ABSTRACT
Tumors acquire numerous mutations during development and progression. When translated into proteins, these mutations give rise to neoantigens that can be recognized by T cells and generate antibodies, representing an exciting direction of cancer immunotherapy. While neoantigens have been reported in many cancer types, the profiling of neoantigens often focused on the class-I subtype that are presented to CD8 + T cells, and the relationship between neoantigen load and clinical outcomes was often inconsistent among cancer types. In this study, we described an informatics workflow, REAL-neo, for identification, quality control (QC), and prioritization of both class-I and class-II human leukocyte antigen (HLA) bound neoantigens that arise from somatic single nucleotide mutations (SNM), small insertions and deletions (INDEL), and gene fusions. We applied REAL-neo to 835 primary breast tumors in the Cancer Genome Atlas (TCGA) and performed comprehensive profiling and characterization of the detected neoantigens. We found recurrent HLA class-I and class-II restricted neoantigens across breast cancer cases, and uncovered associations between neoantigen load and clinical traits. Both class-I and class-II neoantigen loads from SNM and INDEL were found to predict overall survival independent of tumor mutational burden (TMB), breast cancer subtypes, tumor-infiltrating lymphocyte (TIL) levels, tumor stage, and age at diagnosis. Our study highlighted the importance of accurate and comprehensive neoantigen profiling and QC, and is the first to report the predictive value of neoantigen load for overall survival in breast cancer.
Introduction
Immune responses play a critical role in carcinogenesis and harnessing the immune system is a promising approach for cancer prevention and treatment. Cancers arise from somatic alterations, which can result in the production of proteins with altered amino acid sequences, termed tumor-specific antigens (TSAs) or neoantigens, that the immune system recognizes as foreign and may evoke immune responses.Citation1–Citation3 Specifically, neoantigens can potentially be presented by both class-I and class-II human leukocyte antigen (HLA, the major histocompatibility complex [MHC] in human) and induce protective sustained cytotoxic T-lymphocyte responses that destroy cancer cells, while sparing normal tissue.Citation4,Citation5 Higher tumor mutational burden (TMB) has been linked to better overall survival (OS) after immune checkpoint blockade therapies in multiple cancer types, Citation6 prompting the hypothesis that higher TMB is associated with higher neoantigen load and more effective antitumor immune responses.Citation7,Citation8 Higher TMB has been linked to improved survival for bladder, colorectal, head and neck, and lung cancer after adjustment for other clinical covariates, but interestingly not for breast and several other cancers.Citation6
In this study, we bypassed the surrogate of TMB and investigated the relationship between predicted neoantigen load and OS in breast cancer (BRCA). First, we developed an improved bioinformatics workflow for neoantigen prediction and quality control (QC). Most prior analyses focused on neoantigen load predicted to result from somatic nonsynonymous single nucleotide mutations (SNM) and small frame-shift insertions and deletions (INDEL) without considering large genomic rearrangements or gene fusions. Oftentimes only immunogenic peptides presented to CD8 + T cells on restricted HLA-I subtypes were included, leaving out those binding to HLA-II subtypes on CD4 + T cells. In addition, effects of frame-shift INDEL and gene fusion, and to a lesser extent nonsynonymous SNM, on protein sequences are highly dependent on which transcriptional isoforms are expressed; accordingly, our method includes prioritization algorithms to predict which neoantigens are most likely expressed. Furthermore, the predicted neoantigens should be screened to eliminate mutant peptides that are part of naturally occurring wild-type proteins. Our recently developed REAL-neo pipeline was designed to optimally address these limitations often found in other neoantigen prediction approaches. To demonstrate the potential of the REAL-neo algorithm, we applied this method to 835 primary BRCAs in the Cancer Genome Atlas (TCGA) and performed comprehensive profiling and characterization of the predicted neoantigens. We observed that the neoantigen loads varied greatly between patients, and were significantly different between BRCA molecular subtypes and immune subtypes described in the recent TCGA publication.Citation9 In peri- and post-menopausal women, the neoantigen loads were significantly different between race groups stratified by BRCA subtypes. Gene fusion, an often ignored genomic mutation type regarding neoantigen discovery, contributed to more than 1/3 of total neoantigen load. Lower HLA class-I and class-II restricted neoantigen loads were found to be associated with worse OS independent of other clinical variables including TMB, BRCA subtype, level of tumor-infiltrating lymphocytes (TILs), tumor clinical stage, and age at diagnosis.
Materials and methods
Sample description
We downloaded the exome and RNA sequencing BAM files of 1,099 patients with BRCA from TCGA, among which 835 cases had information about patient race, BRCA subtype, immune subtype, Citation9 tumor stage and other clinical variables, and were included in the current study. The additional sample annotations, including TILs and age at diagnosis, were obtained from the same TCGA publication.Citation9 All patients described in this paper have given written consent to the inclusion of material pertaining to them as part of the TCGA project. Local Institutional Review Boards (IRBs) at the tissue source sites reviewed protocols to approve submission of cases. This study is in full compliance with all relevant codes of experimentation and legislation, and follows the principles of the Declaration of Helsinki.
HLA genotyping
We selected OptiType version 1.3.1Citation10 and HLA-HD version 1.2.0Citation11 for class-I and class-II HLA genotyping, respectively. The tumor exome sequencing BAM files were converted to FASTQ format using an in-house-developed script for HLA genotyping.
