of materials may take place at a later point in time than indicated by the material indicators. Policy-makers in Europe and around the world have recently made the reduction of material use a central policy objective, with the explicit aim to reduce environmental pressures associated with it (EC Citation2011; G7 Citation2015; UN Citation2015; EC Citation2015b; OECD Citation2016).
The empirical literature on the relationship between economic growth, measured by gross domestic product (GDP), and material use predominantly find a positive correlation between the two variables (e.g. Bringezu et al. Citation2004; van der Voet, van Oers, and Moll Citation2005b; Wiedmann et al. Citation2006; Jaunky Citation2013), but investigations on the shape of the relationship between GDP and material use produce inconclusive results (Hüttler, Schandl, and Weisz Citation1999; Bruyn Citation2002; Canas, Ferrão, and Conceição Citation2003; Bringezu et al. Citation2004). Studies investigating the drivers of material use, however, conclude that GDP is an important, if not the most important, determinant (Hoffren, Luukkanen, and Kaivo-oja Citation2001; van der Voet, van Oers, and Moll Citation2005b; Weisz et al. Citation2006; Steger and Bleischwitz Citation2011; Weinzettel and Kovanda Citation2011; Pothen and Schymura Citation2015).
Crucially, some studies claim that most European countries, in particular Western Europe, have managed to ‘decouple’ economic growth from material use, at least in relative terms (Bringezu and Schütz Citation2001; Moll, Bringezu, and Schütz Citation2005; van der Voet, van Oers, and Moll Citation2005b), a belief which is accepted by policy-makers (Ecorys Citation2011; OECD Citation2011; BMUB Citation2016) and reflected in several policy initiatives, for instance, the Raw Materials Initiative (EC Citation2008), the Europe 2020 strategy declaring resource efficiency as one of seven flagship initiatives (EC Citation2010), the Roadmap to a Resource Efficient Europe (EC Citation2011), and the Circular Economy Package (EC Citation2015b). From an economics perspective, ‘decoupling’ economic growth from material use is essentially about increasing the productivity by which materials are transformed into economic goods and services and thus linked to the total factor productivity of economies (Baptist and Hepburn Citation2013).
In summary, investigating the effect of economic growth on material use entails (1) a more comprehensive investigation on environmental pressures arising from economic activity, (2) an analysis on the drivers of material use, and (3) a verification on the ‘decoupling’ efforts in Europe.
We identify and address one major shortcoming in the literature. Most studies rely on correlation and simple regression analyses, failing to take the endogeneity of GDP into account, which results in biased and inconsistent estimates. In this paper, we estimate the causal relationship between GDP and material use, measured by domestic material consumption (DMC)Footnote2 by explicitly acknowledging that GDP and DMC are simultaneously determined. On the one hand, income drives consumption, including the consumption of materials (Ando and Modigliani Citation1963; Hall and Mishkin Citation1982). On the other hand, materials are an input into economic production functions (Ayres and van den Bergh Citation2005; O'Mahony and Timmer Citation2009). We address this endogeneity problem, along with the endogeneity problem caused by omitted variable bias by using an instrumental variable approach.
In our paper, we consider 32 European countries,Footnote3 which is a group of industrialised countries that are bound by similar regulation and use a standardised method to measure DMC. We use 2SLS, including country and time effects, and instrument GDP with the number of storm occurrences. This allows us to make causal inference under two conditions. First, storms need to be correlated with GDP, i.e. the instrument needs to be relevant. Second, storms must not impact DMC other than through GDP, i.e. the instrument needs to comply with the exclusion restriction.
With regard to the first point, the literature suggests that severe weather events have an impact on countries’ current and future GDP, with the majority of the literature suggesting a negative effect (Cavallo, Powell and Becerra Citation2010; World Bank and UN Citation2010; Cavallo et al. Citation2013; Noy and DuPont Citation2016). With regard to second point, two potential links between storms and DMC are discussed in Section 2, i.e. changes in trade, a link which is captured by GDP, and changes in material prices as a result of a storm. We present empirical evidence that some material prices are affected in the short term but this effect disappears two years after a storm occurs. As a consequence, we use a temporal instrumentation strategy to estimate the causal impact of GDP on DMC with the instrument lagged two years to meet the exclusion restriction.
Our results provide new evidence that increasing the GDP growth rate causes an increase in the DMC growth rate for Western Europe, whereas the effect is insignificant for Eastern European economies, which questions the current wisdom, especially among European policy-makers, that the link between GDP and material use in Western Europe has been broken. Why would Western European economies demand more materials when their economies expand? We discuss two explanations that are consistent with these results. Lower energy prices in Western Europe compared to the rest of Europe increase the demand for materials through its complementarity to energy, and higher labour cost in Western Europe compared to the rest of Europe could have stimulated substitution from labour to materials.
The remainder of the paper is structured as follows: Section 2 introduces the modelling approach, where we explain our instrumentation strategy in detail. Section 3 describes the data. Section 4 outlines the results. Section 5 places the results in a wider context and Section 6 concludes.
2. Modelling approach
2.1. The problem of endogeneity
A considerable body of literature investigating drivers of material use and ‘decoupling’ suggests that GDP affects DMC (van der Voet, van Oers, and Moll Citation2005b; Weisz et al. Citation2006; Steger and Bleischwitz Citation2011; Pothen and Schymura Citation2015). As national income increases, consumption also increases as proposed by the theoretical work on the Modigliani's life-cycle hypothesis, which essentially argues that consumption is a function of income (Ando and Modigliani Citation1963). Empirical studies provide evidence for short- and long-term effects of changes in income on consumption (Hall and Mishkin Citation1982; Campbell and Mankiw Citation1989). Although the size of the impact of changes in income on the use of material depends on the material requirements of economic goods being consumed, economic activity ultimately leads to an increase in the use of materials as long as economic activity affects the consumption of economic goods.
Two sources of endogeneity are likely to be present in models investigating the impact of GDP on DMC – reverse causality and omitted variables. Reverse causality between GDP and DMC arises since materials are inputs to a country's production function (Ayres and van den Bergh Citation2005; O'Mahony and Timmer Citation2009). This follows from the basic production process where inputs are converted into outputs, and materials have also been included as production inputs in recent growth models (O'Mahony and Timmer Citation2009). The second source of endogeneity, i.e. omitted variables, occurs when determinants of the dependent variable which are omitted from the model, e.g. economic structure, energy prices, etc., are correlated with GDP. Both sources of endogeneity would bias OLS estimation.
