ABSTRACT
Sialic acid-binding immunoglobulin-like lectin 15 (Siglec-15) is considered a novel anti-tumor target comparable to programmed cell death 1 ligand 1(PD-L1). However, little is known about Siglec-15. Our study aims to understand its expression signature, prognosis value, immune infiltration pattern, and biological function using multi-omic bioinformatics from public databases and verify them in lung cancer patients. Integrated analysis of The Cancer Genome Atlas and Genotype-Tissue Expression portals showed Siglec-15 was overexpressed across cancers. Genetic and epigenetic alteration analysis was performed using cBioportal and UALCAN, showed Siglec-15 was regulated at the genetic and epigenetic levels. Survival estimated using Kaplan–Meier plotter indicated high Siglec-15 expression correlated with favorable or unfavorable outcomes depending on the different type and subtype of cancer. Components of immune microenvironment were analyzed using CIBERSORT, and the correlation between immune cells and Siglec-15 was found to be distinct across cancer types. Based on Gene Set Enrichment Analysis, Siglec-15 was implicated in pathways involved in immunity, metabolism, cancer, and infectious diseases. Lung cancer patients with positive Siglec-15 expression showed significantly short survival rates in progression-free survival concomitant with reduced infiltration of CD20 + B, and dendritic cells by immunohistochemistry. Quantitative real-time PCR results indicated the overexpression of Siglec-15 was correlated with activation of the chemokine signaling pathway. In conclusion, Siglec-15 could serve as a vital prognostic biomarker and play an immune-regulatory role in tumors. These results provide us with clues to better understand Siglec-15 from the perspective of bioinformatics and highlight the importance of Siglec-15 in many types of cancer.
Introduction
Tumor cells and the tumor microenvironment (TME) can secrete or express various signaling molecules, that act on immune checkpoints expressed in immune cells to suppress immune responses. The immune escape mechanisms in the TME are highly heterogeneous, and this inherent genomic instability helps cancer cells avoid cytotoxic or targeted therapy in metastatic tumors.Citation1 The approval of ipilimumab in 2011 as cancer immunotherapy has opened a new chapter in anti-cancer therapy.Citation2 Immunotherapy as a fourth method of cancer treatment after surgery, radiotherapy, and chemotherapy is turning the dream of a clinical cure for advanced tumor patients into reality.Citation3–Citation7 Surprisingly, neoadjuvant immunotherapy appears to rewrite the current status of treatment for early cancer.Citation8 Blocking programmed cell death-1 (PD-1)/PD-1 ligand 1 (PD-L1) is widely regarded as a successful strategy in normalization cancer immunotherapy.Citation9,Citation10 However, treatment using anti-PD-1/PD-L1 pathway is not applicable to all patients.Citation11,Citation12 Finding new targets to make up for the lack of PD-1/PD-L1 antibodies is imperative.
Siglecs are a family of sialic acid-binding immunoglobulin-like lectins that specifically recognize sialylated glycans and regulate immune cell function.Citation13 In recent years, an increasing number of Siglec members have been found to play a crucial role in tumor immunosuppression.Citation14–Citation16 Siglec-7 and Siglec-9 expressed on the surface of natural killer (NK) cells and interacting with sialoglycans on cancer cells inhibited NK cells cytolytic capacity.Citation17,Citation18 Siglec-9 on T cells and tumor-associated macrophages (TAMs) also play a role in “immune checkpoints” leading to immune evasion.Citation15 The glycoprotein CD24 is a novel “do not eat me” signal that prevents cancer cells from phagocytosis by binding to Siglec-10 on macrophages.Citation14 Siglec-15, originally classified into Siglecs family as a type I transmembrane protein. It is well conserved through vertebrate evolution and is mainly expressed on a subset of myeloid lineage cells.Citation19 Siglec-15 was found to be overexpressed in giant cell tumors of the bone and regulates bone remodeling and osteoclast differentiation through interaction with DAP12.Citation20–Citation24 Siglec-15 expressed on TAMs may contribute to tumor immunosuppression by cooperating with DAP12 and Syk to increase TGF-β secretion.Citation25 Chen et al. first revealed that Siglec-15 showed high homology and similar domain composition with B7 family members, which have the ability to sustainably suppress T-cell responses. The expression of Siglec-15 is mutually exclusive to that of PD-L1.Citation26 Siglec-15 is considered a new promising target for immune normalization independent of the PD-1/PD-L1 pathway. Targeting Siglec-15 may be an effective alternative therapy for patients who do not respond to PD-1/PD-L1 antibodies.Citation26–Citation28 A humanized monoclonal antibody against Siglec-15 is currently being evaluated in patients with advanced or metastatic solid tumors in phase I clinical trials.Citation29
This study was conducted to comprehensively analyze Siglec-15 mRNA expression signature, genetic and epigenetic characteristics, prognostic value, correlation with tumor-infiltrating immune cells, and associated pathways using next-generation sequencing (NGS) data from public platforms,Citation30 and we further performed immunohistochemistry (IHC) and real-time quantitative PCR (RT-qPCR) to verify its association with TME and signaling pathway. Our research aims to provide more information to better understand the significance of Siglec-15 in various cancers.
Materials and methods
The cancer genome atlas (TCGA)
TCGA is a web-based, freely accessible database, which has generated large amounts of NGS data with a landscape of more than 11,000 tumors across 33 cancer types until 2018. It provided gene expression, methylation, copy number variation data sets, and clinical data.Citation30,Citation31
Genotype-tissue expression (GTEx)
GTEx provides gene expression data from 53 normal tissue sites across nearly 1000 people by RNA sequencing, which is publicly available. RNA-seq data including GTEx and TCGA data were derived from UCSC Xena data hubs (http://xena.ucsc.edu/) for a pan-cancer differential expression of Siglec-15. TCGA and GTEx data were integrated using R version 3.5.3 and limma package.
cBioPortal
The cBioPortal for Cancer Genomics (http://www.cbioportal.org) is a repository of cancer genomics datasets.Citation32,Citation33 We investigated the copy number alterations (CNA) and mutations of Siglec-15 in different cancers.
UALCAN
The UALCAN network (http://ualcan.path.uab.edu)Citation34 was used to analyze DNA methylation levels of the Siglec-15 promoter between tumor and normal tissues of lung adenocarcinoma (LUAD), cervical squamous cell carcinoma and endocervical adenocarcinoma (CESC), colon adenocarcinoma (COAD), kidney renal papillary cell carcinoma (KIRP), rectum adenocarcinoma (READ), kidney renal clear cell carcinoma (KIRC), and prostate adenocarcinoma (PRAD). Data were collected and shown as violin plots created using R version 3.5.3 and ggplot2 package.
Kaplan–Meier plotter
Using the Kaplan–Meier plotter (http://kmplot.com/analysis/),Citation35 we analyzed the prognostic values of Siglec-15 mRNA expression in different cancers mainly from TCGA (RNA-seq) and Gene Expression Omnibus (GEO) (microarray). We computed the log-rank p-value and HR with 95% confidence intervals. A log p-value <0.05 was considered statistically significant. The significant survival data were collected and shown as forest plots created using R version 3.5.3 and survival package.
TISIDB
TISIDB is a website for gene- and tumor-immune interaction (http://cis.hku.hk/TISIDB/index.php).Citation36 It was used to analyze Siglec-15 gene expression in different immune subtypes, including C1 (wound healing), C2 (IFN-γ dominant), C3 (inflammatory), C4 (lymphocyte deplete), C5 (immunologically quiet), and C6 (TGF-β dominant) subtypes; and Siglec-15 gene expression in different molecular subtypes tumor samples from TCGA.
