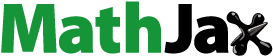
ABSTRACT
Tobacco smoking causes DNA damages in epithelial cells and immune dysfunction in the lung, which collectively contribute to lung carcinogenesis and progression. However, potential mechanisms by which tumor-infiltrating immune cells contribute to lung cancer survival and their differential contributions in ever-smokers and never-smokers are not well studied. Here, we performed integrative analysis of 11 lung cancer gene-expression datasets, including 1,111 lung adenocarcinomas and 200 adjacent normal lung samples. Distinct pathways were altered in lung carcinogenesis in ever-smokers and never-smokers. Never-smoker patients had a better outcome than ever-smoker patients. We characterized compositional patterns of 21 types of immune cells in lung adenocarcinomas and revealed the complex association between immune cell composition and clinical outcomes. Interestingly, we found two subsets of immune cells, mast cells and CD4+ memory T cells, which had completely opposite associations with outcomes in resting and activated status. We further discovered that several chemokines and their associated receptors (e.g., CXCL11-CX3CR1 axis) were selectively altered in lung tumors in response to cigarette smoking and their abundances showed stronger correlation with fractions of these immune subsets in ever-smokers than never-smokers. The status switched from the resting to activated forms in mast cells and CD4+ memory T cells might manifest some important processes induced by cigarette smoking during tumor development and progression. Our findings suggested that aberrant activation of mast cells and CD4+ memory T cells plays crucial roles in cigarette smoking-induced immune dysfunction in the lung, which contributes to tumor development and progression.
Introduction
Lung cancer is one of the most frequently diagnosed cancers and the leading cause of cancer-related death worldwide.Citation1 Despite recent advances in therapy, the overall 5-year survival has changed little in the last several decades and remains at 18.1% in the United States; outcomes are, on average, even worse in the developing countries. Approximately 10%-15% of patients with lung cancer are lifelong never-smokers; active cigarette smoking accounts for the majority of lung cancer.Citation2 Among all types of lung cancer, adenocarcinoma is one of the most frequent subtypes of non-small cell lung carcinoma (NSCLC), accounting for about 40% lung cancers, which is also the most common type seen in never-smokers. Compared to other types of lung cancer, adenocarcinomas tend to form metastases widely at an early stage, and the response to radiation therapy is not as effective as it is in small cell lung carcinoma. Most lung cancer results from multiple changes in the genome of susceptible pulmonary cells caused by exposure to carcinogens found in tobacco smoke, the environment, and the workplace. Patients exposed to a smoking environment had more frequent gene mutations, such as the epidermal growth factor receptor (EGFR) gene,Citation3 the K-ras gene,Citation4 and the p53 gene.Citation5,Citation6
In addition to higher-frequency gene mutations, cigarette smoking also plays an important role in the immunological homeostasis. The impact of smoking is not identical on different immune cells, and the adverse effect can be summarized as follows: inflammatory cells are recruited into the lungs but weaken the ability of those cells, and cell populations of some subtypes decrease and switch the immune response to a more harmful pattern.Citation7 On the other hand, immune cells play an important role in shaping the tumor microenvironment, which interacts with the tumor cells and can be involved in carcinogenesis, development, invasion, and metastasis of tumors.Citation8 Some antibody-based anticancer drugs that target immune-related receptors improve patients’ survival time to some extent, for example, ipilimumab targets cytotoxic T-lymphocyte-associated antigen 4 (CTLA-4), and nivolumab and lambrolizumab target the Programmed Death 1 (PD1) receptor and the PD1 ligand (PD-L1).Citation8
Tobacco smoking causes DNA damage in epithelial cells and impacts the immune system in the lung, which collectively contribute to lung carcinogenesis and disease progression in smokers. Considerable epidemiological and genetic analysis of lung tumors suggests that alternative mechanisms of lung carcinogenesis and tumor microenvironments are also important in never-smokers, and these alternative mechanisms remain unclear.Citation9–Citation11 The specific recognition of the mechanisms by which tumor-infiltrating immune cells contribute to the metastatic cascade in lung cancer and their differential contributions in ever-smokers and never-smokers is the important first step toward successful cancer immunotherapy.
In this study, we collected 11 lung cancer microarray datasets, including 1,111 lung adenocarcinomas and 200 adjacent normal lung samples (Figure S1). A recently developed machine-learning method, CIBERSORT,Citation12 was applied to characterize the composition of leukocytes in these lung tumor and normal tissues using their gene expression profiles. To investigate tissue-specific tumor microenvironment, we refined a new signature gene matrix as a benchmark for CIBERSORT to sort and enumerate leukocytes. Another in silco approach, xCell,Citation13 which is based on single-sample gene set enrichment analysis (ssGSEA), was also used to verify our results. We determined distinct pathways involved in lung carcinogenesis in ever-smokers and never-smokers and substantial influences of compositional differences in immune cells on patients’ clinical outcome. In particular, we found two subsets of immune cells, mast cells and CD4+ memory T cells, which had completely opposite associations with outcomes in resting and activated states. Several chemokines and their associated receptors (e.g., CXCL11-CX3CR1 axis) were selectively altered in response to cigarette smoking and their abundances showed stronger correlation with fractions of these two immune subsets in ever-smokers than never-smokers. These findings provided a therapeutic opportunity for modulating cancer immunity to prevent tumor invasion and metastasis in lung cancer patients.
Results
Expression and function of dysregulated genes in tumors
We analyzed 160 tumor samples and their corresponding adjacent normal samples across the four datasets (GSE19188, GSE10072, GSE31547, and GSE7670) to investigate lung adenocarcinoma-associated dysregulation of gene expression (). We found that 3,100 genes were consistently differentially expressed between tumor and normal samples among the four datasets. These included 1,720 and 1,380 genes upregulated and downregulated in tumors, respectively, accounting for 16.73% and 11.42% of all genes shared among the four datasets. To characterize the function of these dysregulated genes, pathway enrichment was performed on the upregulated and downregulated gene sets separately. Most of pathways enriched by upregulated genes were involved in cell cycle regulation, cellular stress, and injury functions, whereas pathways enriched by downregulated genes were related to cellular immune response function (). Together, these results revealed aberrant expression and function of signaling pathways in tumor tissues, and there might be different inflammatory response patterns between tumor and normal tissues.
