ABSTRACT
Multiple soluble factors including proteins (in particular chemokines), non-proteinaceous factors released by dead cells, as well as receptors for such factors (in particular chemokine receptors, formyl peptide receptors and purinergic receptors), influence the recruitment of distinct cell subsets into the tumor microenvironment. We performed an extensive bioinformatic analysis on tumor specimens from 5953 cancer patients to correlate the mRNA expression levels of chemotactic factors/receptors with the density of immune cell types infiltrating the malignant lesions. This meta-analysis, which included specimens from breast, colorectal, lung, ovary and head and neck carcinomas as well as melanomas, revealed that a subset of chemotactic factors/receptors exhibited a positive and reproducible correlation with several infiltrating cell types across various solid cancers, revealing a universal pattern of association. Hence, this meta-analysis distinguishes between homogeneous associations that occur across different cancer types and heterogeneous correlations, that are specific of one organ. Importantly, in four out of five breast cancer cohorts for which clinical data were available, the levels of expression of chemotactic factors/receptors that exhibited universal (rather than organ-specific) positive correlations with the immune infiltrate had a positive impact on the response to neoadjuvant chemotherapy. These results support the notion that general (rather than organ-specific) rules governing the recruitment of immune cells into the tumor bed are particularly important in determining local immunosurveillance and response to therapy.
Introduction
Although cancer has been viewed for a long time as a cell-autonomous, genetic and epigenetic disease, there is little doubt that non-transformed cells, in particular leukocytes have a major influence on the development, progression, therapeutic response and fatal relapse of malignant disease.Citation1–Citation4 In essence, the relationship between cancer pathogenesis and leukocytes can be defined as ambiguous. On one hand, chronic subclinical or manifest inflammation can contribute to oncogenesis by favoring local generation of reactive oxygen species, inducing genomic instability, by increasing the turnover of parenchymal or stromal cells, increasing the probability of genetic and chromosomal aberrations, by disrupting homeostatic regulation in the affected tissue, favoring the emergence of more ‘competitive’ cells, and by inducing local immunosuppression, hence subverting immunosurveillance.Citation5,Citation6 On the other hand, specific leukocyte subpopulations may contribute to the recognition of premalignant and fully transformed cells, causing their elimination (the best-case scenario) or engaging in a precarious equilibrium state between malignant cells and immune effectors. In most cases, it is only when cancer cells manage to suppress the anticancer immune response (or to hide from recognition) that the disease becomes clinically detectable.Citation2,Citation4,Citation7–Citation11
The attraction of leukocytes into premalignant and malignant lesions is dictated by multiple soluble factors including so-called danger-associated molecular patterns (DAMPs) that include extracellular nucleotides and nucleosides (with ATP as a prominent chemotactic factor for myeloid cells), proteins that are usually confined in intracellular compartments yet are released from stressed, dying and dead cells (such as annexin-1, ANXA1; calreticulin, CALR, F-actin, and high molecular group protein-1, HMGB1)Citation12–Citation17 and proteins that are actively secreted by cancer or stromal cells, in particular chemokines (C-C motif chemokine ligand 1 to 9 and 11 to 28, CCL1-9, CCL11-28; X-C motif chemokine ligand 1 and 2, XCL1 and 2; C-X-C motif chemokine ligand 1 to 17, CXCL1-17; C-X3-C motif chemokine ligand 1, CX3CL1).Citation18 These factors act on a series of receptors, such as the adenosine receptor family AdoR (ADOR A1, A2A, A2B and A3), metabotropic purinergic receptors (P2RY1, 2, 4, 6, 8 and 10 to 14), ionotropic purinergic receptors (P2RX1 to 7), formylpeptide receptors (FPR1 to FPR3) and chemokine receptors (XCR1, CXCR1 to 7, CX2CR1, CCR1 to 10, CCRL1 and 2) to induce the chemotaxis of different leukocyte subtypes into the tumor.Citation18–Citation21 The expression of such chemotactic factors and their receptors determines the composition of the tumor immune infiltrate and has a major impact on the therapeutic response and prognosis of cancer.Citation9,Citation22–Citation25
Most of the knowledge about the immune infiltrate that can be retrieved from large data sets is based on microarray or RNAseq data obtained on whole tumor specimens and biopsies, requiring bioinformatic deconvolution of the data.Citation26 Methods for estimating the abundance of leukocyte subsets in tumor, based on gene expression data (microarray or RNAseq), have been developed.Citation27–Citation30
Here, we report a meta-analysis of the relationship between the relative abundance of distinct leukocyte subsets and the level of expression of individual chemotactic factors and their receptors in a large set of human tumors.
Results and discussion
Datasets and expression variability
We first assembled a series of public microarray data into one single database. Five different datasets were included for several major cancer types, in particular breast carcinoma (TCGA consortium),Citation31–Citation34 colorectal carcinoma (http://www.intgen.org or TCGA consortium),Citation35,Citation36 non-small cell lung carcinoma (TCGA consortium)Citation37–Citation40 and melanoma,Citation41–Citation45 to study the correlation between the expression level of metagenes indicating the presence of immune cell types and a variety of chemotactic factors and receptors. An extra dataset concerning breast carcinomaCitation46 was used for studying expression variability and treatment response. We also used two datasets for ovarian (TCGA consortium)Citation47 and head and neck carcinomaCitation48,Citation49 each. The total number of tumors analyzed in this study amounts to 5953 (). We first examined the expression variability of each of the metagenes relevant to tumor-infiltrating immune cell types (determined by using the MCP counter method)Citation27,Citation30 and mRNAs encoding annexins (ANXA), chemokines (CCL, CXCL, XCL), chemokine receptors (CCR, CXCR, XCR, CCRL), formyl peptide receptors (FPR), purinergic receptors (ADOR, P2RX, P2RY), compared to housekeeping genes (ACTB, GADPH, TUB), as this is typically done when protein expression is measured by immunoblot analysis. However the variability in the expression of housekeeping genes was not expected to be smaller than that of the genes of interest (including immune metagenes) due to the normalization methods of microarrays. Nevertheless, a systematic low variance of genes of interest (including immune metagenes) compared to housekeeping genes, might produce correlation coefficients (computed below) that are overestimated. Therefore, genes that have a systematic low variance should be removed from the analysis. We analyzed the relative variance of each gene of interest, expressed in a log10 scale for all datasets (). In addition, variance difference tests were performed, between housekeeping genes and genes of interest (Supplementary Figure 1). As a general tendency, the selected genes and metagenes corresponding to distinct immune types exhibited a normalized variance around 1 (i.e. 0 on a log10 scale), with no major difference between immune-relevant and housekeeping genes. Nevertheless, some genes have a smaller variance compared to housekeeping genes, especially in the two breast cancer datasets (METABRIC and TCGA). As a result, we decided to include these close-to-invariant genes in the analyses, because the possible overestimation of correlation coefficients is not systematic.