CIBERSORT
CIBERSORT calculates the putative proportion of immune cell fraction from gene expression profiles.Citation37 To estimate relative abundance of tumor-infiltrating immune cells in tumor mass from TCGA and GEO, we used a reference set with 22 sorted immune cell subtypes (LM22) by online analytical platform CIBERSORT (https://cibersort.stanford.edu/).
Gene set enrichment analysis (GSEA)
We downloaded the RNA-seq data from TCGA portal (https://portal.gdc.cancer.gov/). We have chosen the Siglec-15 and its co-expression genes to perform GSEA to discover potential pathways by Kyoto Encyclopedia of Genes and Genomes (KEGG) and gene ontology (GO) terms affected by Siglec-15 using R version 3.5.3 and clusterProfiler, ggplot2, enrichplot, and org.Hs.eg.db packages. GO analysis was classified into three categories: biological process (BP), cellular component (CC), and molecular function (MF). Results with a normalized enrichment score (NES) ≥1.0 and false discovery rate (FDR) adjusted p-value <0.25 were considered statistically significant.
Patients and tissue samples
Data from 103 patients who had received radical (R0) resection with histological diagnosis of LUAD at the Tianjin Medical University Cancer Institute and Hospital, China, from January 2013 to May 2013, were retrospectively collected. All patients underwent R0 resection and lymph node dissection. The lymph node and lung cancer stages were categorized according to the eighth edition of the International Association for the Study of Lung Cancer TNM staging system. Histological analysis was reviewed by a pathologist after H&E staining. The 2 mm tissue cores are chosen as representative tumor parts on tissue microarray (TMA), obtained from nonnecrotic areas in the primary tumors using a needle. The use of patient information and tissues was sanctioned by the Ethics Committee of the Tianjin Medical University Cancer Institute and Hospital.
Immunohistochemical staining and evaluation
After deparaffinized, rehydrated, and antigen retrieval (Siglec-15, citrate buffer pH 6.0; CD4, CD8, CD20, CD11 c, FoxP3, CD68, CD163, EDTA 9.0), TMA slides were blocked with 3% hydrogen peroxide and 5% goat serum. After being incubated overnight at 4°C with primary antibodies, slides were incubated with enhancer and secondary antibody for half an hour at room temperature. A DAB Substrate Kit was used for chromogenic reaction. Finally, the sections were counterstained with hematoxylin, then dehydrated, cleared, and evaluated. Primary antibodies include anti-Siglec-15 antibody (PA5-72765, Thermo Fisher Scientific, USA, 1:100), anti-CD4 antibody (ab133616, Abcam, USA, 1:200), anti-CD8 antibody (SP16, Thermo Fisher Scientific, USA, 1:200), anti-CD20 antibody (EP459Y, Abcam, USA, 1:200), anti-CD68 antibody (KP1, Thermo Fisher Scientific, USA, 1:100), anti-CD163 antibody (10D6, Thermo Fisher Scientific, USA, 1:100), anti-FoxP3 antibody (236A/E7, Abcam, USA, 1:100), and anti-CD11c antibody (EP1347Y, Abcam, USA, 1:200)
Immunostaining was evaluated under light microscopy at 400× magnification by two independent pathologists. Siglec-15 was observed in the membrane and/or cytoplasm of the tumor cells and immune cells. In our study, we identified positive Siglec-15 expression on tumor cells as membrane staining. The absolute number of immune cells was counted manually. The total number of stained immune cells (in the central tumor and peritumoral stroma) was included in the analyses.
Statistical analysis
We summarized patient characteristics through descriptive statistics. Overall survival (OS) was defined as the time interval between the date of surgery and date of death. Progression-free survival (PFS) was defined as the time interval between the date of surgery and date of progression. Survival analyses were conducted by Kaplan–Meier curves (p-values from Log-rank test) by R version 3.5.3 and survival package. Hazard ratios (HR) were calculated using R package. All statistics in – were two-sided and analyzed through SPSS statistical software (version 24.0.0). Statistical significance was defined as p < .05.
Table 1. Patient clinical parameters and their association with Siglec-15 expression.
Table 3. Univariate survival analysis of Siglec-15 expression in subgroups with different clinical parameters.
RT-qPCR
Total RNA was isolated from patients with LUAD by Trizol and converted into cDNA by reverse transcription (GoScript™ Reverse Transcription System, USA). The mRNA expression of Siglec-15, immune-related genes, and pathway-related genes were assayed by RT-qPCR. Siglec-15 primers purchased from Sino Biological Inc. RT-qPCR primers of other genes are provided in Supplementary Table S1.
Results
Siglec-15 mRNA expression in human cancers
We integrated tumor samples from TCGA and normal samples from GTEx databases to identify Siglec-15 mRNA expression characteristics. Collectively, Siglec-15 was upregulated across most cancer types, such as breast invasive carcinoma (BRCA), cholangiocarcinoma (CHOL), COAD, esophageal carcinoma (ESCA), glioblastoma multiforme (GBM), head and neck squamous cell carcinoma (HNSC), kidney chromophobe (KICH), acute myeloid leukemia (LAML), brain lower-grade glioma (LGG), liver hepatocellular carcinoma (LIHC), LUAD, ovarian serous cystadenocarcinoma (OV), pancreatic adenocarcinoma (PAAD), READ, skin cutaneous melanoma (SKCM), stomach adenocarcinoma (STAD), thyroid carcinoma (THCA), uterine corpus endometrial carcinoma (UCEC), and uterine carcinosarcoma (UCS) (). When only tumor and adjacent nearby tissues in TCGA were included, Siglec-15 was found to be upregulated in COAD, HNSC, KICH, KIRP, LUAD, READ, the metastatic tissue of SKCM, THCA, and UCEC. Siglec-15 was downregulated in PRAD via the TIMER database (Supplementary Figure S1a). Using Oncomine, Siglec-15 was found to be overexpressed in bladder cancer, brain, and central nervous system (CNS) cancer, breast cancer, cervical cancer, colorectal cancer, kidney cancer, leukemia, lymphoma, ovarian cancer, and sarcoma but was underexpressed in gastric and pancreatic cancers (Supplementary Figure S1b).
Figure 1. The transcription levels of Siglec-15 in human cancers.
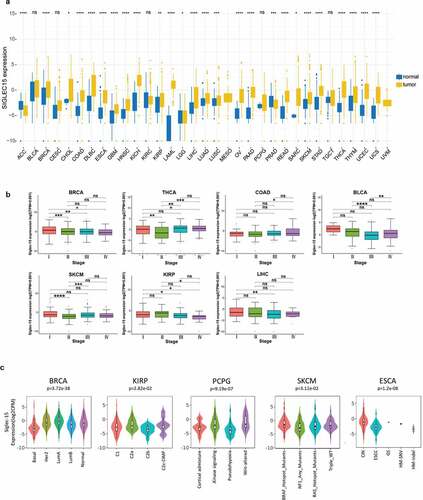
We then analyzed mRNA expression patterns of Siglec-15 in different clinical stages and molecular subtypes. Siglec-15 expression varied significantly in different clinical stages of BRCA, THCA, COAD, SKCM, KIRP, LIHC, and bladder urothelial carcinoma (BLCA) (). Siglec-15 expression in different molecular subtypes of BRCA, KIRP, pheochromocytoma and paraganglioma (PCPG), SKCM, and ESCA was significantly different (). No association was found between Siglec-15 expression and cancer stage, or molecular subtype in other cancers (Supplementary Figure S1c, S1d).