2.2. Choice of instrument
We choose the number of storms occurred in a country as an instrument for GDP. Natural hazards have previously been used as instruments for economic variables such as trade, aid inflows, and oil income (Ramsay Citation2011; Felbermayr and Gröschl Citation2013; Jackson Citation2014). The main rationale for using storm occurrences as an instrument is that such events affect GDP in an exogenous manner.
Kahn (Citation2005) finds that the general level of economic development, institutional quality, and geography affect the consequences of natural hazards. However, the economy's sensitiveness to storm disasters is unlikely to change in a relatively short time period (World Bank and UN Citation2010). In addition, we minimise this risk by using the number of storms rather than monetary variables, e.g. damages caused by storm, as these are more likely to be influenced by the level of economic development. Moreover, we eliminate country-specific trends by taking the first difference which might further reduce the risk of storms being endogenous, as changes in storm occurrences are less likely to correlate with economic development. Additionally, by taking the first difference, we avoid any standard complication related to spurious regressions.
Storms occur relatively frequently, both across countries and time. Since we estimate a fixed effects model in first differences, we need to consider an instrument that varies over time, a consideration that led us to exclude earthquakes, volcanoes, and droughts, which either do not occur frequently or not at all in several countries. We preferred using storms rather than floods, since storms typically affect larger areas. For instance, storms could affect transportation routes and energy generation as well as supply networks, thus impacting economic activity regardless of its proximity to economic centres. Areas affected by storms are also more likely to vary across time, as areas affected by floods need to be close to rivers or to the sea. Storms have been reported in every European country except Finland and Serbia, whereas eight European countries have not reported any flood during the sample period used in this study. In addition, flood defence mechanisms may reduce the likelihood of re-occurring floods or their impact on GDP, therefore potentially compromising the exogeneity of the instrument.
Data on extreme weather events are publically available in the Emergency Events Database EM-DAT (Guha-Sapir, Below, and Hoyois Citation2014). EM-DAT contains indicators that measure storms in different ways.Footnote4 Storm occurrences, rather than any of the alternative measures, have the advantage of being more likely to be a precise, objective, and exogenous measure. However, this comes at the expense of treating all storms equally, regardless of their impact. Nevertheless, the EM-DAT database only comprises those storms that had a significant impact, i.e. 10 or more people reported killed, 100 or more people reported affected, declaration of a state of emergency, or call for international assistance.
We are also mindful that reporting might be heterogeneous across countries, although we believe that this potential problem has limited relevance in our sample, since most European countries are industrialised economies with established monitoring authorities and reporting requirements. Under-reporting is not likely to be an issue as European countries have an incentive to correctly report disasters, since they become eligible to emergency aids to cope with immediate damages as well as long-term investments in prevention from the European Union's Solidarity Fund and the Civil Protection Mechanism.
2.3. Instrumentation strategy
As already mentioned, instruments need to be relevant and valid. Relevant instruments require a strong correlation between the instrument and the endogenous variable. The great majority of investigations embrace the view that disasters are a setback for economic growth due to damages to the capital stock and additional disruptions of economic activity during their immediate aftermath (e.g. electricity cuts, obstructing people to work) as well as their subsequent course (e.g. crowding-out investments, migration, reconstruction delays, welfare transfers) (Hochrainer Citation2009; Raddatz Citation2009; Hsiang and Jina Citation2014; DuPont et al. Citation2015).Footnote5
This can be exemplified by the extra-tropical cyclone Xynthia which affected France in February 2010. It had severe negative impacts on economic activity, both during the immediate aftermath and a few years after the disaster.Footnote6 However, the reconstruction after the storm can positively contribute to economic growth. Regardless of whether storms have a positive or a negative effect, they are thought to affect economic activity.
The exclusion restriction requires the instrument to affect the dependent variable (DMC) only through the endogenous variable which has been instrumented for (GDP). Felbermayr and Gröschl (Citation2013) use natural hazards in neighbouring countries rather than the home country to instrument for the endogenous regressor in the home country to increase the chances of meeting the exclusion restriction. However, this spatial differentiation approach is not suitable for our study, since storms tend to affect several neighbouring countries simultaneously. Instead, we adopt a temporal instrumentation strategy that attenuates the potential direct impact of storms on DMC.
One way storms could impact DMC is through trade. If a country is hit by a storm, the national production capacity is temporarily reduced, which could reduce its exports and increase imports. As net exports are part of GDP, changes in trade due to storms would affect DMC only through GDP, which is not a violation of the exclusion restriction. Another way storms could affect DMC is through their impact on material prices. We explore this possibility by assessing the relationship between storms and prices of the subgroups of DMC (biomass, metals, minerals, and fossil fuels), since there is no DMC price indicator available across Europe.
Metals and fossil fuels are traded on global markets and local storms are unlikely to affect those prices. This is particularly likely for our sample since Europe is a small producer of metals and fossil fuels. As the markets for biomass and minerals are mainly local, we investigate whether storms have an impact on those prices, as a statistical association would cast doubts on our instrument. We estimate the following models:(1) where
are time effects,
are country fixed effects,
indicates the number of storm occurrences. These are taken from the EM-DAT database unless explicitly stated. The letter h indicates the time lag with
.
indicates the price of biomass, material inputs, and mineral prices as dependent variables in the three different models we estimate – as shown in .
Table 1. Relationship between the number of storm occurrences and material prices in Europe.
To proxy biomass prices, we chose real industrial crop prices in Europe between 2000 and 2014 from Eurostat. As shown in Columns 1–3, we find no significant relationship between storms and crop prices. In the case of material input prices, Columns 4–6, we consider the correlation between the number of storm occurrences and the material input prices for constructing residential building from Eurostat in Europe between 2000 and 2014. As shown in , we find no statistically significant association between storms and material input prices across time. In the case of mineral prices, Columns 7–9, we use data from the Nordic Statistic Database on the mineral costs of dwelling construction and the number of storms from the Extreme Wind Storms Catalogue for Finland, Sweden and Denmark from 2000 to 2013. The Nordic countries are the only group of countries that collect mineral price data using a standardised approach, both in terms of data collection and variable definition.
As shown in , we find that the relationship between storms and mineral prices in the current period is significant at the 10% level and negative. The impact of a storm in the previous period on mineral prices is positive (possibly due to reconstruction) and is just outside the 10% significance level, but when storms are lagged two years, the statistical association completely disappears. This brief analysis suggests that the relationship between storms and material prices becomes statistically insignificant after two years. In line with our temporal strategy mentioned above, we lag our instrument twice to ensure that our instrument satisfies the exclusion restriction so that no impact of storms affect DMC without going through changes in the level of economic activity.
