ABSTRACT
Soft-tissue sarcomas (STS) are a group of rare, heterogeneous, and aggressive tumors, with high metastatic risk and relatively few efficient systemic therapies. In the quest for new treatments, the immune system represents an attractive therapeutic target. Recently, PD1/PDL1 inhibitors showed very promising results in patients with solid tumors. PDL1 expression has been rarely studied in STS, in small series only, by using immunohistochemistry (IHC), and with non-concordant prognostic implications. Here, we have analyzed PDL1 mRNA expression in 758 clinical STS samples retrospectively profiled using DNA microarrays and RNAseq, and searched for correlations with clinicopathological variables including metastasis-free survival (MFS) after surgery. PDL1 expression was heterogeneous across the samples. PDL1-high samples (41%) were more frequently leiomyosarcomas and liposarcomas, and showed more frequently a complex genetic profile and a high-risk CINSARC signature. No correlation existed with other clinicopathological features such as tumor site, depth, and pathological tumor grade and size. In multivariate prognostic analysis, the PDL1-high class was associated with shorter MFS, independently of the pathological type and the CINSARC signature. Analysis of correlations with biological factors suggested the existence in tumors of the PDL1-high class of a strong and efficient cytotoxic T-cell response, however associated with some degree of T-cell exhaustion and negative regulation. In conclusion, we show that PDL1 expression refines the prediction of metastatic relapse in operated localized STS, and that PD1/PDL1 blockade holds potential to improve patient survival by reactivating inhibited T cells to increase the antitumor immune in PDL1-high tumors.
Abbreviations | ||
AUC | = | area under curve |
CINSARC | = | complexity index in sarcomas |
DC | = | dendritic cells |
FC | = | fold change |
FDR | = | false discovery rate |
FNCLCC | = | Fédération Nationale des Centers de Lutte Contre le Cancer |
GEO | = | gene expression omnibus |
GES | = | gene expression signatures |
GIST | = | gastrointestinal stromal tumor |
GO | = | gene ontology |
HR | = | hazard ratio |
IHC | = | immunohistochemistry |
ISH | = | in situ hybridization |
MFS | = | metastasis-free survival |
MHC | = | major histocompatibility complex |
MPNST | = | malignant peripheral nerve sheath tumor |
NCBI | = | National Center for Biotechnology Information |
NK-cells | = | natural killer cells |
OS | = | overall survival |
PCA | = | principal component analysis |
PD1 | = | programmed cell death 1 |
PDL1 | = | programmed cell death ligand 1 |
qRT-PCR | = | quantitative reverse transcription–polymerase chain reaction |
REMARK | = | recommendations for tumor MARKer |
RMA | = | robust multichip average |
RNAseq | = | RNA sequencing |
ROC | = | receiver operating characteristic |
STS | = | soft-tissue sarcomas |
TILs | = | tumor-infiltrating lymphocytes |
T-reg | = | T-regulator |
Introduction
Soft-tissue sarcomas (STS) are rare tumors, accounting for less than 2% of human cancers, and predominantly arising from the embryonic mesoderm. They represent a heterogeneous group with approximately 50 different pathological subtypes.Citation1 Surgery is the main treatment of early-stage STS in adult patients, but approximately 50% of them will develop post-operative recurrence and die.Citation2 Conventional chemotherapy benefits patients with advanced STS with objective response rates from 15 to 25% with drugs such as doxorubicin, ifosfamide, and dacarbazine. A current paradigm shift favors pathological subtype-specific chemotherapy regimen,Citation3 and recent drug approvals were linked with specific sarcoma subtypes, such as trabectedin for leiomyosarcomas and liposarcomas,Citation4 eribulin for liposarcomas,Citation5 and pazopanib for non-liposarcoma STS.Citation6 However, these drugs represent only marginal rather than radical progressCitation7 with exceptionally complete and durable responses and a median survival of less than 15 mo. Clearly, new treatment options are warranted.
An emerging therapeutic option in oncology is immunotherapy. In sarcomas, several data suggest it is an interesting strategy,Citation8,9 even if our current understanding of the immune response remains limited when compared with other cancers. The role of immunity as a mechanism of cancer therapy was first described in sarcoma patients, whose tumor regressed after an infectionCitation10 or after local injections of streptococcal broth cultures.Citation11 Similar to melanoma or kidney cancer, cases of spontaneous regression were reported in patients with sarcoma.Citation12 Patients with immune deficiencies, such as organ transplant patients, show an increased incidence of sarcomas.Citation13 Lymphocytic infiltration of the tumor was demonstrated in sarcomas, with positive prognostic value in cutaneous angiosarcomas,Citation14 negative prognostic value in STS or liposarcomas,Citation8,15-19 or no prognostic value in MPNST.Citation20 An unfavorable prognostic role of tumor-associated macrophages was reported in leiomyosarcomasCitation21 and myxoid liposarcomas.Citation22 Non-specific cytokines such as interferon and muramyl tripeptide showed clinical efficacy, notably in osteosarcoma.Citation23 More recent immunotherapies showed promising pre-clinical and/or clinical results. For example, sarcomas often express cancer-testis antigens, which provide potential therapeutic targets for active immunization. NY-ESO-1 is expressed in approximately 90% of myxoid liposarcomas and 50% of synovial sarcomas.Citation24 T cells genetically engineered to target NY-ESO-1 expressing synovial sarcoma led to objective responses in four of six patients, including one partial response lasting more than 18 mo.Citation25,26 NK-cell dysfunction has also been reported in STS patients with advanced disease.Citation27 Thus, immunotherapeutic strategies may be promising in sarcoma patients, and all the more for two reasons. First, the biological heterogeneity of sarcomas makes unlikely the identification of a unified molecular pathway that could be exploited to treat a large proportion of them. Second, immunotherapy can better adapt to the emergence of resistant clones than targeted therapies thanks to the high adaptability of the immune response. Finally, the mutational load may be high in sarcomas with complex genetic profile, resulting in neoantigens that can be recognized immunologically.
The inhibition of immune checkpoints such as CTLA4 or PD1 (Programmed cell Death 1) with targeted antibodies has recently emerged as an exciting and effective therapeutic strategy in multiple cancers.Citation28 The PD1 pathway is a key inhibitor of the immune response, regulating the balance between activation and inhibition signals that regulate the activity of tumor-infiltrating lymphocytes (TILs). Disturbance of this balance in cancers favors tumor progression. Activation of PD1, expressed at the surface of immune cells such as T cells, by its ligand PDL1/CD274, expressed by antigen-presenting cells such as macrophages or B cells, but also by cancer cells,Citation29 inhibits lymphocyte activation,Citation30 and promotes T-reg cell development and function, allowing tolerance acquisition or to terminate the immune response. Recently, PD1 and PDL1 inhibitors showed promising antitumor activity with durable response, notably in melanoma and lung, renal and bladder carcinomas,Citation31,32 where a relationship between PDL1 expression on cancer cells and/or TILs and objective response has been evidenced.Citation32-36 Today, these drugs are being investigated in various cancers,Citation37 as well as PDL1 expression and its prognostic and predictive role for therapeutic response.Citation38 In sarcomas, response and stabilization rates reported so far remain of limited magnitude,Citation39-41 but most trials included a mixed bag of histological types without biomarker selection. Identification of candidate patients for PD1/PDL1 inhibitors is therefore a crucial goal.
PDL1 expression has been very rarely studied in STS with to our knowledge, only three published studies,Citation8,15,42 in small series only, by using immunohistochemistry (IHC) with different antibodies, and with non-concordant prognostic implications. Here, we have analyzed PDL1 mRNA expression in 758 clinical STS samples retrospectively profiled using DNA microarrays and RNAseq, and searched for correlations with clinicopathological variables including metastasis-free survival (MFS) after surgery.