Figure 1. Dysregulated genes and their associated altered pathways in lung adenocarcinoma. (A) Heatmaps for dysregulated genes across four datasets. There are distinct gene expression patterns between tumor and normal tissue samples. (B) Pathway enrichment by dysregulated genes across four datasets. P-values of Fisher’s exact test for pathway enrichments were calculated by Ingenuity Pathway Analysis (IPA).
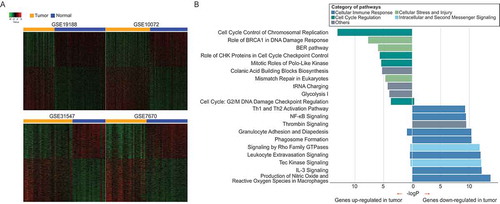
Cigarette smoking causes immune dysfunction and influences clinical outcome
Smoking is a major risk factor for the development of lung cancer. We made use of six datasets (GSE30219, GSE31210, GSE50081, GSE14814, GSE31547, and GSE68465), which contained both smoking status and survival information. In our survival analysis, we integrated patient samples in the above datasets to increase statistical power, but patients with radiotherapy or chemotherapy were excluded in the analysis. In addition, past-smokers and current-smokers were pooled together as ever-smokers because their gene expression profiles are similar [6]. We can conclude from the Kaplan-Meier survival curves that never-smoker patients had a better outcome in overall survival () and recurrence-free survival () than ever-smoker patients. This survival difference remains significantly after accounting for patients’ age, gender and mutation status (Figure S2).
Figure 2. Survival analyses and pathway enrichments for lung cancer patients in ever- and never-smokers. (A) Overall survival of lung adenocarcinoma patients in ever- and never-smokers. (B) Recurrence-free survival of lung adenocarcinoma patients in ever- and never-smokers. (C) Pathways enriched by genes significantly downregulated (left) and upregulated (right) in tumor samples from smokers as compared with those from never-smokers. (D) Pathways enriched by DEGs in the tumor-normal comparison in never-smokers (left) and in ever-smokers (right).
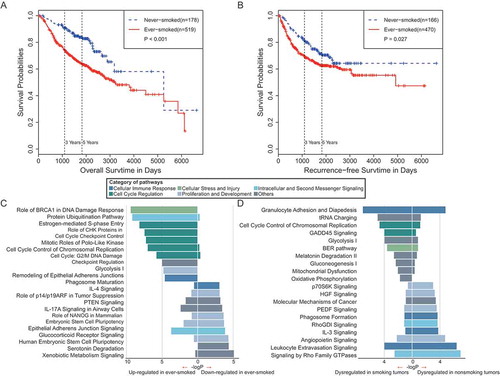
To investigate the mechanism underlying the difference in clinical outcome between smoking and nonsmoking lung cancer, we further analyzed differentially expressed genes (DEGs) between tumor samples exposed to tobacco and those from never-smokers. We detected 2,275 DEGs, including 1,298 and 977 genes upregulated and downregulated in smoking patients, respectively. Similarly, pathway enrichment was applied to these DEGs; we observed that most of the enriched pathways were associated with the cell cycle regulation, proliferation, and development categories ().
To further evaluate the effects of smoking on lung tumorigenesis and progression, we performed a comparison of genes dysregulated in tumor samples from never-smokers and ever-smokers. We identified 1,108 genes as DEGs between tumor and adjacent normal tissues in never-smokers, and 1,732 genes as DEGs between tumor and adjacent normal tissues in ever-smokers. Only 3 of 20 pathways were commonly altered in both never-smokers and ever-smokers, including two pathways related to immune response and a growth signaling pathway by Rho Family GTPase. In particular, IL-3 signaling and Phagosome formation pathways that are involved in cellular immune response were specifically enriched in never-smokers (). Taken together, these results suggested that lung tumors in ever-smokers and never-smokers show distinct alteration of pathways, and that cigarette smoking has dramatic effects in altering cancer signaling pathways and the tumor microenvironment.
Compositional differences in tumor immune cells in ever- and never-smokers
In the preceding sections, all of the results pointed to a key element, the immune system, which may play a pivotal role in tumor development and progression. To better understand the relationship of immune cell infiltration with cancer progression, we identified a relative fraction of immune cell subtypes in samples with different tissue types and smoking status using CIBERSORT.Citation12 CIBERSORT requires an input matrix of reference gene expression signatures (with a total of 547 genes), which are collectively used to estimate the relative proportions of each cell type of interest. This leukocyte signature matrix (termed LM22) that can distinguish 22 different human immune cell subsets was initially constructed as a benchmark for CIBERSORT across cancer types. To make the signature gene matrix more applicable for lung adenocarcinoma, genes which were overexpressed in lung adenocarcinoma cell lines compared to other cancer cell lines were excluded. As the expression of CD138, a marker of plasma cells, is common in human lung cancers,Citation14 which might lead to overestimation of the fraction of plasma cells, we removed genes specifically associated with plasma cells from the signature and only made estimation for the other 21 cell subtypes. To evaluate the robustness and reliability of the refined signature gene matrix, we applied to deconvolution of 1,000 simulated datasets of gene expression profiles generated from LM22 reference samples. Simulations showed an extremely high accuracy for prediction of 21 leukocyte types, suggesting the validity of our refined signature gene matrix for leukocyte deconvolutions (). We then evaluated the comparability of all 11 datasets by calculating the mean value of the relative fraction of each immune subtype. The result confirmed that these datasets had similar tumor immune cell infiltration levels, indicating the homogeneity of tissue collection, process, and storage across these microarray studies ().