2.4. Model specification
In our model, DMC in the current period is influenced by GDP in the previous period. Increasing material use involves coordination across several stages of the supply chain, as inventories are not likely to be able to accommodate large demand shifts. Also, the material use of households tend to respond with a delay to changes in income, e.g. cars and houses are not purchased immediately after a raise in income (Shapiro and Slemrod Citation2009; Jappelli and Pistaferri Citation2010). Hence, we formulate our model as(2) where DMC is the domestic material consumption in tonnes per capita, GDP is the per capita real gross domestic product in PPP,
are time effects to control for any year-specific events, e.g. the financial crisis,
are country fixed effects, and
is the idiosyncratic error term. Countries are likely to have a different cultural and historic background, economic development paths, institutional quality, material policies and other country-specific trends which might influence the time pattern of DMC and GDP (Bahn-Walkowiak and Steger Citation2015). As discussed above, by taking the first differences of EquationEquation (2)
(2) , country-specific trends are eliminated, therefore, reducing the risk of spurious regressions. Thus, our main model is specified as follows:
(3) where
are time effects and
are country fixed effects. The first stage of our model considers the impact of storm occurrences on GDP. As discussed above, storms are lagged twice in order to comply with the exclusion restriction. The first stage of our model is specified as
(4) where
are time effects,
are country fixed effects,
are the number of storm occurrences, and
is the idiosyncratic error term. EquationEquations (3)
(3) and (Equation4
(4) ) are estimated using 2SLS with standard errors clustered over countries. All data are in natural logarithm.Footnote7
3. Data description
Materials, i.e. usable substances obtained or derived from natural resources, consist of biomass, metals, minerals, and fossil fuels (EC Citation2017; OECD Citation2007). Material flow analysis (MFA) is an established method to calculate material use indicators (OECD Citation2008). MFA has been increasingly standardised over time and adopted by several statistical offices in industrialised countries, including all European countries (Hinterberger, Giljum, and Hammer Citation2003). We use data on DMC in tonnes per capita for 32 European countries between 2000 and 2014, extracted from Eurostat. DMC is the only measure of material use with publically available data which is directly comparable across our sample (EC Citation2015a).Footnote8 For economic activity, real GDP in Euro (in PPP) per capita is extracted from Eurostat.
displays the observations used in the main model (EquationEquation (3)(3) ) of the estimation. Western and Eastern European countries are considered separately because they were exposed to different political and economic systems in the recent past.Footnote9
As an instrument, we use the number of storm occurrences from the EM-DAT database. This database, which is maintained by the Centre for Research on the Epidemiology of Disasters at the Université Catholique de Louvain in Belgium, contains information on more than 18,000 extreme weather events and accidents. Data are collected from UN agencies, non-governmental organisations, insurance companies, and research institutes.
Storms are included in the database if at least one of the following criteria applies: (1) 10 or more people reported killed, (2) 100 or more people reported affected, (iii) declaration of a state of emergency, or (iv) call for international assistance. We complement the existing data by ‘recovering’ several observations. If a country in a given year has reported any disasters other than storms, we assume that no storms occurred in that year. shows the main statistics for our dependent variable (DMC per capita), endogenous variable (GDP per capita), and instrument (number of storm occurrences) for the observations used in our study.
4. Results
Starting with the first stage model (EquationEquation (4)(4) ), we estimate the relationship between storms and GDP. As indicated in , the results show a negative and statistically significant association between changes in the number of storms and the GDP growth rate for all three groups of countries. Those results support the literature advocating that disasters have a negative impact on economic activity (Hochrainer Citation2009; Raddatz Citation2009; Hsiang and Jina Citation2014; DuPont et al. Citation2015). The impact of changes in storm occurrences on the GDP growth rate is lower in Western Europe compared to Eastern Europe, suggesting that the Western European economies can cope with storms more efficiently.
Table 2. First stage results for Europe, Western Europe, and Eastern Europe between 2000 and 2014.
Using the number of storms to instrument GDP appears to be a robust instrumentation strategy. The Kleibergen–Paap Wald F-statistics for all country groups is well above 10, which is a common rule of thumb for considering an instrument to be sufficiently strong (Angrist and Pischke Citation2009). The Kleibergen–Paap rk LM test, which assesses whether the equation is identified, i.e. that the instrument is ‘relevant’, allows us to reject the null hypothesis of the equation being underidentified for all groups. All test statistics in the table are robust to heteroscedasticity and arbitrary within-correlation. Additionally, the conditional likelihood ratio (CLR) test proposed by Moreira (Citation2003) verifies the validity of our instrumentation strategy for Western Europe. However, the fact that the null hypothesis of the CLR test is rejected at the 5% level only for Western Europe somewhat limits the validity of the results for Europe as a whole and Eastern Europe. Therefore, we interpret the results of these groups as indications.
4.1. OLS results
We now consider our main model (EquationEquation (3)(3) ) estimated with OLS. As displayed in , the impact of the GDP growth rate on the DMC growth rate is positive for all three groups but insignificant for Eastern Europe. This means that we cannot make any strong claims about how the DMC growth rates changes in Eastern European countries, whereas the GDP growth rate across Europe and Western Europe has a positive and statistically significant impact on the DMC growth rate. The relatively low number of observations compared to the panel considered stems from the fact that using variables in first difference requires data for at least two consecutive years across all variables in the model.
Table 3. OLS and second stage results for Europe, Western Europe, and Eastern Europe between 2000 and 2014.
4.2. 2SLS results
The results obtained when using 2SLS are slightly different. There is no indication for a significant link between the GDP growth rate and the DMC growth rate for Europe as a whole and Eastern Europe. However, the results for Western Europe indicate that a 1% increase in the GDP growth rate causes the DMC growth rate to increase by 2.7%, which is three times as big as the OLS coefficient. Thus, OLS is considerably biased downwards, implying that the omitted variable bias might dominate over the reverse causality bias, because we expected the effect of DMC on GDP to be positive, which would have led OLS to be upwards biased. However, the median value of the OLS results for Western Europe lies within the 95% confidence interval of the 2SLS result.