Results
Patients' characteristics and PDL1 expression
Gene expression profiles of 758 STS samples, including PDL1 expression level, were available. Their characteristics are summarized in . The sex-ratio was balanced, with 49% of females and 51% of males. The median patients' age was 62 y. The most frequent anatomical sites were extremities, followed by internal trunk, and 89% of cases were deeply seated, below or through the superficial fascia. As expected, the most frequent pathological types included leiomyosarcomas, liposarcomas, and undifferentiated sarcomas. Based on the pathological type, most of the samples were STS with complex genetics. The median pathological tumor size at the time of surgical resection was 10 cm and 83% of the samples had a size superior to 5 cm. Most of the samples were FNCLCC grade 2 or 3, and 55% of them were classified as high-risk according to the CINSARC prognostic signature. As shown in Fig. S1, the PDL1 mRNA expression level varied among these 758 tumors with a range of intensities over three decades in log10 scale, suggesting a heterogeneous expression across STS. Fig. S2 shows the PDL1 mRNA expression levels measured by RNA-Seq in 30 different cancers, including sarcomas, profiled by the TCGA Consortium (http://www.cbioportal.org/).
Table 1. Clinicopathological characteristics of patients and tumors (N = 758).
Correlation of PDL1 expression with metastatic relapse
Follow-up was available for 470 patients, including 134 who experienced a metastatic relapse. The 5-y MFS was 65% (95% CI 60–71; ). Higher PDL1 expression (continuous value) was associated with shorter MFS (p = 2.63E−03, Wald test) with a Hazard Ratio for metastatic relapse of 1.22 (95% CI 1.07–1.38; data not shown). We repeated the analysis using PDL1 expression as a binary variable. To avoid overfitting during discretization, we divided our population in two randomly selected sets designated as learning set and validation set. In the learning set of 235 samples, we defined the optimal expression cut-off associated with the occurrence of metastatic relapse: it resulted into two classes, PDL1-high and PDL1-low, with respective 5-y MFS of 61% (95% CI 50–73) versus 72% (95% CI 63–83, p = 7.75E−03; log-rank test; ). We then confirmed its prognostic robustness in the validation set of 235 samples. The 5-y MFS were 53% (95% CI 43–66) and 71% (95% CI 63–81, p = 3.44E−03; log-rank test) in the PDL1-high vs. PDL1-low classes, respectively (). Thus, out of the 470 pooled samples, 275 were in the PDL1-low class (59%) and 195 in the PDL1-high class (41%). The 5-y MFS was different between the two classes: 72% (95%CI 65–79) in the PDL1-low class and 57% (95%CI 49–66) in the PDL1-high class (p = 1.80E−04, log-rank test; ).
Figure 1. Metastasis-free survival according to PDL1 expression in soft-tissue sarcomas. (A) Kaplan–Meier MFS curves in all patients (N = 470). (B) Similar to (A), but in the learning set (N = 235) and according to PDL1 expression. (C) Similar to (B), but in the validation set (N = 235). (D) Similar to (B), but in the pooled learning and validation sets (N = 470).
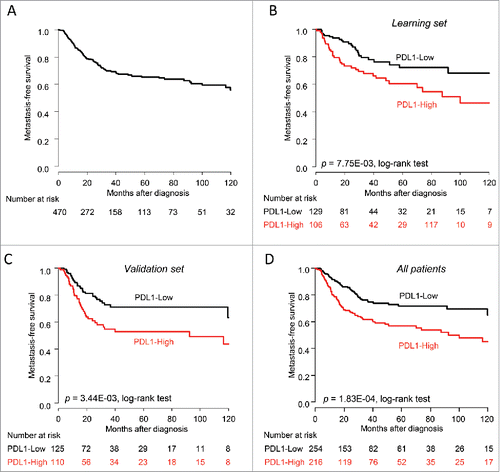
Correlations of PDL1-based classification with clinicopathological variables and prognostic analysis
We searched for correlations between this PDL1-based classification and clinicopathological variables in the 758 samples (). No correlation existed with patients' sex and age, tumor site, tumor grade, size, and depth. By contrast, correlations were found with pathological type, genetic profile, and CINSARC classification, with more leiomyosarcomas and liposarcomas (p = 2.84E−04), more samples with complex genetics (p = 1.82E−04) and with high-risk CINSARC classification (p = 1.87E−04) in the PDL1-high class. PDL1 expression as continuous value was higher in leiomyosarcomas than in all other pathological types (Fig. S3).
Table 2. Correlations of PDL1 expression with clinicopathological variables (N = 758).
In univariate analysis (), patients' sex and age, tumor site, were not associated with MFS, nor the pathological grade and size, and depth likely because of the relatively small number of informative samples. By contrast, the pathological type (p = 3.03E−06), the genetic profile (p = 1.59E−03), the CINSARC classification (p = 1.59E−07), and the PDL1-based classification (p = 2.35E−04) were associated with MFS. In multivariate analysis, the pathological type, the CINSARC classification, and the PDL1-based classification remained significant, whereas the genetic profile did not (). Interestingly, the stratification of patients according to the PDL1-classification and the CINSARC classification identified subgroups with different 5-y MFS (Fig. S4). For example, the PDL1 classification affected the clinical outcome of CINSARC high-risk patients, with shorter 5-y MFS in the PDL1-high class (47%) than in the PDL1-low class (56%; p = 2.71E−02, log-rank test). Similarly, the CINSARC low-risk patients were subdivided into the PDL1-high class with 66% 5-y MFS and the PDL1-low class with 80% 5-y MFS (p = 1.85E−02, log-rank test).
Table 3. Univariate and multivariate prognostic analyses for metastasis-free survival (N = 470).
Correlation of PDL1-based classification with immune features
We searched for correlation between our PDL1-based classification and immunity-related features in the 758 samples. First, the PDL1-high class (N = 302; 42%) was strongly enriched in samples showing high expression of T cells and CD8+ gene signatures described by Palmer et al.Citation43 when compared with the PDL1-low class (N = 456; 58%; p <1.00E−08; ). This profile was confirmed and refined using the Bindea's signatures for immune cell subsets,Citation44 showing a strong enrichment for activated T cells, and notably cytotoxic T cells, Th1-cells, T-helper cells, and Tγδ cells in the PDL1-high class as compared to the PDL1-low class (p < 1.00E−11; Fig. S5). This antitumor activation was also correlated to subsets involved in antigen presentation, such as dendritic cells (DC), B cells, and macrophages (p < 1.00E−07). Second, the PDL1-high class was enriched (p < 1.00E−07; ) in samples showing high expression of the LCKCitation45 and immune kinaseCitation46 signatures, which reflect the cytotoxic T-cell response, although this enrichment was rather low, with only 44% of samples for the LCK signatureCitation45 and 10% for the immune kinase signature.Citation46 Finally, we found that the probability of activation of IFNα, IFNγ, and TNFα pathwaysCitation47 was higher (p < 1.00E−12) in the PDL1-high class than in the PDL1-low class (). Altogether, these results suggested that the PDL1-high samples were associated with transcriptional signs of an efficient antitumor T-cell response.
Figure 2. Correlations of PDL1 expression with immune features in soft-tissue sarcomas. (A) Metagene expression scores in all STS (N = 758) reported as a box plot according to PDL1 expression status for T cells and CD8+ T-cells metagenes and two prognostic immune kinase gene expression signature (LCK and immune kinases). (B) Similar to (A), but showing the probability of activation of immune pathways including IFNα, IFNγ, and TNFα pathways. The p-values (Student's t-test) are indicated.