Somatic mutation profiling and prediction of putative neoepitopes
Somatic SNM and INDEL from patients with BRCA were downloaded from TCGA GDC (https://portal.gdc.cancer.gov/). Gene fusions were obtained from The Jackson Laboratory’s Tumor Fusion Gene Data PortalCitation12 (https://www.tumorfusions.org/). Because the TCGA annotations of the SNM and INDEL were based on a randomly selected transcriptional splicing isoform of the gene, we re-annotated all mutations as following: (1) the chromosomal position of each mutation was mapped to all transcriptional isoforms annotated by Ensembl reference genome GRCh38.p13 using gene/exon/transcript definitions described in Ensembl Genes 88; (2) the transcriptional isoform expressions in each sample were quantified using SalmonCitation13 and the expressed isoforms were determined using a threshold (Log2TPM ≥ −5) defined by the bi-modal distribution (Supplement Figure S1); (3) the expressed transcriptional isoforms harboring mutations were then translated into proteins to obtain 8–11 amino acid (aa) long neoepitopes (mutant peptides) for class-I HLA binding prediction, and 15-aa neoepitopes for class-II HLA binding prediction; (4) for gene fusions, the exonic regions of the driver gene before breakpoint and the recipient gene after breakpoint were fused and translated based on the transcription direction of the driver gene. The fusion transcripts from expressed isoforms of the driver and recipient genes harboring the breakpoints were translated to obtain neoepitopes; and (5) all neoepitopes were further screened against wild-type protein sequences to filter out those that are part of naturally occurring wild-type peptides in a different protein. For example, a neoepitope could be a wild-type peptide from another member of the same protein family due to sequencing homology.
Binding affinity prediction and neoepitope selection
For each BRCA sample, NetMHC v4.0Citation14 was used to predict the binding affinities between the patient-specific 8–11 aa neoepitopes and class-I HLA genotyped by OptiType, and NetMHCII v2.3Citation15 was used to predict the bindings between 15-aa neoepitopes and class-II HLA genotyped by HLA-HD.
Nonsynonymous germline and somatic mutations in BRCA1 and BRCA2 genes
The germline variants were not readily available from TCGA. The tumor-paired normal tissue or peripheral blood exome sequencing data were used to identify germline nonsynonymous mutations in BRCA1 and BRCA2 genes using a Mayo in-house exome analytic pipeline.Citation16 Briefly, the FASTQ files were aligned to human reference genome GRCh38 using BWA-MEM.Citation17 Single nucleotide variants (SNV) and small INDELs were identified and prioritized following the best practice guideline by Broad (https://software.broadinstitute.org/gatk/best-practices/workflow?id=11145) using the Genome Analysis Toolkit (GATK).Citation18 The variants that passed QC were annotated using BioR to identify nonsynonymous variants in BRCA1 and BRCA2 genes.Citation19 The somatic nonsynonymous mutations in BRCA1 and BRCA2 genes were part of the downloaded TCGA mutation data.
Statistics
All statistics were performed in R. Pearson correlation coefficients and p values were calculated between neoantigen load and mutation burden. The comparisons of neoantigen load between groups with different clinical traits were performed using student’s t-test assuming unequal variance. The survival analyses were performed using the Cox proportional hazards models while correcting for covariates.
Results
The REAL-neo pipeline
The REAL-neo pipeline is described in . The pipeline starts by detecting tumor somatic mutations from patient samples, including SNM, INDELs, and expressed fusion genes. These mutations are then re-annotated and mapped to all human transcriptional isoforms based on the gene/exon/transcript definitions from Ensembl Genes 88, allowing the users to examine the impacts of mutations on all transcripts rather than focusing on the canonical or longest transcript. In the next step, the mutated nucleotide sequences are translated into peptide sequences. The isoform expressions in each sample are quantified and the expressed isoforms are selected based on the bi-modal distribution of the expression values. At this point, only mutated peptides on expressed isoforms are kept as putatively expressed mutant peptides. These peptides are then compared to our database of wild-type peptides from the human proteome to rule out mutant peptides that are part of the naturally occurring wild-type proteins. In parallel, HLA genotyping of both class-I and class-II HLA alleles are performed for each patient. We used OptiType for class-I and HLA-HD for class-II genotyping for the BRCA cohort based on the comparison of performance of multiple tools (Supplement Figure S2, manuscript in preparation); however, the users have the option to choose other genotypers.
Once the HLA genotypes are determined, the workflow proceeds to predict the binding affinity between the mutant peptides and the patient-specific HLA alleles. By default, peptide-HLA pairs with binding affinity <500 nM are kept as putative MHC-binding neoepitopes. In the meanwhile, the pipeline has an optional function to predict binding affinity between the HLA alleles and all wild-type peptides from the human proteome, which serves as a reference for MHC-binding affinities. Instead of using the pre-defined binding affinity of 500 nM, the users can choose to compare the binding affinity between mutant peptide and its native wild-type protein, and keep mutant peptides that have higher binding affinity than its native wild-type sequence. From either option, the users will end up with a list of predicted neoantigens. Finally, the pipeline will examine the read depth of the mutant allele in tumor RNA-Seq data to evaluate whether the mutant alleles of the predicted neoantigens are expressed. This step allows users to further refine the neoantigen list to identify likely vaccine candidates.
In summary, our REAL-neo pipeline integrates all steps of neoantigen identification. It incorporates both DNA and RNA level data, applies several layers of filtering to control for false positives, and delivers a highly processed and immunologically relevant list of predicted neoantigens that arise from both simple and complex tumor mutational events and bind to both class-I and class-II restricted HLA alleles. It also allows the users to choose their own bioinformatics tools and filtering strategies, making it highly individualizable.
