ABSTRACT
Serum autoantibodies that react with tumor-associated antigens (TAAs) can be used as potential biomarkers for diagnosis of cancer. This study aims to evaluate the immunodiagnostic value of 11 anti-TAAs autoantibodies for detection of breast cancer (BC) and establish a diagnostic model for distinguishing BC from normal human controls (NHC) and benign breast diseases (BBD). Sera from 10 BC patients and 10 NHC were used to detect 11 anti-TAAs autoantibodies by western blotting. The 11 anti-TAAs autoantibodies were further assessed in 983 sera by relative quantitative enzyme-linked immunosorbent assay (ELISA). Binary logistic regression and Fisher linear discriminant analysis were conducted to establish a prediction model by using 184 BC and 184 NHC (training cohort, n = 568) and validated by leave-one-out cross-validation. Logistic regression model was selected to establish the prediction model. Results were validated using an independent validation cohort (n = 415). The five anti-TAAs (p53, cyclinB1, p16, p62, 14-3-3ξ) autoantibodies were selected to construct the model with the area under the curve (AUC) of 0.943 (95% CI, 0.919–0.967) in training cohort and 0.916 (95% CI, 0.886–0.947) in the validation cohort. In the identification of BC and BBD, AUCs were 0.881 (95% CI, 0.848–0.914) and 0.849 (95% CI, 0.803–0.894) in training and validation cohort, respectively. In summary, our study indicates that the immunodiagnostic model can distinguish BC from NHC and BC from BBD and this model may have a potential application in immunodiagnosis of breast cancer.
1. Introduction
Breast cancer (BC) is the most commonly diagnosed cancer and the leading cause of cancer-related death among women worldwideCitation1 According to the data from GLOBOCAN 2018, BC alone accounts for 24.2% of all cancer cases and 15.0% of all cancer deaths among femalesCitation1 So far, the etiology of BC has not been completely revealed. As a result, it is imperative to develop strategies for screening, early diagnosis, and monitoring prognosis as well as risk stratification to decrease mortality and increase the possibility of curing the disease. At present, mammography screening for BC is widely available in many countries. However, not all breast cancers can be visualized on screening mammogramsCitation2 Both sensitivity and specificity of mammography are lower, especially in breast tissues that are extremely dense and also in younger women.Citation3,Citation4 Therefore, it is not recommended for women with average risk and under the age of 50 yearsCitation5 In addition, mammography can not only lead to overdiagnosis, but also makes women experience the harms and side effects of treatment.Citation6,Citation7 In comparison, serological tests based on the tumor-associated biomarkers could be a good way to remedy some defects of mammographyCitation.8
Recently, with the development of proteomic technologies, a variety of tumor-associated antigens (TAAs) have been identified and employed in immunoassay.Citation9–Citation11 Meanwhile, many researchers have demonstrated that serum anti-TAAs autoantibodies may be useful as biomarkers for cancer immunodiagnosis.Citation12–Citation17 In 1982, the anti-p53 autoantibody, for the first time, was reported to be existing in sera from patients with BCCitation18 Since then, many other anti-TAAs autoantibodies were detected and evaluated in BC diagnosis. Autoantibodies against p53, MUC1, and HER2 were the ones proposed mostly among those autoantibodies. It is universally acknowledged that anti-p53 antibody exists in the sera of various cancer patients and has certain value in the diagnosis of cancer.Citation19 MUC1 was the most commonly used antigen in BC detection.Citation20,Citation21 However, Burford et al. investigated a large number of sera which were collected prospectively, and they demonstrated that autoantibodies to MUC1 are unlikely to be useful as a screening test for BCCitation22 Besides, the diagnostic value of anti-HER2 antibody seems not superior to other reported autoantibodies to the corresponding antigens involved in cell cycle regulation (cyclinB1, cyclinD1, and p16), apoptosis regulation (survivin), cell proliferation (c-myc) and shield mRNAs from natural physiological degradation (IMP1, p62, koc).Citation23–Citation28 Moreover, previous studies confirmed that the detection of single autoantibody usually offers insufficient diagnostic power, but the detection of multiple autoantibodies to TAAs was shown to be more sensitive.Citation27,Citation29,Citation30 However, those studies have their own disadvantages, such as less sample size, lack of benign tumor control group, no prediction model or failure to set external validation group.
In this study, the immunodiagnostic values of 11 anti-TAAs (p53, cyclinB1, cyclin D1, p16, p62, IMP1, koc, c-myc, RalA, survivin, 14-3-3ξ) autoantibodies were evaluated. The existence of the 11 autoantibodies had been demonstrated in the serum samples from patients with hepatocellular carcinoma, ovarian and gastric cancer, which were collected in the previous study.Citation29,Citation31–Citation34 Then, an optimal prediction model of multiple autoantibodies was constructed to detect BC with a higher diagnostic value. Both of the Binary logistic regression and Fisher linear discriminant analysis models were established to predict BC based on the relative concentrations of serum autoantibodies. An independent validation cohort was incorporated to verify the prediction model.