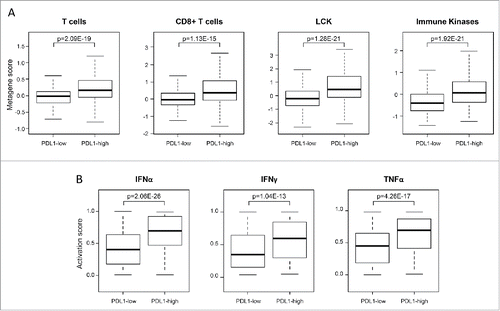
Biological processes associated with PDL1-based classification
To further explore the biological alterations associated with the PDL1-based classification, we compared the whole-genome expression profiles of the PDL1-high (N = 302) and PDL1-low (N = 456) samples. We identified 526 genes differentially expressed, including 419 genes overexpressed and 107 genes underexpressed in the PDL1-high class (Table S1). These genes could be grouped into markers of lymphocytes subsets (CD2, CD3D, CD3E, CD4, CD8A, CD27, CD247, IL2RG, KLRK1, PTPRC ….) or related to pro-cytotoxic T-cells activation and signaling (CD69, DOCK2, EOMES, GBP1-5, ITK, LCK, LCP1, STAT1, TAGAP …) and cytotoxic functions (C1R, C1QA-C, CD52, CD53, FCGR1G, FCGR2A, GZMA, GZMB, GZMK, GZMH, IFNG, IL15, IL2RB, IL2RG, IL7 and IL7R, ISG20, PRF1, TRAF). Consistent with this activation, numerous MHC-related molecules, i.e., involved in the processing of endogenous antigens and presentation to cytotoxic and helper T cells, were also overexpressed. They included not only most HLA-I or HLA-I-related molecules (HLA-A-C, HLA-E-, and -F, …), but also HLA-II molecules (HLA-DR, HLA-DP, HLA-DQ, HLA-DM, HLA-DO, CD74, CIITA…), and molecules involved in the degradation of cytosolic peptides across the endoplasmic reticulum into the membrane-bound compartment where class-I molecules assemble (TAP1, TAPBP, PSMB8-9, CTSS, UBD, …). These two protagonists might probably be recruited within the tumor through the overexpression of chemokine receptors (CCR2, CCR5, CCR7, CXCR3, CXCR6) and chemokines (CCL4, CCL5, CCL8, CCL13, CCL18, CXCL9-11, CXCL13, LTB…). Ontology analysis (Table S2) confirmed the strong involvement of these genes in immune response regulation, and notably in antitumor T-cell activation. Thus, this signature suggested the presence of a strong and efficient cytotoxic response, principally conducted by cytotoxic CD8+ cells, in PDL1-high STS. However, major negative regulators of T-cell immune response were also overexpressed in the PDL1-high samples (HAVCR2, IDO1, LAG3, PDCD1LG2/PDL2, TIGIT…), notably a few hallmark molecules of exhausted T cells (HAVCR2, LAG3, TIGIT).
Discussion
STS are aggressive cancers with limited systemic therapeutic options that could benefit from innovating immunotherapies. Given the promising results of PDL1 inhibitors in different cancers, we analyzed PDL1 expression in 758 previously untreated STS samples and showed that high expression was an independent unfavorable prognostic factor for metastatic relapse. To our knowledge, this is by far the largest study analyzing PDL1 expression in STS.
Our analysis was based on mRNA expression rather than protein expression measured using IHC for several reasons. PDL1 IHC remains challenging today, with different non-standardized protocols. This accounts for the discrepancies of published results discordant and not conclusive, regarding notably the percentage of expression and its prognostic value.Citation48-50 Several antibodies exist but lack specificity and reproducibilityCitation49-52 and the optimal scoring system and positivity cut-off are not defined.Citation53 Thus, the use of different PDL1 IHC assays as a “companion diagnostic” still raises many issues, and efforts for clarification are ongoing. For example, the “Blueprint PD-L1 IHC assay comparison project” was launched last year by a consortium of pharmaceutical companies, diagnostic companies, and academic associationsCitation54 to compare four PD-L1 IHC diagnostic assays developed in conjunction with four PD-1/PD-L1 immune checkpoint inhibitors used in lung cancer clinical trials. This current limitation of IHC led to use alternative methods, such as mRNA analysis based on in situ hybridization (ISH),Citation55 DNA microarrays,Citation56 or qRT-PCR,Citation57 all the more that a positive relationship exists between protein and mRNA expression.Citation50,55,57,58 The mRNA analysis based on DNA microarrays and RNA sequencing allowed not only to avoid the limitations of IHC, but also to work on a large series of samples and to search for associations with other genes.
We showed that PDL1 expression was heterogeneous in STS samples with a wide range of values (three decades on logarithmic scale), providing the opportunity to search for correlations with clinicopathological features. Based on a validated and robust expression cut-off defined upon MFS, we identified two classes of samples, the PDL1-high class representing 41% of cases and the PDL1-low class representing 59%. The choice of cut-off deserves discussion since different calculation methods are used in the literature. Here, we defined a cut-off optimized for separating two patients' classes with different MFS in learning set, and importantly, validated its robustness in a completely independent large validation set. We did not use normal samples, as we did in our breast cancer study,Citation59 because defining the “optimal” normal tissue for STS is more complicated. However, the same cut-off (upregulation defined by Tumor/Normal ratio ≥2 by using the expression of 28 normal connective tissues) identified two STS patients' classes with 93% concordance with our present classification and correlation with MFS (p = 0.05, log-rank test, data not shown). PDL1 expression, as continuous value, was also associated with shorter MFS (p = 2.6 E−03, Wald test, data not shown). No correlation existed between our two PDL1 classes and patients' sex and age, tumor site, depth, and pathological tumor grade and size, but correlations were found with the pathological type, the genetic profile and the CINSARC prognostic classification: the PDL1-high class comprised more leiomyosarcomas and liposarcomas, and more samples with complex genetic profile and with a high-risk CINSARC signature. These associations suggest that PDL1 expression in STS, and more generally the immune microenvironment, may be in part driven by tumor cell-intrinsic features. In univariate prognostic analysis, the pathological type, the genetic profile, the CINSARC classification, and the PDL1-based classification were associated with MFS, whereas in multivariate analysis, all but the genetic profile remained significant. For several decades, efforts have been made to improve the prognostic classification of STS, historically based on clinicopathological features. Different tumor cell-intrinsic molecular prognosticators have been suggested, mainly related to cell cycleCitation60 such as CINSARC.Citation61 The prognostic value of PDL1 expression suggests a role of the immune microenvironment in influencing the clinical outcome of STS patients.
Considering the immunosuppressive function of PDL1, it was not surprising to find high expression associated with shorter survival. This has already been reported in several cancers,Citation15,58,62-66 although a favorable prognostic value has been reported in other cancers.Citation50,55,59,67-69 For comparison and to our knowledge, only four studies—three publicationsCitation8,15,42 and one meeting presentation70—have described PDL1 expression and clinicopathological correlations in STS. All were based on IHC on standard slides or tissue microarrays. Three different PDL1 antibodies were used across the three informative published studies, with different scoring systems and cut-offs. The number of samples remains relatively small when compared to our present series, ranging from 50 to 105 in the three publications and 371 in the meeting presentation. The pathological types represented were numerous (meaning a low number of samples per type), variable across the studies, and sometimes included non-STS samples, with 18 different types (including Ewing sarcoma) in the 105-cases study,Citation15 21 different types (including chondrosarcoma, GIST, solitary fibrous tumor, desmoid tumor, Ewing sarcoma) in the 50-cases study,Citation8 5 different types (including chondrosarcoma, Ewing sarcoma) in the 82-cases study,Citation42 and different types among the 371 STS with genomic complexity in the most recent study.Citation70 Such methodological differences may explain the divergent results in terms of positivity rates and clinicopathological correlations observed across all these studies including ours. In the 105-samples study,Citation15 65% of cases were defined as PDL1-positive (cut-off defined using ROC curve applied to OS); PDL1-positivity was associated with higher tumor stage, deep-seated location, distant metastasis, higher histologic grade, PD1-positive TILs, and shorter overall survival (OS) and event-free survival in uni- and multivariate analyses. In the 50-samples study,Citation8 12% of cases were defined as PDL1-positive (staining >1% tumor cells); PDL1-positivity was not associated with clinicopathological features including OS, but was associated with high-density CD8+ TILs and high-density CD3+ TILs, which were associated with shorter OS. In the 82-samples study,Citation42 43% of cases were defined as PDL1-positive (staining ≥10% tumor cells); PDL1-positivity was not associated with clinicopathological features, except the pathological type, with higher expression in epithelioid sarcoma, followed by synovial sarcoma then rhabdomyosarcoma, which were the only three pathological types of STS represented. Here too, PDL1-positivity was associated with shorter OS in uni- and multivariate analyses. In the meeting presentation,Citation70 19% of cases were defined as PDL1-positive (staining ≥1% tumor and/or immune cells); PDL1-positivity was not associated with relapse or OS. Four additional studies investigated PDL1 expression in a single pathological type of sarcoma. Protein expression was low in a series of 53 malignant peripheral nerve sheath tumors (MPNST) without any correlation with patient outcome.Citation20 In a series of 139 operated imatinib-untreated localized GIST, we found mRNA expression higher in AFIP low-risk and associated with biological signs of cytotoxic T-cell response and longer MFS.Citation68 In a series of 78 chordomas, protein expression was identified in 95% of samples and higher in metastatic than primary tumor samples, and was associated with presence of TILs and shorter OS.Citation16 The same team developed an RNA-based assay to measure PDL1 mRNA expression in a series of 38 osteosarcomas, and found high expression in 24% of samples, positively correlated with TILs with a trend for shorter OS.Citation71 Thus, among the eight studies investigating the potential prognostic value of PDL1 expression in sarcoma samples, four showed negative prognostic value (two in mixed STS, one in chordoma, and one in osteosarcoma) as observed in our present series, whereas three showed no prognostic value (two in mixed STS and one in MPNST), and one showed positive prognostic value (GIST study). In all six studies that addressed the issue, PDL1 expression positively correlated with the density of TILs, as we observed here with the correlation between our PDL1-classification and immune signatures.