Table 1. Overview on the cohorts included in this meta-analysis.
Figure 1. Heatmap representation of gene expression variances and immune cell type activity variances, in log10, for the different datasets. For each gene, in each dataset, gene variance was normalized by the variance all gene expressions pooled together. For each immune cell type activities, in each dataset, immune cell type activity variance is normalized by the variance all immune cell type activities pooled together. Housekeeping genes are highlighted.
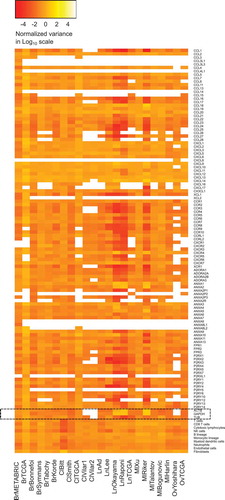
General correlation patterns across cancer types
We computed the Spearman correlation coefficients between immune cell type-relevant metagenes and our selection of chemotaxis-relevant genes. We used Fisher’s method (or Fisher’s combined probability test, for multiple testing) to identify reproducible correlations for five cancer types, namely breast cancer (), colorectal cancer (), non-small cell lung cancer () and melanoma (), while performing a meta-analysis of five different data sets for each cancer (). This procedure was not applicable to ovarian and head & neck cancers because it was not possible to collect 2 × 5 sufficiently large datasets. Correlations that were reproducible across the five data sets were color-coded to indicate positive (red) or negative (green) associations between the expression levels of individual chemotactic factors/receptors and leukocyte subset-specific metagenes, while the absence of reproducible correlations were indicated by white boxes. As to be expected, we detected a mostly positive correlation between leukocyte subsets and chemotactic factors/receptors in all four cancer types ( and ). The reproducibility of correlations was less pronounced in melanoma (), probably because the datasets available for this cancer type were comparatively small ().
Figure 2. Heatmap representation of reproducible Spearman’s correlation coefficients, in breast carcinomas.
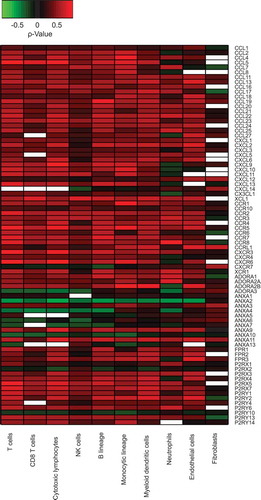
Figure 3. Heatmap representation of reproducible Spearman’s correlation coefficients, in colorectal carcinomas (A), in non-small cell lung cancers (B) and in melanomas (C).
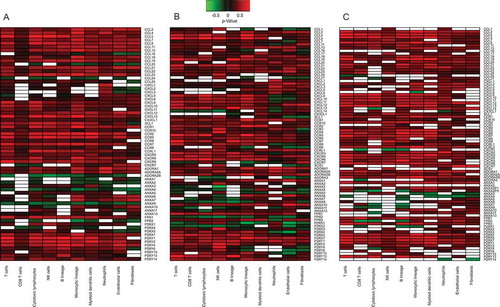
When all these figures were grouped together, (Supplementary Figure 2), one could observe that the variability seemed larger among cancer types than among distinct immune cell types. For this reason, we performed separate analyses of distinct cancer types in different (sub-)figures and then grouped immune cell types. We applied Fisher’s method to identify correlations that were reproducible across the aforementioned five cancer types (). Unsupervised hierarchical clustering of these results identified two types of genes coding for chemotactic factors/receptors, namely (i) genes that exhibit reproducible positive correlations with most of the leukocyte subtypes (in the lower part of ) and (ii) genes that have almost no reproducible correlations with immune cell types (in the upper part of ). The interpretation of this separation in two groups is not obvious. The second group of chemotactic factors/receptors could be interpreted as a cancer type-specific immune system activation. To visualize this possibility, we removed the reproducible correlations across cancer types () from each organ-specific correlation pattern ( and ) with the scope of identifying cancer type-specific correlation patterns (Supplementary Figure 3–6). Again, this separation between fully reproducible and cancer specific-reproducible correlations supported the idea that part of the chemotaxis of different leukocyte subtypes may occur in an organ-specific fashion. We tested the robustness of this two-group separation of chemotactic factors/receptors, by changing the p-value threshold used for reproducibility tests from 0.05 to 0.01. Changing the threshold of the p-value did not affect the final result (Supplementary Fig. 7B) as in .
Figure 4. Heatmap representation of reproducible Spearman’s correlation coefficients, across different cancer types, integrating the results shown in to Fig. 5 on breast, colorectal, non-small cell lung cancer and melanoma.
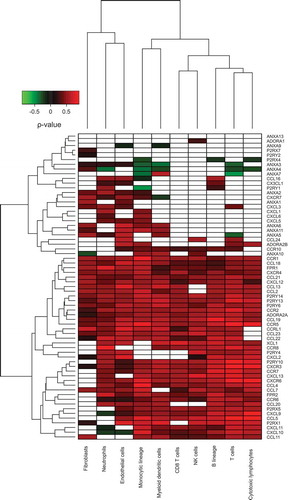
Next, we investigated the reproducible correlation pattern identified in on two other cancer types, namely, ovarian cancer and head and neck carcinoma. Because the number of large datasets for these two cancers types is rather limited (two datasets for each of them, ), it was not possible to determine reproducible correlation patterns within each of these two cancer types. For each dataset, we computed the correlation matrix between immune cell types and the selection of genes. Then, we removed the reproducible correlations identified in . The remaining “specific” correlations are illustrated in Supplementary Fig. 8–11. The number of such specific correlations was rather small, exhibiting no obvious pattern and hence resembled those obtained for breast, colorectal, non-small cell lung cancer and melanoma (Supplementary Figure 3–6). Therefore, ovarian cancer and head and neck carcinoma exhibited a similar global correlation pattern between chemotactic factors/receptors and the density of the immune infiltrate, supporting the universal validity of the common correlation pattern () across distinct malignancies.
One question that can be raised concerns the cancer specificity of these reproducible correlations (fully reproducible or organ-specific reproducible) compared to normal tissue. Although a full analysis, comparing normal and cancer tissue is beyond the scope of this work, we produced a similar Figure than , using normal breast samples available from the METABRIC dataset (Supplementary Fig. 12). The global pattern is not strongly different from . Therefore, it seems that the mechanism involving chemotactic factors/receptors in immune infiltrate in cancer in not fundamentally different from a normal immune activation.