2. Materials and methods
2.1 Serum samples
The case–control study included two cohorts of cases from two different hospitals. The training cohort sera from 184 BC patients were obtained from a hospital in Henan Province, China, from March 2018 to May 2018. The validation cohort consisting of 197 BC patients were recruited from another hospital of Henan Province, China, between February 2011 and June 2014. All cancer sera were collected once patients were diagnosed and before receiving any treatment. Two normal control groups (training cohort 184, validation cohort 109) and patients (training cohort 200, validation cohort 109) with benign breast disease (BBD) were enrolled in this study from December 2017 to March 2018 at a hospital in Henan province, China. All female cases were age-matched with participants in benign breast diseases and normal control groups. The Institutional Review Board of Zhengzhou University approved the study. All participants enrolled in the study signed an informed consent form. The characteristics of study populations are shown in .
Table 1. Characteristics of study participants
2.2 Recombinant TAAs
Two tumor-associated antigens, 14-3-3ξ and RalA were constructed and purified using the same approach as used in our previous study.Citation27,Citation31 The rest of TAAs, including p53, cyclin B1, cyclin D1, p16, IMP1, p62, koc, c-myc, survivin, were derived from our previous studies.Citation31,Citation34
2.3 Western blotting
Ten patients with BC and 10 normal individuals were randomly selected to examine the reactivity of sera autoantibodies to TAAs by western blotting analysis. The protocol for western blotting analysis was performed as described in more detail in our previous study.Citation14,Citation31
2.4 Relative quantitative enzyme-linked immunosorbent assay (ELISA)
A relative quantitative ELISA, which was previously used by Zhang et al.Citation35 was adopted to detect serum autoantibodies in patients with BC. In brief, a group of IgG gradients, coupled with one blank control, were arranged on the last two columns of wells in duplicate to provide the standard curve. Next, purified recombinant TAAs were tested by ELISA that described in our previous studyCitation29 A solution of 3, 3ʹ, 5, 5ʹ-tetramethyl benzidine (TMB)-H2O2-urea was used as the detecting agent and 50 ul 2M sulfuric acid was added into each well as the stopping solution. The optical density (OD) of each well was read at 450 and 620nm by a Microplate reader (Thermo Fisher Scientific).
2.5 Statistical analysis
The optical density (OD) of each well in each sample was converted into relative concentrations of autoantibodies using the standard curve. Since the concentration of autoantibodies existing in sera was not distributed normally (Kolmogorov–Smirnov), Kruskal–Wallis H test and Mann–Whitney U test were used for comparison of the three groups (p-value was obtained by exact test, the α value was adjusted by Bonferroni correction). The Chi-square test and Fisher exact test were performed to determine the positive rate of individual autoantibody or a panel of autoantibodies among BC, BBD, and NHC. A p-value <0.0167 (Bonferroni correction, two-tails) was considered statistically significant. A backward stepwise logistic regression (LR) model was conducted to select a panel of autoantibodies based on the training cohort. The two-class Fisher linear discriminant analysis model was also established (stepwise method). The performance of the two models was compared using leave-one-out cross-validation (LOOCV). The area under the receiver operating characteristics (ROC) was constructed to examine the diagnostic value of single autoantibody and the diagnostic performance of the prediction model. Meanwhile, the Youden’s index, positive predictive value (PPV), negative predict value (NPV), and kappa value were calculated as an attempt to evaluate the validity and reliability of the diagnostic test based on sera autoantibodies as biomarkers.
The cutoff value was set at the maximum Yoden index when the specificity was greater than 95%. All statistical analyses were performed by IBM SPSS Statistics 21.0 and GraphPad Prism 5.
3. Results
3.1 Reactivity of sera autoantibodies among 10 BC and 10 NHC by western blotting
Ten patients with BC and correspondingly age-matched 10 normal controls were randomly selected to explore the reactivity of autoantibodies to 11 TAAs (). For the 10 BC patients, the number of positive reactivity of serum autoantibodies ranged from 3 to 6 autoantibodies, more than that of 10 controls showing 1 or 2 autoantibody-positive responses ().
3.2 Autoantibodies in BC
A total of 983 serum samples were collected from three groups of participants (). Eleven purified recombinant proteins were used as coating antigens to detect anti-TAAs autoantibodies in the sera from BC, BBD and NHC groups. The dilution gradients of IgG were explored and demonstrated to have a good fit of a linear relationship between the amounts of coating IgG and OD values. This relationship could be used to convert the original OD values into relative concentrations of autoantibodies (Supplemental Figure 1). The relative concentrations of 11
Anti-TAAs autoantibodies are shown in . Eight anti-TAAs (p53, cyclinB1, p16, p62, c-myc, RalA, survivin, 14-3-3ξ) autoantibodies in BC were significantly increased in the two cohorts compared to NHC (). The five anti-TAAs (p53, cyclinB1, p16, p62, 14-3-3ξ) autoantibodies had significantly different levels between BC and BBD (). The area under the curve (AUC) of the individual autoantibody ranged from 0.527 to 0.779, and the sensitivity ranged between 2.2% and 41.8% in two cohorts when the specificity was greater than 95% (Supplemental Figure 2 and Supplemental Figure 3).