At first sight, the 2SLS results are in disagreement with previous findings. For instance, investigations conducted by the G7 concludes that ‘for every 1% increase in GDP, raw material use has risen by 0.4%’.Footnote10 Bringezu et al. (Citation2004) find that GDP increases material useFootnote11 by approximately 0.6–0.8 tonnes per 1000 USD. Wiedmann et al. (Citation2006) find that the elasticity of DMC with respect to GDP is around 0.15. Interestingly, we find somewhat similar magnitudes for Europe and Western Europe using the OLS model. However, our models are estimated in first difference whereas previous results are based on models in levels, thus a direct comparison is not possible. Other studies have found that most European countries, in particular Western Europe, supposedly have broken the link between economic activity and material use, at least in relative terms (Bringezu and Schütz Citation2001; Moll, Bringezu, and Schütz Citation2005; van der Voet, van Oers, and Moll Citation2005b). Our IV-estimations provide new evidence casting doubt on such claims, which seem to be acratically accepted by policy-makers (Ecorys Citation2011; OECD Citation2011; BMUB Citation2016).
4.3. Robustness checks
summarises our robustness checks for Europe, Western Europe, and Eastern Europe. Throughout all checks, our instrumentation remains robust. The time effects are highly significant and thus included in all estimations. We assessed the impact of excluding the country fixed effects and discovered that dropping them does not change our results significantly.
Table 4. Robustness checks for Europe, Western Europe, and Eastern Europe using 2SLS.
We also tested our results’ robustness and sensitivity by excluding individual countries and years. In particular, excluding the years of the financial crisis 2008–2010 (Columns 1–3) somewhat reduces the significance of the coefficient for Western Europe, but the magnitudes of the coefficients are very similar. We also estimated our model after excluding the smallest European countries, which have a population below 1 million, i.e. Malta, Luxembourg, and Cyprus (Columns 4–6). This does not change our results significantly. We further investigated whether the financial crisis caused a structural break in the data but we could not find a structural break in 2007 or 2008 based on visual inspection of pre- and post-crisis trends for each country.
As an additional robustness check, we conducted a grid search approach to test various transformations of GDP and DMC to see whether any transformation (other than natural logarithms) better explains the relationship. Considering the residual sum of squares and the value of the coefficients, results from other transformations of the variables were found to be only marginally different from the natural logarithm. Furthermore, we analyse the residuals of the estimations for Europe, Western Europe, and Eastern Europe separately in order to evaluate whether the assumption of a common relationship between GDP and DMC within these three groups is reasonable. Since there are no obvious outliers visible in the residuals of the country groups, we are confident that the assumption of a common relationship within these groups is justified.
As part of our robustness checks, we control for three variables that are considered important drivers of DMC (Schandl and West Citation2010; Giljum et al. Citation2012; Steinberger et al. Citation2013). First, we control for real per capita labour productivity () retrieved from Eurostat. Labour productivity has a positive effect on DMC in Europe and Eastern Europe. The impact of the GDP growth rate on the DMC growth rate becomes negative and significant for Eastern Europe, which seems to be an unreliable and most of all not robust result given the large p-value of the CLR test. Second, we control for the service sector share of GDP (
) from Eurostat. Although the service sector is associated with lower material use, it does not appear to have a significant impact on DMC. Third, we control for industrial electricity prices in Euro per kWh including all taxes and levies retrieved from Eurostat (
). We find no significant relationship between electricity prices and DMC. The results are shown in . It is worth mentioning that the standard errors in Columns 4–6 are fairly similar to the ones in .
Table 5. Control variables for Europe, Western Europe and Eastern Europe.
We evaluated different types of standard errors, i.e. those assuming homoscedasticity and those robust to heteroscedasticity, but all give qualitatively the same results. We also consider the possibility of serial correlation, using the approach suggested by Newey and West (Citation1987) and estimate EquationEquation (3)(3) controlling for various autocorrelation structures. In , we show that our results are essentially unchanged when using AR(1), AR(3), and AR(5) error structures, particularly the standard errors for Western Europe are fairly similar to the ones in .
Table 6. Results assuming different autocorrelation structures.
So far, we have tested for weak instruments by showing that the Kleibergen–Paap rk Wald F-statistics is sufficiently high and the CLR test produces a low p-value for Western Europe. An alternative approach is to generate random variables, i.e. variables with random values, but with the same mean and standard deviation as our instrument and use these variables with the same statistical properties as our instrument to estimate our model by ‘placebo’ 2SLS regressions. If the results were similar to those displayed in , our instrument could be questioned (Bound, Jaeger, and Baker Citation1995). This approach is chosen over using alternative instruments as a robustness check, because other measures of storms, including their severity measured by the number of people affected, are only imprecisely and sparsely reported in the EM-DAT database. We also believe that the number of storms is more likely to be an exogenous indicator than measures of storms’ severity which are likely to be affected by the level of economic development.
Therefore, we run 1000 ‘placebo’ 2SLS regressions to estimate our model with random variables which have the same statistical properties as our ‘real’ instruments. The second stage coefficients are shown in and for Europe. The results for Western Europe are shown in and and for Eastern Europe in and . As expected, the median and, to a lesser extent, the mean values are similar to the OLS coefficients in , but they are imprecisely estimated. In short, our instrument passes the non-robustness test proposed by Bound, Jaeger, and Baker (Citation1995).
Figure 2. ‘Pseudo’ 1000 2SLS regressions for Europe: to increase readability we have limited the range between −40 and 40, thereby excluding 29 coefficients.
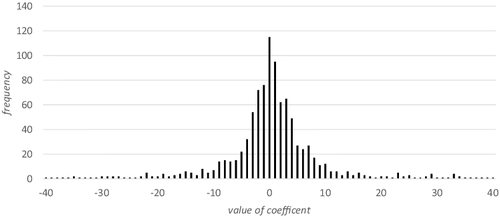
Table 7. ‘Pseudo’ regression statistics for Europe.
5. Discussion
The results suggest that increasing the GDP growth rate causes an increase in the DMC growth rate for Western European countries, while there is no indication for an effect on the DMC growth for Eastern Europe. Therefore, a potential difference between Western and Eastern Europe can be explored further using statistical methods.
This section descriptively explores if substitution and complementarity across input factors are consistent with the potential difference between the two groups. It has sometimes been claimed that energy and materials are complements (Nordic Council of Ministers Citation2001; Hannon Citation2013), while labour and materials are substitutes (Bruyn et al. Citation2009; Allwood et al. Citation2011; Bleischwitz Citation2012). In , we display energy prices and labour costs in Western and Eastern European countries. Energy prices are PPP-adjusted and include taxes and levies; the arithmetic average of 21 European countriesFootnote12 for the years 2000, 2004, 2008, and 2012 from International Energy Agency (IEA) is shown. It is clear that energy prices are lower in the Western Europe compared to Eastern European countries. Given complementarity between energy and materials, lower energy prices in Western Europe are consistent with, ceteris paribus, a higher demand for materials in Western Europe.