HLA genotypes and neoantigen load in the TCGA BRCA patients
A total of 67 unique class-I and 24 unique class-II HLA subtypes () were identified among 835 BRCA patients. Class-I HLAs were detected in all but one case, and each case had up to six different HLA-I subtypes. HLA-II subtypes were not identified in 149 (17.84%) out of 835 BRCAs and the remaining cases had up to 6 class-II HLA subtypes (). Among the three somatic mutation types, SNM, INDEL, and gene fusion, that resulted in neoantigens, SNMs contributed to only 6.25% of the total neoantigens (number of class-I neoantigens vs. number of class-II neoantigens = 1:3.5); INDELs accounted for 57.17% of the total (class-I: class-II = 1:2), and gene fusions accounted for 36.58% of the total (class-I: class-II = 1:2.2) (). The number of neoantigens per patient varied widely. For HLA class-I restricted neoantigens it ranged from 0 to 1953 contributed by SNMs, 0–17,743 by INDELs, and 0–7255 by gene fusion; and 0–3728 contributed by SNMs, 0–45,883 by INDELs, and 0–20,383 by gene fusion for HLA class-II restricted neoantigens (). Compared to previous studies, Citation9,Citation20 we identified significantly higher numbers of predicted neoantigens for several reasons: (1) unique neoepitopes from all expressed transcriptional isoforms were considered instead of one randomly selected isoform; (2) the threshold for determining expressed isoforms was based on the bi-modal distribution (Supplement Figure S1, Log2TPM ≥ −5) instead of an arbitrarily high threshold ;Citation9 (3) fusion genes were included in deriving putative neoepitopes which contributed to more than one-third of the total neoantigen load; and (4) both class-I and class-II neoantigens were predicted.
Figure 2. HLA genotyping and neoantigen profiling in 835 TCGA BRCA tumors. (a) The number of unique HLA genotypes in the 835 TCGA BRCA patients. (b) Distribution of the number of class-I and class-II HLA genotypes per patient. (c) Total number of neoantigens in the BRCA cohort, stratified by: (i) type of somatic mutations: SNM, INDEL, and gene fusion from which neoantigens arose; and (ii) the class of HLA molecules neoantigens bind to. (d) The numbers of class-I (top panel) and class-II (bottom panel) neoantigens per patient, stratified by somatic mutation types of SNM, INDEL and Fusion. The x-axis is in Log2 scale
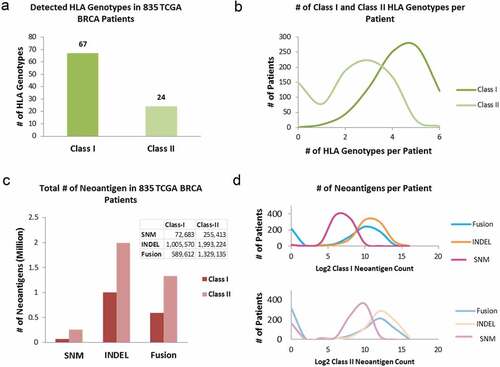
Correlation between tumor mutational burden and neoantigen load
Despite the significantly higher neoantigen load per sample reported here, TMB and total neoantigen load were positively correlated (r = 0.42, p < 2.2E-16) (). In addition, TMB also correlated with each sub-categories of neoantigen load (class I: SNM: r = 0.59, p < 2.2E-16; INDEL: r = 0.28, p < 2.2E-16; gene fusion: r = 0.26, p = 2.01E-11; class II: SNM: r = 0.47, p < 2.2E-16; INDEL: r = 0.16, p = 1.7E-05; gene fusion: r = 0.31, p = 4.37E-13) (–c). The distribution of the binding affinities of neoantigens resulted from SNM, INDEL and gene fusion were similar for both class-I () and class-II HLA binders (), peaking at IC50 of 20 nM.
Figure 3. (a) The correlation between log2-transformed tumor mutation burden and neoantigen load per case in 835 TCGA BRCA tumors. Each dot represents a sample. The oval circle represents normal confidence ellipses. The Pearson correlation coefficient and the corresponding p-value were used to measure the strength of a linear association between TMB and neoantigen load. (b) The correlations between log2-transformed TMB and class-I neoantigen load, stratified by type of somatic mutations: SNM (pink), INDEL (orange) and fusion (blue). (c) The correlations between log2-transformed TMB and class-II neoantigen load, stratified by SNM (purple), INDEL (brown) and fusion (green). (d) The distribution of binding affinities between neoantigens and class-I HLA measured by IC50 (nM), stratified by SNM (pink), INDEL (orange) and fusion (blue). Smaller IC50 values indicate stronger bindings between neoantigens and HLA. (e) The distribution of binding affinities between neoantigens and class-II HLA (SNM in purple, INDEL in brown, and fusion in green)
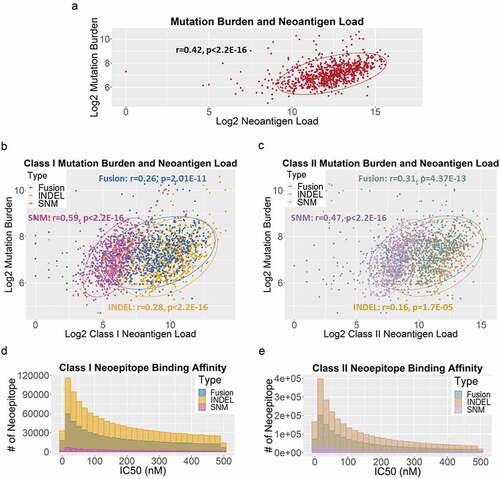
Neoantigen recurrence across breast cancer cases
Similar to previous reports, Citation1 the vast majority (99.75%) of the predicted neoantigens occurred in ≤1% of the cases and 83.76% were patient-specific found in one patient only. One thousand four hundred and eighty-four class-I neoantigens from 94 genes and 8583 class-II neoantigens from 146 genes were shared by 10–17 (1-2%) patients; 180 class-I neoantigens from 12 genes, and 1784 class-II neoantigens from 20 genes were shared by 18–42 (2-5%) patients; and 1 class-I neoantigen from gene DIXDC1 (DIX Domain Containing 1), and 17 class-II neoantigens from DIXDC1 and PIK3CA (Phosphatidylinositol-4,5-Bisphosphate 3-Kinase) were shared by >42 (5%) patients (). The overwhelmingly large number of class-II recurrent neoantigens suggests that the influence of HLA-restricted CD4+ responses could be well underlying the tumor immunogenicity mechanisms and should not be neglected.