If the association of PDL1 expression with shorter MFS is not surprising, it was rather surprising to find simultaneous association with signs of efficient and strong cytotoxic T-cell response, as suggested by the correlation with immune signatures and the results of supervised analysis. In breast cancer and in GISTs, we had previously shown a favorable prognostic value of PDL1 expression,Citation59,68 also associated with a sustained activation of antitumor T-cells, with few transcriptional stigmata of T-cell exhaustion. In these cases, PDL1 was upregulated likely because of a negative feedback loop that follows cytotoxic cells activation, notably through the production of IFNγ, a known regulator of PDL1 expression. In contrast, PDL1 expression was unfavorable in pancreatic carcinoma,Citation66 where expression was associated with a less strong cytotoxic profile than in breast cancer and GISTs and with several signs of T-cell exhaustion, with enrichment in inhibitory molecules and pro-tumor populations (Tregs, myeloid-derived suppressor cells), and downregulation of most MHC class-I members suggesting a defect in antigen presentation. In the present study, the PDL1-high signature suggested a strong cytotoxic T-cell response, without defect in antigen presentation or enrichment in inhibitory molecules and pro-tumor populations, but with some degree of T-cell exhaustion and negative regulation of T-cell response. Indeed, several other immune checkpoints were overexpressed, such as IDO1,Citation72 LAG3,Citation73 HAVCR2/TIM3,Citation74 and TIGIT,Citation75 which represent new targets for new inhibitors under development. In fact, the negative prognostic value of tumor or peri-tumor lymphocyte infiltration in STS has already been reported in several studies,Citation8,15-19 but the explanations of this rather counter-intuitive correlation remain to be defined.
In conclusion, we showed that PDL1 mRNA expression was heterogeneous in STS samples and associated with metastatic relapse independently from the pathological type and the CINSARC classification, suggesting that PDL1 expression cooperates with tumor cell-intrinsic features to influence survival. The strength of our study lies in the great size of the series (the largest reported to date with 758 samples including 470 in the prognostic analysis), its originality (the first one describing PDL1 mRNA expression in STS), the biological and clinical relevance of PDL1 expression and its independent prognostic value. Limitations include its retrospective nature, the heterogeneity with several different pathological types that likely display different intrinsic immunogenicity, the analysis at the mRNA level on whole tissue samples rather than protein level, and the absence of information regarding the cell type (tumor cells and/or TILs) expressing PDL1. Analysis of larger series, retrospective, then prospective, is warranted to confirm our observation and to assess each pathological subtype independently, as well as protein analyses when reliable antibodies are commercially available. IHC analyses on larger series will help confirm that PDL1 is predominantly expressed in tumor cells in STS samples, as previously reported.Citation15 If such prognostic value is confirmed, PDL1 expression might refine the prediction of metastatic relapse and improve our ability to tailor adjuvant chemotherapy. In the metastatic setting, the positive correlation between PDL1 expression and TILs reported in our series and literature suggest that immune checkpoint blockade holds great potential to improve patient survival. The blockade of PDL1 might help to reactivate inhibited T cells to increase the antitumor immune response. Clinical trials are ongoing. Since a relationship between PDL1 protein expression and objective response to PD1/PDL1 inhibitors has been evidenced,Citation32-36 it will be important to test whether PDL1 mRNA expression predicts the clinical response to these drugs. Furthermore, investigating other inhibitor molecules co-expressed with PDL1 such as IDO1, LAG3, HAVCR2/TIM3, and TIGIT may yield additional findings and identify other potential targets for future clinical trials beyond PD1 and PDL1.
Patients and methods
Soft-tissue sarcoma samples
We gathered clinicopathological and gene expression data of clinical STS samples from eight public data sets comprising at least one probe set representing PDL1. Sets and raw data were collected from the National Center for Biotechnology Information (NCBI)/Genbank GEO database and authors' websites (Table S3). Samples were profiled using DNA microarrays (Affymetrix, Agilent, Illumina, and spotted cDNA microarrays)Citation61,76-81 and RNASeq (https://tcga-data.nci.nih.gov). The pooled data set contained a total of 758 STS samples included in the present analysis. The study was approved by our institutional board.
Gene expression data analysis
Data analysis required a step of pre-analytic processing. We first normalized each data set separately, by using quantile normalization for the available processed non-Affymetrix microarray data and Robust Multichip Average (RMA)Citation82 with the non-parametric quantile algorithm for the raw Affymetrix data. Normalization was done in R using Bioconductor and associated packages. We then mapped hybridization probes across the technological platforms represented as previously reported.Citation83 When multiple probes mapped to the same GeneID, we retained the one with the highest variance in each data set. PDL1 (CD274) expression of DNA microarray data sets was measured by analyzing different probe sets whose identity and specificity were verified using the NCBI program BLASTN 2.2.29+ (Table S4). We log2-transformed the available TCGA RNAseq data that were already normalized. Next, we corrected the batch effects through the eight studies using z-score normalization. Briefly, for each expression value in each study separately, all values were transformed by subtracting the mean of the gene in that data set divided by its standard deviation, mean and standard deviation (SD) being measured on leiomyosarcoma samples. Global data set was obtained after concatenation of the eight normalized sets by matching their EntrezGeneID. Principal component analysis (PCA), using the top 6,944 most variable genes (SD>0.75) extracted from the eight pooled data sets, was applied before and after normalization to verify the accuracy of the normalization in removing the set-specific variation in gene expression (Fig. S6).
To define the optimal cut-off of PDL1 expression associated with the occurrence of metastatic relapse, we divided our population of 470 informative samples in two randomly selected sets of 235 samples: the learning set in order to define the cut-off by using a ROC curve (cut-off at the highest AUC value) and the validation set in order to validate it in independent samples. Once validated, the cut-off was applied to all samples to define the PDL1-low class (expression level inferior to the cut-off) and the PDL1-high class (expression level superior or equal to the cut-off). Because of the role of PDL1 in immunity, we searched for correlations of our PDL1-based classification with immune gene expression signatures. We thus applied several multigene signatures to each tumor in each data set separately, including metagenes associated with immune cell populations such as T cells, CD8+ T cells, and B cells defined by Palmer et al.Citation43 transcriptional signatures of 24 different innate and adaptative immune cell subpopulations defined by Bindea et al.Citation44 two prognostic signatures reflecting the cytotoxic T-cell response including the “LCK signature,”Citation45 and the “kinase immune” signature,Citation46 and signatures of immune pathway activity such as IFNα, IFNγ, and TNFα pathways.Citation47 Finally, to explore the biological pathways associated with our PDL1-based classification, we applied a supervised analysis to the whole-genome profiles of 758 samples to search for genes differentially expressed between the PDL1-high vs. PDL1-low classes. We used a moderated t-test with empirical Bayes statistic included in the limma R packages. False discovery rate (FDR)Citation84 was applied to correct the multiple testing hypothesis and significant genes were defined by the following thresholds: p < 5%, q <25% and fold change (FC) superior to |1.5x|. Ontology analysis of the resulting gene list was based on GO biological processes of the Database for Annotation, Visualization and Integrated Discovery (DAVID; http://david.abcc.ncifcrf.gov/).