Figure 3. Robustness and accuracy of inferring cell compositions using the refined new signature gene matrix, and the comparability of immune cell compositions among 11 datasets. (A) The accuracy of estimating immune cell fractions in simulated mixture gene expression profiles using the refined new signature gene matrix. Heatmap displayed accuracy for each type of immune cells in 1,000 simulations, and barplot showed the median accuracy for each cell subtype in the simulations. (B) Mean tumor immune cell compositions estimated by CIBERSORT across 11 datasets. These datasets were comparable and had similar tumor immune cell infiltration levels.
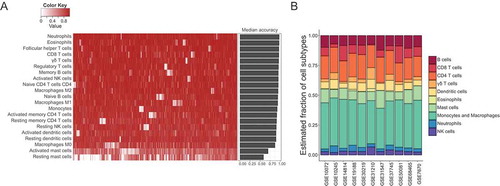
We further estimated the absolute immune infiltration scores in tumor and adjacent normal tissues using CIBERSROT. A significantly higher immune cell content was observed in normal tissues compared to tumor tissues among different datasets (), which showed high degree of concordance with immune scores estimated by xCellCitation13 (Figure S3). Combined with the pathway enrichment results as described above, these findings support that there are different patterns of immune response between tumor and normal tissues. Finally, we estimated the relative fraction of immune cell subtypes and determined their prognostic values among these datasets. We identified 14 kinds of immune cell subtypes that had significantly different fractions between tumor and normal specimens, which were consistent in at least two datasets. Five kinds of immune cells are significantly associated with patient survival, among which resting mast cells, M0 macrophages, activated mast cells and activated CD4+ memory T cells are in accordance with tumor-normal comparisons (). In other words, resting mast cells that were downregulated in tumors as compared with adjacent normal tissues were predictive of favorable outcome, whereas macrophages M0 and activated mast and CD4+ memory T cells that tended to be upregulated in tumors were predictive of adverse outcome. Interestingly, the same compositional differences in these two of three immune cell subtypes were also observed between ever-smokers and never-smokers (). More importantly, resting mast cells and resting CD4+ memory T cells had lower fractions in ever-smokers than never-smokers and were significant predictors of favorable survival outcome, whereas activated CD4+ memory T cells and activated mast cells had higher factions in ever-smokers than never-smokers and were significant predictors of adverse survival outcome.
Figure 4. Immune cell compositions and their association with clinical outcome. (A) Immune cell infiltration score in tumor and adjacent normal tissues. (B) Compositional differences in immune cells between tumor and normal samples (left) and associations of tumor immune cell fractions with survival (right). (C) Compositional differences in immune cells between tumors from ever- and never-smokers (left) and associations of tumor immune cell fractions with survival (right). Meta-z-scores were used to measure the overall association of each type of immune cell with survival across all datasets.
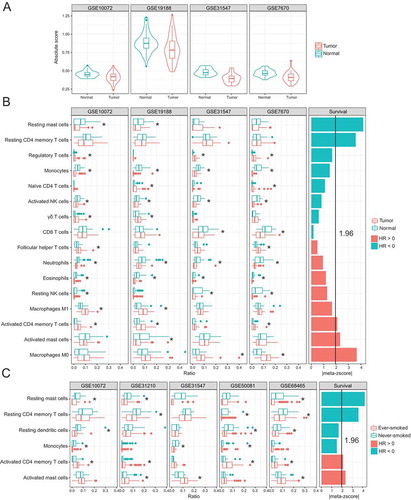
Chemokine/receptor networks are selectively altered in smokers
To further explore the heterogeneity of tumor immune infiltration under different smoking statuses, we conducted linear regression analyses between the fraction of tumor-infiltrating leukocytes and the expression of their related chemokines. In general, both mast cell and CD4+ memory T cell populations show stronger correlations with several chemokines and their associated receptors in ever-smokers than in never-smokers (). For instance, the fraction of mast cells appeared to be related to an abundance of CCR1/CCR5 and their corresponding chemokine ligands in ever-smokers. Conversely, these relationships were much less frequently observed in never-smokers. Likewise, the fraction of CD4+ memory T cells appeared to be related to an abundance of CCR1/CXCR3 and their corresponding chemokine ligands in ever-smokers, whereas these relationships were much less evident in never-smokers. These results suggested that these chemokines were selectively altered as a response to cigarette-smoke exposure and subsequently led to activation of mast cells and CD4+ memory T cells in tumor microenvironments.
Figure 5. Chemokine-receptor networks differ between ever- and never-smokers. Nodes in green are chemokine ligands and nodes in red are corresponding chemokine receptors; edges indicate existing molecular interactions between a chemokine and its receptor. For each study, the association between the gene expression of the chemokine/receptor and tumor-infiltrating immune cell fractions was calculated using a linear regression analysis for mast cells (A and B) and CD4+ memory T cells (C and D) separately, under smoking status (left) and nonsmoking status (right). If and only if both the chemokine ligand and receptor in a pair were significantly associated with the infiltrating immune cell levels, a colored dot representing the study was placed on the edge connecting the chemokine and receptor.