The reproducible correlation pattern across cancers () has been established without any knowledge about the abundance of the chemotactic factors/receptors in different immune cell types or in distinct cancer types. This information is not directly accessible from microarray data, because of normalization methods. Nevertheless, we explored the possibility that the abundance of chemotactic factors/receptors that have a reproducible correlation with immune cell types might be higher or lower than that of those failing to exhibit reproducible correlations, while investigating absolute expression in our microarray datasets and the mRNA expression data from the Human Protein Atlas.Citation50,Citation51 Chemotactic factors/receptors were removed from the analyses if their expression was systemically low in the microarray data or in the spleen and lymph node (see Materials and Methods for the exact procedure). Among the 66 chemotactic factors/receptors that were classed as non-reproducible in their correlation with immune metagenes (in , 12 exhibited a low expression. Among the remaining 39 chemotactic factors/receptors reproducibly correlating with immune metagenes (in ), only one exhibited a low expression. Hence, correlation reproducibility is anti-correlated with low expression (p-value = 0.029, exact Fisher’s test). Indeed, lowly expressed genes should not yield reproducible correlations with immune cell infiltrates. Because the choice for filtering thresholds was quite arbitrary (see Material and Methods), we applied the same procedure by a) doubling threshold on our mRNA datasets, b) doubling threshold on mRNA data from Human Protein Atlas. We still observed the same tendency; in the case a) Fishers’s p-value = 0.155 (filtering 2 genes from the reproducible list and 14 from the non-reproducible one), in case b), Fishers’s p-value = 0.028 (filtering 2 genes from the reproducible list and 14 from the non-reproducible one).
reveals 13 chemotactic receptors and chemokines that positively correlated with all identified cell subsets (irrespective of their stromal and leukocytic nature) contained in the tumor environment, regardless of the cancer type. This applies to CCR1,2,5; CXCR4; FPR1; ADORA2A; P2RY6,13,14; CCL18,19,21; and CXCL12. In other words, these factors appear intrinsically linked to the neoplastic process. Accordingly, CXCL12 and its receptor CXCR4 have been involved in promoting cancer proliferation, survival, invasion, metastasis, stemness and angiogenesis.Citation52–Citation57 CCR1 and its ligand CCL5 have been associated with cancer cell invasion and metastasis.Citation58–Citation60 Similar observations have been reported for the CCR2-CCL2, CCR5-CCL2,3,4,5, CCR7-CCL19,21 and CCR8-CCL18 axes.Citation61–Citation69 The role of FPR1 in tumor progression remains controversial as it has been associated with tumor invasion in colorectal cancer but with tumor suppression in gastric cancer.Citation70,Citation71 As far as P2RY6 is concerned, Placet et al. recently suggested its role in cancer cell survival.Citation72 Overall, these 13 chemotactic receptors and chemokines may constitute universal targets for cancer therapy. Further consolidating the relevance of our results, additional correlations between tumor-infiltrating immune cell subtypes and chemokine/receptors across cancer types previously described in separate preclinical/clinical studies were found (). Among these, we can mention the association between the infiltration of T cells and the requirement of the receptor ADORA2Citation73 or the role of CXCR3 and its ligands CXCL9 to 11 in the recruitment of CD8+ T cells, Th1 cells and NK cells.Citation18 However, also revealed original interactions between immune cell subtypes. For instance, neutrophil infiltrates appeared negatively correlated with the chemokines CXCL9-11. Based on these results, the recruitment of T lymphocytes and neutrophils into the tumor microenvironment could be mutually exclusive. Indeed, a recent work demonstrating an inhibitory activity of CXCL9 peptides on neutrophil migration in murine models supporting such a hypothesis.Citation74
Impact of chemotactic factors and receptors on breast cancer prognosis
It is well established that breast cancer is under immunosurveillance and that neoadjuvant chemotherapy is particularly successful if the cancer is massively infiltrated by immune effectors including T lymphocytes.Citation75–Citation80 Driven by the dichotomy between mRNAs coding for chemotactic factors/receptors that either (i) positively correlated with leukocyte infiltration across distinct cancer types or (ii) lacked a clear pattern of correlation (), we investigated the impact of these two groups of genes on the survival of breast cancer patients. We subdivided the second group into two subgroups (see Supplementary Table 1): a) genes that positively correlate with leukocyte infiltration in breast cancer (but fail to do so across the entire spectrum of cancers analyzed in this study), b) remaining chemotactic factors/receptors (that fail to correlate with immune metagenes in breast cancer). We also considered the group of chemotactic factors/receptors that positively correlated with leukocyte infiltration across distinct cancer types, without the single genes that are characterized by low expression (see Supplementary Table 1).
We explored the link between chemotactic factors/receptors and response to treatment in the four out of the five Breast Carcinoma datasets for which the treatment response was annotated (as pathological complete response or simple response, see ) after neo-adjuvant chemotherapy: Bonnefoi,Citation32 Hatzis,Citation33 Tabchy,Citation34 and Korde.Citation46 We also included the METABRIC datasetCitation81 in this analysis, because we had access to the information whether patients were alive or deceased, a status that is related to disease prognosis and treatment response. In four out of these five datasets (all except the Korde cohort, probably due to its small size), expression of chemotactic factors/receptors that positively correlated with leukocyte infiltration across distinct cancer types (as defined in ) was positively associated with treatment response (Bonnefoi, Hatzis and Tabchy datasets) or overall survival (METABRIC dataset) (). METABRIC results differed for non-reproducible chemotactic factors/receptors, probably because treatment response is measured indirectly. In contrast, no correlation was observed between the remaining chemotactic factors/receptors and prognostic features (). This is also illustrated by the Volcano Plots of different groups of chemotactic factors/receptors (Supplementary Figure 13). Because METABRIC dataset has the clinical information about survival, we attempted to build simple biomarkers (see Material and Methods) from the different groups of chemotactic factors/receptors (Supplementary Figure 14). Only the group of chemotactic factors/receptors reproducibly detected across different cancer types tended to separate the cohort into two groups based on the expression level of the biomarkers, with a difference between survival outcomes that happened to be almost significant (p = 0.081). Altogether, these data support the idea that chemotactic factors and receptors that positively and reproducibly correlate with the immune infiltrate of different cancer types play a positive role in local immunosurveillance, hence improving the probability of complete therapeutic responses in the context of neoadjuvant chemotherapy. The global function of this group of reproducible chemotactic factors/receptors is not well established, and therefore this group cannot be considered as a single metagene, like for immune cell types. Nevertheless it may be interesting to compare the differential abundance of these chemotactic factors/receptors with the differential expression of the immune cell metagenes (in Supplementary Table 2, one sided t-tests of differential expression of treatment response). Although the combined t-tests produce significant differential expression of immune cell metagenes, only one cohort (Bonnefoi) has significant p-values on its own (thresholds 0.05), which appears less significant than the tests for chemotactic factors/receptors of . It is always difficult to interpret such variation between cohorts. The main reason could reside in the treatment regimens and in the clinical definition of treatment response that were not the same for all cohorts.
Table 2. Combined p-values are obtained by applying Fisher’s method.