Table 8. Energy prices and labour costs in Western and Eastern Europe in 2000, 2004, 2008, and 2012.
also compares labour costs in Western and Eastern European countries by using data on total average hourly labour costs in Euros, i.e. including social security contributions, taxes, and subsidies. The data show the arithmetic means of 28 European countriesFootnote13 for the years 2000, 2004, 2008, and 2012 (from Eurostat). Labour costs are PPP-adjusted using the price level ratio of PPP conversion factors from the World Bank. Labour costs in Western Europe are more than double the costs in Eastern European countries. Thus, given substitutability between labour and materials, the demand for materials in Western Europe is likely to be higher than demand in Eastern Europe.
6. Conclusions
The aim of this paper has been to determine the causal impact of economic growth on material use in Europe. A number of academic studies have advocated the use of material and footprint indicators, arguing that they better represent environmental pressures compared to single-pressure indicators such as CO2 emissions (Alfsen and Sæbø Citation1993; Bringezu et al. Citation2003; Ibenholt Citation2003; van der Voet, van Oers, and Moll Citation2005b; Hertwich Citation2010; Bleischwitz Citation2010; Hoang and Alauddin Citation2012; Bouwmeester and Oosterhaven Citation2013; Wiedmann et al. Citation2006; Teixidó-Figueras and Duro Citation2015). In addition, a number of recent policy initiatives have adopted material indicators (EC Citation2008, Citation2010, Citation2011). Unfortunately, the empirical literature investigating the link between economic growth and material use has not convincingly dealt with the endogeneity of GDP, thus making previous estimates biased and inconsistent.
We provide new evidence for a causal and positive impact of the GDP growth rate on the DMC growth rate for Western European economies, using the number of storm occurrences as an instrument for GDP. OLS, which has been used in the previous literature, underestimates that relationship. In the case of Eastern Europe and Europe as a whole, our work does not provide any evidence contrary to the general belief that economic growth and material use have been ‘decoupled’. However, in the case of Western Europe, our results contradict previous studies and the current wisdom among policy circles, both suggesting that Western European countries have indeed ‘decoupled’ economic growth from material use. Thus, we present new evidence that the link between the two variables is likely to exist and to be higher than previously thought.
We offer two potential explanations that are consistent with our findings. First, energy prices are lower in Western Europe compared to Eastern European countries, suggesting that material demand has increased in Western Europe due to the complementary between energy and materials. Second, we find that labour costs in Western Europe are higher compared to Eastern European countries. This indicates that labour might have been substituted by materials.
Our results are important from a policy perspective since they imply that more effective policy interventions are necessary to break the link between GDP and DMC, in particular for Western European economies. As such, our findings put in question the effectiveness of current policies to ‘decouple’ economic growth and material use. Future research efforts could complement our approach by more analyses on the relationship between GDP and various material indicators, taking policies and technology into account, and expanding the scope of the study to the global level. Additionally, the differences between Western and Eastern European countries could be researched in greater detail.
Acknowledgments
The authors would like to thank Raimund Bleischwitz, Matthieu Glachant, Frank Pothen, Sandy Skelton, Simone Cooper, Manfred Rosentock, Jun Rentschler, and all participants of the Sino-European Circular Economy and Resource Efficiency (SINCERE) project seminars in October 2015 in Shanghai and September 2016 in Paris for their valuable contributions and suggestions. The comments of two anonymous reviewers significantly improved the paper. All remaining errors are entirely ours. Söderberg acknowledges financial support from ANR [ANR-14-ORAR-0001]. Agnolucci acknowledges financial support from ESRC [ES/L015838/1].
Disclosure statement
No potential conflict of interest was reported by the authors.
Additional information
Funding
Notes
1. Other researchers, however, oppose this view since materials are typically measured in weight and aggregated across material types, leading to a biased and imprecise measure of specific environmental pressures (Cleveland and Ruth Citation1998; Voet, Oers and Nikolic Citation2005a). These considerations do not limit the significance of our analysis, because such studies (1) fail to include the temporal effect of material use on the environmental across time, (2) the composition of material use is unlikely to change substantially across our sample, thus not significantly affecting the results of our model which includes country fixed effects, and (3) are often interested in specific environmental pressures, whereas our analysis considers general effects.
2. DMC measures the total amount of materials directly used within an economy. It comprises biomass, metals, minerals, and fossil fuels and is defined as the quantity (in terms of weight) of domestically extracted raw materials, plus direct material imports minus direct material exports (EC Citation2015a).
3. A list of the countries can be found in .
4. Such measures include total deaths, number of people affected, damages in dollars, and a count variable on the number of storm occurrences.
5. Some studies find a positive relationship between natural hazards and economic activity which is often linked to reconstruction efforts and the long term (Cavallo et al. Citation2013; Noy and DuPont Citation2016).
6. The storm killed 53 people, affected more than 500,000 people nation-wide, and caused more than USD 4.2 billion worth of damages (EM-DAT database). Approximately half of the damages were insured, the rest had to be borne privately, suggesting a reduction in long-term consumption of affected households assuming Modigliani's life-time consumption smoothing hypothesis. Workers were prevented from going to work, electricity supply was cut for over a million homes and the train infrastructure was damaged. The storm triggered a major flood in Charente-Maritime, which had a number of impacts: oyster farms (a major employer in the area) were destroyed, the tourism sector suffered, and productivity of agricultural land (including wine production) was affected by salty sea water (Lumbroso and Vinet Citation2011; Genovese and Przyluski Citation2013).
7. In the case of the number of storm occurrences, we take the natural logarithm after adding one to avoid missing values caused by this transformation.
8. Alternative indicators, for instance, Raw Material Consumption, are only available for the EU-aggregate and a few individual countries for a limited time period and without a standardised calculation method. Similar limitations apply to the indicator Total Material Requirement or comparable material footprint data.
9. An overview of the country groupings is shown in . There is no universally agreed definition of Western Europe. We consider Western Europe to be those countries that neither have been part of the Soviet Union nor Yugoslavia, as those countries have not been integrated into the free-market economies of the West. Turkey is considered part of Eastern Europe. Finland, Malta, and Cyprus are considered more closely connected to the Western European economies, in particular during our sample period, and we acknowledge that Malta and Cyprus have strong historic links to the United Kingdom.
10. http://www.consilium.europa.eu/en/meetings/international-summit/2015/06/02_2015-06-08-ANNEX_FINAL_CLEAN_pdf (last accessed on 28 June 2016).