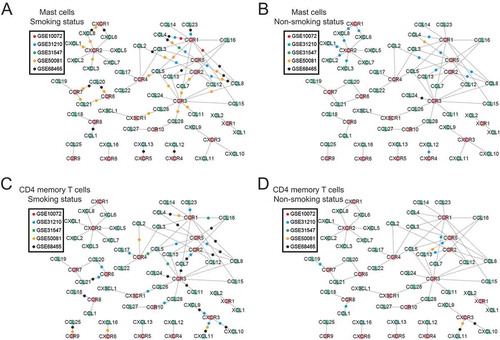
Discussion
Previous studies have investigated the relationship between immune cells and clinical outcomes of lung cancer patients, and the impact of cigarette smoking on the host immunity. Recently, several computational methods have been developed to make use of existing gene expression profiles of complex tissues to estimate tumor infiltrating leukocyte subtypes, including various innate and adaptive immune cells. By incorporating clinical outcome of patients, characterization of immune cell compositions of tumor and adjacent normal tissues could provide new insights into tumor-immune interactions. In this study, we integrated 11 lung cancer microarray studies conducted on GPL570 and GPL96 platforms including 1,111 lung adenocarcinoma and 200 adjacent normal samples, making it one of the largest lung cancer immunological studies. Using the recently developed machine-learning method CIBERSORT and the refined tissue-specific signature gene matrix, we estimated the relative proportion of 21 immune cell subtypes from gene expression profiles in these samples. We discovered the immune compositional patterns in tumor and normal tissues, and in smoking and nonsmoking tumor samples of lung adenocarcinoma.
Hierarchical clustering of dysregulated genes demonstrated a dramatic variation in gene expression in lung tumors compared with adjacent normal tissues (). Interestingly, overexpressed genes were enriched in different pathways between tumors and adjacent normal tissues, with most pathways related to cell cycle control in tumors and cellular immune responses in normal lung tissues. Lung is the organ that has the largest surface in contact with external environment, which not only works for gas exchange, but also plays an important role in immunity Citation15. As expected, a significantly higher immune cell content was observed in normal tissues compared with tumor tissues among different datasets (). Taken together, these results revealed aberrant expression and function of signaling pathways in tumor tissues, and there are different inflammatory response patterns in lung tumor and normal tissues.
We also found that lung tumors in ever-smokers and never-smokers showed distinct pathway alterations (). Only 3 of 20 pathways enriched by DEGs were commonly altered in both ever- and never-smokers. IL-3 signaling and phagosome formation pathways that are involved in cellular immune response were specifically enriched in never-smokers. These results suggested that the molecular pathways involved in the differential pattern of lung carcinogenesis according to smoking status, and cigarette smoking have dramatic effects in altering cancer signaling pathways and the tumor microenvironment.
Never-smokers have markedly better outcomes than ever-smokers (). To reveal this prognostic difference, we determined the relative fraction of immune cell subtypes in samples with different tissue types and smoking status using CIBERSORT. We observed compositional differences in 14 kinds of immune subsets between tumor and adjacent normal samples (). The fractions of most of these subsets were reduced in tumor samples, except for regulatory T cells, gama delta T cells, T cells follicular helper, M1 macrophages, activated CD4+ memory T cells, and M0 macrophages. We then investigated the relationship between these immune cell fractions and survival outcomes. To make a more credible conclusion, we also employed another computational method, xCell, for cell types enrichment analysis using ssGSEA.Citation13 We made a comparison of the influence of leukocyte subtypes on patients’ outcomes between xCell and CIBERSORT. Our comparisons showed high concordance in the association of patients’ outcome with the overlapped leukocyte subtypes (Figure S4).
M0 macrophages and total macrophages were strongly associated with poorer outcomes. A dichotomy has been proposed for macrophage activation: classic vs. alternative, and M1 and M2, respectively. M1 macrophages are activated through interferon (IFN)–γ or lipopolysaccharide (LPS), whereas M2 macrophages are activated through type 2 cytokines including IL-4, IL-13, and IL-10.Citation16 M2 macrophages express chemokines CCL17, CCL22, and CCL24, and their corresponding receptors, which are present on Th2 cells, a cell linked to adverse prognosis in our analysis, and thus participate in amplification of polarized Th2 responses,Citation17 leading to a suppression of immunity. However, we did not observe a significant link between M2 macrophages and patients’ outcomes in our datasets, and further studies are needed to examine M2 macrophage functions in NSCLC. In addition, the immune score of CD8 + T cells was associated with better outcome of NSCLC patients in the analysis with xCell, whereas the similar tendency remained but was not significant in the analysis with CIBERSORT. CD8 + T cells were previously reported to associate with favorable prognosis in colorectal cancer, ovarian cancer and breast cancer,Citation18 but it seems that the impact of CD8 + T cells on NSCLC patients was controversial. In stage IV NSCLC patients with chemotherapy, CD8+ T cells in cancer nest link to a favorable outcome,Citation19 but some other studies suggested that they could not demonstrate an influence on survival of lung adenocarcinoma patients.Citation20–Citation22 A recent study using in silico analysis of tumor immunity also underpinned this conclusion, when taking patient age and stage into consideration.Citation23
In addition to macrophages, we caught identified two subsets of immune cells, namely mast cells and CD4+ memory T cells, which had completely opposite associations with outcomes in resting and activated status. The fractions of activated mast cells and CD4+ memory T cells were higher in ever-smokers than in never-smokers, whereas the resting status of these two were exactly opposite of activated status. The activated forms are adverse predictors of prognosis, whereas the resting forms are favorable predictors of prognosis. Our findings suggested that aberrant activation of these two immune subtypes plays a crucial role in cigarette smoking-induced immune dysfunction in the lung, which might contribute to tumor development and progression. Regarding the impact of mast cells on lung cancer patient outcomes, both adverse and favorable, several contradictory reports have been published.Citation24,Citation25 This is perhaps due to the mixture of resting and activated mast cells in the previous studies. Interestingly, when resting and activated mast cells were combined together, they were significantly correlated with prolonged survival of NSCLC patients (Figure S3). Therefore, separating these two types of mast cells is necessary for more credible conclusions, otherwise, the function of activated mast cells might be masked by resting mast cells. Our survival analysis confirmed the potential dual role of mast cells in tumor progression and metastasis. The most well-known activation method for mast cells is engagement of the high-affinity receptor for IgE immunoglobulins (FcεRI).Citation26 Upon activation, mast cells can produce and release a vast array of mediators. These mediators lead to crosstalk with immune cells and tumor cells, and separately contribute to the formation of an immunosuppressive environment, and enhance tumor cell activity.Citation27 Furthermore, an association of cigarette smoking with a rise of mast cells was observed.Citation28 Increasing evidence now implies that mast cells promote expression of pro-inflammatory chemokines.Citation29 Taken together, the existing evidence and our results support a link between cigarette smoking and the activation of mast cells and their involvement in tumor progression and metastasis. Although our findings also revealed that CD4+ memory T cells were strongly associated with patients’ survival, such associations have rarely been reported. Future studies are required to further confirm this finding and investigate the mechanisms of CD4+ memory T cell activation in tumor microenvironments under smoking status.