Concluding remarks
The present bioinformatic study has been conducted with the explicit aim of identifying universal (rather than organ-specific) correlations between the expression level of mRNAs coding for chemotactic factors and receptors, and metagene signatures reflecting distinct leukocyte subtypes infiltrating a variety of cancer types. The specific strategy that we have chosen for this meta-analysis addresses important aspects with respect to the homogeneity and heterogeneity of patient cohorts. Undoubtedly, there are major interindividual variations in the density, composition, architecture and functional state of the tumor infiltrate.Citation9,Citation11,Citation82,Citation83 Notwithstanding this fact, the variations in metagene expression across different cancer types were not higher than those observed for a well known ‘house-keeping’ gene (ACTB, GAPDH, TUB), suggesting that the heterogeneity of the immune infiltrate is not substantially higher than that of the expression of mRNAs coding for essential components of the cytoskeleton (ACTB) or glycolytic metabolism (GAPDH).
A second notion that emerges from this meta-analysis concerns the regulation of the immune infiltrate by chemotactic factors and receptors occurring in a universal (homogeneous) pattern across distinct cancer types, irrespective of their localization in the body, versus organ-specific (heterogeneous) patterns. Surprisingly, it appears that most of the (positive) correlations between the chemotactic factors and receptors analyzed herein and different infiltrating leukocyte subtypes are highly reproducible across different cancer types, while few of them are organ-specific. This points to the importance of common rules dictating the recruitment and permanence of immune cells into the tumor bed, irrespective of the precise cancer type that is concerned. Such universal rules appear to supersede in importance those that may influence organ-specific circuitries. Because this analysis is based on correlation, the causal interpretation is not obvious (are chemotactic factors/receptors attracted by cell types, or is it the contrary?). A better understanding of these circuitries would include a description/modeling of signaling networks at the microenvironment level, which is beyond the scope of the present work.
Most importantly, it appears that the mRNAs coding for chemotactic factors and receptors that reproducibly and positively correlate with the local presence of distinct immune cell types seem to have a positive impact on breast cancer prognosis. This contrasts with chemotactic factors and receptors that exhibit heterogeneous and organ-specific patterns of correlation with the immune infiltrate that have no such prognostic impact. On a positive note, this implies that, at least in the realm of chemotaxis, insights that have been gained in one cancer type can be extrapolated to other malignancies, irrespective of their cell of origin.
Material and methods
Datasets and immune infiltrate estimation
We explored public datasets of transcriptome microarrays. The complete list of these datasets is shown in . We use data that were already normalized, as provided from the different repository websites. The MCPcounterCitation27 R-package was used for estimating immune cell type activities. This method uses groups of genes to construct metagenes, whose expressions measure indirectly immune cell abundances. The identification of gene groups have been done in a strongly reproducible manner, learned from datasets where immune cell type abundances are known.
Test of variance difference
One sided F-test was used, to test if variance of genes of interest (including immune metagenes) is smaller than variance of housekeeping genes.
Patterns of reproducible correlation
For each dataset, we computed the correlation coefficients between immune cell activities and expression of individual mRNAs coding for chemotactic factors/receptors. We decided to use the Spearman’s correlation method, because the normal hypothesis necessary for using Pearson’s approach is not guaranteed for immune activities estimated by MCP counter method. For each cancer type, we used the Fisher’s method in the following way. We computed one-sided Spearman correlation test for both cases (H1: positive correlation and H1: negative correlation). For each case (H1 positive and H1 negative), we combined the p-value by using Fisher’s method (Chi-square test on sum of p-value logarithms). If both p-values were higher than 0.05, the correlation was considered as non-reproducible. In any other case, we considered that the correlation was reproducible. We then used the coefficient that has the smallest p-value and whose sign is compatible with the hypothesis H1 that had a significant combined p-value. These correlation coefficients are illustrated in –.
For the fully reproducible correlation coefficients listed in Figure 6, we applied the same procedure as above. Thus, we considered the correlations coefficients of – and their associated p-values, as computed above (combined one-sided correlation tests by Fisher’s method). These p-values were one-sided ones, related to the sign of the associated correlation coefficient. We computed the p-values associated to one-sided correlation test of opposite sign (‘one sided p-value’ → (1-‘one sided p-value’). Therefore, we could apply Fisher’s method for both H1 hypothesis (positive and negative correlation) to a common set of cancer types. Again, if both p-values were higher than 0.05, the correlation was interpreted as non-reproducible. In the other case, we considered that correlation was reproducible; we then used the coefficient that has the smallest p-value and whose sign is compatible with the hypothesis H1 that has a significant combined p-value. These correlation coefficients are shown in . Suppl. Fig 7 is produced by using the same analysis, replacing the p-value threshold of 0.05 () by 0.01 (Suppl. Fig. 7).
Filtering for low expression
The first filter is based on microarray expression among the five cancer types. For each dataset, we computed the average (among different patients) of the expression rank, for each chemotactic factors/receptors. Because no obvious threshold emerged from these mean rank expressions, we choose it arbitrarily (value = 0.2) to filter out a reasonable amount of these chemotactic factors/receptors that are expressed at a low level.
The second filter is based on mRNA expression from the Human Protein Atlas Citation50,Citation51,Citation84 from the three methods (HPA, GTEx, FANTOM5), in the spleen and lymph node. Again, no obvious threshold emerged from these data. Therefore we arbitrarily considered that a gene has a low expression if its mRNA abundance is lower than (TPM/RPKM = 1), as determined by all three methods (HPA, GTEx, FANTOM5) and in both spleen and lymph node.
We applied these two filters to the list of chemotactic factors/receptors. We also considered a threshold of 2 for (TPM/RKM), applying the same analysis.
Fisher’s exact test for treatment response
We considered genes that were overexpressed in responsive tumors (or alive patients in METABRIC dataset), using a p-value threshold of 5%, a logFC threshold of log2(1.2) for the METABRIC, Bonnefoi and Korde datasets, a logFC threshold of log2(1.5) for Hatzis’ and Tabchy’s datasets (these thresholds varied in order to obtain non-empty intersections with the reproducible correlation gene list of ), using limma R-package.Citation85 For each dataset, we computed the contingency tables regarding overexpressed genes and reproducible correlation gene list of and applied Fisher’s exact tests. Significant results are shown in .