Table 2. Serum relative concentration levels of individual autoantibody among breast cancer patients, breast benign disease and normal individual
Figure 3. Scatter plots of sera relative concentration levels of individual autoantibody among breast cancer patients (NTC = 184, NVC = 197), benign breast disease (NTC = 200, NVC = 109) and normal individual (NTC = 184, NVC = 109) in two cohorts (TC, training cohort; VC, validation cohort). BC, breast cancer; NHC, normal human controls; BBD, benign breast disease
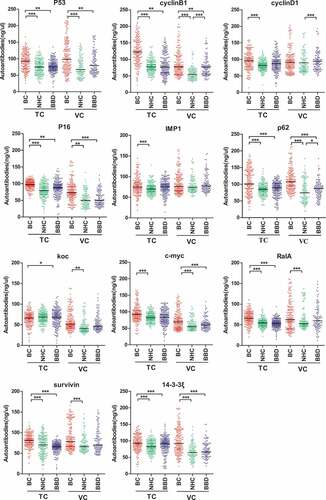
3.3 Establishment of immunodiagnostic model to distinguish BC from BBD or NHC
The serum samples of 184 BC and 184 NHC in the training cohort were selected to establish LR model and Fisher linear discriminant analysis model (). The dependent variable was whether a participant was considered as BC or not. Eight anti-TAAs autoantibodies with different expression levels between BC and NHC were used as independent variables. The logistic regression model with five anti-TAAs autoantibodies was produced as follows: Logit (p = BC) = – 9.833 + 0.024 × p53 + 0.040 × CyclinB1 + 0.019 × p16 + 0.028 × p62 + 0.022 × 14-3-3ξ. Furthermore, based on the eight autoantibodies, a Fisher linear discriminant analysis model was also constructed to separate participants into the BC group and a NHC group. The six anti-TAAs (p53, CyclinB1, p16, p62, 14-3-3ξ, survivin) autoantibodies were identified to be potential biomarkers in BC. The Classification function (Cf) for distinguishing BC from healthy people was obtained as: Cf 1 = 0.035 × p53 + 0.041 × CyclinB1 + 0.045 × p16 + 0.065× p62 + 0.028 × 14-3-3ξ + 0.075 × survivin – 10.631 and Cf 2 = 0.052 × p53 + 0.066× CyclinB1 + 0.066× p16 + 0.092× p62 + 0.043 × 14-3-3ξ+ 0.075 × survivin – 19.947.
LOOCV was employed in both models, and results are shown in . The LR model was chosen for subsequent analysis due to its higher separation ability between BC and NHC with a PPV of 90.0% compared with Fisher linear discriminant analysis model with a PPV of 88.0%. Finally, predicted probabilities of the 184 BC and 184 NHC were computed and used for ROC analysis in order to find the optimal threshold (p = .692). The model had an AUC of 0.943 to discriminate individuals with BC from NHC with a sensitivity of 80.4%, specificity of 95.1% and the accuracy of 87.8% ()). In the differentiation of BC and BBD in the training cohort, the LR model showed the AUC of 0.881, a sensitivity of 59.5%, specificity of 88.5%, and a 74.5% accuracy, at p = .692 cutoff value ()).
Table 3. LOOCV results predicted by the two models of BC
Figure 4. Performance of the prediction model with five anti-TAAs autoantibodies to detect breast cancer. Receiver operating characteristic (ROC) curves from training cohort (a, b) and validation cohort (c, d). Performance shown for distinguishing individuals with BC from NHC (a, c) or BBD controls (b, d). TC, training cohort; VC, validation cohort; BC, breast cancer; NHC, normal human controls; BBD, benign breast disease; Se, sensitivity; Sp, specificity
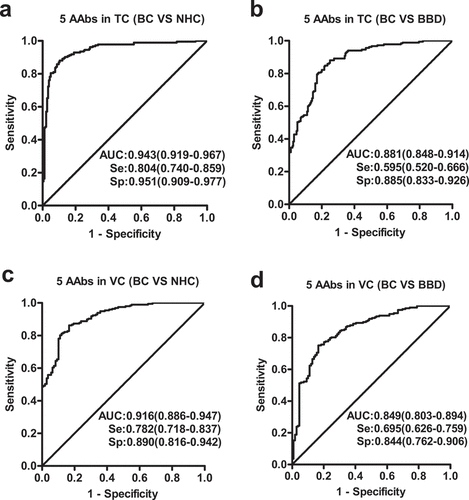
3.4 The external validation of the immunodiagnostic model
Classification performance of the LR model for BC was further assessed in the validation cohort, which is an external validation group. Discrimination between BC and NHC gave an AUC of 0.916 with a sensitivity of 78.2%, a specificity of 89.0%, and a diagnostic accuracy of 82.4% at a cut- off value of p = .692 ()). In addition, the model was able to distinguish BC from BBD with the AUC of 0.849 yielding a sensitivity of 69.5%, a specificity of 84.4%, and a diagnostic accuracy of 74.8% ()).
3.5 The immunodiagnostic model for early stage and under the age of 50 years detection
The patients from both training and validation cohorts were divided into early-stage (stage I, II) and late-stage (stage III, IV) of BC. For the early-stage BC detection in the training cohort, the model showed a high AUC value (0.949) for the comparison between BC and NHC with the sensitivity of 72.1% and specificity of 96.7%. The AUC for discriminating BC from BBD was 0.890 with the sensitivity of 53.2% and specificity of 94.0%. Similar results were observed in the validation cohort. The AUC was 0.912 with the sensitivity of 69.5% and specificity of 89.9% when comparing BC to NHC. The AUC was 0.851 with the sensitivity of 54.4% and specificity of 93.6% when the control group was BBD.