CNA and DNA methylation alterations of Siglec-15 across different human cancers
Genetic and epigenetic changes play a critical role in regulating cancer development and immune tolerance. Genetic alterations of Siglec-15 were further explored using cBioPortal. We found that patients with high Siglec-15 expression were accompanied by gene alterations in OV, HNSC, sarcoma (SARC), UCEC, BLCA, BRCA, SKCM, and lymphoid neoplasm diffuse large B-cell lymphoma (DLBC) (, Supplementary Figure S2a). The trends in Siglec-15 genetic alteration were consistent with its mRNA levels in these cancers. To explore gene expression variation contributed by CNA, we analyzed the relationship between gene expression and relative linear copy number values. The results revealed a positive correlation between Siglec-15 expression and CNA in COAD, HNSC, LUSC, OV, STAD, testicular germ cell tumors (TGCT), and UCEC, but a negative correlation was observed in SARC and PAAD (). No association was found between Siglec-15 expression and CNA in other cancers (Supplementary Figure S2b). However, expression alterations of Siglec-15 cannot be reasonably explained by CNA in other cancers, including LUAD, CESC, COAD, KIRP, READ, and KIRC. We then determined whether epigenetic mechanisms played a role in regulating Siglec-15 mRNA expression. DNA methylation is an epigenetic mechanism that can control gene expression without incurring any change to the genomic sequence.Citation38 To link promoter DNA methylation levels to Siglec-15 expression, we conducted a correlation analysis between DNA methylation states and Siglec-15 expression (). A significant negative correlation was found between DNA methylation and Siglec-15 expression in THCA, BLCA, UCEC, SARC, TGCT, thymoma (THYM), BRCA, UCS, SKCM, LIHC, STAD, PAAD (−1 < Pearson r < −0.3, ), and in CESC, COAD, KICH, KIRP, LUAD, PCPG, PRAD, and READ (−0.3 < Pearson r < −0.1, Supplementary Figure S3a). No association was found between Siglec-15 expression and DNA methylation in other cancers (Supplementary Figure S3b). We then investigated the differential promoter DNA methylation status of Siglec-15 between cancer and adjacent normal tissues by using UALCAN (). Siglec-15 had lower DNA methylation levels in LUAD, CESC, COAD, KIRP, READ, and KIRC tissues than in adjacent normal tissues. Therefore, the abnormal increase of Siglec-15 mRNA expression in some cancers is likely a result of both genetic alterations and lower DNA methylations levels.
Figure 2. CNA and DNA methylation of Siglec-15 in human cancers.
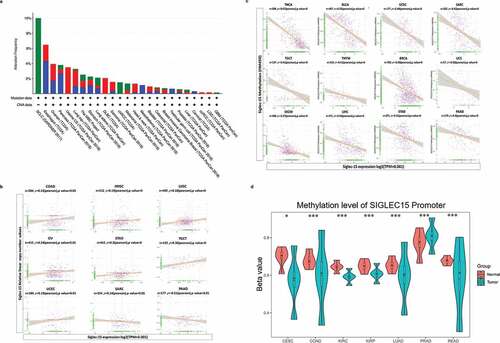
Correlation analysis between mRNA expression of Siglec-15 and prognostic value
After exploring the characteristics of Siglec-15 expression at the DNA and mRNA levels, we investigated the effect of abnormal Siglec-15 mRNA expression on prognosis by using Kaplan–Meier plotter. Our results showed that Siglec-15 had different prognostic values in different types of cancer (). In TCGA data, upregulated Siglec-15 expression was found to be associated with longer OS in BLCA, BRCA, HNSC, THCA, and UCEC and with longer relapse-free survival (RFS) in BRCA, LIHC, OV, and UCEC (). By contrast, upregulated Siglec-15 expression was associated with shorter OS in KIRC, PAAD, and SARC, and with shorter RFS in PAAD and SARC (). Siglec-15 did not show prognostic values for other cancers (Supplementary Figure S4). In addition to TCGA, we analyzed ChIP and RNAseq data from GEO and other projects to acquire survival information in different cancer subtypes. We found that high mRNA expression of Siglec-15 indicated a better prognosis for BRCA-luminal A, BRCA-luminal B, OV and STAD-diffuse (). High Siglec-15 expression indicated good OS, RFS, post-progression survival (PPS), and distant metastasis-free survival (DMFS) in BRCA-luminal A; good OS and RFS in BRCA-luminal B; good OS and PFS in OV-serous; and good first-progression (FP) in STAD-diffuse. High Siglec-15 expression predicted worse outcomes in LUAD, BRCA-basal, BRCA-Her2+ ,and STAD (). Furthermore, high Siglec-15 expression was associated with poor OS and FP in LUAD; poor OS in BRCA-basal; poor RFS in BRCA-Her2+; poor OS and PPS in STAD-intestinal; and poor OS in STAD-mixed. Unexpectedly, high Siglec-15 expression predicted good RFS in BRCA-basal. Siglec-15 expression had the contrasting prognostic significance in different BRCA or STAD subtypes, which may be owing to its different immune infiltration properties. Siglec-15 mRNA expression was not significantly associated with the prognosis in other cancers from the Kaplan-Meier plotter (Supplementary Figure S5).
Figure 3. The prognostic significance of Siglec-15 assessed by Kaplan-Meier analysis.
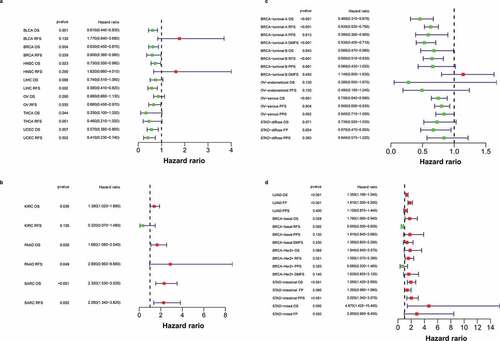
Relationship between mRNA expression of Siglec-15 and the tumor-immune microenvironment
After defining the prognostic value of Siglec-15, we explored the relationship between Siglec-15 and tumor-infiltrating immune cells in cancers (). First, we assessed components of the immune microenvironment across 33 cancers from TCGA by CIBERSORT algorithm. The clustering heatmap according to the relationship between Siglec-15 and immune cells showed a positive correlation between Siglec-15 and regulatory T cells (Tregs) in several cancers, especially ESCA (Spearman r = 0.31, p = .0002) and TGCT (Spearman r = 0.59, p = 3.77E-15). Most correlations with macrophages were positive. However, Siglec-15 was negatively correlated with macrophages in BLCA, and adrenocortical carcinoma (ACC). Because of its function in suppressing T cells, we focused on the relationship between Siglec-15 and CD8 + T cells. Siglec-15 was positively associated with CD8 + T cells in ACC and negatively associated with CD8 + T cells in BRCA-basal, THCA, and UCS. It is essential to note that the relationship between Siglec-15 and immune cells represents diverse functions in different breast cancer subtypes. In BRCA-basal, Siglec-15 was negatively correlated with follicular helper T cells (Spearman r = −0.37, p = 5.74E-07), activated NK cells, activated dendritic cells (DCs), and CD8 + T cells. However, Siglec-15 was not correlated with immune cells in the other BRCA subtypes. Additionally, no significant correlation was found between the composition of immune cells and Siglec-15 in COAD, LIHC, PAAD, READ, STAD, THCA, and UCEC.
Figure 4. Correlation analysis between Siglec-15 expression and tumor-infiltrating immune cell.