Figure 4. Neoantigen recurrence in the TCGA BRCA patients. (a) The occurrences of class-I (brown box) and class-II (green box) neoepitopes in 1, 2–5 (<0.6%), 6–9 (0.6%-1%), 10–17 (1%-2%), 18–42 (2%-5%), and >42 (>5%) patients. For neoepitopes that occurred in >1% cohort, the numbers of recurrent neoepitopes were followed by the numbers of genes they affected. (b) The peptide sequences, number of recurrence among patients, gene names and mutation types of the 18 neoepitopes that occurred in >42 patients
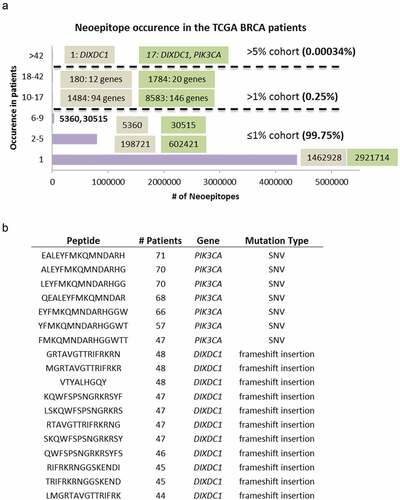
DIXDC1 is a positive regulator of the Wnt signaling pathway and is associated with gamma tubulin at the centrosome. PIK3CA is a well-known cancer driver gene and is the most recurrently mutated gene in multiple cancer types including breast cancer. Seven neoantigens from PIK3CA occurred in 47–71 (5.63–8.5%) patients (). This prompted us to study all neoantigens predicted from cancer driver genes in breast cancer defined as q < 0.1 by MutSigCVCitation21 downloaded from cBioPortal (https://www.cbioportal.org/). We calculated the number of mutations and neoantigens in these 37 driver genes from our cohort, as well as the numbers of patients affected by neoepitopes of these genes (). Interestingly, 7 genes had many neoantigens that occurred in >1% patients, including GATA3 (58 neoantigens, 8 class-I and 50 class-II), TBX3 (61 neoantigens, 0 class-I and 61 class-II), GPRIN2 (110 neoantigens, 1 class-I and 109 class-II), TP53 (252 neoantigens, 46 class-I and 206 class-II), MAP3K1 (746 neoantigens, 167 class-I and 579 class-II), and CDH1 (764 neoantigens, 146 class-I and 618 class-II). PIK3CA also had 37 neoantigens (6 class-I and 31 class-II) that occurred in >1% patients.
Table 1. Neoantigens in BRCA driver genes
Neoantigen load and BRCA1/BRCA2 mutation status
BRCA1 and BRCA2 are the two most important breast cancer susceptibility genes mutated in 21–40% of all inherited BRCA.Citation22–Citation24 BRCA1 and BRCA2 deficiency has been associated with higher TMB.Citation25 We evaluated 835 BRCAs for presence of any of 115 known deleterious BRCA1/BRCA2 germline mutationsCitation26 and identified individuals with BRCA1/BRCA2 somatic mutations from TCGA data. As shown in , cases with deleterious germline BRCA1/BRCA2 variants, compared to those with wild-type BRCA1/BRCA2, had suggestively higher TMB (, left panel, p = .067) but neoantigen loads were not significantly different (, left panel). The cases with germline and somatic BRCA1/BRCA2 mutations had both significantly higher TMB (, right panel, p = 2.76E-06) and neoantigen loads than BRCAs with wild-type genes (, right panel, p = .009).
Figure 5. Neoantigen load and BRCA1/2 mutation status, BRCA subtypes and immune subtypes. (a) BRCA1 and BRCA2 mutation status and overall mutation burden. Left panel: patients with known deleterious BRCA1 or BRCA2 germline mutations (labeled as Mutated, dark purple) had suggestive higher overall mutation burden than patients without (labeled as WT, light purple) (p = .067). Right panel: patients with known deleterious BRCA1 or BRCA2 germline and somatic mutations (labeled as Mutated, dark blue) had significantly higher overall mutation burden than patients without (labeled as WT, light blue) (p = 2.67E-06). (b) BRCA1 and BRCA2 mutation status and neoantigen load. Left panel: patients with known deleterious BRCA1 or BRCA2 germline mutations (labeled as Mutated, dark yellow) did not show significant difference in neoantigen load from patients without (labeled as WT, light yellow). Right panel: patients with known deleterious BRCA1 or BRCA2 germline and somatic mutations (labeled as Mutated, dark green) had significantly higher neoantigen load than patients without (labeled as WT, light green) (p = .009). (c) Breast cancer subtype and neoantigen load. Significant differences in neoantigen load were found between Basal and LumA (p = 4.04E-05), Her2 and LumA (p = .005), LumA and LumB (p = 4.31E-06), and LumA and Normal (p = .0003). (d) Immune subtype and neoantigen load. Significant differences in neoantigen load were found between C1 and C3 (p = .01), C2 and C3 (p = .001), and C1 and C6 (p = .03)
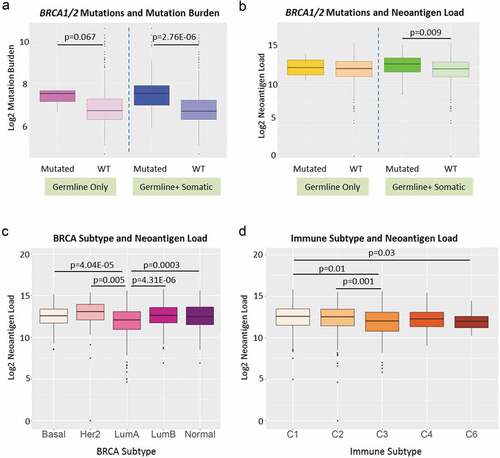
Neoantigen load and BRCA clinical traits
We next investigated the relationships between neoantigen load and other clinical traits, including race, tumor stage, BRCA subtypes and immune subtype.Citation9 As shown in , Her2 subtype had the highest neoantigen load while LumA breast tumors had the lowest load. In addition, immune subtype C1 had the highest mutational load (). We found no significant difference in neoantigen load between different tumor stages (Supplement Figure S3A) or races (Asian, Black and White) for BRCA overall (Supplement Figure S3B). However, when we stratified by BRCA subtype and age group (pre-menopausal: ages 26–44; peri-menopausal: ages 45–54; or post-menopausal: ages 55–90), we found significant differences of neoantigen load for subsets of peri- and post-menopausal women by race (–c). In older women, the general trend is that Black and White women had higher predicted neoantigen load compared to Asians. Neoantigen loads did not differ between black and white women in any strata of age or molecular subtype.