11. Material use here is measured as Domestic Material Input (DMI) which is equivalent to DMC plus material exports.
12. Out of the 21 European countries, 15 are Western European (Austria, Belgium, Denmark, Finland, France, Germany, Greece, Ireland, Italy, Luxembourg, Netherlands, Portugal, Spain, Sweden, and the United Kingdom) and 6 are Eastern European countries (Czech Republic, Estonia, Hungary, Poland, Slovak Republic, and Slovenia).
13. All countries in are considered with the exception of Norway, Switzerland, Serbia, and Turkey.
References
- Alfsen, K.H., and H.V. Sæbø. 1993. “Environmental Quality Indicators : Background , Principles and Examples from Norway.” Environmental & Resource Economics 3: 415–435. doi:10.1007/BF00310246.
- Allwood, J.M., M.F. Ashby, T.G. Gutowski, and E. Worrell. 2011. “Material Efficiency: A White Paper.” Resources, Conservation and Recycling 55: 362–381. doi:10.1016/j.resconrec.2010.11.002.
- Ando, A., and F. Modigliani. 1963. “The ‘Life Cycle’ Hypothesis of Saving: Aggregate Implications and Tests.” The American Economic Review 53: 55–84. doi:10.1126/science.151.3712.867-a.
- Angrist, J.D., and J.-S. Pischke. 2009. Mostly Harmless Econometrics: An Empiricist's Companion. Princeton, NJ: Princeton University Press.
- Arrow, K., B. Bolin, R. Costanza, P. Dasgupta, C. Folke, C.S. Holling, B.-O. Jansson, et al. 1995. “Economic Growth, Carrying Capacity, and the Environment.” Ecological Economics: The Journal of the International Society for Ecological Economics 15: 91–95. doi:10.1016/0921-8009(95)00059-3.
- Ayres, R.U., and J. C. J. M. van den Bergh. 2005. “A Theory of Economic Growth with Material/Energy Resources and Dematerialization: Interaction of Three Growth Mechanisms.” Ecological Economics: The Journal of the International Society for Ecological Economics 55: 96–118. doi:10.1016/j.ecolecon.2004.07.023.
- Bahn-Walkowiak, B., and S. Steger. 2015. “Resource Targets in Europe and Worldwide: An Overview.” Resources 4: 597–620. doi:10.3390/resources4030597.
- Baptist, S., and C. Hepburn. 2013. “Intermediate Inputs and Economic Productivity.” Philosophical Transactions of the Royal Society 371: 1–21.
- Bleischwitz, R. 2010. “International Economics of Resource Productivity – Relevance, Measurement, Empirical Trends, Innovation, Resource Policies.” International Economics and Economic Policy 7: 227–244. doi:10.1007/s10368-010-0170-z.
- Bleischwitz, R. 2012. “Towards a Resource Policy – Unleashing Productivity Dynamics and Balancing International Distortions.” Mineral Economics 24: 135–144.
- BMUB. 2016. Deutsches Ressourceneffizienzprogramm II – Programm zur nachhaltigen Nutzung und zum Schutz. Berlin: German Federal Ministry for the Environment, Natural Conservation, Building and Nuclear Safety.
- Bound, J., D.A. Jaeger, and R.M. Baker. 1995. “Problems with Instrumental Variables Estimation When the Correlation Between the Instruments and the Endogenous Explanatory Variable Is Weak.” Journal of the American Statistical Association 90: 443–450. doi:10.1080/01621459.1995.10476536.
- Bouwmeester, M.C., and J. Oosterhaven. 2013. “Specification and Aggregation Errors in Environmentally Extended Input–Output Models.” Environmental & Resource Economics 56: 307–335. doi:10.1007/s10640-013-9649-8.
- Bringezu, S., and H. Schütz. 2001. “Total Material Requirement of the European Union.” Technical report No 55. Wuppertal: Wuppertal Institute.
- Bringezu, S., H. Schütz, and S. Moll. 2003. “Rationale for and Interpretation of Economy-Wide Material Flow Analysis and Derived Indicators.” Journal of Industrial Ecology 7: 43–64.
- Bringezu, S., H. Schütz, S. Steger, and J. Baudisch. 2004. “International Comparison of Resource use and its Relation to Economic Growth.” Ecological Economics: The Journal of the International Society for Ecological Economics 51: 97–124. doi:10.1016/j.ecolecon.2004.04.010.
- Bruyn, S., A. Markowska, F. de Jong, and M. Blom. 2009. Resource Productivity, Competitiveness and Environment Policies. The Netherlands: Delft.
- Bruyn, S.M. 2002. Dematerialization and Rematerialization as Two Recurring Phenomena of Industrial Ecology. Cheltenham: Edward Elgar.
- Campbell, J., and N. Mankiw. 1989. “Consumption, Income and Interest Rates: Reinterpreting the Time Series Evidence.” NBER Macroeconomics Annual 4: 185–246. doi:10.2307/3584974.
- Canas, Â., P. Ferrão, and P. Conceição. 2003. “A New Environmental Kuznets Curve? Relationship Between Direct Material Input and Income per Capita: Evidence from Industrialised Countries.” Ecological Economics: The Journal of the International Society for Ecological Economics 46: 217–229. doi:10.1016/S0921-8009(03)00123-X.
- Cavallo, E., S. Galiani, I. Noy, and J. Pantano. 2013. “Catastrophic Natural Disasters and Economic Growth.” The Review of Economics and Statistics 95: 1549–1561. doi:10.1162/REST_a_00413.
- Cavallo, E., A. Powell, and O. Becerra. 2010. “Estimating the Direct Economic Damages of the Earthquake in Haiti.” Economic Journal 120: 298–312. doi:10.1111/j.1468-0297.2010.02378.x.
- Cleveland, C., and M. Ruth. 1998. “Indicators of Dematerialization and the Materials Intensity of use.” Journal of Industrial Ecology 2: 15–50.
- DuPont, W., I. Noy, Y. Okuyama, and Y. Sawada. 2015. “The Long-Run Socio-economic Consequences of a Large Disaster: The 1995 Earthquake in Kobe.” PloS One 10: 1–17. doi:10.1371/journal.pone.0138714.
- EC. 2008. The Raw Materials Initiative – Meeting Our Critical Needs for Growth and Jobs in Europe COM(2008) 699 Final. Brussels: The European Commission.
- EC. 2010. Europe 2020 – a Strategy for Smart, Sustainable and Inclusive Growth. Brussels: The European Commission.