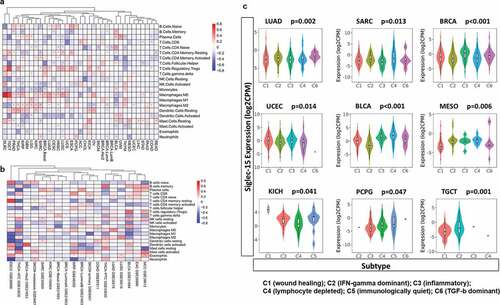
We also used multiple databases from GEO to analyze the relationship between Siglec-15 and the immune microenvironment for further validation (). Siglec-15 was positively associated with memory B cells and negatively macrophage (M0, M1) in BLCA; positively with γδT cells in BRCA-Basal; positively with macrophage (M0) in LUAD, which were consistent with the results in TCGA. We found that Siglec-15 was negatively associated with activated NK cells and positively associated with activated DCs and Tregs in BLCA in GSE31684. Siglec-15 was positively associated with naive B cells, neutrophils, and activated mast cells and negatively associated with follicular helper T cells in metastatic SKCM in GSE8401 compared to the associations in primary SKCM. Combining the results in TCGA and GSE30219 for LUAD and LUSC resulted a similar clustering pattern. This included a positive relationship with macrophages and a negative relationship with activated DCs. In BRCA-basal, Siglec-15 showed a significantly positive correlation with γδT cells in both TCGA and GSE21653. These results suggest opposite correlation patterns in esophageal squamous cell carcinoma (ESCC) and esophageal adenocarcinoma (EAC) in GSE26886; however, the results were not significant. This is possibly owing to the limited sample size (ESCC n = 9; EAC n = 21). Additional details can be found in Supplementary Table S2.
Using molecular typing of immune subtypes,Citation39 we investigated Siglec-15 mRNA expression in different immune subtypes. A significant difference in Siglec-15 expression characteristics was observed across C1 (wound healing), C2 (IFN-γ dominant), C3 (inflammatory), C4 (lymphocyte deplete), C5 (immunologically quiet), and C6 (TGF-β dominant) subtypes in LUAD, SARC, BRCA, UCEC, BLCA, mesothelioma (MESO), KICH, PCPG, and TGCT (). No significant difference was observed for other cancers (Supplementary Figure S5). The highest level of Siglec-15 expression was observed in subtype C6 in LUAD, C4 in SARC, C3 in BRCA and UCEC. Differential expression of Siglec-15 in different immune subtypes may partially explain why Siglec-15 played contrasting roles in the prognosis of various cancers.
Significant pathways influenced by Siglec-15
To further investigate the potential functions of Siglec-15, we performed KEGG and GO GSEA across many cancer types (). The heatmap showed a clear clustering pattern by KEGG analysis (NES ≥ 1.0, FDR < 0.25). Immune-related pathways were highly enriched in a large fraction of cancers, such as TGCT, SARC, HNSC, PRAD, STAD, LGG, THCA, LUSC, GBM, KIRP, LUAD, and SKCM. Siglec-15 was significantly involved in activation of the “chemokine signaling pathway,” “JAK-STAT signaling pathway,” “NF-kappa B signaling pathway,” “toll-like receptor signaling pathway,” “T cell receptor signaling pathway,” “natural killer cell-mediated cytotoxicity,” “B cell receptor signaling pathway,” and “TNF signaling pathway.” It also influenced several cancer-related pathways, including the “PI3 K-Akt signaling pathway,” “MAPK signaling pathway,” “VEGF signaling pathway,” and “apoptosis.” Siglec-15 affected “metabolic pathways,” “mTOR signaling pathway” and “HIF-1 signaling pathway” in several cancers. It is interesting to note that Siglec-15 suppressed most pathways mentioned above, while some pathways such as “metabolic pathways” were activated in BLCA. Additional details can be found in Supplementary Table S3.
Figure 5. Significant pathways influenced by Siglec-15.
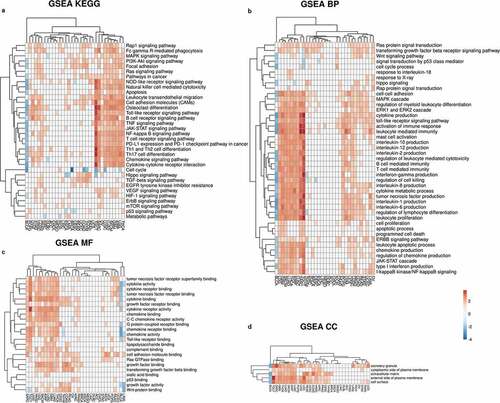
GO analysis indicated that Siglec-15 had an effect on immune-related BP, CC, and MF in most cancers. In particular, “cytokine production,” “cytokine metabolic process,” “MAPK cascade,” “ERK1 and ERK2 cascade,” “ERBB signaling pathway,” “JAK-STAT cascade,” and “toll-like receptor signaling pathway” in GO BP terms were found to be active in LGG, GBM, SKCM, LUAD, THCA, KIRP, and TGCT (). In GO MF terms, cytokine-related MFs were enriched in SARC, TGCT, UVM, HNSC, LGG, SKCM, KIRP, LUSC, GBM, LUAD, and THCA (). In the CC category, “external side of plasma membrane,” “cytoplasmic side of plasma membrane,” “cell surface,” “secretory granule,” and “extracellular matrix” were the main enriched terms ().
Preliminary experimental verification of Siglec-15 signature in LUAD
To verify the above results, we focused on Siglec-15 expression, prognostic value, relationship with immune cells, and associated pathways in LUAD and conducted in vitro experiment using tissues samples collected from the Tianjin Medical University Cancer Institute and Hospital. We first assessed the expression of Siglec-15 by 20 matched-pair LUAD tissue samples by RT-qPCR. The results showed Siglec-15 was significantly upregulated in primary tumor tissues (p = .0266) (). As mentioned above, Siglec-15 was associated with activation of the “chemokine signaling pathway” and “cytokine-cytokine receptor interaction” in KEGG and GO terms “cytokine production” and “regulation of cytokine production” in LUAD (). We focused on the “chemokine signaling pathway” (Supplementary Figure S6a) to verify it by RT-qPCR using 20 LUAD tissue samples (). Expressions of genes belonging to chemokine signaling pathway (CXCR3, GNAI2, RAC2, and MMP9) and the downstream PI3 K-AKT signaling pathway (AKT3, LPAR6, and CDK6) showed a trend toward positive association with Siglec-15, although this was not significant. And PI3 K was significantly positively associated with Siglec-15 expression (Pearson r = 0.54, p = .01). We divided the cancer tissues into two groups (Siglec-15 high and Siglec-15 low) and evaluated mRNA expression levels of key genes in the pathways (Supplementary Figure S6b). PI3 K was highly expressed in the Siglec-15 high group (p < .05). Other genes showed a trend toward higher expression in Siglec-15 high group, although this was not significant.
Figure 6. Preliminary experimental verification of Siglec-15 signature in LUAD.
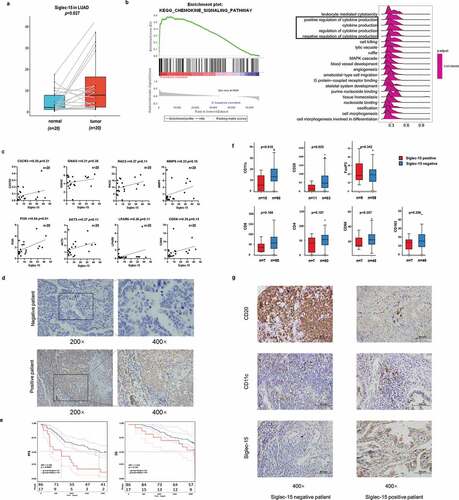
Simultaneously, we performed IHC to evaluate the expression and prognosis value of Siglec-15 in a cohort of 103 LUAD specimens. The patient demographics and relationship between Siglec-15 expression and clinicopathological factors are shown in . Siglec-15 positive expression was not associated with tumor size, lymphatic metastasis, TNM stage, smoking index, age, and gender by the Fisher’s exact test. A total of 17 (16.5%) patients had positive Siglec-15 staining and were classified as either membrane positive or membrane negative (). Images of representative negative and positive samples were taken at 200× and 400× magnification (). T classification, N classification, and clinical stage were risk factors used to determine OS and PFS (). The survival curves revealed that patients with positive expression of Siglec-15 had an unfavorable PFS (HR = 2.755, p = .0003). No significant difference in OS was observed between Siglec-15 positive and negative patients (HR = 1.734, p = .138) ().