Figure 6. Neoantigen load and race in stratified age groups and breast cancer subtypes. (a) Neoantigen load of patients in pre-menopausal stage (ages 26–44) in Asian (red), Black (green) and White (blue) races separated by Basal, Her2, LumA and LumB subtypes. There is no significant difference among races in any breast cancer subtype. (b) Neoantigen load of patients in peri-menopausal stage (ages 45–54) in Asian (red), Black (green) and White (blue) races separated by Basal, Her2, LumA and LumB subtypes. White had significantly higher neoantigen load compared to Asian in Her2 subtype (p = .0033); both black and white patients had higher neoantigen loads in LumB subtype compared to Asian (p = .037 and p = .04, respectively). (c) Neoantigen load of patients in post-menopausal stage (ages 55–90) in Asian (red), Black (green) and White (blue) races separated by Basal, Her2, LumA and LumB subtypes. White patients had significantly higher neoantigen load than Asian in Basal (p = .0057) and LumB subtypes (p = .0052); both black and white patients had higher neoantigen loads compared to Asian in LumA subtype (p = .037 and p = .0097, respectively)
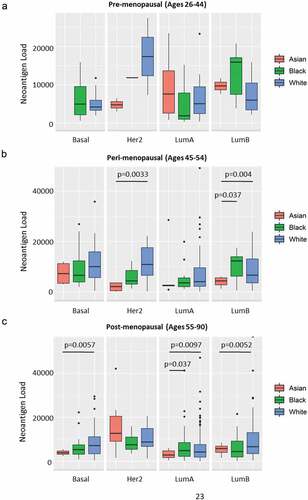
Neoantigen load and survival
The standardized annotations of survival data of all TCGA cases were downloaded from the TCGA Pan-Cancer Clinical Data Resource, Citation27 including clinical outcome endpoints of OS, progression-free interval (PFI), disease-free interval (DFI), and disease-specific survival (DSS). The neoantigen load was divided into different categories by HLA type (class-I or class-II), and mutation types (SNM, INDEL, and gene fusion). The 835 patients were divided into low (bottom 25%), medium (middle 50%) or high (top 25%) neoantigen load groups.
We first performed univariate Cox proportional hazards model of survival analyses for covariate selection (data not shown) and our final covariates included immune filtration, tumor stage, breast cancer subtype, and age at diagnosis. Neoantigen load was not associated with PFI, DFI, or DSS (data not shown). Similar to previous report, Citation6 TMB was not predictive of OS, nor was total neoantigen load (Supplement Figure S4A, S4B). When we grouped the neoantigens into class-I or class-II HLA binders, and into neoantigens arising from small somatic mutations (SNM and INDEL) and those from large structural rearrangements (gene fusion), we found that lower class-I neoantigen load from SNM and INDEL (), as well as lower class-II neoantigen load from SNM and INDEL (), corresponded to worse OS independent of TIL, BRCA subtype, tumor stage, and patient age at diagnosis. As expected, in this multivariate Cox proportional hazards model, both older age at diagnosis and later tumor stages predicted worse OS (,b). Higher TIL regional fraction values were trending toward improved survival, but did not reach statistical significance. Despite the significant correlations between TMB and neoantigen load (–c), including TMB and neoantigen load in the same model did not decrease the predictive value of neoantigen load on OS, and TMB remained not predictive (Supplement Figure S4C-D).
Figure 7. Neoantigen load from SNM and INDEL predicts overall survival. (a) Overall survival of BRCA patients separated by low (bottom 25%), medium (middle 50%) and high (top 25%) class I SNM and INDEL neoantigen load. When corrected for immune filtration, tumor stage, breast cancer subtype, and age at diagnosis using the Cox proportional hazard models, low neoantigen load showed significantly worse survival than medium neoantigen load (p = .04). (b) Overall survival of BRCA patients separated by low (bottom 25%), medium (middle 50%) and high (top 25%) class II SNM and INDEL neoantigen load. When corrected for immune filtration, breast cancer subtype and age cohort using the Cox proportional hazard models, low neoantigen load showed significantly worse survival than medium neoantigen load (p = .042)
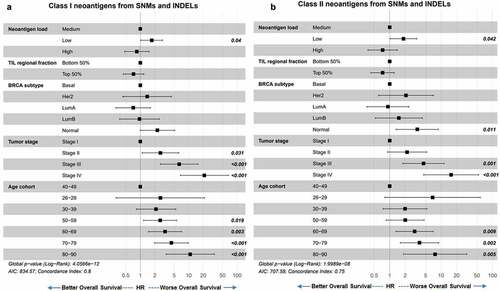
Discussion
TMB has been reported as a prognostic marker for patient overall survival in multiple cancer types after immune checkpoint blockade therapy. Higher TMB in general is associated with better OS with the hypothesis that higher TMB is associated with higher tumor neoantigen load facilitating immune recognition and the development of antitumor immune response.Citation7,Citation8 On the other hand, the relationship between neoantigen load and survival has been controversial in literature. Higher neoantigen load has been linked to better survival in ovarian cancerCitation28 and melanoma, Citation29 but worse survival in multiple myeloma.Citation30 Recently, when screening 33 cancer types, no clear association was found between predicted neoantigen load and survivalCitation9 although only class-I neoantigens were included in the study. Here we studied the TCGA BRCA cohort and directly assessed the prognostic potential of predicted neoantigen load rather than TMB as a predictor of survival independent of known clinical predictors including patient age at diagnosis, molecular subtype, regional TIL fraction and tumor stage. In our study, the combined SNM and INDEL neoantigen load of both HLA class-I and class-II restricted neoepitopes predicted OS in BRCA patients independent of TMB and other clinical factors. To our knowledge, we are the first to report the association between neoantigen load and OS in breast cancer. We did not find an association between fusion neoantigen load and OS. However, previous studies investigating fusion neoantigens have reported reduced survival with higher fusion neoantigen rate in osteosarcoma, Citation31 and no association between fusion neoantigen load and survival in melanoma,Citation32 suggesting that the relationship between fusion neoantigen and survival may be specific to cancer types.