We also found that both mast cell and CD4+ memory T cell levels were significantly associated with an abundance of CCL5-relevant chemokine receptors. This relationship was much more evident in ever-smokers compared with never-smokers. CCL5 is released in the lung in response to many noxious stimuli,Citation30 and an increase of CCL5 expression was found in smoking status.Citation31 By interacting with certain receptors on memory T cells,Citation18 CCL5 might induce recruitment and activation of particular memory T cells. CCR5 is the most well-known receptor for CCL5. Previous studies also demonstrate that the CCL5-CCR5 axis plays an active role in tumor development, acts as a growth factor, stimulates angiogenesis, and participates in immune evasion mechanisms.Citation32 Furthermore, the CXCL11-CX3CR1 axis performed differently between smoking and nonsmoking status in our analysis, which related to angiogenesis and metastasis of tumors in previous experiments.Citation33 Considering all the evidence above, smoking has the potential to affect the expression of certain chemokines, thereby leading to activation of CD4+ memory T cells and promoting the development and progression of tumor.
Lastly, several caveats for our findings should be acknowledged. Firstly, an important assumption made by CIBERSORT and other in silico approaches is that the gene expression profiles of pure primary immune cells extracted from peripheral blood mononuclear cells (PBMC) do not significantly differ from that in tumor tissues. However, in silico approach is a valuable approach to study tumor infiltration and can make use of existing historical datasets which includes thousands of patients. In the present study, we performed integrative analysis of 11 lung cancer gene-expression datasets, including 1,111 lung adenocarcinomas and 200 adjacent normal lung samples. This represents the largest study of tumor infiltration in lung cancer. Secondly, the immune system is extremely complex and contains various innate and adaptive immune cells. Although our refined signature gene matrix showed high robustness and reliability of inferring immune cell compositions of complex tissues, the number of leukocyte types that can be distinguished by this signature matrix is rather limited (only 21). Further studies are required to develop a new robust signature gene matrix to include as many immune cell types as possible.
In summary, this study characterized compositional patterns of immune cells in lung adenocarcinomas in different tissue types and smoking status, and revealed the complex association between immune composition and clinical outcomes. The status of these cells switched from resting to activated in mast cells and CD4+ memory T cells might manifest some important processes induced by cigarette smoking, which subsequently contributes to tumor development and progression. These immune subsets are not only valuable prognostic biomarkers, but are also potential targets for anti-cancer immunotherapy.
Methods
Sample collection and data processing
This study made use of public online lung cancer data. To reduce tumor heterogeneity, we focused on lung adenocarcinoma, a common subtype of non-small cell lung cancer. We queried Gene Expression Omnibus (GEO) with the keyword “lung” and with the platform set to “GPL570” or “GPL96,” and then selected datasets with a sample size of more than 30. As a result, we got six datasets on platform GPL570, including GSE10245 (n = 40),Citation34 GSE19188 (n = 110),Citation35 GSE30219 (n = 83),Citation36 GSE31210 (n = 224),Citation37,Citation38 GSE37745 (n = 91),Citation39 and GSE50081 (n = 128),Citation40 and five datasets on platform GPL96, including GSE10072 (n = 107),Citation41 GSE14814 (n = 71),Citation42 GSE31547 (n = 50),Citation43 GSE68465 (n = 353),Citation44 and GSE7670 (n = 54).Citation45 Detailed information about the datasets is presented in ; the sample numbers are the result of removing patients treated with chemotherapy or radiotherapy.
Table 1. Clinical summary of patients in the analyzed studies.
Raw CEL files and SOFT files were obtained from GEO datasets. Each dataset was converted to MAS5 normalized and fRMA normalized data by the R package “affy,” and mapped to NCBI Entrez gene symbols by a custom CDF (Chip Definition File; Brainarray version 20.0.0; http://brainarray.mbni.med.umich.edu/Brainarray/Database/CustomCDF/20.0.0/entrezg.asp) according to the CIBERSORT method.Citation12 When more than one probes mapped to the same gene symbol, the highest average expression probe was incorporated. Clinical data was extracted from the SOFT file using the R package “GEOquery.” Samples with adjuvant radiotherapy or chemotherapy were excluded in the following analysis, and only tissue types with lung adenocarcinoma were included in the analysis.
Gene expression analysis and pathway enrichment
We used the R package “limma” to find differentially expressed genes (DEGs) associated with smoking status (ever-smoked/never-smoked) and tissue type (tumor/adjacent normal). Bonferroni correction was used to adjust p-values. GSE19188, GSE10072, GSE31547, and GSE7670 were used for identifying DEGs between tumor and normal tissues, whereas GSE30219, GSE31210, GSE50081, GSE10072, GSE31547, and GSE68465 were used for identifying DEGs between ever-smoked and never-smoked groups. Dysregulated genes refer to either up-regulated or down-regulated DEGs with adjusted p value < 0.05. In the analysis, samples with current smokers or former smokers were assigned to the ever-smoked group, because their gene expressions were similar [6].