Table 2. Univariate survival analysis of clinical parameters and Siglec-15 expression with PFS and OS in patients with LUAD.
Moreover, we investigated the prognostic value of Siglec-15 in selective patient subgroups of LUAD classified by clinicopathological factors (). Siglec-15 correlated with poor prognosis for PFS in patients with larger tumor size (T2+ T3+ T4), without lymph node metastasis (N0), earlier clinical stage (I+ II), and a smoking index less than 400. Positive Siglec-15 expression showed greater significant prognostic value in patients aged ≤60 y (PFS HR = 2.941, p = .003) than >60 y (PFS HR = 2.552, p = .034). Positive Siglec-15 expression indicated poorer PFS in male patients (HR = 5.04, p < .001) than in female patients (HR = 2.065, p = .043). No significant difference in OS was observed. We found that Siglec-15 was a poor prognostic predictor in our LUAD cohort, which is generally consistent with our survival analysis of the database, especially in GEO.
We perform IHC to evaluate the relative levels of immune cells infiltration of Siglec-15 in a cohort of 88 LUAD specimens with Siglec-15 negative and positive patients (, , Supplementary Figure S6). The patient clinicopathological characteristics and Siglec-15 expression are shown in Table S4. The results showed that infiltration of B cells and DCs was stronger in Siglec-15 negative patients than in Siglec-15 positive patients (p < .05). However, there was no difference in the infiltration of macrophages and T cells (CD68 p = .36, CD163 p = .26, CD4 p = .11, CD8 p = .17, and FoxP3 p = .34). We found that Siglec-15 expression was negatively related to B cells and DCs, which is consistent with the results from TCGA. However, Siglec-15 expression was not associated with macrophages and Tregs, which showed positive association with Siglec-15 in TCGA (Supplementary Figure S6d). Then, we further assessed the expression of these genes by RT-qPCR. Siglec-15 was positively associated with CD68 (r = 0.37, p = .11), CD163 (r = 0.50, p = .02), and FoxP3 (r = 0.58, p = .01) in 20 LUAD tissues.
Discussion
Tumor immunotherapy has greatly changed the paradigm in the management of tumor patients. Immune-checkpoint blockade therapy has improved survival in patients with advanced melanoma, non-small-cell lung cancer (NSCLC), and other cancers.Citation40 However, the anti-tumor effect of a single-agent PD-1 inhibitor is limited, with an effective response rate of less than 30%.Citation3,Citation29,Citation41,Citation42 Chen et al. discovered a close relationship between Siglec-15 and the B7 family by protein sequence analysis.Citation26 Although several binding partners on tumor cells have been identified for Siglec-15,Citation43–Citation45 the ligand on T cells for Siglec-15 is unclear. The role of suppressing T cells by Siglec-15 remains to be further investigated.
Glycosylation pattern modification on tumor cells and bacteria to escape immunity has been known for decades;Citation46 however, little progress has been made in treatment strategies targeting glycosylation. Sialylation is one of the most common types of modifications in glycosylation. Siglecs expressed on tumor or immune cells bind to sialoglycans and play a differential immunoregulatory role.Citation47 In recent years, several studies have suggested that Siglecs are responsible for immune cell activation, proliferation, and apoptosis. Meanwhile, Siglecs have also been implicated in immune tolerance regulation and may play an essential role in autoimmune and autoinflammatory diseases and tumorigenesis.Citation16 Therefore, the Siglecs family of proteins have received increasing attention in tumor immunity.Citation14–Citation17,Citation26 An increasing number of Siglecs- or sialoglycan-targeted therapeutic drugs have been developed and may represent a potential novel immune-checkpoint. Targeting Siglec-15 may be an effective alternative therapy for patients that do not respond to PD-1/PD-L1 antibodies.
Genetic and epigenetic changes play a critical role in regulating cancer development and immune tolerance. For example, mutant PD-L1 with structural variations leads to aberrant PD-L1 expression and immunosuppression.Citation48,Citation49 JAK2/PD-L1/PD-L2 (9p24.1) amplifications can also bring about constitutive overexpression of PD-L1 and a significant response to checkpoint inhibitors.Citation50–Citation52 Different mechanisms for regulating PD-L1 expression may reflect the varied roles on different cellular localization and cell types. Preliminary analysis suggested that Siglec-15 expression was genetically and epigenetically regulated through CNA and promoter methylation. In addition to a role in immune regulation, GSEA revealed that Siglec-15 was associated with oncogenic pathways (e.g. MAPK, PI3 K-Akt, Hippo, p53, and apoptosis). Whether Siglec-15 works as an oncogene and the exact mechanisms which regulated Siglec-15 remains to be elucidated.
Many of the genes are found to play different roles through different mechanisms in different cancers.Citation53–Citation56 Similarly, Siglec-15 has variable prognostic significance in diverse cancers, and even in different subtypes of the same cancer. It is well accepted that tumor heterogeneity is a major challenge in cancer treatment.Citation57–Citation59 Siglec-15 may play a role in inhibiting tumorigenesis and development in “cold tumors” like BRCA-luminal A/B. In “hot tumors” like BRCA-basal, Siglec-15 appears to exert an immunosuppressive function by regulating immune-related pathways. In some small sample phase II studies and retrospective studies, the single-agent efficacy of PD-1 or ipilimumab only helped individual patients with certain subtypes achieve tumor remission.Citation60 Siglec-15 overexpression in immune desert tumor, SARC, was involved in immune-related pathways and predicted poor prognosis. If the immune microenvironment can be converted from “cold” to “hot,” anti-Siglec-15 monoclonal antibody may play a more critical role than PD-1/PD-L1 in SARC. In addition, Siglec-15 expression was the highest in the C6 (TGF-β dominant) immune subtype in LUAD, which has a worse prognosis among the six immune subtypes with the highest TGF-β signature.Citation39 It is worth noting that Siglec-15 associated pathways and functions in BLCA were totally different from other cancers. These phenomena may be attributed to inter-/intra-tumor heterogeneity and unique immunophenotyping, which presents new challenges for Siglec-15 studies.
Siglecs on macrophages and DCs could modulate toll-like receptor-induced cytokine responses.Citation45,Citation61,Citation62The pathway analysis also suggested that Siglec15 was related to activation of the chemokine signaling pathway. CXC chemokine receptor CXCR3 is mainly expressed in Treg, B cells, and tumor cells.Citation63,Citation64 There is an increasing trend for CXCR3 and MMP9 in patients with overexpressed Siglec-15, which may recruit Treg.Citation65 Our data analysis demonstrated that Siglec-15 was significantly associated with Tregs infiltration in cancers from TCGA and positively associated with FoxP3 in LUAD tissues by RT-qPCR. This may result in a specific TreghighSiglec15high TME. These results indicate that Siglec-15 may exist in an “immune-excluded” environment, which is consistent with more Tregs and higher TGF-β signaling.Citation66 Therefore, application of anti-Siglec-15 antibody after other therapeutic interventions may hold promise.
There were some differences between the public data analysis and experimental verification by IHC and RT-qPCR. This phenomenon may be due to that we currently focused on Siglec-15 expression on the membrane of tumor cells and used tissue microarray in the assessment of the complex TME. Firstly, Siglec-15 is primarily expressed on macrophage and tumor cells. We cannot exclude the simultaneous expression Siglec-15 in the membrane and cytoplasm. Differences in Siglec-15 expression in macrophage and tumor cells remain unclear. Secondly, TME is widely known for its complexity and variability caused by spatial and temporal heterogeneity.Citation57 In addition to the number of immune cells, the distribution of immune cells in the central core, tumor stroma, and peripheral areasCitation57 contributes to different immune infiltration patterns in the same tissue. Thirdly, the number of patients in our validation cohort was limited to 103 patients. Recurrent patients received different treatment options, which may explain this phenomenon. In one word, more samples and more detailed analysis in specific subgroups are needed to conduct a more accurate and robust evaluation. In addition, our results are based on NGS analysis, which are derived from bulk cells and limited our analysis on accurate differentiation of immune cells and tumor cells. Therefore, single-cell RNA sequencing of sorted immune cells are needed to provide more exact information. Although the experimental results in LUAD samples were generally consistent with data analysis in TCGA and GEO, additional experiments are required to confirm the significance of Siglec-15 in other cancers.