Supplemental Material
Download Zip (701.3 KB)Acknowledgments
We thank Prof. Catherine Sautès-Fridman and Dr. Florent Petitprez (Centre de Recherche des Cordeliers, Paris, France) for critical comments. GK is supported by the Ligue contre le Cancer Comité de Charente-Maritime (équipe labelisée); Agence National de la Recherche (ANR) – Projets blancs; ANR under the frame of E-Rare-2, the ERA-Net for Research on Rare Diseases; Association pour la recherche sur le cancer (ARC); Cancéropôle Ile-de-France; Chancelerie des universités de Paris (Legs Poix), Fondation pour la Recherche Médicale (FRM); the European Commission (ArtForce); the European Research Council (ERC); Fondation Carrefour; Institut National du Cancer (INCa); Inserm (HTE); Institut Universitaire de France; LeDucq Foundation; the LabEx Immuno-Oncology; the RHU Torino Lumière, the Seerave Foundation; the SIRIC Stratified Oncology Cell DNA Repair and Tumor Immune Elimination (SOCRATE); the SIRIC Cancer Research and Personalized Medicine (CARPEM); and the Paris Alliance of Cancer Research Institutes (PACRI).
Disclosure statement
No potential conflict of interest was reported by the authors.
References
- Kroemer G, Pouyssegur J. Tumor cell metabolism: cancer’s Achilles’ heel. Cancer Cell. 2008;13:472–482. doi:10.1016/j.ccr.2008.05.005.
- Schreiber RD, Old LJ, Smyth MJ. Cancer immunoediting: integrating immunity’s roles in cancer suppression and promotion. Science. 2011;331:1565–1570. doi:10.1126/science.1203486.
- Hanahan D, Weinberg RA. Hallmarks of cancer: the next generation. Cell. 2011;144:646–674. doi:10.1016/j.cell.2011.02.013.
- Palucka AK, Coussens LM. The basis of oncoimmunology. Cell. 2016;164:1233–1247. doi:10.1016/j.cell.2016.01.049.
- Balkwill F. Cancer and the chemokine network. Nat Rev Cancer. 2004;4:540–550. doi:10.1038/nrc1388.
- Lin WW, Karin M. A cytokine-mediated link between innate immunity, inflammation, and cancer. J Clin Invest. 2007;117:1175–1183. doi:10.1172/JCI31537.
- Zitvogel L, Apetoh L, Ghiringhelli F, Kroemer G. Immunological aspects of cancer chemotherapy. Nat Rev Immunol. 2008;8:59–73. doi:10.1038/nri2216.
- Zitvogel L, Kepp O, Kroemer G. Immune parameters affecting the efficacy of chemotherapeutic regimens. Nat Rev Clin Oncol. 2011;8:151–160. doi:10.1038/nrclinonc.2010.223.
- Fridman WH, Pages F, Sautes-Fridman C, Galon J. The immune contexture in human tumours: impact on clinical outcome. Nat Rev Cancer. 2012;12:298–306. doi:10.1038/nrc3245.
- Kroemer G, Senovilla L, Galluzzi L, Andre F, Zitvogel L. Natural and therapy-induced immunosurveillance in breast cancer. Nat Med. 2015;21:1128–1138. doi:10.1038/nm.3944.
- Fridman WH, Zitvogel L, Sautes-Fridman C, Kroemer G. The immune contexture in cancer prognosis and treatment. Nat Rev Clin Oncol. 2017;14:717–734. doi:10.1038/nrclinonc.2017.101.
- Obeid M, Tesniere A, Panaretakis T, Tufi R, Joza N, van Endert P, Lionel G, Nathalie A, Caroline C, Evelyn F, et al. Ecto-calreticulin in immunogenic chemotherapy. Immunol Rev. 2007;220:22–34. doi:10.1111/j.1600-065X.2007.00567.x.
- Apetoh L, Ghiringhelli F, Tesniere A, Criollo A, Ortiz C, Lidereau R, Nathalie M, Jean C, Mira P, Delaloge S, André F, et al. The interaction between HMGB1 and TLR4 dictates the outcome of anticancer chemotherapy and radiotherapy. Immunol Rev. 2007;220:47–59. doi:10.1111/j.1600-065X.2007.00573.x.
- Vacchelli E, Ma Y, Baracco EE, Sistigu A, Enot DP, Pietrocola F, Yang H, Adjemian S, Chaba K, Semeraro M, et al. Chemotherapy-induced antitumor immunity requires formyl peptide receptor 1. Science. 2015;350:972–978. doi:10.1126/science.aad0779.
- Ahrens S, Zelenay S, Sancho D, Hanc P, Kjaer S, Feest C, Fletcher G, Durkin C, Postigo A, Skehel M, et al. F-actin is an evolutionarily conserved damage-associated molecular pattern recognized by DNGR-1, a receptor for dead cells. Immunity. 2012;36:635–645. doi:10.1016/j.immuni.2012.03.008.
- Stoll G, Zitvogel L, Kroemer G. Differences in the composition of the immune infiltrate in breast cancer, colorectal carcinoma, melanoma and non-small cell lung cancer: A microarray-based meta-analysis. Oncoimmunology. 2016;5:e1067746. doi:10.1080/2162402X.2015.1067746.
- Stoll G, Iribarren K, Michels J, Leary A, Zitvogel L, Cremer I, Kroemer G. Calreticulin expression: interaction with the immune infiltrate and impact on survival in patients with ovarian and non-small cell lung cancer. Oncoimmunology. 2016;5:e1177692. doi:10.1080/2162402X.2016.1177692.
- Nagarsheth N, Wicha MS, Zou W. Chemokines in the cancer microenvironment and their relevance in cancer immunotherapy. Nat Rev Immunol. 2017;17:559–572. doi:10.1038/nri.2017.49.
- Schulz O, Hammerschmidt SI, Moschovakis GL, Forster R. Chemokines and chemokine receptors in lymphoid tissue dynamics. Annu Rev Immunol. 2016;34:203–242. doi:10.1146/annurev-immunol-041015-055649.
- Di Virgilio F, Adinolfi E. Extracellular purines, purinergic receptors and tumor growth. Oncogene. 2017;36:293–303. doi:10.1038/onc.2016.206.
- Di Virgilio F, Falzoni S, Giuliani AL, Adinolfi E. P2 receptors in cancer progression and metastatic spreading. Curr Opin Pharmacol. 2016;29:17–25. doi:10.1016/j.coph.2016.05.001.
- Qian BZ, Li J, Zhang H, Kitamura T, Zhang J, Campion LR, Kaiser EA, Snyder LA, Pollard JW. CCL2 recruits inflammatory monocytes to facilitate breast-tumour metastasis. Nature. 2011;475:222–225. doi:10.1038/nature10138.
- Bindea G, Mlecnik B, Tosolini M, Kirilovsky A, Waldner M, Obenauf AC, Angell H, Fredriksen T, Lafontaine L, Berger A, et al. Spatiotemporal dynamics of intratumoral immune cells reveal the immune landscape in human cancer. Immunity. 2013;39:782–795. doi:10.1016/j.immuni.2013.10.003.
- Highfill SL, Cui Y, Giles AJ, Smith JP, Zhang H, Morse E, Kaplan RN, Mackall CL. Disruption of CXCR2-mediated MDSC tumor trafficking enhances anti-PD1 efficacy. Sci Transl Med. 2014;6:237ra67. doi:10.1126/scitranslmed.3007974.
