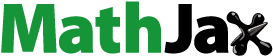
ABSTRACT
The performance limitation of networked control systems (NCSs) with networked delay and two-channel noises constraints are studied in this paper. The networked parameters mainly consider the networked delay in the forward channel and white Gaussian noise constraint existing in forward channel and feedback channel. The tracking performance limitation expression is achieved by using the spectral decomposition technique and selecting the optimal one-parameter structure. The obtained result shows that the tracking performance limitation of NCSs is related to the position of the non-minimum phase zeroes, the position of the unstable poles, networked delay, two-channel noises and input signal. We can also know that the networked delay and white Gaussian noise have influence on the tracking performance limitation of NCSs. Finally, a simulation example is given to demonstrate effectiveness of the theoretical results.
1. Introduction
With the development of internet and computer technology, the traditional control system can't adapt to the needs of daily life. We know that the internet has been inextricably linked to us, so it is a development trend to use the network for traditional control systems. The networked control systems (NCSs) emerge as the times require. And then, it has developed rapidly. Until now it is still an important research issue (Almakhles, Swain, Nasiri, & Patel, Citation2017; Zhang, Shi, Wang, & Yu, Citation2017; Zhang, Yan, Yang, & Chen, Citation2011; Zhang, Han, & Yu, Citation2016). The NCSs have many advantages like: flexibility; low cost; simple installation and maintenance; reduced weight and power requirement, etc. In recent years, much research works have been investigated about the stability analysis of NCSs with communication constraints, e.g. quantization (Fu & Xie, Citation2005; Xiao, Xie, & Fu, Citation2010; Yuan, Yuan, Wang, Guo, & Yang, Citation2017), time delay (Liu, Citation2010; Luan, Shi, & Liu, Citation2011), bandwidth (Rojas, Braslavsky, & Middleton, Citation2008) and packet loss (Chen, Gao, Shi, & Lu, Citation2016; Pang, Liu, Zhou, & Sun, Citation2016) etc. The research progress of NCSs from several aspects was summarized in Mahmoud (Citation2016). The stability of quantitative in feedback control system based on event trigger was considered in Boukens, Boukabou, and Chadli (Citation2017). The sufficient conditions to ensure the stability and dissipativity of the filtering error system by establishing the mode-dependent periodic Lyapunov function was proposed in Lu et al. (Citation2018). The stability analysis of sampling system by a new time-based discontinuous Lyapunov function was studied in Shao, Han, Zhao, and Zhang (Citation2017). In NCSs, the network delay is time-varying or random (Gao, Jiang, & Pan, Citation2018; Su & Chesi, Citation2017; Tao, Wu, Su, Wu, & Zhang, Citation2018; Zhang, Wu, Shi, & Zhao, Citation2015). A new Lyapunov function and stability results are dependent on both the data packet dropouts and the time delay was proposed in Tao, Lu, Wu, and Wu (Citation2017). The stability of time delay of NCSs was studied in Liu, Zhang, and Xie (Citation2017). The problem of output feedback delay compensation controller for NCSs with random network delay was discussed in Zhang, Lam, and Xia (Citation2014). But sometimes, the authors study the determined time delay to reduce the difficult of a problem. The problem of stability for switched positive linear systems with constant time delay was studied in Zhao, Zhang, and Shi (Citation2013). The authors focused on the problems of robust stabilization and robust control for linear systems with a constant time delay in De Souza and Xi (Citation1999).