In summary, we investigated Siglec-15 mRNA expression characteristics, prognostic value landscape, relationship with tumor-infiltrating immune cells, and associated pathways in various cancers from the perspective of multi-omic bioinformatics (). We further verified that Siglec-15 expression was associated with poor prognosis and an inhibitory microenvironment in patients with LUAD. These results validated the importance of Siglec-15 expression in cancer prognosis and treatment and identified significant areas for further exploration and confirmation.
Figure 7. Analysis explanation with a detailed flow chart of this study. The study comprises 4 parts: Ⅰ Siglec-15 mRNA expression characteristics investigated by TCGA, GTEx, and Ocomine; Ⅱ Siglec-15 prognostic value landscape in TCGA and GEO; Щ Tumor infiltrating pattern and associated pathways about Siglec-15 in TCGA and GEO; Ⅳ Experiment verification in LUAD patients.
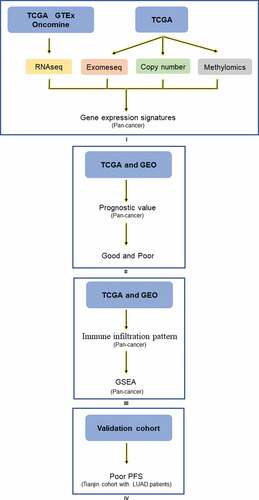
Declaration of interest statement
The authors declare no potential conflicts of interest.
Supplemental Material
Download ()Acknowledgments
We would like to acknowledge tumor tissue banking facility of the Tianjin Medical University Cancer Institute and Hospital for providing us tissues from patients with LUAD.
Supplementary material
Supplemental data for this article can be accessed on the publisher’s website.
Additional information
Funding
References
- Sharma P, Hu-Lieskovan S, Wargo JA, Ribas A. Primary, adaptive, and acquired resistance to cancer immunotherapy. Cell. 2017;168(4):707–11. doi:10.1016/j.cell.2017.01.017.
- Tang J, Yu JX, Hubbard-Lucey VM, Neftelinov ST, Hodge JP, Lin Y. The clinical trial landscape for PD1/PDL1 immune checkpoint inhibitors. Nat Rev Drug Discov. 2018;17(12):854–855. doi:10.1038/nrd.2018.210.
- Gettinger S, Horn L, Jackman D, Spigel D, Antonia S, Hellmann M, Powderly J, Heist R, Sequist LV, Smith DC, et al. Five-year follow-up of nivolumab in previously treated advanced non-small-cell lung cancer: results from the CA209-003 study. J Clin Oncol. 2018;36(17):1675–1684. doi:10.1200/JCO.2017.77.0412.
- Park JH, Rivière I, Gonen M, Wang X, Sénéchal B, Curran KJ, Sauter C, Wang Y, Santomasso B, Mead E, et al. Long-term follow-up of CD19 CAR therapy in acute lymphoblastic leukemia. N Engl J Med. 2018;378(5):449–459. doi:10.1056/NEJMoa1709919.
- Tanyi JL, Bobisse S, Ophir E, Tuyaerts S, Roberti A, Genolet R, Baumgartner P, Stevenson BJ, Iseli C, Dangaj D, et al. Personalized cancer vaccine effectively mobilizes antitumor T cell immunity in ovarian cancer. Sci Transl Med. 2018;10(436):eaao5931. doi:10.1126/scitranslmed.aao5931.
- Horn L, Mansfield AS, Szczęsna A, Havel L, Krzakowski M, Hochmair MJ, Huemer F, Losonczy G, Johnson ML, Nishio M, et al. First-line atezolizumab plus chemotherapy in extensive-stage small-cell lung cancer. N Engl J Med. 2018;379(23):2220–2229. doi:10.1056/NEJMoa1809064.
- Gobbini E, Ezzalfani M, Dieras V, Bachelot T, Brain E, Debled M, Jacot W, Mouret-Reynier MA, Goncalves A, Dalenc F, et al. Time trends of overall survival among metastatic breast cancer patients in the real-life ESME cohort. European Journal of Cancer. 2018;96:17–24. doi:10.1016/j.ejca.2018.03.015.
- Lommatzsch M, Bratke K, Stoll P. Neoadjuvant PD-1 blockade in resectable lung cancer. N Engl J Med. 2018;379:e14. doi:10.1056/NEJMc1808251.
- Jia L, Zhang Q, Zhang RX. PD-1/PD-L1 pathway blockade works as an effective and practical therapy for cancer immunotherapy. Cancer Biol Med. 2018;15(2):116–123. doi:10.20892/j..2095-3941.2017.0086.
- Zou W, Wolchok JD, Chen L. PD-L1 (B7-H1) and PD-1 pathway blockade for cancer therapy: mechanisms, response biomarkers, and combinations. Sci Transl Med. 2016;8(328):328rv324. doi:10.1126/scitranslmed.aad7118.
- Zhang Y, Chen L. Classification of advanced human cancers based on tumor immunity in the microenvironment (TIME) for cancer immunotherapy. JAMA Oncol. 2016;2(11):1403–1404. doi:10.1001/jamaoncol.2016.2450.
- Radvanyi L, Pilon-Thomas S, Peng W, Sarnaik A, Mule JJ, Weber J, Hwu P. Antagonist antibodies to PD-1 and B7-H1 (PD-L1) in the treatment of advanced human cancer–letter. Clin Cancer Res. 2013;19(19):5541. doi:10.1158/1078-0432.CCR-13-1054.
- Macauley MS, Crocker PR, Paulson JC. Siglec-mediated regulation of immune cell function in disease. Nat Rev Immunol. 2014;14(10):653–666. doi:10.1038/nri3737.
- Barkal AA, Brewer RE, Markovic M, Kowarsky M, Barkal SA, Zaro BW, Krishnan V, Hatakeyama J, Dorigo O, Barkal LJ, et al. CD24 signalling through macrophage Siglec-10 is a target for cancer immunotherapy. Nature. 2019;572(7769):392–396. doi:10.1038/s41586-019-1456-0.
- Stanczak MA, Siddiqui SS, Trefny MP, Thommen DS, Boligan KF, von Gunten S, Tzankov A, Tietze L, Lardinois D, Heinzelmann-Schwarz V, et al. Self-associated molecular patterns mediate cancer immune evasion by engaging Siglecs on T cells. J Clin Invest. 2018;128(11):4912–4923. doi:10.1172/JCI120612.
- van de Wall S, Santegoets KCM, van Houtum EJH, Bull C, Adema GJ. Sialoglycans and siglecs can shape the tumor immune microenvironment. Trends Immunol. 2020;41(4):274–285. doi:10.1016/j.it.2020.02.001.
- Jandus C, Boligan KF, Chijioke O, Liu H, Dahlhaus M, Démoulins T, Schneider C, Wehrli M, Hunger RE, Baerlocher GM, et al. Interactions between Siglec-7/9 receptors and ligands influence NK cell-dependent tumor immunosurveillance. J Clin Invest. 2014;124(4):1810–1820. doi:10.1172/JCI65899.