- Peng D, Kryczek I, Nagarsheth N, Zhao L, Wei S, Wang W, Sun Y, Zhao E, Vatan L, Szeliga W, et al. Epigenetic silencing of TH1-type chemokines shapes tumour immunity and immunotherapy. Nature. 2015;527:249–253. doi:10.1038/nature15520.
- Hackl H, Charoentong P, Finotello F, Trajanoski Z. Computational genomics tools for dissecting tumour-immune cell interactions. Nat Rev Genet. 2016;17:441–458. doi:10.1038/nrg.2016.67.
- Becht E, Giraldo NA, Lacroix L, Buttard B, Elarouci N, Petitprez F, Selves J, Laurent-Puig P, Sautès-Fridman C, Fridman WH, et al. Estimating the population abundance of tissue-infiltrating immune and stromal cell populations using gene expression. Genome Biol. 2016;17:218. doi:10.1186/s13059-016-1070-5.
- Newman AM, Liu CL, Green MR, Gentles AJ, Feng W, Xu Y, Hoang CD, Diehn M, Alizadeh AA. Robust enumeration of cell subsets from tissue expression profiles. Nat Methods. 2015;12:453–457. doi:10.1038/nmeth.3337.
- Gaujoux R, Seoighe C. CellMix: a comprehensive toolbox for gene expression deconvolution. Bioinformatics (Oxford, England). 2013;29:2211–2212. doi:10.1093/bioinformatics/btt351.
- Petitprez F, Vano YA, Becht E, Giraldo NA, de Reynies A, Sautes-Fridman C, Fridman WH. Transcriptomic analysis of the tumor microenvironment to guide prognosis and immunotherapies. Cancer Immunol ImmunoTher CII. 2017.
- Curtis C, Shah SP, Chin SF, Turashvili G, Rueda OM, Dunning MJ, Speed D, Lynch AG, Samarajiwa S, Yuan Y, et al. The genomic and transcriptomic architecture of 2,000 breast tumours reveals novel subgroups. Nature. 2012;486:346–352. doi:10.1038/nature10983.
- Bonnefoi H, Piccart M, Bogaerts J, Mauriac L, Fumoleau P, Brain E, Petit T, Rouanet P, Jassem J, Blot E, et al. TP53 status for prediction of sensitivity to taxane versus non-taxane neoadjuvant chemotherapy in breast cancer (EORTC 10994/BIG 1-00): a randomised phase 3 trial. Lancet Oncol. 2011;12:527–539. doi:10.1016/S1470-2045(11)70094-8.
- Hatzis C, Pusztai L, Valero V, Booser DJ, Esserman L, Lluch A, Vidaurre T, Holmes F, Souchon E, Wang H, et al. A genomic predictor of response and survival following taxane-anthracycline chemotherapy for invasive breast cancer. JAMA. 2011;305:1873–1881. doi:10.1001/jama.2011.593.
- Tabchy A, Valero V, Vidaurre T, Lluch A, Gomez H, Martin M, Qi Y, Barajas-Figueroa LJ, Souchon E, Coutant C, et al. Evaluation of a 30-gene paclitaxel, fluorouracil, doxorubicin, and cyclophosphamide chemotherapy response predictor in a multicenter randomized trial in breast cancer. Clin Cancer Res. 2010;16:5351–5361. doi:10.1158/1078-0432.CCR-10-1265.
- Smith JJ, Deane NG, Wu F, Merchant NB, Zhang B, Jiang A, Lu P, Johnson JC, Schmidt C, Bailey CE, et al. Experimentally derived metastasis gene expression profile predicts recurrence and death in patients with colon cancer. Gastroenterology. 2010;138:958–968. doi:10.1053/j.gastro.2009.11.005.
- Vilar E, Bartnik CM, Stenzel SL, Raskin L, Ahn J, Moreno V, Mukherjee B, Iniesta MD, Morgan MA, Rennert G, et al. MRE11 deficiency increases sensitivity to poly(ADP-ribose) polymerase inhibition in microsatellite unstable colorectal cancers. Cancer Res. 2011;71:2632–2642. doi:10.1158/0008-5472.CAN-10-1120.
- Shedden K, Taylor JM, Enkemann SA, Tsao MS, Yeatman TJ, Gerald WL, Eschrich S, Jurisica I, Giordano TJ, Misek DE, et al. Gene expression-based survival prediction in lung adenocarcinoma: a multi-site, blinded validation study. Nat Med. 2008;14:822–827. doi:10.1038/nm.1790.
- Lee ES, Son DS, Kim SH, Lee J, Jo J, Han J, Kim H, Lee HJ, Choi HY, Jung Y, et al. Prediction of recurrence-free survival in postoperative non-small cell lung cancer patients by using an integrated model of clinical information and gene expression. Clin Cancer Res. 2008;14:7397–7404. doi:10.1158/1078-0432.CCR-07-4937.
- Okayama H, Kohno T, Ishii Y, Shimada Y, Shiraishi K, Iwakawa R,Furuta K, Tsuta K, Shibata T, Yamamoto S, et al. Identification of genes upregulated in ALK-positive and EGFR/KRAS/ALK-negative lung adenocarcinomas. Cancer Res. 2012;72:100–111. doi:10.1158/0008-5472.CAN-11-1403.
- Raponi M, Zhang Y, Yu J, Chen G, Lee G, Taylor JM, MacDonald J, Thomas D, Moskaluk C, Wang Y, et al. Gene expression signatures for predicting prognosis of squamous cell and adenocarcinomas of the lung. Cancer Res. 2006;66:7466–7472. doi:10.1158/0008-5472.CAN-06-1191.
- Xu L, Shen SS, Hoshida Y, Subramanian A, Ross K, Brunet JP, Wagner SN, Ramaswamy S, Mesirov JP, Hynes RO. Gene expression changes in an animal melanoma model correlate with aggressiveness of human melanoma metastases. Mol Cancer Res. 2008;6:760–769. doi:10.1158/1541-7786.MCR-07-0344.
- Harlin H, Meng Y, Peterson AC, Zha Y, Tretiakova M, Slingluff C, McKee M, Gajewski TF. Chemokine expression in melanoma metastases associated with CD8+ T-cell recruitment. Cancer Res. 2009;69:3077–3085. doi:10.1158/0008-5472.CAN-08-2281.
- Bogunovic D, O’Neill DW, Belitskaya-Levy I, Vacic V, Yu YL, Adams S, Darvishian F, Berman R, Shapiro R, Pavlick AC, et al. Immune profile and mitotic index of metastatic melanoma lesions enhance clinical staging in predicting patient survival. Proc Natl Acad Sci U S A. 2009;106:20429–20434. doi:10.1073/pnas.0905139106.