Statistical analysis
The primary endpoint was the metastasis-free survival (MFS) calculated from the date of diagnosis until the date of distant relapse or death from STS. The follow-up was measured from the date of diagnosis to the date of last news for event-free patients. Survival was calculated using the Kaplan–Meier method and curves were compared with the log-rank test. Correlations between the PDL1-classes (low vs. high) and the clinicopathological factors were calculated with the Student's t-test for the continuous variables and the Fisher's exact test for the binary variables. Univariate and multivariate analyses were done using Cox regression analysis (Wald test). The variables tested in univariate analysis included the PDL1-based classification (low vs. high), patients' age and sex, pathological type, grade, tumor size, depth, tumor site, genetic profiles (complex vs. simplex), and the CINSARC classificationCitation61 (poor vs. good risk). Multivariate analysis incorporated all variables with a p-value inferior to 5% in univariate analysis. All statistical tests were two-sided at the 5% level of significance. Statistical analysis was done using the survival package (version 2.30) in the R software (version 2.9.1). The paper was written in accordance with reporting recommendations for tumor marker prognostic studies (REMARK) criteria.Citation85
Disclosure of potential conflicts of interest
No potential conflicts of interest were disclosed.
supplementary_materials.zip
Download Zip (1.2 MB)Funding
Our work was supported by Institut Paoli-Calmettes, Inserm, Institut National du Cancer, and la Ligue Nationale contre le Cancer.
References
- Wunder JS, Nielsen TO, Maki RG, O'Sullivan B, Alman BA. Opportunities for improving the therapeutic ratio for patients with sarcoma. Lancet Oncol 2007; 8:513-24; PMID:17540303; http://dx.doi.org/10.1016/S1470-2045(07)70169-9
- Group ESESNW. Soft tissue and visceral sarcomas: ESMO clinical practice guidelines for diagnosis, treatment and follow-up. Ann Oncol 2014; 25 Suppl 3:iii102-12; PMID:25210080; http://dx.doi.org/10.1093/annonc/mdu254
- Pang A, Carbini M, Maki RG. Contemporary therapy for advanced soft-tissue sarcomas in adults: a review. JAMA 0ncol 2016; 2:941-7; PMID:27148906; http://dx.doi.org/10.1001/jamaoncol.2016.0241
- Demetri GD, von Mehren M, Jones RL, Hensley ML, Schuetze SM, Staddon A, Milhem M, Elias A, Ganjoo K, Tawbi H et al. Efficacy and safety of trabectedin or dacarbazine for metastatic liposarcoma or leiomyosarcoma after failure of conventional chemotherapy: results of a phase III randomized multicenter clinical trial. J Clin Oncol 2016; 34:786-93; PMID:26371143; http://dx.doi.org/10.1200/JCO.2015.62.4734
- Schoffski P, Chawla S, Maki RG, Italiano A, Gelderblom H, Choy E, Grignani G, Camargo V, Bauer S, Rha SY et al. Eribulin versus dacarbazine in previously treated patients with advanced liposarcoma or leiomyosarcoma: a randomised, open-label, multicentre, phase 3 trial. Lancet 2016; 387:1629-37; PMID:26874885; http://dx.doi.org/10.1016/S0140-6736(15)01283-0
- van der Graaf WT, Blay JY, Chawla SP, Kim DW, Bui-Nguyen B, Casali PG, Schoffski P, Aglietta M, Staddon AP, Beppu Y et al. Pazopanib for metastatic soft-tissue sarcoma (PALETTE): a randomised, double-blind, placebo-controlled phase 3 trial. Lancet 2012; 379:1879-86; PMID:22595799; http://dx.doi.org/10.1016/S0140-6736(12)60651-5
- Ratan R, Patel SR. Chemotherapy for soft tissue sarcoma. Cancer 2016; 122:2952-60; PMID:27434055; http://dx.doi.org/10.1002/cncr.30191
- D'Angelo SP, Shoushtari AN, Agaram NP, Kuk D, Qin LX, Carvajal RD, Dickson MA, Gounder M, Keohan ML, Schwartz GK et al. Prevalence of tumor-infiltrating lymphocytes and PD-L1 expression in the soft tissue sarcoma microenvironment. Hum Pathol 2015; 46:357-65; PMID:25540867; http://dx.doi.org/10.1016/j.humpath.2014.11.001
- Hu JS, Skeate JG, Kast WM, Wong MK. Immunotherapy in sarcoma: a brief review. Sarcoma Res Int 2014; 1:1-8.
- Coley WB II. Contribution to the knowledge of sarcoma. Ann Surg 1891; 14:199-220; PMID:17859590; http://dx.doi.org/10.1097/00000658-189112000-00015
- Wiemann B, Starnes CO. Coley's toxins, tumor necrosis factor and cancer research: a historical perspective. Pharmacol Ther 1994; 64:529-64; PMID:7724661; http://dx.doi.org/10.1016/0163-7258(94)90023-X
- Sakamoto A, Shiba E, Hisaoka M. Short-term spontaneous regression of myxofibrosarcoma in the scapular region. Skeletal Radiol 2014; 43:1487-90; PMID:24910124; http://dx.doi.org/10.1007/s00256-014-1914-6
- Penn I. Sarcomas in organ allograft recipients. Transplantation 1995; 60:1485-91; PMID:8545879; http://dx.doi.org/10.1097/00007890-199560120-00020
- Fujii H, Arakawa A, Utsumi D, Sumiyoshi S, Yamamoto Y, Kitoh A, Ono M, Matsumura Y, Kato M, Konishi K et al. CD8(+) tumor-infiltrating lymphocytes at primary sites as a possible prognostic factor of cutaneous angiosarcoma. Int J Cancer 2014; 134:2393-402; PMID:24243586; http://dx.doi.org/10.1002/ijc.28581
- Kim JR, Moon YJ, Kwon KS, Bae JS, Wagle S, Kim KM, Park HS, Lee H, Moon WS, Chung MJ et al. Tumor infiltrating PD1-positive lymphocytes and the expression of PD-L1 predict poor prognosis of soft tissue sarcomas. PLoS One 2013; 8:e82870; PMID:24349382; http://dx.doi.org/10.1371/journal.pone.0082870
- Feng Y, Shen J, Gao Y, Liao Y, Cote G, Choy E, Chebib I, Mankin H, Hornicek F, Duan Z. Expression of programmed cell death ligand 1 (PD-L1) and prevalence of tumor-infiltrating lymphocytes (TILs) in chordoma. Oncotarget 2015; 6:11139-49; PMID:25871477; http://dx.doi.org/10.18632/oncotarget.3576
- Sorbye SW, Kilvaer T, Valkov A, Donnem T, Smeland E, Al-Shibli K, Bremnes RM, Busund LT. Prognostic impact of lymphocytes in soft tissue sarcomas. PLoS One 2011; 6:e14611; PMID:21298041; http://dx.doi.org/10.1371/journal.pone.0014611
- Sorbye SW, Kilvaer TK, Valkov A, Donnem T, Smeland E, Al-Shibli K, Bremnes RM, Busund LT. Prognostic impact of peritumoral lymphocyte infiltration in soft tissue sarcomas. BMC Clin Pathol 2012; 12:5; PMID:22375962; http://dx.doi.org/10.1186/1472-6890-12-5