- Hudak JE, Canham SM, Bertozzi CR. Glycocalyx engineering reveals a Siglec-based mechanism for NK cell immunoevasion. Nat Chem Biol. 2014;10(1):69–75. doi:10.1038/nchembio.1388.
- Angata T, Tabuchi Y, Nakamura K, Nakamura M. Siglec-15: an immune system Siglec conserved throughout vertebrate evolution. Glycobiology. 2007;17(8):838–846. doi:10.1093/glycob/cwm049.
- Hiruma Y, Hirai T, Tsuda E. Siglec-15, a member of the sialic acid-binding lectin, is a novel regulator for osteoclast differentiation. Biochem Biophys Res Commun. 2011;409(3):424–429. doi:10.1016/j.bbrc.2011.05.015.
- Ishida-Kitagawa N, Tanaka K, Bao X, Kimura T, Miura T, Kitaoka Y, Hayashi K, Sato M, Maruoka M, Ogawa T, et al. Siglec-15 protein regulates formation of functional osteoclasts in concert with DNAX-activating protein of 12 kDa (DAP12). J Biol Chem. 2012;287(21):17493–17502. doi:10.1074/jbc.M111.324194.
- Stuible M, Moraitis A, Fortin A, Saragosa S, Kalbakji A, Filion M, Tremblay GB. Mechanism and function of monoclonal antibodies targeting siglec-15 for therapeutic inhibition of osteoclastic bone resorption. J Biol Chem. 2014;289(10):6498–6512. doi:10.1074/jbc.M113.494542.
- Hiruma Y, Tsuda E, Maeda N, Okada A, Kabasawa N, Miyamoto M, Hattori H, Fukuda C. Impaired osteoclast differentiation and function and mild osteopetrosis development in Siglec-15-deficient mice. Bone. 2013;53(1):87–93. doi:10.1016/j.bone.2012.11.036.
- Kameda Y, Takahata M, Komatsu M, Mikuni S, Hatakeyama S, Shimizu T, Angata T, Kinjo M, Minami A, Iwasaki N, et al. Siglec-15 regulates osteoclast differentiation by modulating RANKL-induced phosphatidylinositol 3-kinase/Akt and Erk pathways in association with signaling Adaptor DAP12. J Bone Miner Res. 2013;28(12):2463–2475. doi:10.1002/jbmr.1989.
- Takamiya R, Ohtsubo K, Takamatsu S, Taniguchi N, Angata T. The interaction between Siglec-15 and tumor-associated sialyl-Tn antigen enhances TGF-beta secretion from monocytes/macrophages through the DAP12-Syk pathway. Glycobiology. 2013;23(2):178–187. doi:10.1093/glycob/cws139.
- Wang J, Sun J, Liu LN, Flies DB, Nie X, Toki M, Zhang J, Song C, Zarr M, Zhou X, et al. Siglec-15 as an immune suppressor and potential target for normalization cancer immunotherapy. Nat Med. 2019;25(4):656–666. doi:10.1038/s41591-019-0374-x.
- Xiubao R. Immunosuppressive checkpoint Siglec-15: a vital new piece of the cancer immunotherapy jigsaw puzzle. Cancer Biology & Medicine. 2019;16(2):205. doi:10.20892/j..2095-3941.2018.0141.
- Cao G, Xiao Z, Yin Z. Normalization cancer immunotherapy: blocking Siglec-15! Signal Transduct Target Ther. 2019;4(1):10. doi:10.1038/s41392-019-0045-x.
- Poh A. Siglec-15: an attractive immunotherapy target. Cancer Discov. 2020;10:7–8. doi:10.1158/2159-8290.CD-NB2019-136.
- Deng M, Bragelmann J, Schultze JL, Perner S. Web-TCGA: an online platform for integrated analysis of molecular cancer data sets. BMC Bioinformatics. 2016;17(1):72. doi:10.1186/s12859-016-0917-9.
- Wei L, Jin Z, Yang S, Xu Y, Zhu Y, Ji Y. TCGA-assembler 2: software pipeline for retrieval and processing of TCGA/CPTAC data. Bioinformatics. 2018;34(9):1615–1617. doi:10.1093/bioinformatics/btx812.
- Cerami E, Gao J, Dogrusoz U, Gross BE, Sumer SO, Aksoy BA, Jacobsen A, Byrne CJ, Heuer ML, Larsson E, et al. The cBio cancer genomics portal: an open platform for exploring multidimensional cancer genomics data: figure 1. Cancer Discov. 2012;2(5):401–404. doi:10.1158/2159-8290.CD-12-0095.
- Gao J, Aksoy BA, Dogrusoz U, Dresdner G, Gross B, Sumer SO, Sun Y, Jacobsen A, Sinha R, Larsson E, et al. Integrative analysis of complex cancer genomics and clinical profiles using the cBioPortal. Sci Signal. 2013;6(269):pl1. doi:10.1126/scisignal.2004088.
- Chandrashekar DS, Bashel B, Balasubramanya SAH, Creighton CJ, Ponce-Rodriguez I, Chakravarthi BVSK, Varambally S. UALCAN: A portal for facilitating tumor subgroup gene expression and survival analyses. Neoplasia. 2017;19(8):649–658. doi:10.1016/j.neo.2017.05.002.
- Nagy A, Lanczky A, Menyhart O, Gyorffy B. Validation of miRNA prognostic power in hepatocellular carcinoma using expression data of independent datasets (vol 8, 9227, 2018). Sci Rep-Uk. 2018:8. doi:10.1038/s41598-018-29514-3.
- Ru B, Wong CN, Tong Y, Zhong JY, Zhong SSW, Wu WC, Chu KC, Wong CY, Lau CY, Chen I, et al. TISIDB: an integrated repository portal for tumor-immune system interactions. Bioinformatics. 2019;35(20):4200–4202. doi:10.1093/bioinformatics/btz210.
- Newman AM, Steen CB, Liu CL, Gentles AJ, Chaudhuri AA, Scherer F, Khodadoust MS, Esfahani MS, Luca BA, Steiner D, et al. Determining cell type abundance and expression from bulk tissues with digital cytometry. Nat Biotechnol. 2019;37(7):773–782. doi:10.1038/s41587-019-0114-2.
- Anastasiadi D, Esteve-Codina A, Piferrer F. Consistent inverse correlation between DNA methylation of the first intron and gene expression across tissues and species. Epigenetics Chromatin. 2018;11(1):37. doi:10.1186/s13072-018-0205-1.
- Thorsson V, Gibbs DL, Brown SD, Wolf D, Bortone DS, Ou Yang T-H, Porta-Pardo E, Gao GF, Plaisier CL, Eddy JA, et al. The Immune Landscape of Cancer. Immunity. 2019;51(2):411–412. doi:10.1016/j.immuni.2019.08.004.
- Cogdill AP, Andrews MC, Wargo JA. Hallmarks of response to immune checkpoint blockade. Br J Cancer. 2017;117(1):1–7. doi:10.1038/bjc.2017.136.
- Pan C, Liu H, Robins E, Song W, Liu D, Li Z, Zheng L. Next-generation immuno-oncology agents: current momentum shifts in cancer immunotherapy. J Hematol Oncol. 2020;13(1):29. doi:10.1186/s13045-020-00862-w.
- Hamid O, Robert C, Daud A, Hodi FS, Hwu WJ, Kefford R, Wolchok JD, Hersey P, Joseph R, Weber JS, et al. Five-year survival outcomes for patients with advanced melanoma treated with pembrolizumab in KEYNOTE-001. Ann Oncol. 2019;30(4):582–588. doi:10.1093/annonc/mdz011.
- Murugesan G, Correia VG, Palma AS, Chai W, Li C, Feizi T, Martin E, Laux B, Franz A, Fuchs K, et al. Siglec-15 recognition of sialoglycans on tumor cell lines can occur independently of sialyl Tn antigen expression. Glycobiology. 2020. doi:10.1093/glycob/cwaa048.