Additionally, the diagnostic value of the model was determined between the patients whose ages were under 50 years and above 50 years. For patients under the age of 50 years, the AUC remained high within the training cohort with a value of 0.963 when comparing BC to NHC. The autoantibodies panel exhibited a sensitivity of 71.3%, a specificity of 96.7%. The AUC was 0.898 with the sensitivity of 51.5% and specificity of 95.0% when BC was compared to BBD. In the validation cohort, the model showed an AUC of 0.927 in BC versus NHC with the sensitivity of 73.8% and specificity of 89.9%. The AUC was 0.865 in BC versus BBD with the sensitivity of 59.8% and specificity of 90.8%. All the results above are shown in , as well as the diagnostic value of the model in late-stage and that in the patients older than 50 years.
Table 4. Diagnostic value of the prediction model with five anti-TAAs autoantibodies for different stages and ages of BC patients
3.6 Positive reactivity of the immunodiagnostic model among subgroups
For the immunodiagnostic model, if the predicted probability was greater than 0.692, a sample could be classified as BC. As shown in ,b), in both training and validation cohorts, the positive response of every single autoantibody in BC group, ranging from 10.2% to 57.6%, was significantly different from that in NHC (ranged from 1.0% to 4.9%) and that in BBD (ranged between 2.0% and 25.7%). Additionally, among the training cohort, 80.4% of 184 BC patients were identified to have positive reactivity to five TAAs of the model compared to 11.5% in BBD and 4.9% in NHC. In the other cohort, 78.2% of 197 BC patients were identified as having positive reactivity to the five TAAs compared to 15.6% in BBD and 11.0% in NHC.
Figure 5. Positive rates of autoantibodies against eight TAAs or combined five TAAs in individual sera of BC patients, BBD patients and NHC (a, b). The cutoff value was set at the maximum Yoden index when the specificity was greater than 95%. Positive rates of the prediction model with five anti-TAAs autoantibodies among subgroups of BC patients based on tumor stage (c-f), molecular subtypes (g), lymph node metastasis (h), histological type (i) and family history of tumor (j). TC, training cohort; VC, validation cohort; BC, breast cancer; NHC, normal human controls; BBD, benign breast disease
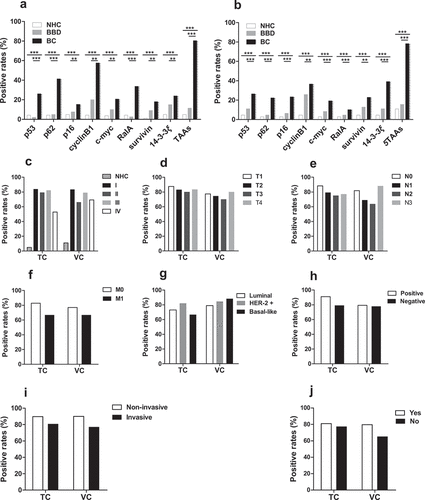
The model showed no significant difference when data were subdivided by clinical stage, prognostic index, lymph node status, histological type or family history of tumor (–). Interestingly, the autoantibodies to p16 were significantly different between stage I and other stages (II, III and IV) in validation cohort (Supplemental Figure 4). In addition, the model also showed no differences between patients of early-stage and late-stage. Nevertheless, the difference of positive reactivity between patients at the ages of under and above 50 years was statistically significant in the training cohort ().
Table 5. Positive reactivity of the prediction model with five anti-TAAs autoantibodies among subgroups
4. Discussion
In this study, Western Blotting was first performed to confirm the ELISA results which were previously obtained. The results from western blotting analysis visually revealed the differences of frequency of autoantibodies between cancer patients and normal controlsCitation14 For all 10 BC patients, there were 3–6 anti-TAAs autoantibodies showing positive reactivity in each patient, and for 10 normal controls, only 1 or 2 sera showed positive reactivity for anti-TAAs autoantibodies. This observation has provided us a clue that multiple autoantibodies detection could achieve higher sensitivity in the diagnosis of BC than a single autoantibody. These findings were further supported by published data that the application of a panel of TAAs could enhance the detection of autoantibodies.Citation29,Citation31,Citation36,Citation37 Moreover, the ELISA results of the 10 BC patients and 10 controls showed similar results to those of Western Blot (Supplemental Table 1–2).
To control systematic errors and selection bias, 11 autoantibodies were tested in two BC groups from different hospitals, and were also tested in two different control groups. Among the 11 anti-TAAs autoantibodies, significantly increased levels of eight anti-TAAs (p53, cyclin B1, p16, p62, c-myc, RalA, survivin, and 14-3-3ξ) autoantibodies were testified between cancer group and NHC group both in training cohort and validation cohort. Many reported data presented the evidence for the existence in breast cancer sera of autoantibodies that used in the present study.Citation19,Citation27,Citation38 Ye et al. examined 42 BC cases and 81 normal controls, and they showed the sensitivities of autoantibodies to cyclin B1 (17.1%), survivin (22.0%), p53 (12.2%)Citation30 Additionally, this study also showed that the serum levels of five autoantibodies to p53, cyclin B1, p16, p62, and 14-3-3ξ were significantly different between cancer group and BBD group. This finding provided the foundation for the following study of a panel of autoantibodies in the identification of BC and BBD.