In nowadays research, more and more researchers are interested in the performance study of the control system in the control community, see Wu, Zhan, Zhang, Jiang, and Han (Citation2017); Wu, Zhou, Zhan, Yan, and Ge (Citation2017); Zhan, Guan, Zhang, and Yuan (Citation2013); Zhan, Wu, Jiang, and Jiang (Citation2015) for details. The optimal tracking performance of single-input single-output (SISO) NCSs with bandwidth and network-induced delay constraints was studied in Zhan, Guan, Zhang, and Yuan (Citation2014). The relationship between bandwidth constraint and optimal modified tracking performance of MIMO NCSs was studied in Sun, Wu, Zhan, and Han (Citation2016). The authors Guan, Chen, Feng, and Li (Citation2013) investigated the tracking performance limitation for MIMO LTI systems with coloured channel noise and limited bandwidth channel. The above results only consider one-way channel constraint. However, the networked delay often appears, the time delay can result in the performance degradation of control systems and even worse cause a system to become unstable. The communication channel constraint both in forward and feedback channels often exist, the tracking performance limitation of NCSs with two-way channel constraints is more difficult to study. It is necessary and important to study the performance limitation of the NCSs with networked delay and two-channel noises constraints.
The main contributions of this paper are as follows. This paper introduces a model for the NCSs, the networked delay exists in the forward channel, and the noise exists in the forward channel and feedback channel, simultaneously. In this system, the relationships between the networked delay and white noise constraint existing in forward and feedback channels is investigated. An expression for the tracking performance limitation is then achieved by using the co-prime factorization and the spectral decomposition technique. The achieved results will reveal that the tracking performance limitation of the NCSs is affected by the non-minimum phase zeroes, unstable poles and time delay, white noise and the reference input signal. The results provide a good guidance for the design of the NCSs.
2. Problem statement and preliminaries
To discuss the tracking performance limitation of NCSs with networked delay and two-channel noises constraints, we consider the NCSs model as Figure . In Figure , the signal r is a random reference signal, the variance of r is . K denotes the one-parameter compensator. G denotes the plant model, τ is the networked delay, the signal y is the systems output and
,
are white Gaussian noise and variance are
and
. For a given plant, a tracking error of the NCSs is defined as
.
According to Figure , we have
(1)
(1) Then
(2)
(2) The tracking error is obtained by
(3)
(3) where
(4)
(4) It is clearly that the rational transfer function G can be given by:
(5)
(5) where,
, and satisfied the Bezout identity
(6)
(6) where,
. All the compensators which can make the system become stable can be characterized by Youl parameterization
(7)
(7) It is well known that a non-minimum phase transfer function could factorize a minimum phase part and an all-pass factor
(8)
(8) where
and
are the minimum phase part
and
are the all-pass factor
includes all non-minimum phase zeros
,
includes all the unstable poles of the given plant
,
and
can be represented as
(9)
(9) The tracking performance of NCSs is defined as
(10)
(10)
3. Performance limitations of the NCSs with the networked delay and two-channel noises
The tracking performance limitation of NCSs is measured by the possible minimal tracking error achievable by all possible linear stabilizing controllers (denote by ), determined as
(11)
(11) Assuming the input signal has no connection with white Gaussian noise
,
, then the tracking performance can be shown as
(12)
(12) According to (Equation3
(3)
(3) ), (Equation4
(4)
(4) ), (Equation5
(5)
(5) ), (Equation11
(11)
(11) ) and (Equation12
(12)
(12) ), we can obtain
(13)
(13)
Theorem 3.1
Assume that a given system has many unstable poles and NMP zeros
with the networked delay and two-channel noises constraints, the tracking performance limitation of the NCSs be shown as
where
.
Proof.
According to (Equation7(7)
(7) ) and (Equation13
(13)
(13) ), we can obtain
(14)
(14) According to (Equation8
(8)
(8) ) and (Equation14
(14)
(14) ), we can obtain
(15)
(15) According to (Equation6
(6)
(6) ), we can obtain
(16)
(16) Then we define
(17)
(17) According to (Equation15
(15)
(15) ) and (Equation17
(17)
(17) ), we can obtain
(18)
(18) Because
,
, we can obtain
(19)
(19) Because of the
and
are the all-pass factors, it follows that
(20)
(20) Based on a partial fraction procedure, we can obtain
where
According to (Equation9(9)
(9) ), we can obtain
, then
(21)
(21) Then
Therefore, we have
(22)
(22) Then
(23)
(23) Because of
and
are the minimum phase part, we can choose a suitable Q to derive
Then we can have
(24)
(24) According to Toker, Chen, and Qiu (Citation2002)
(25)
(25) Then
where
.