- Chang L, Chen Y-J, Fan C-Y, Tang C-J, Chen Y-H, Low P-Y, Ventura A, Lin -C-C, Chen Y-J, Angata T, et al. Identification of siglec ligands using a proximity labeling method. J Proteome Res. 2017;16(10):3929–3941. doi:10.1021/acs.jproteome.7b00625.
- Briard JG, Jiang H, Moremen KW, Macauley MS, Wu P. Cell-based glycan arrays for probing glycan-glycan binding protein interactions. Nat Commun. 2018;9(1):880. doi:10.1038/s41467-018-03245-5.
- Jandus P, Boligan KF, Smith DF, de Graauw E, Grimbacher B, Jandus C, Abdelhafez MM, Despont A, Bovin N, Simon D, et al. The architecture of the IgG anti-carbohydrate repertoire in primary antibody deficiencies. Blood. 2019;134(22):1941–1950. doi:10.1182/blood.2019001705.
- Demaria O, Cornen S, Daëron M, Morel Y, Medzhitov R, Vivier E. Harnessing innate immunity in cancer therapy. Nature. 2019;574(7776):45–56. doi:10.1038/s41586-019-1593-5.
- Kataoka K, Shiraishi Y, Takeda Y, Sakata S, Matsumoto M, Nagano S, Maeda T, Nagata Y, Kitanaka A, Mizuno S, et al. Aberrant PD-L1 expression through 3 ‘-UTR disruption in multiple cancers. Nature. 2016;534(7607):402. doi:10.1038/nature18294.
- Bellone S, Buza N, Choi J, Zammataro L, Gay L, Elvin J, Rimm DL, Liu Y, Ratner ES, Schwartz PE, et al. Exceptional response to pembrolizumab in a metastatic, chemotherapy/radiation-resistant ovarian cancer patient harboring a PD-L1-genetic rearrangement. Clinical Cancer Research. 2018;24(14):3282–3291. doi:10.1158/1078-0432.Ccr-17-1805.
- Gupta S, Cheville JC, Jungbluth AA, Zhang Y, Zhang L, Chen Y-B, Tickoo SK, Fine SW, Gopalan A, Al-Ahmadie HA, et al. JAK2/PD-L1/PD-L2 (9p24.1) amplifications in renal cell carcinomas with sarcomatoid transformation: implications for clinical management. Modern Pathol. 2019;32(9):1344–1358. doi:10.1038/s41379-019-0269-x.
- Wang YC, Wenzl K, Manske MK, Asmann YW, Sarangi V, Greipp PT, Krull JE, Hartert K, He R, Feldman AL, et al. Amplification of 9p24.1 in diffuse large B-cell lymphoma identifies a unique subset of cases that resemble primary mediastinal large B-cell lymphoma. Blood Cancer J. 2019;9(9). doi:10.1038/s41408-019-0233-5
- Godfrey J, Tumuluru S, Bao R, Leukam M, Venkataraman G, Phillip J, Fitzpatrick C, McElherne J, MacNabb BW, Orlowski R, et al. PD-L1 gene alterations identify a subset of diffuse large B-cell lymphoma harboring a T-cell-inflamed phenotype. Blood. 2019;133(21):2279–2290. doi:10.1182/blood-2018-10-879015.
- Imperato S, Mistretta C, Marone, Marone M, Migliaccio I, Pulcinelli I, Faraone Mennella MR. Automodified Poly(ADP-Ribose) polymerase analysis to monitor DNA damage in peripheral lymphocytes of floriculturists occupationally exposed to pesticides. Cells. 2019;8(2). doi:10.3390/cells8020137.
- Otto JE, Kadoch C. A two-faced mSWI/SNF subunit: dual roles for ARID1A in tumor suppression and oncogenicity in the liver. Cancer Cell. 2017;32(5):542–543. doi:10.1016/j.ccell.2017.10.014.
- Rankin LC, Arpaia N. Treg cells: A LAGging hand holds the double-edged sword of the IL-23 axis. Immunity. 2018;49(2):201–203. doi:10.1016/j.immuni.2018.08.008.
- Zhao S, Zhang MH, Zhang Y, Meng HX, Wang Y, Liu YP, Jing J, Hang L, Sun MQ, Zhang Y, et al. The prognostic value of programmed cell death ligand 1 expression in non-Hodgkin lymphoma: a meta-analysis. Cancer Biol Med. 2018;15(3):290–298. doi:10.20892/j..2095-3941.2018.0047.
- Kondratova M, Czerwinska U, Sompairac N, Amigorena SD, Soumelis V, Barillot E, Zinovyev A, Kuperstein I. A multiscale signalling network map of innate immune response in cancer reveals cell heterogeneity signatures. Nat Commun. 2019;10(1):4808. doi:10.1038/s41467-019-12270-x.
- Gerlinger M, Rowan AJ, Horswell S, Larkin J, Endesfelder D, Gronroos E, Martinez P, Matthews N, Stewart A, Tarpey P, et al. Intratumor heterogeneity and branched evolution revealed by multiregion sequencing. N Engl J Med. 2012;366(10):883–892. doi:10.1056/NEJMoa1113205.
- McDonald K-A, Kawaguchi T, Qi Q, Peng X, Asaoka M, Young J, Opyrchal M, Yan L, Patnaik S, Otsuji E, et al. Tumor heterogeneity correlates with less immune response and worse survival in breast cancer patients. Ann Surg Oncol. 2019;26(7):2191–2199. doi:10.1245/s10434-019-07338-3.
- Pecuchet N, Vieira T, Rabbe N, Antoine M, Blons H, Cadranel J, Laurent-Puig P, Wislez M. Molecular classification of pulmonary sarcomatoid carcinomas suggests new therapeutic opportunities. Ann Oncol. 2017;28(7):1597–1604. doi:10.1093/annonc/mdx162.
- Boyd CR, Orr SJ, Spence S, Burrows JF, Elliott J, Carroll HP, Brennan K, Gabhann JN, Coulter WA, Johnston JA, et al. Siglec-E is up-regulated and phosphorylated following lipopolysaccharide stimulation in order to limit TLR-driven cytokine production. J Immunol. 2009;183(12):7703–7709. doi:10.4049/jimmunol.0902780.
- Miyazaki K, Sakuma K, Kawamura YI, Izawa M, Ohmori K, Mitsuki M, Yamaji T, Hashimoto Y, Suzuki A, Saito Y, et al. Colonic epithelial cells express specific ligands for mucosal macrophage immunosuppressive receptors siglec-7 and −9. J Immunol. 2012;188(9):4690–4700. doi:10.4049/jimmunol.1100605.
- Tokunaga R, Zhang W, Naseem M, Puccini A, Berger MD, Soni S, McSkane M, Baba H, Lenz H-J. CXCL9, CXCL10, CXCL11/CXCR3 axis for immune activation – A target for novel cancer therapy. Cancer Treat Rev. 2018;63:40–47. doi:10.1016/j.ctrv.2017.11.007.
- Pellegrino A, Antonaci F, Russo F, Merchionne F, Ribatti D, Vacca A, Dammacco F. CXCR3-binding chemokines in multiple myeloma. Cancer Lett. 2004;207(2):221–227. doi:10.1016/j.canlet.2003.10.036.
- Wang B-Q, Zhang C-M, Gao W, Wang X-F, Zhang H-L, Yang P-C. Cancer-derived matrix metalloproteinase-9 contributes to tumor tolerance. J Cancer Res Clin Oncol. 2011;137(10):1525–1533. doi:10.1007/s00432-011-1010-4.
- Hegde PS, Chen DS. Top 10 challenges in cancer immunotherapy. Immunity. 2020;52(1):17–35. doi:10.1016/j.immuni.2019.12.011.