- Riker AI, Enkemann SA, Fodstad O, Liu S, Ren S, Morris C, Xi Y, Howell P, Metge B, Samant RS, et al. The gene expression profiles of primary and metastatic melanoma yields a transition point of tumor progression and metastasis. BMC Med Genomics. 2008;1:13. doi:10.1186/1755-8794-1-13.
- Talantov D, Mazumder A, Yu JX, Briggs T, Jiang Y, Backus J,Atkins D, Wang Y. Novel genes associated with malignant melanoma but not benign melanocytic lesions. Clin Cancer Res. 2005;11:7234–7242. doi:10.1158/1078-0432.CCR-05-0683.
- Korde LA, Lusa L, McShane L, Lebowitz PF, Lukes L, Camphausen K, Parker JS, Swain SM, Hunter K, Zujewski JA. Gene expression pathway analysis to predict response to neoadjuvant docetaxel and capecitabine for breast cancer. Breast Cancer Res Treat. 2010;119:685–699. doi:10.1007/s10549-009-0651-3.
- Yoshihara K, Tajima A, Yahata T, Kodama S, Fujiwara H, Suzuki M, Onishi Y, Hatae M, Sueyoshi K, Fujiwara H, et al. Gene expression profile for predicting survival in advanced-stage serous ovarian cancer across two independent datasets. PLoS One. 2010;5:e9615. doi:10.1371/journal.pone.0009615.
- Peng CH, Liao CT, Peng SC, Chen YJ, Cheng AJ, Juang JL, Tsai C-Y, Chen T-C, Chuang Y-J, Tang C-Y, et al. A novel molecular signature identified by systems genetics approach predicts prognosis in oral squamous cell carcinoma. PLoS One. 2011;6:e23452. doi:10.1371/journal.pone.0023452.
- Rickman DS, Millon R, De Reynies A, Thomas E, Wasylyk C, Muller D, Abecassis J, Wasylyk B. Prediction of future metastasis and molecular characterization of head and neck squamous-cell carcinoma based on transcriptome and genome analysis by microarrays. Oncogene. 2008;27:6607–6622. doi:10.1038/onc.2008.251.
- Uhlen M, Bjorling E, Agaton C, Szigyarto CA, Amini B, Andersen E, Andersson A-C, Angelidou P, Asplund A, Asplund C, et al. A human protein atlas for normal and cancer tissues based on antibody proteomics. Mol Cell Proteomics MCP. 2005;4:1920–1932. doi:10.1074/mcp.M500279-MCP200.
- UhlenM, Oksvold P, Fagerberg L, Lundberg E, Jonasson K, Forsberg M, Lundberg E, Jonasson K, Forsberg M, Zwahlen M, et al. Towards a knowledge-based human protein atlas. Nat Biotechnol. 2010;28:1248–1250. doi:10.1038/nbt1210-1248.
- Kryczek I, Wei S, Keller E, Liu R, Zou W. Stroma-derived factor (SDF-1/CXCL12) and human tumor pathogenesis. Am J Physiol Cell Physiol. 2007;292:C987–95. doi:10.1152/ajpcell.00406.2006.
- Murakami T, Maki W, Cardones AR, Fang H, Tun Kyi A, Nestle FO, Hwang ST. Expression of CXC chemokine receptor-4 enhances the pulmonary metastatic potential of murine B16 melanoma cells. Cancer Res. 2002;62:7328–7334.
- Helbig G, Christopherson KW, 2nd, Bhat-Nakshatri P, Kumar S, Kishimoto H, Miller KD, Broxmeyer HE, Nakshatri H. NF-kappaB promotes breast cancer cell migration and metastasis by inducing the expression of the chemokine receptor CXCR4. J Biol Chem. 2003;278:21631–21638. doi:10.1074/jbc.M300609200.
- Kang Y, Siegel PM, Shu W, Drobnjak M, Kakonen SM, Cordon-Cardo C,Guise TA, Massaguè J. A multigenic program mediating breast cancer metastasis to bone. Cancer Cell. 2003;3:537–549. doi:10.1016/S1535-6108(03)00132-6.
- Jung MJ, Rho JK, Kim YM, Jung JE, Jin YB, Ko YG, Lee J-S, Lee S-J, Lee JC, Park M-J. Upregulation of CXCR4 is functionally crucial for maintenance of stemness in drug-resistant non-small cell lung cancer cells. Oncogene. 2013;32:209–221. doi:10.1038/onc.2012.37.
- Zhang SS, Han ZP, Jing YY, Tao SF, Li TJ, Wang H, Wang Y, Li R, Yang Y, Zhao X, et al. CD133(+)CXCR4(+) colon cancer cells exhibit metastatic potential and predict poor prognosis of patients. BMC Medicine. 2012;10:85. doi:10.1186/1741-7015-10-85.
- Akram IG, Georges R, Hielscher T, Adwan H, Berger MR. The chemokines CCR1 and CCRL2 have a role in colorectal cancer liver metastasis. Tumour Biol J Int Soc Oncodevelopmental Biol Med. 2016;37:2461–2471. doi:10.1007/s13277-015-4089-4.
- Wang CL, Sun BS, Tang Y, Zhuang HQ, Cao WZ. CCR1 knockdown suppresses human non-small cell lung cancer cell invasion. J Cancer Res Clin Oncol. 2009;135:695–701. doi:10.1007/s00432-008-0505-0.
- Shin SY, Lee DH, Lee J, Choi C, Kim JY, Nam JS,Lim Y, Lee YH. C-C motif chemokine receptor 1 (CCR1) is a target of the EGF-AKT-mTOR-STAT3 signaling axis in breast cancer cells. Oncotarget. 2017;8:94591–94605.
- Lim SY, Yuzhalin AE, Gordon-Weeks AN, Muschel RJ. Targeting the CCL2-CCR2 signaling axis in cancer metastasis. Oncotarget. 2016;7:28697–28710. doi:10.18632/oncotarget.v7i19.
- Nywening TM, Wang-Gillam A, Sanford DE, Belt BA, Panni RZ, Cusworth BM, Toriola AT, Nieman RK, Worley LA, Yano M, et al. Targeting tumour-associated macrophages with CCR2 inhibition in combination with FOLFIRINOX in patients with borderline resectable and locally advanced pancreatic cancer: a single-centre, open-label, dose-finding, non-randomised, phase 1b trial. Lancet Oncol. 2016;17:651–662. doi:10.1016/S1470-2045(16)00078-4.
- Chen X, Wang Y, Nelson D, Tian S, Mulvey E, Patel B,Conti I, Jaen J, Rollins BJ. CCL2/CCR2 regulates the tumor microenvironment in HER-2/neu-driven mammary carcinomas in mice. PLoS One. 2016;11:e0165595. doi:10.1371/journal.pone.0165595.