Even though the significant difference of certain autoantibodies existed between two BC groups and two control groups, the result showed that the sensitivity of single autoantibody in BC was low, which will limit its clinical application.Citation30,Citation31 Therefore, based on the results of western blotting, multiple autoantibodies were examined as a panel to reach the higher sensitivity. In addition, to establish a diagnostic model with classification, multivariate statistical analysis was used, which can improve the diagnostic accuracy and predict the probability of developing BC. It can also increase the specificity of BC detection. This study chose the Fisher linear discriminant analysis model and LR model and compared their predictive effects for finding the ideal classifier in BC prediction.Citation39,Citation40 The LOOCV approach was used to compare the performances of two prediction models and to ensure a statistically independent prediction of the multivariate classification modelCitation41 Finally, it was noticed that the LR model had higher PPV with fewer amounts of autoantibodies (5 TAAs as a panel). The 5 TAAs (p53, cyclinB1, p16, p62, and 14-3-3ξ) panel showed rather high sensitivity and specificity in this study. Liu et al. showed that expression of autoantibodies against the panel (p16, c-myc, p53, and ANXA-1) was significantly higher in the breast cancer group (102 breast cancer patients and 146 age-matched healthy volunteers) with the sensitivity of 33.3% (90% specificity)Citation38 Ye et al. examined 42 BC cases and 81 controls, and they showed the sensitivity of 61.0% and a specificity of 86.6% in BC by the combination of ten TAAs (Imp1,p62, Koc, p53, c-myc, survivin, p16, cyclinB1, cyclinD1, and p16)Citation30 This study takes advantage of large sample size and the 2 cohorts (one for training and one for validation).
In order to make a solid conclusion, the case–control study included two cohorts of patients from different hospitals. Both healthy individuals and benign diseases age-matched with BC patients were selected as controls. Hermann1 et al. presented the evidence that CA15-3 showed significant differences in BBD and BC. The AUC for discriminating between BC and BBD achieved 0.71 with a sensitivity of 33.8% at 95% specificityCitation42 Liu et al. evaluate the immunodiagnostic values of autoantibodies to a panel of 6 TAAs in 49 sera from breast cancer patients, 35 sera from patients with benign breast disease, and 38 sera from normal controls. They indicated the positive reactions of autoantibody reaching a sensitivity of 67.3% and a specificity of 92.2% (Imp1, p16, Koc, survivin, cyclinB1, and c-myc)Citation26 Furthermore, reported data of previous studies set different cutoff values for various markers to distinguish BC from NHC.Citation14,Citation36 However, it will increase the difficulty of using multiple markers in the detection of cancer. For an optimal combination of various autoantibodies, using only one cutoff value to discriminate BC from normal control or distinguish BC from BBD would facilitate the screening of BC. The advantage of this study is the achievement of the aforementioned problem.
Additionally, the present study had provided some relevant exploration findings of potential biomarkers for detection and screening of breast cancer in early-stage BCCitation43 The immunodiagnostic model showed that in early-stage (stage I and II) AUC had more accuracy than that in the late stage (stage III and IV) in the diagnosis of BC no matter what the control group was (NHC or BBD). In addition, this study also revealed that the positive reactivity of the five anti-TAAs autoantibodies was significantly different between patients at the age under and above 50 years in the training cohort. This finding suggests that the immunodiagnostic model is more capable of predicting BC among young women. Peer et al. screened 23000 women and followed up. The data from them showed that for patients under age 50, the sensitivity was 64% for cancers within one year after screening. The sensitivity was lower than those of the 50–69 and >70 age groups, with 85% and 80% sensitivity, respectivelyCitation.44 High density of breast tissue impairs the sensitivity of mammography, especially in women between 40 and 49 years of age (about 74% of them with high breast density) and 45% of women in their 60s have high breast densityCitation6 Therefore, the immunodiagnostic model could make up the low sensitivity of mammography in young women, especially at high breast density.Citation2,Citation4,Citation6 Furthermore, the results of this study demonstrated that at stage I of BC, the level of anti-p16 autoantibody was significantly higher than that in stage II, stage III and stage IV. The anti-p16 autoantibody was firstly reported by our group in 2006Citation45 The p16 protein is a cyclin-dependent kinase inhibitor, and also a negative regulator of the mammalian cell cycleCitation46 Despite it is not specific for BC, the finding indicates that the anti-p16 antibody could be a biomarker in the early detection of BC.Citation45 However, this is a retrospective case–control study, the pre-diagnostic sera of BC were unavailable. Therefore, there is a need to confirm autoantibody signatures and its diagnostic values on a large prospective studyCitation2 In addition, more works are needed to further confirm the clinical utility of the five anti-TAAs autoantibodies.
In summary, this study suggests that the immunodiagnostic prediction model with the combination of five anti-TAAs autoantibodies could identify BC from NHC as well as BBD. It has a potentially important role in future prediction and diagnosis of BC as effective biomarkers for early diagnosis are lacking. The data from this study also reveal that as a supplement approach of mammography, the model could improve the power of immunodiagnosis in breast cancer. Recently, we noticed some other approaches to the detection of autoantibodies to TAAs, such as whole genome derived peptide arrays and protein microarray technology.Citation47–Citation50 The future directions would detect autoantibody signatures using those approaches, and we anticipate obtaining a panel of new biomarkers.
Disclosure of potential conflicts of interest
No potential conflicts of interest were disclosed.