Our approach addressed several key considerations related to neoantigen prediction from genomic sequencing data. For example, the impact of somatic mutations on protein sequences is highly dependent on the transcription splicing isoforms, especially for fusion genes and frame-shift INDELs. Conventionally, only one transcript isoform is used to generate neoepitopes which can result in the underestimate of the neoantigen load if other isoforms are also expressed that harbor a different set of neoepitopes. Second, the accuracy and sensitivity of HLA typing methods are essential for class-I and class-II HLA genotyping. The comparison of HLA genotyping tools has been carried out by multiple groups relying on either real-time PCR validation of a small set of HLA allelesCitation33 or correlations of HLA-types between family trios based on haplotype inferences.Citation34 We have established a novel approach for evaluating HLA genotyping methods, eliminating the uncertainty of haplotype inference and including all called HLA alleles. The details of our approach will be described in a separate manuscript (Ren Y. et al., in preparation), but as illustrated in Supplement Figure S2 using 12 TCGA cases, the performances of the current class-I and class-I HLA genotyping methods are vastly different, as represented by consistencies of the called HLA subtypes between germline (blood), tumor, and normal tissue exomes of the same patient. We selected OptiType and HLA-HD for class-I and class-II for HLA typing based on our test results (more tools were tested, results not shown). In addition, it is worth noting that novel methods are being developed to improve class-II neoantigen prediction, such as the incorporation of affinity-tagging protocols and machine learning models to improve peptide binding prediction.Citation35 With the impact of class-II neoantigens being gradually recognized, better prediction approaches will greatly benefit HLA class-II directed cancer therapies. Third, multiple filtering steps need to be implemented to exclude somatic mutations that are (i) polymorphic germline variants; (ii) also present in a wild-type protein family member or another wide-type protein; or (iii) expressed in normal tissues according to an in-house-curated normal RNA-Seq databases. Furthermore, DNA library preparation and sequencing approaches may affect the detection of fusions. A recent study identified multiple chromosomal rearrangements that had neoantigenic potential in mesothelioma using mate-pair sequencingCitation36 whereas prior approaches did not identify many gene fusions.Citation37,Citation38
Studies have shown that the recognition of neoepitopes by endogenous T cells may elicit protective immune responses without being affected by central T cell tolerance, making neoantigens an ideal target for cancer immunotherapy.Citation4,Citation39,Citation40 Neoantigen vaccines can be developed using different strategies. Premanufactured vaccines may be developed to target neoepitopes related to recurrent somatic mutations. As we showed in , among 37 BRCA driver genes with recurrent mutations, 7 genes (18.92%) harbored many recurrent neoantigens occurring in >1% TCGA BRCA cohort. Another source of recurrent neoantigens is recurrent fusion transcripts. We showed that fusion genes contributed to more than 1/3 of total neoantigen load and will be a rich source for mining recurrent neoantigens. Patients can be screened for these recurrent neoantigen-causal mutations as candidates for neoantigen therapy using premanufactured vaccines. The second approach is the patient-specific neoantigen therapy which requires tumor sequencing, neoantigen prediction, and vaccine manufacturing. Due to the potential large number of neoantigens predicted bioinformatically in each tumor, additional filtering is required to nominate top vaccine candidates.
When correlating neoantigen load with clinical traits, we found that patients with BRCA1 or BRCA2 mutations had higher neoantigen load. This finding is consistent with a previous report that linked higher predicted neoantigens in BRCA1/2 mutated tumors compared to tumors without such mutations in ovarian cancer, Citation28 therefore confirming the link between BRCA1/BRCA2 mutations and immunogenicity. The neoantigen load is lower in Luminal A subtype compared to any other molecular subtype. In addition, we found higher neoantigen load in C1 (wound healing) immune subtype compared to C2 (IFN-γ dominant), C3 (inflammatory) and C6 (TGF-β dominant). Compared to the other immune subtypes, the C1 subtype has elevated expression of angiogenic genes, a high proliferation rate, and a Th2 cell bias to the adaptive immune infiltrate, Citation9 which generally poses less anti-tumor effect and may explain the higher neoantigen load associated with it compared to other immune subtypes.
In summary, by comprehensive neoantigen detection and careful QC, we were able to associate neoantigen load and overall survival in patients with breast cancer from TCGA, and identify INDELs and gene fusions as major contributors to neoantigen burden in BRCA.
Disclosure of potential conflicts of interest
No potential conflicts of interest were disclosed.
Supplemental Material
Download ()Acknowledgments
The results shown here are based upon data generated by the TCGA Research Network: https://www.cancer.gov/tcga. We thank Mayo Clinic Bioinformatics Core and IT team for providing supporting in download and processing TCGA sequencing data.
Supplementary material
Supplemental data for this article can be accessed on the publisher’s website.
Additional information
Funding
References
- Schumacher TN, Schreiber RD. Neoantigens in cancer immunotherapy. Science. 2015;348(6230):69. doi:10.1126/science.aaa4971.
- Wirth TC, Kühnel F. Neoantigen targeting-dawn of a new era in cancer immunotherapy? Front Immunol. 2017;8: 1848-1848. doi:10.3389/fimmu.2017.01848.
