ABSTRACT
Background
Behavior change interventions have a vital role in enhancing human health and well-being. Nevertheless, concerns have been raised about suboptimal reporting of behavior change interventions, making analyses, replications, and intervention re-use hard or impossible to conduct.
Objective
This paper introduces acyclic behavior change diagrams (ABCDs) to achieve more transparent development, evaluation, and reporting of behavior change interventions. ABCDs are a visual representation of the assumptions regarding causal-structural chains that underlie putative active ingredients of behavior change interventions. These causal-structural chains link the behavior change principles that are applied in an intervention to the (determinants of) behavior targeted in that intervention.
Conclusions
ABCDs are helpful in making implicit assumptions explicit and help communicate assumptions with team members and other stakeholders. Moreover, we believe they make evaluation easier, and their machine-readability allows for ABCDs to be imported directly into (systematic review) databases with negligible costs while disclosing complete and accurate data. Finally, the ABCD approach fits well with other initiatives to gain a deeper understanding and synthesis of the literature on active intervention elements.
The overall majority of deaths worldwide are caused by human behaviors such as smoking and unhealthy food intake (Ritchie & Roser, Citation2019). These behaviors are a function of the people enacting them and their environments (Lewin, Citation1936). Considering all of these factors in the context of behavior change inevitably leads to complex interventions (Kelly & Barker, Citation2016). Unfortunately, there are no 6 (Cialdini, Citation2008) or 93 (Michie et al., Citation2013) ways to change behavior directly – rather, interventions operate on parts of the human psyche (i.e. psychological constructs) that are conceptualized as determinants of behavior change (Crutzen & Peters, Citation2018). Interventions usually target multiple determinants with multiple components and media, as well as agents at multiple socio-ecological levels (Craig et al., Citation2008).
Given this inevitable complexity, great responsibility for behavior change lies on researchers and practitioners to comprehensively document the assumptions and decisions underlying behavior change interventions. Unfortunately, concerns have been raised about suboptimal reporting of these complex multi-component behavior change interventions, making analyses, replications or re-use of interventions hard or even impossible (Albrecht et al., Citation2013; De Bruin et al., Citation2021; Kok & Mesters, Citation2011; Schaalma & Kok, Citation2009). One example is a systematic analysis and meta-analysis on reporting quality of behavior change techniques (BCTs) for experimental and comparator groups in randomized trials of smoking cessation interventions (Black et al., Citation2020; De Bruin et al., Citation2021). After labor intensive manual coding, it was shown that 65% of intervention BCTs and 74% of comparator BCTs were not reported.
The recommendations made by the Workgroup for Intervention Development and Evaluation Research (WIDER) were an explicit push to open this black box of behavior change interventions (Abraham et al., Citation2014). The workgroup concluded that oftentimes, information about putative mechanisms by which interventions generate their effects, as well as descriptions of the design and implementation of interventions lacked sufficient detail. Suboptimal reporting often has its roots in the start of the intervention development process, and ultimately leads to interventions that cannot be replicated and generalized across contexts. Bearing in mind the four WIDER recommendations (1. standardized descriptions of intervention characteristics that allow accurate replication across contexts, by 2. clarifying the change processes considered necessary to prompt a change in the specified behaviors, how the intervention design was informed by theoretical considerations or models of causal or regulatory processes and what mechanism-based change techniques were included, 3. published in detailed behavior change intervention manuals at the same time as behavior change intervention evaluation reports, 4. also including details of any services or care provided to control groups, such as usual care), we introduce acyclic behavior change diagrams (ABCDs) as an operational innovation to help optimize transparent development and reporting of interventions. ABCDs should not be seen as an intervention planning framework on its own (e.g. Intervention Mapping) but rather as a complementary tool to such frameworks, visualizing the active components of behavior change interventions. In this paper, we will also outline the advantages of using ABCDs. Notably, one advantage is their machine-readability (or: computer-readability), meaning that ABCD matrices are in a format that can be processed automatically and can therefore be imported directly into a database without time-consuming manual coding of active components. This is expected to help future systematic review processes once ABCDs are used more widely in future interventions. Since using ABCDs requires insight into how an intervention works, we will start with a description of seven aspects of behavior (change) that together form the causal-structural chain of an intervention.