- Tseng WW, Malu S, Zhang M, Chen J, Sim GC, Wei W, Ingram D, Somaiah N, Lev DC, Pollock RE et al. Analysis of the intratumoral adaptive immune response in well differentiated and dedifferentiated retroperitoneal liposarcoma. Sarcoma 2015; 2015:547460; PMID:25705114; http://dx.doi.org/10.1155/2015/547460
- Shurell E, Singh AS, Crompton JG, Jensen S, Li Y, Dry S, Nelson S, Chmielowski B, Bernthal N, Federman N et al. Characterizing the immune microenvironment of malignant peripheral nerve sheath tumor by PD-L1 expression and presence of CD8+ tumor infiltrating lymphocytes. Oncotarget 2016; 7(39):64300-8; PMID:27588404; http://dx.doi.org/10.18632/oncotarget.11734
- Lee CH, Espinosa I, Vrijaldenhoven S, Subramanian S, Montgomery KD, Zhu S, Marinelli RJ, Peterse JL, Poulin N, Nielsen TO et al. Prognostic significance of macrophage infiltration in leiomyosarcomas. Clin Cancer Res 2008; 14:1423-30; PMID:18316565; http://dx.doi.org/10.1158/1078-0432.CCR-07-1712
- Nabeshima A, Matsumoto Y, Fukushi J, Iura K, Matsunobu T, Endo M, Fujiwara T, Iida K, Fujiwara Y, Hatano M et al. Tumour-associated macrophages correlate with poor prognosis in myxoid liposarcoma and promote cell motility and invasion via the HB-EGF-EGFR-PI3K/Akt pathways. Br J Cancer 2015; 112:547-55; PMID:25562433; http://dx.doi.org/10.1038/bjc.2014.637
- Meyers PA, Schwartz CL, Krailo MD, Healey JH, Bernstein ML, Betcher D, Ferguson WS, Gebhardt MC, Goorin AM, Harris M et al. Osteosarcoma: the addition of muramyl tripeptide to chemotherapy improves overall survival–a report from the children's oncology group. J Clin Oncol 2008; 26:633-8; PMID:18235123; http://dx.doi.org/10.1200/JCO.2008.14.0095
- Lai JP, Rosenberg AZ, Miettinen MM, Lee CC. NY-ESO-1 expression in sarcomas: A diagnostic marker and immunotherapy target. Oncoimmunology 2012; 1:1409-10; PMID:23243610; http://dx.doi.org/10.4161/onci.21059
- Robbins PF, Kassim SH, Tran TL, Crystal JS, Morgan RA, Feldman SA, Yang JC, Dudley ME, Wunderlich JR, Sherry RM et al. A pilot trial using lymphocytes genetically engineered with an NY-ESO-1-reactive T-cell receptor: long-term follow-up and correlates with response. Clin Cancer Res 2015; 21:1019-27; PMID:25538264; http://dx.doi.org/10.1158/1078-0432.CCR-14-2708
- Robbins PF, Morgan RA, Feldman SA, Yang JC, Sherry RM, Dudley ME, Wunderlich JR, Nahvi AV, Helman LJ, Mackall CL et al. Tumor regression in patients with metastatic synovial cell sarcoma and melanoma using genetically engineered lymphocytes reactive with NY-ESO-1. J Clin Oncol 2011; 29:917-24; PMID:21282551; http://dx.doi.org/10.1200/JCO.2010.32.2537
- Bucklein V, Adunka T, Mendler AN, Issels R, Subklewe M, Schmollinger JC, Noessner E. Progressive natural killer cell dysfunction associated with alterations in subset proportions and receptor expression in soft-tissue sarcoma patients. Oncoimmunology 2016; 5:e1178421; PMID:27622032; http://dx.doi.org/10.1080/2162402X.2016.1178421
- Zitvogel L, Kroemer G. Targeting PD-1/PD-L1 interactions for cancer immunotherapy. Oncoimmunology 2012; 1:1223-5; PMID:23243584; http://dx.doi.org/10.4161/onci.21335
- Gatalica Z, Snyder C, Maney T, Ghazalpour A, Holterman DA, Xiao N, Overberg P, Rose I, Basu GD, Vranic S et al. Programmed cell death 1 (PD-1) and its ligand (PD-L1) in common cancers and their correlation with molecular cancer type. Cancer Epidemiol Biomarkers Prev 2014; 23(12):2965-70; PMID:25392179; http://dx.doi.org/10.1158/1055-9965.EPI-14-0654
- Amarnath S, Mangus CW, Wang JC, Wei F, He A, Kapoor V, Foley JE, Massey PR, Felizardo TC, Riley JL et al. The PDL1-PD1 axis converts human TH1 cells into regulatory T cells. Science Transl Med 2011; 3:111ra20; PMID:22133721; http://dx.doi.org/10.1126/scitranslmed.3003130
- Brahmer JR, Tykodi SS, Chow LQ, Hwu WJ, Topalian SL, Hwu P, Drake CG, Camacho LH, Kauh J, Odunsi K et al. Safety and activity of anti-PD-L1 antibody in patients with advanced cancer. N Engl J Med 2012; 366:2455-65; PMID:22658128; http://dx.doi.org/10.1056/NEJMoa1200694
- Topalian SL, Hodi FS, Brahmer JR, Gettinger SN, Smith DC, McDermott DF, Powderly JD, Carvajal RD, Sosman JA, Atkins MB et al. Safety, activity, and immune correlates of anti-PD-1 antibody in cancer. N Engl J Med 2012; 366:2443-54; PMID:22658127; http://dx.doi.org/10.1056/NEJMoa1200690
- Taube JM, Klein A, Brahmer JR, Xu H, Pan X, Kim JH, Chen L, Pardoll DM, Topalian SL, Anders RA. Association of PD-1, PD-1 Ligands, and Other Features of the Tumor Immune Microenvironment with Response to Anti-PD-1 Therapy. Clin Cancer Res 2014; 20:5064-74; PMID:24714771; http://dx.doi.org/10.1158/1078-0432.CCR-13-3271
- Herbst RS, Baas P, Kim DW, Felip E, Perez-Gracia JL, Han JY, Molina J, Kim JH, Arvis CD, Ahn MJ et al. Pembrolizumab versus docetaxel for previously treated, PD-L1-positive, advanced non-small-cell lung cancer (KEYNOTE-010): a randomised controlled trial. Lancet 2016; 387:1540-50; PMID:26712084; http://dx.doi.org/10.1016/S0140-6736(15)01281-7
- Herbst RS, Soria JC, Kowanetz M, Fine GD, Hamid O, Gordon MS, Sosman JA, McDermott DF, Powderly JD, Gettinger SN et al. Predictive correlates of response to the anti-PD-L1 antibody MPDL3280A in cancer patients. Nature 2014; 515:563-7; PMID:25428504; http://dx.doi.org/10.1038/nature14011
- Powles T, Eder JP, Fine GD, Braiteh FS, Loriot Y, Cruz C, Bellmunt J, Burris HA, Petrylak DP, Teng SL et al. MPDL3280A (anti-PD-L1) treatment leads to clinical activity in metastatic bladder cancer. Nature 2014; 515:558-62; PMID:25428503; http://dx.doi.org/10.1038/nature13904
- Bertucci F, Finetti P, Birnbaum D, Mamessier E. The PD1/PDL1 axis, a promising therapeutic target in aggressive breast cancers. Oncoimmunology 2016; 5:e1085148; PMID:27141340; http://dx.doi.org/10.1080/2162402X.2015.1085148
- Zou W, Wolchok JD, Chen L. PD-L1 (B7-H1) and PD-1 pathway blockade for cancer therapy: Mechanisms, response biomarkers, and combinations. Science Transl Med 2016; 8:328rv4; PMID:26936508; http://dx.doi.org/10.1126/scitranslmed.aad7118
- George S, Barysauskas CM, Solomon S, Tahlil K, Malley R, Hohos M, Polson K, Loucks M, Wagner AJ, Merriam P et al. Phase 2 study of nivolumab in metastatic leiomyosarcoma of the uterus. J Clin Oncol 2016; 34: (suppl; abstr 11007).