- Castle JC, Uduman M, Pabla S, Stein RB, Buell JS. Mutation-derived neoantigens for cancer immunotherapy. Front Immunol. 2019;10(1856). doi:10.3389/fimmu.2019.01856.
- Lennerz V, Fatho M, Gentilini C, Frye RA, Lifke A, Ferel D, Wölfel C, Huber C, Wölfel T.The response of autologous T cells to a human melanoma is dominated by mutated neoantigens. Proc Natl Acad Sci USA. 2005;102(44):16013. doi:10.1073/pnas.0500090102.
- Yarchoan M, Johnson Iii BA, Lutz ER, Laheru DA, Jaffee EM. Targeting neoantigens to augment antitumour immunity. Nat Rev Cancer. 2017;17:209. doi:10.1038/nrc.2016.154.
- Samstein RM, Lee C-H, Shoushtari AN, Hellmann MD, Shen R, Janjigian YY, Barron DA, Zehir A, Jordan EJ, Omuro A, Kaley TJ. Tumor mutational load predicts survival after immunotherapy across multiple cancer types. Nat Genet. 2019;51(2):202–12. doi:10.1038/s41588-018-0312-8.
- Segal NH, Parsons DW, Peggs KS, Velculescu V, Kinzler KW, Vogelstein B, Allison JP. Epitope landscape in breast and colorectal cancer. Cancer Res. 2008;68(3):889. doi:10.1158/0008-5472.CAN-07-3095.
- Verdegaal EME, de Miranda NFCC, Visser M, Harryvan T, van Buuren MM, Andersen RS, Hadrup SR, Van Der Minne CE, Schotte R, Spits H, Haanen JB. Neoantigen landscape dynamics during human melanoma–T cell interactions. Nature. 2016;536:91. doi:10.1038/nature18945.
- Thorsson V, Gibbs DL, Brown SD, Wolf D, Bortone DS, Ou Yang T-H, Porta-Pardo E, Gao GF, Plaisier CL, Eddy JA, Ziv E. The immune landscape of cancer. Immunity. 2018;48(4):812–830.e14. doi:10.1016/j.immuni.2018.03.023.
- Szolek A, Schubert B, Mohr C, Sturm M, Feldhahn M, Kohlbacher O. OptiType: precision HLA typing from next-generation sequencing data. Bioinformatics. 2014;30(23):3310–3316. doi:10.1093/bioinformatics/btu548.
- Kawaguchi S, Higasa K, Shimizu M, Yamada R, Matsuda F. HLA-HD: an accurate HLA typing algorithm for next-generation sequencing data. Hum Mutat. 2017;38(7):788–797. doi:10.1002/humu.2017.38.issue-7.
- Torres-García W, Zheng S, Sivachenko A, Vegesna R, Wang Q, Yao R, Berger MF, Weinstein JN, Getz G, Verhaak RG. PRADA: pipeline for RNA sequencing data analysis. Bioinformatics. 2014;30(15):2224–2226. doi:10.1093/bioinformatics/btu169.
- Patro R, Duggal G, Love MI, Irizarry RA, Kingsford C. Salmon provides fast and bias-aware quantification of transcript expression. Nat Methods. 2017;14:417. doi:10.1038/nmeth.4197.
- Nielsen M, Andreatta M. NetMHCpan-3.0; improved prediction of binding to MHC class I molecules integrating information from multiple receptor and peptide length datasets. Genome Med. 2016;8(1):33. doi:10.1186/s13073-016-0288-x.
- Jensen KK, Andreatta M, Marcatili P, Buus S, Greenbaum JA, Yan Z, Sette A, Peters B, Nielsen M. Improved methods for predicting peptide binding affinity to MHC class II molecules. Immunology. 2018;154(3):394–406. doi:10.1111/imm.12889.
- Asmann YW, Middha S, Hossain A, Baheti S, Li Y, Chai H-S, Sun Z, Duffy PH, Hadad AA, Nair A, Liu X. TREAT: a bioinformatics tool for variant annotations and visualizations in targeted and exome sequencing data. Bioinformatics. 2011;28(2):277–278. doi:10.1093/bioinformatics/btr612.
- Li H. Aligning sequence reads, clone sequences and assembly contigs with BWA-MEM. ArXiv. 2013;1303:3997v2.
- McKenna A, Hanna M, Banks E, Sivachenko A, Cibulskis K, Kernytsky A, Garimella K, Altshuler D, Gabriel S, Daly M, DePristo MA. The genome analysis toolkit: a MapReduce framework for analyzing next-generation DNA sequencing data. Genome Res. 2010;20(9):1297–1303. doi:10.1101/gr.107524.110.
- Kocher J-PA, Quest DJ, Duffy P, Meiners MA, Moore RM, Rider D, Hossain A, Hart SN, Dinu V. the biological reference repository (BioR): a rapid and flexible system for genomics annotation. Bioinformatics. 2014;30(13):1920–1922. doi:10.1093/bioinformatics/btu137.
- Narang P, Chen M, Sharma AA, Anderson KS, Wilson MA. The neoepitope landscape of breast cancer: implications for immunotherapy. BMC Cancer. 2019;19(1):200. doi:10.1186/s12885-019-5402-1.
- Lawrence MS, Stojanov P, Polak P, Kryukov GV, Cibulskis K, Sivachenko A, Carter SL, Stewart C, Mermel CH, Roberts SA, Kiezun A. Mutational heterogeneity in cancer and the search for new cancer-associated genes. Nature. 2013;499:214. doi:10.1038/nature12213.
- Couch FJ, DeShano ML, Blackwood MA, Calzone K, Stopfer J, Campeau L, Ganguly A, Rebbeck T, Weber BL, Jablon L, Cobleigh MA. BRCA1 mutations in women attending clinics that evaluate the risk of breast cancer. N Engl J Med. 1997;336(20):1409–1415. doi:10.1056/NEJM199705153362002.
- Berry DA, Parmigiani G, Sanchez J, Schildkraut J, Winer E. Probability of carrying a mutation of breast-ovarian cancer gene BRCA1 based on family history. JNCI. 1997;89(3):227–237. doi:10.1093/jnci/89.3.227.