This completes the proof.
4. Illustrative examples
In this section, the obtained result is illustrated by a classical example. The transfer function of the given plant is
For k>0, we may see that NMP zeros is located at
, the unstable pole is located at
. Supposing the networked delay is
, the input signal is
, k=1, according to Theorem 3.1, we have
The tracking performances limitation of the NCSs with different two-channel noises are shown in Figure . In Figure , the two-channel noises affect the tracking performance limitation of the NCSs, when the two-channel noises become big, the tracking performances limitation of NCSs become bad.
The influence of the networked delay is also existing in the NCSs. Thus, the simulation about the influence of the networked delay to the NCSs is necessary. In Zhan, Guan, and WU (Citation2010), the performance limitation of networked control systems with networked induce-delay is discussed. In this paper we consider about the NMP zeros, the unstable poles and the input signal. The choice of date is the same in previous example, the networked delay is ,
,
and
, according to the Theorem, we can obtain
According to Zhan et al. (Citation2010), we can obtain
The tracking performance limitation of the NCSs with different NMP zeros is shown in Figure . Figure reveals that the tracking performance limitation of the NCSs is based on the NMP zeros and unstable poles of the given plant. When the NMP zeros move closer to the unstable poles, the tracking performance limitation of the NCSs tends to the infinity. By comparing
and
, we can know that the noise exists in the communication channel will make the performance limitation of NCSs become worse.
According to the research before, there are a few articles talk about noise in both forward and feedback channel, so it is valuable to talk about the difference of tracking performance limitation by the effect of noise in forward and feedback channel. Assuming that the networked delay is , the input signal is
, k=1, according to Theorem,
Supposing there is no noise in the forward channel, ,
Supposing there is no noise in the feedback channel, ,
When the noise in the forward channel, ,
When the noise in the feedback channel, ,
Figure shows the tracking performance limitation of the NCSs is effect by the noise, no matter the noise appears in the forward channel or in the feedback channel. we can know the degree of impact of noise in the forward channel or in the feedback channel are different. Also, Figure indicates the tracking performance limitation is more affected by the noise in the forward channel. Form Figure , we can find the tracking performance limitation will intersect in somewhere (defined as P), before point P, noise in the forward channel has a greater impact on the tracking performance limitation of the NCSs; after point P, noise in the feedback channel has a greater impact on the tracking performance limitation of the NCSs.
5. Conclusion
In this paper, we explore the tracking performance limitation of NCSs with the two-channel noises. The proposed model takes into consideration the channel noise in the feedback channel and the networked delay in the forward path. The analytical expression of the tracking performance limitation is derived by using the method of -norm and spectral factorizations. The obtained results show that the tracking performance limitation mainly depends on the position of the non-minimum phase zeros, position of the unstable poles, networked delay and two-channel noises. An illustrative example is analysed to demonstrate the effectiveness of the proposed method.
Possible future extensions to this work include study of more general plants such as multiple-input multiple-output nonlinear NCSs, more complex channel models such as the fading channel, and more parameters of communication channel constraints such as quantization effect, bandwidth, time-varying delay and signal-to-noise ratio.
Disclosure statement
No potential conflict of interest was reported by the authors.
Additional information
Funding
References
- Almakhles, D., Swain, A. K., Nasiri, A., & Patel, N. (2017). An adaptive two-level quantizer for networked control systems. IEEE Transactions on Control Systems Technology, 25(3), 1084–1091. doi: 10.1109/TCST.2016.2574768
- Boukens, M., Boukabou, A., & Chadli, M. (2017). Robust adaptive neural network-based trajectory tracking control approach for nonholonomic electrically driven mobile robots. Robotics and Autonomous Systems, 92, 30–40. doi: 10.1016/j.robot.2017.03.001
- Chen, H., Gao, J., Shi, T., & Lu, R. (2016). H-∞ control for networked control systems with time delay, data packet dropout and disorder. Neurocomputing, 179, 211–218. doi: 10.1016/j.neucom.2015.11.092
- De Souza, C. E., & Xi, L. (1999). Delay-dependent robust H-∞ control of uncertain linear state-delayed systems. Automatica, 35(7), 1313–1321. doi: 10.1016/S0005-1098(99)00025-4
- Fu, M., & Xie, L. (2005). The sector bound approach to quantized feedback control. IEEE Transactions on Automatic Control, 50(11), 1698–1711. doi: 10.1109/TAC.2005.858689
- Gao, M., Jiang, S., & Pan, F. (2018). Fault detection for time-delayed networked control systems with sensor saturation and randomly occurring faults. International Journal of Innovative Computing Information and Control, 14(4), 1511–1525.