Overlapped significant DEGs (adjusted P-value ≤ 0.05) were divided into adverse and favorable groups on the basis of their expression profile. Specifically, in the tumor-normal comparison, DEGs upregulated in tumor tissues were assigned to the adverse group, whereas those upregulated in normal tissues were assigned to the favorable group. In the comparison of ever-smoked and never-smoked, DEGs upregulated in tumor tissues from smoking patients were assigned to the adverse group, whereas those upregulated in tumor tissues from nonsmoking patients were assigned to the favorable group. We then performed pathway enrichment analysis on these DEGs separately using Ingenuity Pathway Analysis (IPA) through Fisher’s exact test, and compared outcomes of adverse and favorable DEGs. In order to investigate different functions between these two groups of DEGs, we only displayed the pathways which were enriched differently between these two situations.
Inference of infiltrating immune cells from gene expression profiles
To determine the fraction of immune cells in tumors, we applied a linear support vector regression-based method, CIBERSORT,Citation12 to estimate the relative ratios of 21 leukocytes. At the same time, CIBERSORT produces an empirical P-value for each sample and tests the null hypothesis that there are no cell types in the tested sample. Samples with a P-value ≥ 0.05 were eliminated in the following analysis. We performed CIBERSORT in R and the source code of CIBERSORT (R version 1.03) was downloaded from the CIBERSORT website (https://cibersort.stanford.edu). The absolute infiltration score was defined as the median expression level of all genes in the signature gene matrix divided by the median expression level of all genes in the mixture.Citation12 A permutation test was then applied to evaluate differential distribution of these inferred immune cell subtypes in groups using the R package “permute”. To make a more credible conclusion, we also used another computational method, xCell,Citation13 which provides immune scores for 64 cell subtypes, spanning multiple innate and adaptive immune cells. xCell is a method for cell types enrichment analysis using ssGSEA, and it employs a spillover compensation technique to reduce dependencies between closely related cell types. Immune scores of xCell were obtained by R package “xCell” for each sample.
Construction of a new signature gene matrix
To generate a lung adenocarcinoma specific signature gene matrix, we removed genes that were overexpressed in lung adenocarcinoma cell lines compared to other cancer cell lines. Expression data of cell lines were downloaded from Cancer Cell Line Encyclopedia (CCLE)Citation46,Citation47 and were normalized by Robust Multiarray Averaging (RMA) method. Probesets annotation and differential gene expression were carried out as described above. The overexpressed genes were defined as adjusted p-value ≤ 0.05, and log2(Fold-change) ≥ 1. In our preliminary estimation, we observed an overestimation on plasma cells. We thus removed differentially expressed genes which only contributed to deconvolution of plasma cells. In addition, we made adaptive changes for these datasets, and genes absent in the custom CDF were excluded from the signature gene matrix.
We generated gene expression profiles from 21 immune cell profiles by randomly assigning a fraction for each kind of cells, and applied CIBERSROT to these simulated gene expression profiles to estimate the relative ratio of these immune cells. The performance of the refined new signature gene matrix was assessed by accuracy, which was defined as follows: .
Survival analysis
The association of survival with each type of immune cell was evaluated using the univariate Cox proportional hazards model. P-values and hazard ratios with a 95% confidence interval and z-scores were estimated. Survival distributions in different groups were visualized using Kaplan-Meier curves, and the significance was assessed by a log-rank test. The events of overall survival were defined as death, while recurrence-free survival was ended by any disease recurrence or death. Survival analyses were performed using the R package “survival.”
To generate a stable outcome for survival analysis, we incorporated meta-z-scores to access the influence of each type of immune cell on all datasets for CIBERSORT and xCell. Z-scores for each immune subtype were summarized in a single meta-z-score using Lipták’s weighted average; the formula is as follows:
where is the z-score of the i-th study and
is the square root of sample size of the i-th study.
Linear regression analysis
We performed a linear regression analysis with the fraction of immune cell as a dependent variable and with expression levels of chemokine receptor/ligand as independent variables. The significant association of the fraction of immune cell with expression levels of chemokines meets the following criteria: 1) Benjamin-Hochberg correction for p-value of the regression model ≤ 0.05, and 2) raw P-value for both ligand and receptor in the model ≤ 0.05.
Conflict of interest
The authors disclose no potential conflicts of interest.
Authors‘ contributions
Enguo Chen, Pengyuan Liu and Yan Lu considered and designed the study. Xufan Li, Jia Li and Pin Wu performed the data analysis. Enguo Chen, Pengyuan Liu, Yan Lu, and Xufan Li wrote the manuscript. All of the authors discussed and commented the study.
Supplemental Material
Download PDF (928.3 KB)Acknowledgments
We thank the Bioinformatics Core Facility at Zhejiang University School of Medicine for providing computing capacity and anonymous reviewers for reading and commenting on the manuscript.
SUPPLEMENTAL DATA
Supplementary data for this article can be accessed here
Additional information
Funding
References
- Torre LA, Bray F, Siegel RL, Ferlay J, Lortet-Tieulent J, Jemal A. Global cancer statistics, 2012. Cancer J Clin. 2015;65:87–108. doi:10.3322/caac.21262.
- Thun MJ, Hannan LM, Adams-Campbell LL, Boffetta P, Buring JE, Feskanich D, et al. Lung cancer occurrence in never-smokers: an analysis of 13 Cohorts and 22 cancer registry studies. PLoS Med. 2008. doi:10.1371/journal.pmed.0050185.
- Pao W, Miller V, Zakowski M, Doherty J, Politi K, Sarkaria I, et al. EGF receptor gene mutations are common in lung cancers from “never smokers” and are associated with sensitivity of tumors to gefitinib and erlotinib. Proc Natl Acad Sci. 2004;101:13306–13311. doi:10.1073/pnas.0405220101.