- King M-C, Marks JH, Mandell JB. Breast and ovarian cancer risks due to inherited mutations in BRCA1 and <em>BRCA2</em>. Science. 2003;302:643.
- Wen WX, Leong C-O. Association of BRCA1- and BRCA2-deficiency with mutation burden, expression of PD-L1/PD-1, immune infiltrates, and T cell-inflamed signature in breast cancer. PLoS One. 2019;14(4):e0215381. doi:10.1371/journal.pone.0215381.
- Borg Å, Haile RW, Malone KE, Capanu M, Diep A, Törngren T, Teraoka S, Begg CB, Thomas DC, Concannon P, Mellemkjaer L. Characterization of BRCA1 and BRCA2 deleterious mutations and variants of unknown clinical significance in unilateral and bilateral breast cancer: the WECARE study. Hum Mutat. 2010;31(3):E1200–E1240. doi:10.1002/humu.21202.
- Liu J, Lichtenberg T, Hoadley KA, Poisson LM, Lazar AJ, Cherniack AD, Kovatich AJ, Benz CC, Levine DA, Lee AV, Omberg L. An integrated TCGA pan-cancer clinical data resource to drive high-quality survival outcome analytics. Cell. 2018;173(2):400–416.e11. doi:10.1016/j.cell.2018.02.052.
- Strickland KC, Howitt BE, Shukla SA, Rodig S, Ritterhouse LL, Liu JF, Garber JE, Chowdhury D, Wu CJ, D’Andrea AD, Matulonis UA. Association and prognostic significance of BRCA1/2-mutation status with neoantigen load, number of tumor-infiltrating lymphocytes and expression of PD-1/PD-L1 in high grade serous ovarian cancer. Oncotarget. 2016;7:12. doi:10.18632/oncotarget.7277.
- Lauss M, Donia M, Harbst K, Andersen R, Mitra S, Rosengren F, Salim M, Vallon-Christersson J, Törngren T, Kvist A, Ringnér M. Mutational and putative neoantigen load predict clinical benefit of adoptive T cell therapy in melanoma. Nat Commun. 2017;8(1):1738. doi:10.1038/s41467-017-01460-0.
- Miller A, Asmann Y, Cattaneo L, Braggio E, Keats J, Auclair D, Lonial S, Russell SJ, Stewart AK. High somatic mutation and neoantigen burden are correlated with decreased progression-free survival in multiple myeloma. Blood Cancer J. 2017;7(9):e612–e612. doi:10.1038/bcj.2017.94.
- Rathe SK, Popescu FE, Johnson JE, Watson AL, Marko TA, Moriarity BS, Ohlfest JR, Largaespada DA. Identification of candidate neoantigens produced by fusion transcripts in human osteosarcomas. Sci Rep. 2019;9(1): 358-358. doi:10.1038/s41598-018-36840-z.
- Wei Z, Zhou C, Zhang Z, Guan M, Zhang C, Liu Z, Liu Q. The landscape of tumor fusion neoantigens: a pan-cancer analysis. iScience. 2019;21:249–260. doi:10.1016/j.isci.2019.10.028.
- Kiyotani K, Mai TH, Nakamura Y. Comparison of exome-based HLA class I genotyping tools: identification of platform-specific genotyping errors. J Hum Genet. 2017;62(3):397–405. doi:10.1038/jhg.2016.141.
- Matey-Hernandez ML, Maretty L, Jensen JM, Petersen B, Sibbesen JA, Liu S, et al. Benchmarking the HLA typing performance of polysolver and optitype in 50 danish parental trios. BMC Bioinf. 2018;19(1):239. doi:10.1186/s12859-018-2239-6.
- Abelin JG, Harjanto D, Malloy M, Suri P, Colson T, Goulding SP, Creech AL, Serrano LR, Nasir G, Nasrullah Y, McGann CD. Defining HLA-II ligand processing and binding rules with mass spectrometry enhances cancer epitope prediction. Immunity. 2019;51(4):766–779.e17. doi:10.1016/j.immuni.2019.08.012.
- Mansfield AS, Peikert T, Smadbeck JB, Udell JBM, Garcia-Rivera E, Elsbernd L, Erskine CL, Van Keulen VP, Kosari F, Murphy SJ, Ren H. Neoantigenic potential of complex chromosomal rearrangements in mesothelioma. J Thoracic Oncol. 2019;14(2):276–287. doi:10.1016/j.jtho.2018.10.001.
- Hmeljak J, Sanchez-Vega F, Hoadley KA, Shih J, Stewart C, Heiman D, Tarpey P, Danilova L, Drill E, Gibb EA, Bowlby R. Integrative molecular characterization of malignant pleural mesothelioma. Cancer Discov. 2018;8(12):1548. doi:10.1158/2159-8290.CD-18-0804.
- Bueno R, Stawiski EW, Goldstein LD, Durinck S, De Rienzo A, Modrusan Z, Gnad F, Nguyen TT, Jaiswal BS, Chirieac LR, Sciaranghella D. Comprehensive genomic analysis of malignant pleural mesothelioma identifies recurrent mutations, gene fusions and splicing alterations. Nat Genet. 2016;48:407. doi:10.1038/ng.3520.
- Ostroumov D, Fekete-Drimusz N, Saborowski M, Kühnel F, Woller N. CD4 and CD8 T lymphocyte interplay in controlling tumor growth. Cellular and Mol Life Sci. 2018;75(4):689–713. doi:10.1007/s00018-017-2686-7.
- Rizvi NA, Hellmann MD, Snyder A, Kvistborg P, Makarov V, Havel JJ, Lee W, Yuan J, Wong P, Ho TS, Miller ML. Mutational landscape determines sensitivity to PD-1 blockade in non–small cell lung cancer. Science. 2015;348(6230):124. doi:10.1126/science.aaa1348.