Supplemental Material
Download ()Supplementary material
Supplemental data for this article can be accessed on the publisher’s website.
Additional information
Funding
References
- Bray F, Ferlay J, Soerjomataram I, Siegel RL, Torre LA, Jemal A. Global cancer statistics 2018: GLOBOCAN estimates of incidence and mortality worldwide for 36 cancers in 185 countries. CA Cancer J Clin. 2018;68(6):394–11. PMID:30207593. doi:10.3322/caac.21492.
- Lacombe J, Mange A, Solassol J. Use of autoantibodies to detect the onset of breast cancer. J Immunol Res . 2014;2014:574981. PMID:25143958. doi:10.1155/2014/574981.
- Bleyer A, Welch HG. Effect of three decades of screening mammography on breast-cancer incidence. N Engl J Med. 2012;367(21):1998–2005. PMID:23171096. doi:10.1056/NEJMoa1206809.
- Fernández-Madrid F, Maroun M-C, Autoantibodies in breast cancer. Adv Clin Chem. 2014;64:221–240. doi: 10.1016/b978-0-12-800263-6.00005-7.
- Qaseem A, J S L, Mustafa RA, Horwitch CA, Wilt TJ. Screening for breast cancer in average-risk women: a guidance statement from the American College of Physicians. Ann Intern Med. 2019. PMID:30959525. doi:10.7326/M18-2147
- Saadatmand S, Geuzinge HA, Rutgers EJT, Mann RM, de Roy van Zuidewijn DBW, Zonderland HM, Tollenaar RAEM, Lobbes MBI, Ausems MGEM, van ‘t Riet M, et al. MRI versus mammography for breast cancer screening in women with familial risk (FaMRIsc): a multicentre, randomised, controlled trial. Lancet Oncol. 2019;20:1136–1147. doi:10.1016/s1470-2045(19)30275-x.
- Jørgensen KJ, Gøtzsche PC, Kalager M, Zahl PH. Breast cancer: tumor Size and overdiagnosis. Ann Intern Med. 2017;166(5). PMID:28114656. doi:10.7326/p16-9029.
- Chapman C, Murray A, Chakrabarti J, Thorpe A, Woolston C, Sahin U, Barnes A, Robertson J. Autoantibodies in breast cancer: their use as an aid to early diagnosis. Ann Oncol. 2007;18(5):868–873. PMID:17347129. doi:10.1093/annonc/mdm007.
- Sidransky D. Emerging molecular markers of cancer. Nat Rev Cancer. 2002;2(3):210–219. PMID:11990857. doi:10.1038/nrc755.
- Chen YT, Gure AO, Scanlan MJ. Serological analysis of expression cDNA libraries (SEREX): an immunoscreening technique for identifying immunogenic tumor antigens. Methods Mol Med. 2005; 207–216. PMID:15542909.
- Zhu Q, Liu M, Dai L, Ying X, Ye H, Zhou Y, Han S, Zhang J-Y. Using immunoproteomics to identify tumor-associated antigens (TAAs) as biomarkers in cancer immunodiagnosis. Autoimmun Rev. 2013;12(12):1123–1128. PMID:23806562. doi:10.1016/j.autrev.2013.06.015.
- Liu W, Peng B, Lu Y, Xu W, Qian W, Zhang J-Y. Autoantibodies to tumor-associated antigens as biomarkers in cancer immunodiagnosis. Autoimmun Rev. 2011;10(6):331–335. PMID:21167321. doi:10.1016/j.autrev.2010.12.002.
- Bassaro L, Russell SJ, Pastwa E, Somiari SA, Somiari RI. Screening for multiple autoantibodies in plasma of patients with breast cancer. Cancer Genomics Proteomics. 2017;14(6):427–435. PMID:29109092.
- Li P, Shi J-X, Dai L-P, Chai Y-R, Zhang H-F, Kankonde M, Kankonde P, Yu B-F, Zhang J-Y. Serum anti-MDM2 and anti-c-Myc autoantibodies as biomarkers in the early detection of lung cancer. Oncoimmunology. 2016;5(5):e1138200. PMID:27467958. doi:10.1080/2162402x.2016.1138200.
- Tan EM, Zhang J. Autoantibodies to tumor-associated antigens: reporters from the immune system. Immunol Rev . 2008;222:328–340. PMID:18364012. doi:10.1111/j.1600-065X.2008.00611.x.
- Tan HT, Low J, Lim SG, Chung MC. Serum autoantibodies as biomarkers for early cancer detection. Febs J. 2009;276(23):6880–6904. PMID:19860826. doi:10.1111/j.1742-4658.2009.07396.x.
- Loke SY, Lee ASG. The future of blood-based biomarkers for the early detection of breast cancer. Eur J Cancer . 2018;92:54–68. PMID:29413690. doi:10.1016/j.ejca.2017.12.025.
- Crawford LV, Pim DC, Bulbrook RD. Detection of antibodies against the cellular protein p53 in sera from patients with breast cancer. Int J Cancer. 1982;30(4):403–408. PMID:6292117. doi:10.1002/(ISSN)1097-0215.
- Soussi T. p53 Antibodies in the sera of patients with various types of cancer: a review. Cancer Res. 2000;60(7):1777–1788. PMID:10766157.