- Guan, Z. H., Chen, C. Y., Feng, G., & Li, T. (2013). Optimal tracking performance limitation of networked control systems with limited bandwidth and additive colored white gaussian noise. IEEE Transactions on Circuits and Systems I: Regular Papers, 60(1), 189–198. doi: 10.1109/TCSI.2012.2215717
- Liu, G. P. (2010). Predictive controller design of networked systems with communication delays and data loss. IEEE Transactions on Circuits and Systems II: Express Briefs, 57(6), 481–485. doi: 10.1109/TCSII.2010.2048377
- Liu, X., Zhang, K., & Xie, W. C. (2017). Consensus seeking in multi-agent systems via hybrid protocols with impulse delays. Nonlinear Analysis: Hybrid Systems, 25, 90–98.
- Lu, R., Tao, J., Shi, P., Su, H., Wu, Z. G., & Xu, Y. (2018). Dissipativity-based resilient filtering of periodic Markovian jump neural networks with quantized measurements. IEEE Transactions on Neural Networks and Learning Systems, 29(5), 1888–1899. doi: 10.1109/TNNLS.2017.2688582
- Luan, X. L., Shi, P., & Liu, F. (2011). Stabilization of networked control systems with random delays. IEEE Transactions on Industrial Electronics, 58(9), 4323–4330. doi: 10.1109/TIE.2010.2102322
- Mahmoud, M. S. (2016). Networked control systems analysis and design: An overview. Arabian Journal for Science and Engineering, 41(3), 711–758. doi: 10.1007/s13369-015-2024-z
- Pang, Z. H., Liu, G. P., Zhou, D., & Sun, D. (2016). Data-based predictive control for networked nonlinear systems with network-induced delay and packet dropout. IEEE Transactions on Industrial Electronics, 63(2), 1249–1257. doi: 10.1109/TIE.2015.2497206
- Rojas, A. J., Braslavsky, J. H., & Middleton, R. H. (2008). Fundamental limitations in control over a communication channel. Automatica, 44(12), 3147–3151. doi: 10.1016/j.automatica.2008.05.014
- Shao, H., Han, Q. L., Zhao, J., & Zhang, D. (2017). A separation method of transmission delays and data packet dropouts from a lumped input delay in the stability problem of networked control systems. International Journal of Robust and Nonlinear Control, 27(11), 1963–1973. doi: 10.1002/rnc.3647
- Su, L., & Chesi, G. (2017). Robust stability analysis and synthesis for uncertain discrete-time networked control systems over fading channels. IEEE Transactions on Automatic Control, 62(4), 1966–1971. doi: 10.1109/TAC.2016.2585124
- Sun, X. X., Wu, J., Zhan, X. S., & Han, T. (2016). Optimal modified tracking performance for MIMO systems under bandwidth constraint. ISA Transactions, 62(6), 145–153. doi: 10.1016/j.isatra.2016.01.018
- Tao, J., Lu, R., Wu, Z. G., & Wu, Y. (2017). Reliable control against sensor failures for Markov jump systems with unideal measurements. IEEE Transactions on Systems, Man, and Cybernetics: Systems. Advance online publication. doi:10.1109/TSMC.2778298
- Tao, J., Wu, Z. G., Su, H., Wu, Y., & Zhang, D. (2018). Asynchronous and resilient filtering for Markovian jump neural networks subject to extended dissipativity. IEEE Transactions on Cybernetics, Advance online publication. doi:10.1109/TCYB.2824853.