- Paoluzzi L, Ghesani MV, Cacavio A, Rapkiewicz A, Rosen G. Anti-PD1 therapy with nivolumab in sarcoma. J Clin Oncol 2016; 34 (suppl; abstr 11047).
- Tawbi HA, Burgess MA, Crowley J, Van Tine BA, Hu J, Schuetze S, D'Angelo SP, Attia S, Priebat DA, Okuno SH et al. Safety and efficacy of PD-1 blockade using pembrolizumab in patients with advanced soft tissue (STS) and bone sarcomas (BS): results of SARC028—A multicenter phase II study. J Clin Oncol 2016; 34 (suppl; abstr 11006).
- Kim C, Kim EK, Jung H, Chon HJ, Han JW, Shin KH, Hu H, Kim KS, Choi YD, Kim S et al. Prognostic implications of PD-L1 expression in patients with soft tissue sarcoma. BMC Cancer 2016; 16:434; PMID:27393385; http://dx.doi.org/10.1186/s12885-016-2451-6
- Palmer C, Diehn M, Alizadeh AA, Brown PO. Cell-type specific gene expression profiles of leukocytes in human peripheral blood. BMC Genomics 2006; 7:115; PMID:16704732; http://dx.doi.org/10.1186/1471-2164-7-115
- Bindea G, Mlecnik B, Tosolini M, Kirilovsky A, Waldner M, Obenauf AC, Angell H, Fredriksen T, Lafontaine L, Berger A et al. Spatiotemporal dynamics of intratumoral immune cells reveal the immune landscape in human cancer. Immunity 2013; 39:782-95; PMID:24138885; http://dx.doi.org/10.1016/j.immuni.2013.10.003
- Rody A, Holtrich U, Pusztai L, Liedtke C, Gaetje R, Ruckhaeberle E, Solbach C, Hanker L, Ahr A, Metzler D et al. T-cell metagene predicts a favorable prognosis in estrogen receptor-negative and HER2-positive breast cancers. Breast Cancer Res 2009; 11:R15; PMID:19272155; http://dx.doi.org/10.1186/bcr2234
- Sabatier R, Finetti P, Mamessier E, Raynaud S, Cervera N, Lambaudie E, Jacquemier J, Viens P, Birnbaum D, Bertucci F. Kinome expression profiling and prognosis of basal breast cancers. Mol Cancer 2011; 10:86; PMID:21777462; http://dx.doi.org/10.1186/1476-4598-10-86
- Gatza ML, Lucas JE, Barry WT, Kim JW, Wang Q, Crawford MD, Datto MB, Kelley M, Mathey-Prevot B, Potti A et al. A pathway-based classification of human breast cancer. Proc Natl Acad Sci U S A 2010; 107:6994-9; PMID:20335537; http://dx.doi.org/10.1073/pnas.0912708107
- Chakravarti N, Prieto VG. Predictive factors of activity of anti-programmed death-1/programmed death ligand-1 drugs: immunohistochemistry analysis. Transl Lung Cancer Res 2015; 4:743-51; PMID:26798583; http://dx.doi.org/10.3978/j.issn.2218-6751.2015.12.10
- Rimm D, Schalper K, Pusztai L. Unvalidated antibodies and misleading results. Breast Cancer Res Treat 2014; 147:457-8; PMID:25086631; http://dx.doi.org/10.1007/s10549-014-3061-0
- Velcheti V, Schalper KA, Carvajal DE, Anagnostou VK, Syrigos KN, Sznol M, Herbst RS, Gettinger SN, Chen L, Rimm DL. Programmed death ligand-1 expression in non-small cell lung cancer. Lab Invest 2014; 94:107-16; PMID:24217091; http://dx.doi.org/10.1038/labinvest.2013.130
- Gadiot J, Hooijkaas AI, Kaiser AD, van Tinteren H, van Boven H, Blank C. Overall survival and PD-L1 expression in metastasized malignant melanoma. Cancer 2011; 117:2192-201; PMID:21523733; http://dx.doi.org/10.1002/cncr.25747
- McLaughlin J, Han G, Schalper KA, Carvajal-Hausdorf D, Pelekanou V, Rehman J, Velcheti V, Herbst R, LoRusso P, Rimm DL. Quantitative assessment of the heterogeneity of PD-L1 expression in non-small-cell lung cancer. JAMA Oncol 2016; 2:46-54; PMID:26562159; http://dx.doi.org/10.1001/jamaoncol.2015.3638
- Ilie M, Hofman V, Dietel M, Soria JC, Hofman P. Assessment of the PD-L1 status by immunohistochemistry: challenges and perspectives for therapeutic strategies in lung cancer patients. Virchows Arch 2016; 468:511-25; PMID:26915032; http://dx.doi.org/10.1007/s00428-016-1910-4
- Sholl LM, Aisner DL, Allen TC, Beasley MB, Borczuk AC, Cagle PT, Capelozzi V, Dacic S, Hariri L, Kerr KM et al. Programmed death ligand-1 immunohistochemistry–a new challenge for pathologists: a perspective from members of the pulmonary pathology society. Arch Pathol Lab Med 2016; 140:341-4; PMID:26780537; http://dx.doi.org/10.5858/arpa.2015-0506-SA
- Schalper KA, Velcheti V, Carvajal D, Wimberly H, Brown J, Pusztai L, Rimm DL. In situ tumor PD-L1 mRNA expression is associated with increased TILs and better outcome in breast carcinomas. Clin Cancer Res 2014; 20:2773-82; PMID:24647569; http://dx.doi.org/10.1158/1078-0432.CCR-13-2702
- Soliman H, Khalil F, Antonia S. PD-L1 expression is increased in a subset of basal type breast cancer cells. PLoS One 2014; 9:e88557; PMID:24551119; http://dx.doi.org/10.1371/journal.pone.0088557
- Shen JK, Cote GM, Choy E, Yang P, Harmon D, Schwab J, Nielsen GP, Chebib I, Ferrone S, Wang X et al. Programmed cell death ligand 1 expression in osteosarcoma. Cancer Immunol Res 2014; 2:690-8; PMID:24866169; http://dx.doi.org/10.1158/2326-6066.CIR-13-0224
- Ohigashi Y, Sho M, Yamada Y, Tsurui Y, Hamada K, Ikeda N, Mizuno T, Yoriki R, Kashizuka H, Yane K et al. Clinical significance of programmed death-1 ligand-1 and programmed death-1 ligand-2 expression in human esophageal cancer. Clin Cancer Res 2005; 11:2947-53; PMID:15837746; http://dx.doi.org/10.1158/1078-0432.CCR-04-1469
- Sabatier R, Finetti P, Mamessier E, Adelaide J, Chaffanet M, Ali HR, Viens P, Caldas C, Birnbaum D, Bertucci F. Prognostic and predictive value of PDL1 expression in breast cancer. Oncotarget 2015; 6:5449-64; PMID:25669979; http://dx.doi.org/10.18632/oncotarget.3216
- Ottaiano A, De Chiara A, Fazioli F, Talamanca AA, Mori S, Botti G, Milano A, Apice G. Biological prognostic factors in adult soft tissue sarcomas. Anticancer Res 2005; 25:4519-26; PMID:16334136
- Chibon F, Lagarde P, Salas S, Perot G, Brouste V, Tirode F, Lucchesi C, de Reynies A, Kauffmann A, Bui B et al. Validated prediction of clinical outcome in sarcomas and multiple types of cancer on the basis of a gene expression signature related to genome complexity. Nat Med 2010; 16:781-87; PMID:20581836; http://dx.doi.org/10.1038/nm.2174
- Choueiri TK, Fay AP, Gray KP, Callea M, Ho TH, Albiges L, Bellmunt J, Song J, Carvo I, Lampron M et al. PD-L1 expression in nonclear-cell renal cell carcinoma. Ann Oncol 2014; 25:2178-84; PMID:25193987; http://dx.doi.org/10.1093/annonc/mdu445