- Halama N, Zoernig I, Berthel A, Kahlert C, Klupp F, Suarez-Carmona M, Suetterlin T, Brand K, Krauss J, Lasitschka F. Tumoral immune cell exploitation in colorectal cancer metastases can be targeted effectively by anti-CCR5 therapy in cancer patients. Cancer Cell. 2016;29:587–601. doi:10.1016/j.ccell.2016.03.005.
- Ward ST, Li KK, Hepburn E, Weston CJ, Curbishley SM, Reynolds GM, Hejmadi RK, Bicknell R, Eksteen B, Ismail T, et al. The effects of CCR5 inhibition on regulatory T-cell recruitment to colorectal cancer. Br J Cancer. 2015;112:319–328. doi:10.1038/bjc.2014.572.
- Bronte V, Bria E. Interfering with CCL5/CCR5 at the tumor-stroma interface. Cancer Cell. 2016;29:437–439. doi:10.1016/j.ccell.2016.03.019.
- Lin L, Chen YS, Yao YD, Chen JQ, Chen JN, Huang SY, Zeng Y-J, Yao H-R, Zeng S-H, Fu Y-S, et al. CCL18 from tumor-associated macrophages promotes angiogenesis in breast cancer. Oncotarget. 2015;6:34758–34773. doi:10.18632/oncotarget.5325.
- Zhang X, Wang Y, Cao Y, Zhang X, Zhao H. Increased CCL19 expression is associated with progression in cervical cancer. Oncotarget. 2017;8:73817–73825.
- Xu B, Zhou M, Qiu W, Ye J, Feng Q. CCR7 mediates human breast cancer cell invasion, migration by inducing epithelial-mesenchymal transition and suppressing apoptosis through AKT pathway. Cancer Med. 2017;6:1062–1071. doi:10.1002/cam4.1039.
- Li SQ, Su N, Gong P, Zhang HB, Liu J, Wang D, Sun Y-P, Zhang Y, Qian F, Zhao B, et al. The expression of formyl peptide receptor 1 is correlated with tumor invasion of human colorectal cancer. Sci Rep. 2017;7:5918. doi:10.1038/s41598-017-06368-9.
- Prevete N, Liotti F, Visciano C, Marone G, Melillo RM, de Paulis A. The formyl peptide receptor 1 exerts a tumor suppressor function in human gastric cancer by inhibiting angiogenesis. Oncogene. 2015;34:3826–3838. doi:10.1038/onc.2014.309.
- Placet M, Arguin G, Molle CM, Babeu JP, Jones C, Carrier JC, Robaye B, Geha S, Boudreau F, Gendron F-P. The G protein-coupled P2Y6 receptor promotes colorectal cancer tumorigenesis by inhibiting apoptosis. Biochim Biophys Acta. 2018;1864:1539–1551. doi:10.1016/j.bbadis.2018.02.008.
- Cekic C, Linden J. Adenosine A2A receptors intrinsically regulate CD8+ T cells in the tumor microenvironment. Cancer Res. 2014;74:7239–7249. doi:10.1158/0008-5472.CAN-13-3581.
- Vanheule V, Boff D, Mortier A, Janssens R, Petri B, Kolaczkowska E, Kubes P, Berghmans N, Struyf S, Kungl AJ, et al. CXCL9-derived peptides differentially inhibit neutrophil migration in vivo through interference with glycosaminoglycan interactions. Front Immunol. 2017;8:530. doi:10.3389/fimmu.2017.00530.
- Ladoire S, Mignot G, Dalban C, Chevriaux A, Arnould L, Rebe C, Apetoh L, Boidot R, Penault-Llorca F, Fumoleau P, et al. FOXP3 expression in cancer cells and anthracyclines efficacy in patients with primary breast cancer treated with adjuvant chemotherapy in the phase III UNICANCER-PACS 01 trial. Ann Oncol Off J Eur Soc Med Oncol. 2012;23:2552–2561. doi:10.1093/annonc/mds028.
- Stoll G, Enot D, Mlecnik B, Galon J, Zitvogel L, Kroemer G. Immune-related gene signatures predict the outcome of neoadjuvant chemotherapy. Oncoimmunology. 2014;3:e27884. doi:10.4161/onci.27884.
- Senovilla L, Vitale I, Martins I, Tailler M, Pailleret C, Michaud M,Galluzzi L, Adjemian S, Kepp O, Niso-Santano M, et al. An immunosurveillance mechanism controls cancer cell ploidy. Science. 2012;337:1678–1684. doi:10.1126/science.1224922.
- Sistigu A, Yamazaki T, Vacchelli E, Chaba K, Enot DP, Adam J, Vitale I, Goubar A, Baracco EE, Remèdios C, et al. Cancer cell-autonomous contribution of type I interferon signaling to the efficacy of chemotherapy. Nat Med. 2014;20:1301–1309. doi:10.1038/nm.3708.
- Semeraro M, Adam J, Stoll G, Louvet E, Chaba K, Poirier-Colame V, Sauvat A, Senovilla L, Vacchelli E, Bloy N, et al. The ratio of CD8+/FOXP3 T lymphocytes infiltrating breast tissues predicts the relapse of ductal carcinoma in situ. Oncoimmunology. 2016;5:e1218106. doi:10.1080/2162402X.2016.1218106.
- Stoll G, Ma Y, Yang H, Kepp O, Zitvogel L, Kroemer G. Pro-necrotic molecules impact local immunosurveillance in human breast cancer. Oncoimmunology. 2017;6:e1299302. doi:10.1080/2162402X.2017.1299302.
- Pereira B, Chin SF, Rueda OM, Vollan HK, Provenzano E, Bardwell HA,Pugh M, Jones L, Russell R, Sammut S-J, et al. The somatic mutation profiles of 2,433 breast cancers refines their genomic and transcriptomic landscapes. Nat Commun. 2016;7:11479. doi:10.1038/ncomms11479.
- Fridman WH, Galon J, Pages F, Tartour E, Sautes-Fridman C, Kroemer G. Prognostic and predictive impact of intra- and peritumoral immune infiltrates. Cancer Res. 2011;71:5601–5605. doi:10.1158/0008-5472.CAN-11-1316.
- Ingold Heppner B, Loibl S, Denkert C. Tumor-infiltrating lymphocytes: A promising biomarker in breast cancer. Breast Care (Basel, Switzerland). 2016;11:96–100. doi:10.1159/000444357.
- Uhlen M, Fagerberg L, Hallstrom BM, Lindskog C, Oksvold P, Mardinoglu A, Sivertsson A, Kampf C, Sjöstedt E, Asplund A, et al. Proteomics. Tissue-based map of the human proteome. Science. 2015;347:1260419. doi:10.1126/science.1260419.
- Ritchie ME, Phipson B, Wu D, Hu Y, Law CW, Shi W, Smyth GK. limma powers differential expression analyses for RNA-sequencing and microarray studies. Nucleic Acids Res. 2015;43:e47. doi:10.1093/nar/gkv007.