- Toker, O., Chen, J., & Qiu, L. (2002). Tracking performance limitations in LTI multivariable discrete-time systems. IEEE Transactions on Circuits and Systems I: Fundamental Theory and Applications, 49(5), 657–670. doi: 10.1109/TCSI.2002.1001955
- Wu, J., Zhan, X. S., Zhang, X. H., Jiang, T., & Han, T. (2017). Optimal tracking performance of networked control systems with communication delays under channel input power constraint. Transactions of the Institute of Measurement and Control, 39(9), 1346–1354. doi: 10.1177/0142331216634831
- Wu, J., Zhou, Z. J., Zhan, X. S., Yan, H. C., & Ge, M. F. (2017). Optimal modified tracking performance for MIMO networked control systems with communication constraints. ISA Transactions, 68, 14–21. doi: 10.1016/j.isatra.2017.01.028
- Xiao, N., Xie, L., & Fu, M. (2010). Stabilization of Markov jump linear systems using quantized state feedback. Automatica, 46(10), 1696–1702. doi: 10.1016/j.automatica.2010.06.018
- Yuan, Y., Yuan, H., Wang, Z., Guo, L., & Yang, H. (2017). Optimal control for networked control systems with disturbances: A delta operator approach. IET Control Theory and Applications, 11(9), 1325–1332. doi: 10.1049/iet-cta.2016.1279
- Zhan, X. S., Guan, Z. H., & WU, B. (2010). Optimal tracking performance of networked control systems. Journal of Huazhong University of Science and Technology, 38(12), 48–51.
- Zhan, X. S., Guan, Z. H., Zhang, X. H., & Yuan, F. S. (2013). Optimal tracking performance and design of networked control systems with packet dropouts. Journal of the Franklin Institute, 350(10), 3205–3216. doi: 10.1016/j.jfranklin.2013.06.019
- Zhan, X. S., Guan, Z. H., Zhang, X. H., & Yuan, F. S. (2014). Best tracking performance of networked control systems based on communication constraints. Asian Journal of Control, 16(4), 1155–1163. doi: 10.1002/asjc.789
- Zhan, X. S., Wu, J., Jiang, T., & Jiang, X. W. (2015). Optimal performance of networked control systems under the packet dropouts and channel noise. ISA Transactions, 58(5), 214–221. doi: 10.1016/j.isatra.2015.05.012
- Zhang, X. M., Han, Q. L., & Yu, X. (2016). Survey on recent advances in networked control systems. IEEE Transactions on Industrial Informatics, 12(3), 1740–1752. doi: 10.1109/TII.2015.2506545
- Zhang, J., Lam, J., & Xia, Y. (2014). Output feedback delay compensation control for networked control systems with random delays. Information Sciences, 265, 154–166. doi: 10.1016/j.ins.2013.12.021
- Zhang, D., Shi, P., Wang, Q. G., & Yu, L. (2017). Analysis and synthesis of networked control systems: A survey of recent advances and challenges. ISA Transactions, 66, 376–392. doi: 10.1016/j.isatra.2016.09.026
- Zhang, H., Wu, L., Shi, P., & Zhao, Y. (2015). Balanced truncation approach to model reduction of Markovian jump time-varying delay systems. Journal of the Franklin Institute, 352(10), 4205–4224. doi: 10.1016/j.jfranklin.2015.05.041
- Zhang, H., Yan, H., Yang, F., & Chen, Q. (2011). Quantized control design for impulsive fuzzy networked systems. IEEE Transactions on Fuzzy Systems, 19(6), 1153–1162. doi: 10.1109/TFUZZ.2011.2162525
- Zhao, X., Zhang, L., & Shi, P. (2013). Stability of a class of switched positive linear time-delay systems. International Journal of Robust and Nonlinear Control, 23(5), 578–589. doi: 10.1002/rnc.2777