- EC. 2011. Roadmap to a Resource Efficient Europe - COM(2011) 571 final. Brussels: The European Commission.
- EC. 2015a. Domestic Material Consumption – Explanatory Text. Luxembourg: The European Commission.
- EC. 2015b. Closing the Loop – an EU Action Plan for the Circular Economy. doi: 10.1017/CBO9781107415324.004
- EC. 2017. Material Flow Accounts. Luxembourg: The European Commission.
- Ecorys. 2011. Study on the Competitiveness of the European Companies and Resource Efficiency. Rotterdam: The European Commission.
- Felbermayr, G., and J. Gröschl. 2013. “Natural Disasters and the Effect of Trade on Income: A New Panel IV Approach.” European Economic Review 58: 18–30. doi:10.1016/j.euroecorev.2012.11.008
- G7. 2015. “Leadersʼ Declaration G7 Summit”; June 7–8; Schloss Elmau.
- Galeotti, M., A. Lanza, and F. Pauli. 2006. “Reassessing the Environmental Kuznets Curve for CO2 Emissions: A Robustness Exercise.” Ecological Economics: The Journal of the International Society for Ecological Economics 57: 152–163. doi:10.1016/j.ecolecon.2005.03.031.
- Genovese, E., and V. Przyluski. 2013. “Storm Surge Disaster Risk Management: The Xynthia Case Study in France.” Journal of Risk Research 16: 825–841. doi:10.1080/13669877.2012.737826.
- Giljum, S., B. Lugschitz, C. Polzin, S. Lutter, and M. Dittrich. 2012. Resource Use and Resource Efficiency in Central and Eastern Europe and the Newly Independent States. Vienna: UNIDO.
- Guha-Sapir, D., R. Below, and P. Hoyois. 2014. Emergency Response Database (EM-DAT): International Disaster Database. Brussels: Université Catholique de Louvain.
- Hall, R.E., and F.S. Mishkin. 1982. “The Sensitivity of Consumption to Transitory Income: Estimates from Panel Data on Households.” Econometrica: Journal of the Econometric Society 50: 461–481. doi:10.2307/1912638.
- Hannon, B. 2013. “Energy and Materials Conservation: Applying Pioneering Research and Techniques to Current Non-energy Materials Conservation Issues.” Philosophical Transactions of the Royal Society 371: 20120005. doi: rsta.2012.0005 [pii]\r10.1098/rsta.2012.0005.
- Hertwich, Edgar. 2010. “Assessing the Environmental Impacts of Consumption and Production - Priority Products and Materials.” A Report of the Working Group on the Environmental Impacts of Products and Materials to the International Panel for Sustainable Resource Management. UNEP IRP.
- Hinterberger, F., S. Giljum, and M. Hammer. 2003. “Material Flow Accounting and Analysis (MFA) – a Valuable Tool for Analyses of Society–Nature Interrelationships.” SERI Background Paper. Vienna.
- Hoang, V.N., and M. Alauddin. 2012. “Input-Orientated Data Envelopment Analysis Framework for Measuring and Decomposing Economic, Environmental and Ecological Efficiency: An Application to OECD Agriculture.” Environmental and Resource Economics 51: 431–452. doi:10.1007/s10640-011-9506-6.
- Hochrainer, S. 2009. “Assessing the Macroeconomic Impact of Natural Disasters: Are There Any?” World Bank Policy Research Working Paper 4968, 1–43. Washington, DC.
- Hoffren, J., J. Luukkanen, and J. Kaivo-oja. 2001. “Decomposition Analysis of Finnish Material Flows: 1960 – 1996.” Journal of Industrial Ecology 4: 105–125.
- Hsiang, S.M., and A.S. Jina. 2014 “The Causal Effect of Environmental Catastrophe on Long-Run Economic Growth: Evidence from 6,700 Cyclones.” NBER Working Paper Series, 1–70. doi:10.3386/w20352
- Hüttler, W., H. Schandl, and H. Weisz. 1999. Are Industrial Economies on the Path of Dematerialization? Material Flow Accounts for Austria 1960–1996: Indicators and International Comparison. Amsterdam.
- Ibenholt, K. 2003. “Material Accounting in a Macroeconomic Framework: Forecast of Waste Generated in Manufacturing Industries in Norway.” Environmental and Resource Economics 26: 227–248. doi:10.1023/A:1026346119612.
- Jackson, O. 2014. Natural Disasters, Foreign Aid, and Economic Growth (working paper). http://dx.doi.org/10.2139/ssrn.2329404
- Jappelli, T., and L. Pistaferri. 2010. “The Consumption Response to Income Changes.” Annual Review of Economics 2: 479–506. doi:10.1146/annurev.economics.050708.142933.
- Jaunky, V.C. 2013. “A Cointegration and Causality Analysis of Copper Consumption and Economic Growth in Rich Countries.” Resources Policy 38: 628–639. doi:10.1016/j.resourpol.2013.10.001.
- Kahn, E.M. 2005. “The Death Toll from Natural Disasters: the Role of Income, Geography, and Institutions.” The Review of Economics and Statistics 87: 271–284.
- Lumbroso, D.M., and F. Vinet. (2011) “A Comparison of the Causes, Effects and Aftermaths of the Coastal Flooding of England in 1953 and France in 2010.” Natural Hazards and Earth System Sciences 11: 2321–2333. doi:10.5194/nhess-11-2321-2011.
- Moll, S., S. Bringezu, and H. Schütz. 2005. Resource Use in European Countries – an Estimate of Materials and Waste Streams in the Community, Including Imports and Exports Using the Instrument of Material Flow Analysis. Wuppertal: Wuppertal Institute for Climate, Environment and Energy.
- Moreira, M.J. 2003. “A Conditional Likelihood Ratio Test for Structural Models.” Econometrica: Journal of the Econometric Society 71: 1027–1048.
- Newey, W., and K. West. 1987. “A Simple, Positive Semi-definite, Heteroscedasticity and Autocorrelation Consistent Covariance Matrix.” Econometrica: Journal of the Econometric Society 55: 703–708.
- Nordic Council of Ministers. 2001. Affecting Energy Efficiency: Lessons Learned and Future Prospects. Copenhagen: Nordic Council of Ministers.
- Noy, I., and W. DuPont. 2016. The Long-Term Consequences of Natural Disasters – a Summary of the Literature. Wellington: School of Economics and Finance, Victoria Business School.
- O'Mahony, M., and M.P. Timmer. 2009. “Output, Input and Productivity Measures at the Industry Level: The EU KLEMS Database.” Economics Journal 119: 374–403. doi:10.1111/j.1468-0297.2009.02280.x.