- Kiyasu J, Miyoshi H, Hirata A, Arakawa F, Ichikawa A, Niino D, Sugita Y, Yufu Y, Choi I, Abe Y et al. Expression of programmed cell death ligand 1 is associated with poor overall survival in patients with diffuse large B-cell lymphoma. Blood 2015; 126:2193-201; PMID:26239088; http://dx.doi.org/10.1182/blood-2015-02-629600
- Massi D, Brusa D, Merelli B, Ciano M, Audrito V, Serra S, Buonincontri R, Baroni G, Nassini R, Minocci D et al. PD-L1 marks a subset of melanomas with a shorter overall survival and distinct genetic and morphological characteristics. Ann Oncol 2014; 25:2433-42; PMID:25223485; http://dx.doi.org/10.1093/annonc/mdu452
- Thompson RH, Kuntz SM, Leibovich BC, Dong H, Lohse CM, Webster WS, Sengupta S, Frank I, Parker AS, Zincke H et al. Tumor B7-H1 is associated with poor prognosis in renal cell carcinoma patients with long-term follow-up. Cancer Res 2006; 66:3381-5; PMID:16585157; http://dx.doi.org/10.1158/0008-5472.CAN-05-4303
- Birnbaum DJ, Finetti P, Lopresti A, Gilabert M, Poizat F, Turrini O, Raoul JL, Delpero JR, Moutardier V, Birnbaum D et al. Prognostic value of PDL1 expression in pancreatic cancer. Oncotarget 2016; PMID:27589570; http://dx.doi.org/10.18632/oncotarget.11685
- Droeser RA, Hirt C, Viehl CT, Frey DM, Nebiker C, Huber X, Zlobec I, Eppenberger-Castori S, Tzankov A, Rosso R et al. Clinical impact of programmed cell death ligand 1 expression in colorectal cancer. Eur J Cancer 2013; 49:2233-42; PMID:23478000; http://dx.doi.org/10.1016/j.ejca.2013.02.015
- Bertucci F, Finetti P, Mamessier E, Pantaleo MA, Astolfi A, Ostrowski J, Birnbaum D. PDL1 expression is an independent prognostic factor in localized GIST. Oncoimmunology 2015; 4(5):e1002729; PMID:26155391; http://dx.doi.org/10.1080/2162402X.2014.1002729
- Lipson EJ, Vincent JG, Loyo M, Kagohara LT, Luber BS, Wang H, Xu H, Nayar SK, Wang TS, Sidransky D et al. PD-L1 expression in the Merkel cell carcinoma microenvironment: association with inflammation, Merkel cell polyomavirus and overall survival. Cancer Immunol Res 2013; 1:54-63; PMID:24416729; http://dx.doi.org/10.1158/2326-6066.CIR-13-0034
- Toulmonde M, Adam J, Bessede A, Ranchere-Vince D, Velasco V, Brouste V, Blay JY, Mir O, Italiano A. Integrative assessment of expression and prognostic value of PDL1, IDO, and kynurenine in 371 primary soft tissue sarcomas with genomic complexity. J Clin Oncol 2016; 34:(suppl; abstr 11008) 2016 ASCO Annual Meeting.
- Shen JK, Cote GM, Choy E, Hornicek FJ, Duan Z. Targeting programmed cell death ligand 1 in osteosarcoma: an auto-commentary on therapeutic potential. Oncoimmunology 2014; 3:e954467; PMID:25610746; http://dx.doi.org/10.4161/21624011.2014.954467
- Vacchelli E, Aranda F, Eggermont A, Sautes-Fridman C, Tartour E, Kennedy EP, Platten M, Zitvogel L, Kroemer G, Galluzzi L. Trial watch: IDO inhibitors in cancer therapy. Oncoimmunology 2014; 3:e957994; PMID:25941578; http://dx.doi.org/10.4161/21624011.2014.957994
- He Y, Rivard CJ, Rozeboom L, Yu H, Ellison K, Kowalewski A, Zhou C, Hirsch FR. Lymphocyte-activation gene-3, an important immune checkpoint in cancer. Cancer Sci 2016; 107:1193-7; PMID:27297395; http://dx.doi.org/10.1111/cas.12986
- Anderson AC, Joller N, Kuchroo VK. Lag-3, Tim-3, and TIGIT: Co-inhibitory receptors with specialized functions in immune regulation. Immunity 2016; 44:989-1004; PMID:27192565; http://dx.doi.org/10.1016/j.immuni.2016.05.001
- Kurtulus S, Sakuishi K, Ngiow SF, Joller N, Tan DJ, Teng MW, Smyth MJ, Kuchroo VK, Anderson AC. TIGIT predominantly regulates the immune response via regulatory T cells. J Clin Invest 2015; 125:4053-62; PMID:26413872; http://dx.doi.org/10.1172/JCI81187
- Nielsen TO, West RB, Linn SC, Alter O, Knowling MA, O'Connell JX, Zhu S, Fero M, Sherlock G, Pollack JR et al. Molecular characterisation of soft tissue tumours: a gene expression study. Lancet 2002; 359:1301-7; PMID:11965276; http://dx.doi.org/10.1016/S0140-6736(02)08270-3
- West RB, Nuyten DS, Subramanian S, Nielsen TO, Corless CL, Rubin BP, Montgomery K, Zhu S, Patel R, Hernandez-Boussard T et al. Determination of stromal signatures in breast carcinoma. PLoS Biol 2005; 3:e187; PMID:15869330; http://dx.doi.org/10.1371/journal.pbio.0030187
- Beck AH, Lee CH, Witten DM, Gleason BC, Edris B, Espinosa I, Zhu S, Li R, Montgomery KD, Marinelli RJ et al. Discovery of molecular subtypes in leiomyosarcoma through integrative molecular profiling. Oncogene 2010; 29:845-54; PMID:19901961; http://dx.doi.org/10.1038/onc.2009.381
- Ylipaa A, Hunt KK, Yang J, Lazar AJ, Torres KE, Lev DC, Nykter M, Pollock RE, Trent J, Zhang W. Integrative genomic characterization and a genomic staging system for gastrointestinal stromal tumors. Cancer 2011; 117:380-9; PMID:20818650; http://dx.doi.org/10.1002/cncr.25594
- Gibault L, Perot G, Chibon F, Bonnin S, Lagarde P, Terrier P, Coindre JM, Aurias A. New insights in sarcoma oncogenesis: a comprehensive analysis of a large series of 160 soft tissue sarcomas with complex genomics. J Pathol 2011; 223:64-71; PMID:21125665; http://dx.doi.org/10.1002/path.2787
- Renner M, Wolf T, Meyer H, Hartmann W, Penzel R, Ulrich A, Lehner B, Hovestadt V, Czwan E, Egerer G et al. Integrative DNA methylation and gene expression analysis in high-grade soft tissue sarcomas. Genome Biol 2013; 14:r137; PMID:24345474; http://dx.doi.org/10.1186/gb-2013-14-12-r137
- Irizarry RA, Hobbs B, Collin F, Beazer-Barclay YD, Antonellis KJ, Scherf U, Speed TP. Exploration, normalization, and summaries of high density oligonucleotide array probe level data. Biostatistics 2003; 4:249-64; PMID:12925520; http://dx.doi.org/10.1093/biostatistics/4.2.249
- Bertucci F, Finetti P, Viens P, Birnbaum D. EndoPredict predicts for the response to neoadjuvant chemotherapy in ER-positive, HER2-negative breast cancer. Cancer Lett 2014; 355:70-5; PMID:25218596; http://dx.doi.org/10.1016/j.canlet.2014.09.014
- Hochberg Y, Benjamini Y. More powerful procedures for multiple significance testing. Stat Med 1990; 9:811-8; PMID:2218183; http://dx.doi.org/10.1002/sim.4780090710
- McShane LM, Altman DG, Sauerbrei W, Taube SE, Gion M, Clark GM, Statistics Subcommittee of the NCIEWGoCD. Reporting recommendations for tumour marker prognostic studies (REMARK). Br J Cancer 2005; 93:387-91; PMID:16106245; http://dx.doi.org/10.1038/sj.bjc.6602678