- Ahrendt SA, Decker PA, Alawi EA, Zhu Yr YR, Sanchez-Cespedes M, Yang SC, et al. Cigarette smoking is strongly associated with mutation of the K-ras gene in patients with primary adenocarcinoma of the lung. Cancer. 2001;92:1525–1530.
- Suzuki H, Takahashi T, Kuroishi T, Suyama M, Ariyoshi Y, Takahashi T, et al. p53 mutations in non-small cell lung cancer in Japan: association between mutations and smoking. Cancer Res. 1992;52:734–736.
- Husgafvel-Pursiainen K, Boffetta P, Kannio A, Nyberg F, Pershagen G, Mukeria A, et al. p53 mutations and exposure to environmental tobacco smoke in a multicenter study on lung cancer. Cancer Res. 2000;60:2906–2911.
- Goncalves RB, Coletta RD, Silverio KG, Benevides L, Casati MZ, Da Silva JS, et al. Impact of smoking on inflammation: overview of molecular mechanisms. Inflamm Res. 2011;60:409–424. doi:10.1007/s00011-011-0308-7.
- Quail D, Joyce J. Microenvironmental regulation of tumor progression and metastasis. Nat Med. 2013;19:1423–1437. doi:10.1038/nm.3394.
- Mountzios G, Fouret P, Soria JC. Mechanisms of disease: signal transduction in lung carcinogenesis – a comparison of smokers and never-smokers. Nat Clin Pract Oncol. 2008;5:610–618. doi:10.1038/ncponc1181.
- Wong MP, Fung LF, Wang E, Chow WS, Chiu SW, Lam WK, et al. Chromosomal aberrations of primary lung adenocarcinomas in nonsmokers. Cancer. 2003;97:1263–1270. doi:10.1002/cncr.11183.
- Zeka A, Mannetje A, Zaridze D, Szeszenia-Dabrowska N, Rudnai P, Lissowska J, et al. Lung cancer and occupation in nonsmokers: a multicenter case-control study in Europe. Epidemiology. 2006;17:615–623. doi:10.1097/01.ede.0000239582.92495.b5.
- Newman AM, Liu CL, Green MR, Gentles AJ, Feng W, Xu Y, et al. Robust enumeration of cell subsets from tissue expression profiles. Nat Methods. 2015;12:453–457. doi:10.1038/nmeth.3337.
- Aran D, Hu Z, Butte AJ. xCell: digitally portraying the tissue cellular heterogeneity landscape. Genome Biol. 2017;18:220. doi:10.1186/s13059-017-1349-1.
- Toyoshima E, Ohsaki Y, Nishigaki Y, Fujimoto Y, Kohgo Y, Kikuchi K. Expression of syndecan-1 is common in human lung cancers independent of expression of epidermal growth factor receptor. Lung Cancer. 2001;31:193–202.
- Bienenstock J. The lung as an immunologic organ. Annu Rev Med. 1984;35:49–62. doi:10.1146/annurev.me.35.020184.000405.
- Ruffell B, Affara NI, Coussens LM. Differential macrophage programming in the tumor microenvironment. Trends Immunol. 2012;33:119–126. doi:10.1016/j.it.2011.12.001.
- Biswas SK, Mantovani A. Macrophage plasticity and interaction with lymphocyte subsets: cancer as a paradigm. Nat Immunol. 2010;11:889–896. doi:10.1038/ni.1937.
- Fridman WH, Pages F, Sautes-Fridman C, Galon J. The immune contexture in human tumours: impact on clinical outcome. Nat Rev Cancer. 2012;12:298–306. doi:10.1038/nrc3245.
- Kawai O, Ishii G, Kubota K, Murata Y, Naito Y, Mizuno T, et al. Predominant infiltration of macrophages and CD8(+) T Cells in cancer nests is a significant predictor of survival in stage IV nonsmall cell lung cancer. Cancer. 2008;113:1387–1395. doi:10.1002/cncr.23712.
- Trojan A, Urosevic M, Dummer R, Giger R, Weder W, Stahel RA. Immune activation status of CD8+T cells infiltrating non-small cell lung cancer. Lung Cancer. 2004;44:143–147. doi:10.1016/j.lungcan.2003.11.004.
- Wakabayashi O, Yamazaki K, Oizumi S, Hommura F, Kinoshita I, Ogura S, et al. CD4+ T cells in cancer stroma, not CD8+ T cells in cancer cell nests, are associated with favorable prognosis in human non-small cell lung cancers. Cancer Sci. 2003;94:1003–1009.
- Hiraoka K, Miyamoto M, Cho Y, Suzuoki M, Oshikiri T, Nakakubo Y, et al. Concurrent infiltration by CD8(+) T cells and CD4(+) T cells is a favourable prognostic factor in non-small-cell lung carcinoma. Br J Cancer. 2006;94:275–280. doi:10.1038/sj.bjc.6602934.
- Li B, Severson E, Pignon JC, Zhao HQ, Li TW, Novak J, et al. Comprehensive analyses of tumor immunity: implications for cancer immunotherapy. Genome Biol. 2016;17. doi:10.1186/s13059-016-1028-7.
- Welsh TJ, Green RH, Richardson D, Waller DA, O’Byrne KJ, Bradding P. Macrophage and mast-cell invasion of tumor cell islets confers a marked survival advantage in non-small-cell lung cancer. J Clinical Oncology. 2005;23:8959–8967. doi:10.1200/JCO.2005.01.4910.
- Imada A, Shijubo N, Kojima H, Abe S. Mast cells correlate with angiogenesis and poor outcome in stage I lung adenocarcinoma. Eur Respir J. 2000;15:1087–1093.
- Rivera J, Gilfillan AM. Molecular regulation of mast cell activation. J Allergy Clin Immunol. 2006;117:1214–1225. quiz 26. doi:10.1016/j.jaci.2006.04.015.