- Gion M, Mione R, Leon AE, Dittadi R. Comparison of the diagnostic accuracy of CA27.29 and CA15.3 in primary breast cancer. Clin Chem. 1999;45(5):630–637. PMID:10222349.
- Klee GG, Schreiber WE. MUC1 gene-derived glycoprotein assays for monitoring breast cancer (CA 15-3, CA 27.29, BR): are they measuring the same antigen? Arch Pathol Lab Med. 2004;128(10):1131–1135. PMID:15387710. doi:10.1043/1543-2165(2004)128<1131:mggafm>2.0.co;2.
- Burford B, Gentry-Maharaj A, Graham R, Allen D, Pedersen JW, Nudelman AS, Blixt O, Fourkala EO, Bueti D, Dawnay A, et al. Autoantibodies to MUC1 glycopeptides cannot be used as a screening assay for early detection of breast, ovarian, lung or pancreatic cancer. Br J Cancer. 2013;108(10):2045–2055. PMID:23652307. doi:10.1038/bjc.2013.214.
- Suzuki H, Graziano DF, McKolanis J, Finn OJ. T cell-dependent antibody responses against aberrantly expressed cyclin B1 protein in patients with cancer and premalignant disease. Clin Cancer Res. 2005;11(4):1521–1526. PMID:15746055. doi:10.1158/1078-0432.ccr-04-0538.
- Xiu Y, Sun B, Jiang Y, Wang A, Liu L, Liu Y, Sun S, Huangfu Ml. Diagnostic value of the survivin autoantibody in four types of malignancies. Genet Test Mol Biomarkers. 2018;22(6):384–389. PMID:29924656. doi:10.1089/gtmb.2017.0278.
- Cao J, Mu Q, Huang H. The roles of insulin-like growth Factor 2 mRNA-binding protein 2 in cancer and cancer stem cells. Stem Cells Int . 2018;2018:4217259. PMID:29736175. doi:10.1155/2018/4217259.
- Liu W, De La Torre IG, Gutierrez-Rivera MC, Wang B, Liu Y, Dai L, Qian W, Zhang J-Y. Detection of autoantibodies to multiple tumor-associated antigens (TAAs) in the immunodiagnosis of breast cancer. Tumour Biol. 2015;36(2):1307–1312. PMID:25355596. doi:10.1007/s13277-014-2756-5.
- Lu H, Ladd J, Feng Z, Wu M, Goodell V, Pitteri SJ, Li CI, Prentice R, Hanash SM, Disis ML, et al. Evaluation of known oncoantibodies, HER2, p53, and cyclin B1, in prediagnostic breast cancer sera. Cancer Prev Res (Phila). 2012;5(8):1036–1043. PMID:22715141. doi:10.1158/1940-6207.capr-11-0558.
- Qin J, Wang S, Wang P, Wang X, Ye H, Song C, Dai L, Wang K, Jiang B, Zhang J, et al. Autoantibody against 14-3-3 zeta: a serological marker in detection of gastric cancer. J Cancer Res Clin Oncol. 2019;145(5):1253–1262. PMID:30887154. doi:10.1007/s00432-019-02884-5.
- Wang S, Qin J, Ye H, Wang K, Shi J, Ma Y, Duan Y, Song C, Wang X, Dai L, et al. Using a panel of multiple tumor-associated antigens to enhance autoantibody detection for immunodiagnosis of gastric cancer. Oncoimmunology. 2018;7(8):e1452582. PMID:30221047. doi:10.1080/2162402X.2018.1452582.
- Ye H, Sun C, Ren P, Dai L, Peng B, Wang K, Qian W, Zhang J. Mini-array of multiple tumor-associated antigens (TAAs) in the immunodiagnosis of breast cancer. Oncol Lett. 2013;5(2):663–668. PMID:23420714. doi:10.3892/ol.2012.1062.
- Wang P, Qin J, Ye H, Li L, Wang X, Zhang J. Using a panel of multiple tumor-associated antigens to enhance the autoantibody detection in the immunodiagnosis of ovarian cancer. J Cell Biochem. 2019;120(3):3091–3100. PMID:30484895. doi:10.1002/jcb.27497.
- Dai L, Ren P, Liu M, Imai H, Tan EM, Zhang J-Y. Using immunomic approach to enhance tumor-associated autoantibody detection in diagnosis of hepatocellular carcinoma. Clin Immunol. 2014;152(1–2):127–139. PMID:24667685. doi:10.1016/j.clim.2014.03.007.
- Koziol JA, Imai H, Dai L, Zhang J-Y, Tan EM. Early detection of hepatocellular carcinoma using autoantibody profiles from a panel of tumor-associated antigens. Cancer Immunol Immunother. 2018;67(5):835–841. PMID:29497780. doi:10.1007/s00262-018-2135-y.
- Qin J, Wang S, Shi J, Ma Y, Wang K, Ye H, Zhang X, Wang P, Wang X, Song C, et al. Using recursive partitioning approach to select tumor-associated antigens in immunodiagnosis of gastric adenocarcinoma. Cancer Sci. 2019. PMID:30950146. doi:10.1111/cas.14013
- Zhang Y, Ying X, Han S, Wang J, Zhou X, Bai E, Zhang J,Zhu Q. Autoantibodies against insulin-like growth factor-binding protein-2 as a serological biomarker in the diagnosis of lung cancer. Int J Oncol. 2013;42(1):93–100. PMID:23165420. doi:10.3892/ijo.2012.1699.