- OECD. 2007. Measuring Material Flows and Resource Productivity – Volume I: The OECD Guide. Paris.
- OECD. 2008. Measuring Material Flows and Resource Productivity - Synthesis Report. Paris.
- OECD. 2011. Towards Green Growth: Monitoring Progress Monitoring the Environmental and Resource Productivity of the Economy. doi:10.1787/9789264111356-9-en
- OECD. 2016. Policy Guidance on Resource Efficiency – OECD Publishing. doi:https://doi.org/10.1787/9789264257344-en.
- Pothen, F., and M. Schymura. 2015. “Bigger Cakes with Fewer Ingredients? A Comparison of Material Use of the World Economy.” Ecological Economics: The Journal of the International Society for Ecological Economics. doi:10.1016/j.ecolecon.2014.10.009.
- Raddatz, C. 2009. “The Wrath of God Macroeconomic Costs of Natural Disasters.” World Bank Policy Research Working Paper 5039. Washington, DC.
- Ramsay, K.W. 2011. “Revisiting the Resource Curse: Natural Disasters, the Price of Oil, and Democracy.” International Organization 65: 507–529. doi:10.1017/S002081831100018X
- Rockström, J., W. Steffen, K. Noone, Å. Persson, F. S. III Chapin, E. Lambin, T.M. Lenton. 2009. “A Safe Operating Space for Humanity.” Nature 461: 472–475. doi:10.1038/461472a.
- Schandl, H., and J. West. 2010. “Resource Use and Resource Efficiency in the Asia–Pacific Region.” Global Environmental Change 20: 636–647. doi:10.1016/j.gloenvcha.2010.06.003.
- Schmalensee, R., T.M. Stoker, and R.A. Judson. 1998. “World Carbon Dioxide Emissions: 1950–2050.” The Review of Economics and Statistics 80: 15–27. doi:10.1162/003465398557294.
- Selden, T.M., and D. Song. 1994. “Environmental Quality and Development: Is There a Kuznets Curve for Air Pollution Emissions?” Journal of Environmental Economics and Management 27: 147–162.
- Shapiro, M.D., and J. Slemrod. 2009. “Did the 2008 Tax Rebates Stimulate Spending?” The American Economic Review 99: 374–279. doi:10.1017/CBO9781107415324.004.
- Steger, S., and R. Bleischwitz. 2011. “Drivers for the Use of Materials Across Countries.” Journal of Cleaner Production 19: 816–826. doi:10.1016/j.jclepro.2010.08.016.
- Steinberger, J.K., F. Krausmann, M. Getzner, H. Schandl, and J. West. 2013. “Development and Dematerialization: An International Study.” PloS One 8: 1–11. doi: 10.1371/journal.pone.0070385
- Teixidó-Figueras, J., and J.A. Duro. 2015. “International Ecological Footprint Inequality: A Methodological Review and Some Results.” Environmental & Resource Economics 60: 607–631. doi:10.1007/s10640-014-9784-x.
- UN. 2015. Transforming Our World: The 2030 Agenda for Sustainable Development – United Nations Sustainable Development Goals. New York, NY. https://sustainabledevelopment.un.org/post2015/transformingourworld
- van der Voet E., L. van Oers, and I. Nikolic. 2005a. “Dematerialization – Not Just a Matter of Weight.” Journal of Industrial Ecology 8: 121–137.
- van der Voet E., L. van Oers, and S. Moll. 2005b. Policy Review on Decoupling: Development of Indicators to Assess Decoupling of Economic Development and Environmental Pressure in the EU-25 and AC-3 Countries.” Brussels: EU Commission, DG Environment.
- Vollebergh, H. R. J., B. Melenberg, and E. Dijkgraaf. 2009. “Identifying Reduced-Form Relations with Panel Data: The Case of Pollution and Income.” Journal of Environmental Economics and Management 58: 27–42. doi:10.1016/j.jeem.2008.12.005.
- Wagner, M. 2015. “The Environmental Kuznets Curve, Cointegration and Nonlinearity.” Journal of Applied Economics 30: 948–967. doi:10.1002/jae.
- Weinzettel, J., and J. Kovanda. 2011. “Structural Decomposition Analysis of Raw Material Consumption.” Journal of Industrial Ecology 15: 893–907. doi:10.1111/j.1530-9290.2011.00378.x.
- Weisz, H., F. Krausmann, C. Amann, et al. 2006. “The Physical Economy of the European Union: Cross-country Comparison and Determinants of Material Consumption.” Ecological Economics: The Journal of the International Society for Ecological Economics 58: 676–698. doi:10.1016/j.ecolecon.2005.08.016.
- Wiedmann, T.O., H. Schandl, D. Moran, et al. 2006. “The Material Footprint of Nations – Reassessing Resource Productivity.” Proceedings of the National Academy of Sciences of the USA 112: 6271–6276.
- World Bank, UN. 2010. Natural Hazards, Unnatural Disasters - The Economics of Effective Prevention. doi: 10.1596/978-0-8213-8050-5
Appendix
Figure A1. ‘Pseudo’ 1000 2SLS regressions for Western Europe: to increase readability, we have limited the range between −40 and 40, thereby excluding 11 coefficients.
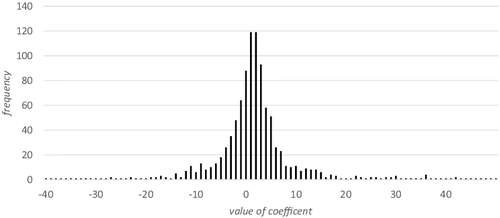
Figure A2. ‘Pseudo’ 1000 2SLS regressions for Eastern Europe: to increase readability, we have limited the range between −40 and 40, thereby excluding 32 coefficients.
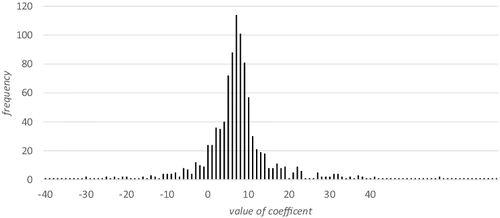
Table A1. Countries grouped into ‘Europe’, ‘Western Europe’, and ‘Eastern Europe’.
Table A2. Statistics on DMC per capita, GDP per capita and the number of storm occurrences in Europe, Western Europe and Eastern Europe between 2000 and 2014.
Table A3. ‘Pseudo’ regression statistics for Western Europe.
Table A4. ‘Pseudo’ regression statistics for Eastern Europe.