- Rigoni A, Colombo MP, Pucillo C. The role of mast cells in molding the tumor microenvironment. Cancer Microenviron. 2015;8:167–176. doi:10.1007/s12307-014-0152-8.
- Small-Howard A, Turner H. Exposure to tobacco-derived materials induces overproduction of secreted proteinases in mast cells. Toxicol Appl Pharmacol. 2005;204:152–163. doi:10.1016/j.taap.2004.09.003.
- Mortaz E, Redegeld FA, Sarir H, Karimi K, Raats D, Nijkamp FP, et al. Cigarette smoke stimulates the production of chemokines in mast cells. J Leukoc Biol. 2008;83:575–580. doi:10.1189/jlb.0907625.
- Moran CJ, Arenberg DA, Huang CC, Giordano TJ, Thomas DG, Misek DE, et al. RANTES expression is a predictor of survival in stage I lung adenocarcinoma. Clin Cancer Res. 2002;8:3803–3812.
- Di Stefano A, Caramori G, Gnemmi I, Contoli M, Bristot L, Capelli A, et al. Association of increased CCL5 and CXCL7 chemokine expression with neutrophil activation in severe stable COPD. Thorax. 2009;64:968–975. doi:10.1136/thx.2009.113647.
- Aldinucci D, Colombatti A. The inflammatory chemokine CCL5 and cancer progression. Mediators Inflamm. 2014;2014:1–12. doi:10.1155/2014/292376.
- Chandrasekar B, Mummidi S, Perla RP, Bysani S, Dulin NO, Liu F, et al. Fractalkine (CX3CL1) stimulated by nuclear factor kappaB (NF-kappaB)-dependent inflammatory signals induces aortic smooth muscle cell proliferation through an autocrine pathway. Biochem J. 2003;373:547–558. doi:10.1042/BJ20030207.
- Kuner R, Muley T, Meister M, Ruschhaupt M, Buness A, Xu EC, et al. Global gene expression analysis reveals specific patterns of cell junctions in non-small cell lung cancer subtypes. Lung Cancer-J Iaslc. 2009;63:32–38. doi:10.1016/j.lungcan.2008.03.033.
- Hou J, Aerts J, Den Hamer B, Van Ijcken W, Den Bakker M, Riegman P, et al. Gene expression-based classification of non-small cell lung carcinomas and survival prediction. PloS One. 2010;5:e10312. doi:10.1371/journal.pone.0010312.
- Rousseaux S, Debernardi A, Jacquiau B, Vitte AL, Vesin A, Nagy-Mignotte H, et al. Ectopic activation of germline and placental genes identifies aggressive metastasis-prone lung cancers. Sci Transl Med. 2013;5:186ra66. doi:10.1126/scitranslmed.3005723.
- Okayama H, Kohno T, Ishii Y, Shimada Y, Shiraishi K, Iwakawa R, et al. Identification of genes upregulated in ALK-positive and EGFR/KRAS/ALK-negative lung adenocarcinomas. Cancer Res. 2012;72:100–111. doi:10.1158/0008-5472.CAN-11-1403.
- Yamauchi M, Yamaguchi R, Nakata A, Kohno T, Nagasaki M, Shimamura T, et al. Epidermal growth factor receptor tyrosine kinase defines critical prognostic genes of stage I lung adenocarcinoma. PloS One. 2012;7:e43923. doi:10.1371/journal.pone.0043923.
- Botling J, Edlund K, Lohr M, Hellwig B, Holmberg L, Lambe M, et al. Biomarker discovery in non-small cell lung cancer: integrating gene expression profiling, meta-analysis, and tissue microarray validation. Clin Cancer Res. 2013;19:194–204. doi:10.1158/1078-0432.CCR-12-1139.
- Der SD, Sykes J, Pintilie M, Zhu CQ, Strumpf D, Liu N, et al. Validation of a histology-independent prognostic gene signature for early-stage, non-small-cell lung cancer including stage IA patients. J Thoracic Oncol. 2014;9:59–64. doi:10.1097/JTO.0000000000000042.
- Landi MT, Dracheva T, Rotunno M, Figueroa JD, Liu H, Dasgupta A, et al. Gene expression signature of cigarette smoking and its role in lung adenocarcinoma development and survival. PloS One. 2008;3:e1651. doi:10.1371/journal.pone.0001651.
- Zhu CQ, Ding K, Strumpf D, Weir BA, Meyerson M, Pennell N, et al. Prognostic and predictive gene signature for adjuvant chemotherapy in resected non-small-cell lung cancer. J Clinical Oncology. 2010;28:4417–4424. doi:10.1200/JCO.2009.26.4325.
- Girard LMJ, Gerald WL, Saintigny P, Zhang L. MSKCC—a primary lung cancer specimens. Gene Express Omnibus GSE31547; 2011.
- Shedden K, Taylor JM, Enkemann SA, Tsao MS, Yeatman TJ, Gerald WL, et al. Gene expression-based survival prediction in lung adenocarcinoma: a multi-site, blinded validation study. Nat Med. 2008;14:822–827. doi:10.1038/nm.1790.
- Su LJ, Chang CW, Wu YC, Chen KC, Lin CJ, Liang SC, et al. Selection of DDX5 as a novel internal control for Q-RT-PCR from microarray data using a block bootstrap re-sampling scheme. BMC Genomics. 2007;8:140. doi:10.1186/1471-2164-8-109.
- Barretina J, Caponigro G, Stransky N, Venkatesan K, Margolin AA, Kim S, et al. The cancer cell line encyclopedia enables predictive modelling of anticancer drug sensitivity (vol 483, pg 603, 2012). Nature. 2012;492:290. doi:10.1038/nature11798.
- Stransky N, Ghandi M, Kryukov GV, Garraway LA, Lehar J, Liu M, et al. Pharmacogenomic agreement between two cancer cell line data sets. Nature. 2015;528:84.