- Xu Y-W, Peng Y-H, Chen B, Wu Z-Y, Wu J-Y, Shen J-H, Zheng C-P, Wang S-H, Guo H-P, Li E-M, et al. Autoantibodies as potential biomarkers for the early detection of esophageal squamous cell carcinoma. Am J Gastroenterol. 2014;109(1):36–45. PMID:24296751. doi:10.1038/ajg.2013.384.
- Zhang JY, Casiano CA, Peng XX, Koziol JA, Chan EK,Tan EM, et al. Enhancement of antibody detection in cancer using panel of recombinant tumor-associated antigens. Cancer Epidemiol Biomarkers Prev. 2003;12(2):136–143. PMID:12582023.
- Liu Y, Liao Y, Xiang L, Jiang K, Li S, Huangfu M, Sun S. A panel of autoantibodies as potential early diagnostic serum biomarkers in patients with breast cancer. Int J Clin Oncol. 2017;22(2):291–296. PMID:27778118. doi:10.1007/s10147-016-1047-0.
- Tsentidis C, Bampilis A, Ntova V, Fragkos D, Liu Y, Liao Y, Xiang L, Jiang K, Li S, Huangfu M, et al. Metabolic syndrome as a predictor of adrenal functional status: a discriminant multivariate analysis versus logistic regression analysis. Horm Metab Res. 2019;51(1):47–53. PMID:30406628. doi:10.1055/a-0754-6464.
- Zou M, Sun C, Liang S, Sun Y, Li D, Li L, Fan L, Wu L, Xia W. Fisher discriminant analysis for classification of autism spectrum disorders based on folate-related metabolism markers. J Nutr Biochem. 2019;64:25–31. PMID:30419423. doi:10.1016/j.jnutbio.2018.09.023.
- Goncalves-Ribeiro S, Sanz-Pamplona R, Vidal A, Sanjuan X, Guillen Díaz-Maroto N, Soriano A, Guardiola J, Albert N, Martínez-Villacampa M, López I, et al. Prediction of pathological response to neoadjuvant treatment in rectal cancer with a two-protein immunohistochemical score derived from stromal gene-profiling. Ann Oncol. 2017;28(9):2160–2168. PMID:28911071. doi:10.1093/annonc/mdx293.
- Hermann N, Dressen K, Schroeder L, Debald M, Schildberg FA, Walgenbach-Bruenagel G, Hettwer K, Uhlig S, Kuhn W, Hartmann G, et al. Diagnostic relevance of a novel multiplex immunoassay panel in breast cancer. Tumour Biol. 2017;39(6):1010428317711381. PMID:28618926. doi:10.1177/1010428317711381.
- Bayo J, Castano MA, Rivera F, Navarro F. Analysis of blood markers for early breast cancer diagnosis. Clin Transl Oncol. 2018;20(4):467–475. PMID:28808872. doi:10.1007/s12094-017-1731-1.
- Peer PG, Verbeek AL, Straatman H, Hendriks JH, Holland R. Age-specific sensitivities of mammographic screening for breast cancer. Breast Cancer Res Treat. 1996;38(2):153–160. PMID:8861833. doi:10.1007/BF01806669.
- Looi K, Megliorino R, Shi F-D, Peng -X-X, Chen Y, Zhang J-Y. Humoral immune response to p16, a cyclin-dependent kinase inhibitor in human malignancies. Oncol Rep. 2006;16(5):1105–1110. PMID:17016600.
- Goel S, DeCristo MJ, Watt AC, BrinJones H, Sceneay J, Li BB, Khan N, Ubellacker JM, Xie S, Metzger-Filho O, et al. CDK4/6 inhibition triggers anti-tumour immunity. Nature. 2017;548(7668):471–475. PMID:28813415. doi:10.1038/nature23465.
- Yan Y, Sun N, Wang H, Kobayashi M, Ladd JJ, Long JP, Lo KC, Patel J, Sullivan E, Albert T, et al. Whole genome-derived tiled peptide arrays detect prediagnostic autoantibody signatures in non-small-cell lung cancer. Cancer Res. 2019;79(7):1549–1557. PMID:30723114. doi:10.1158/0008-5472.CAN-18-1536.
- Yang L, Wang J, Li J, Zhang H, Guo S, Yan M, Zhu Z, Lan B, Ding Y, Xu M, et al. Identification of serum biomarkers for gastric cancer diagnosis using a human proteome microarray. Mol Cell Proteomics. 2016;15(2):614–623. PMID:26598640. doi:10.1074/mcp.M115.051250.
- Toes RE, Hitchon CA, Pan J, Song G, Chen D, Li Y, Liu S, Hu S, Rosa C, Eichinger D, et al. Identification of serological biomarkers for early diagnosis of lung cancer using a protein array-based approach. Adv Rheumatol. 2017;16(12):2069–2078. PMID:29021294. doi:10.1002/art.3966410.1074/mcp.RA117.000212.
- Rho J-H, Ladd JJ, Li CI, Potter JD, Zhang Y, Shelley D, Shibata D, Coppola D, Yamada H, Toyoda H, et al. Protein and glycomic plasma markers for early detection of adenoma and colon cancer. Gut. 2018;67(3):473–484. PMID:27821646. doi:10.1136/gutjnl-2016-312794.