The causal-structural chain
Target behavior and sub-behaviors
Behavior can be seen as an umbrella term; a specific behavior that is targeted in an intervention typically comprises related but different sub-behaviors (Crutzen & Peters, Citation2019). For example, the target behavior of ‘having a healthy diet’ consists of sub-behaviors like ‘buying healthy ingredients’, ‘cooking a healthy meal’, and ‘declining offered snacks’. It is important to gain insight into these various sub-behaviors because they often have different causes. For example, the psychological constructs that determine whether one ultimately engages in a behavior (the behavioral determinants) can differ from behavior to behavior (Peters, Citation2014). Acknowledging that healthy eating is important (which is part of attitude) might be relevant to buying healthy ingredients, while feeling capable of cooking a healthy meal (which is part of self-efficacy) might be important for cooking a healthy meal, and the expectation that declining a snack is met with approval (which is part of perceived norms) might be important for indeed declining that snack.
Determinants and sub-determinants
Changing the relevant behavioral determinants is what lies at the heart of behavior change (Crutzen et al., Citation2017). Here, determinants are defined as psychological constructs that are assumed to causally contribute to behavior performance. In the brief example above about having a healthy diet, relevant determinants that need to be changed would be attitude, self-efficacy, and perceived norms. Behaviors can also be mostly habitual, or determined by self-monitoring, or by risk perception, or any combination of these and dozens of other potential determinants. Moreover, determinants can differ in the degree to which they are cognitive or affective or to which they are explicit or implicit.
Determinants are generic psychological constructs and changing them requires changing the constituent sub-determinants. Sub-determinants are more specific constructs that have a structural or causal relationship with the overarching determinant, and by virtue of their higher specificity are sufficiently concrete to be targeted in an intervention (e.g. by means of text, speech, images or video). In other words, theories that do not provide clear links from their more generic, abstract constructs (i.e. determinants), through lower-level variables (i.e. sub-determinants), to a level of sufficient psychological specificity to enable interfacing with tangible aspects of reality, cannot be utilized in behavior change interventions. Therefore, it is necessary to be specific about sub-determinants when applying a theory – or more broadly when developing an intervention – to target a specific behavior. In the healthy diet example, an intervention does not target attitude or self-efficacy directly, but those sub-determinants that cover specific aspects of human psychology captured by these determinants instead. For example: behavioral beliefs regarding the consequences of buying healthy ingredients (which are part of attitude), and control beliefs regarding specific capabilities to cook a healthy meal (which are part of self-efficacy). Like determinants, which sub-determinants are relevant differs from behavior to behavior and from population to population. For example, beliefs regarding the price of healthy ingredients may be more relevant in lower-income populations than in higher-income populations, even if attitude in general is a relevant determinant in both populations. In that case, it is the relevance of the sub-determinants underlying attitude that differs between populations.
Behavior change principles, practical applications, and conditions for effectiveness
Changes in sub-determinants and determinants involve learning. Crutzen and Peters (Citation2018) explain which learning principles humans acquired throughout their evolution and how these evolutionary learning processes are used to establish behavior change. These learning processes are very rudimentary, and behavior change research often instead concerns higher level behavior change principles (BCPs), termed ‘manipulations’ in experimental psychology, and ‘behavior change methods’ (Bartholomew et al., Citation1998; Kok et al., Citation2016) or ‘behavior change techniques’ (Abraham & Michie, Citation2008; Michie et al., Citation2013) in health psychology.
BCPs are general descriptions of procedures that are able to change (sub)determinants by engaging evolutionary learning processes (Crutzen & Peters, Citation2018; Kok et al., Citation2016). The choice which BCP to use is guided by which determinants need to be changed as BCPs differ in the extent to which they are suitable to change specific determinants. Being theoretical principles, how BCPs manifest in real-life interventions can vary considerably: the same BCP can be used in flyers, movies, counseling protocols, or website modules. For example, the BCP ‘modeling’ may be used to show step by step demonstrations on how to easily cook a healthy meal (and thereby targeting self-efficacy). Nevertheless, it can also be used to demonstrate declining an offered snack, or how to ask for consent in a romantic situation (which thereby also targets self-efficacy). BCPs are, however, only effective in engaging evolutionary learning processes when the conditions for effectiveness (also known as parameters for use) are taken into account. For ‘modeling’, one of the conditions is that the receiver of the intervention identifies with the model and a coping model is used instead of a mastery model (Kok et al., Citation2016). To conclude, regardless of an intervention’s form, it is important to design these practical applications according to the definitions and parameters of the implemented BCPs to secure successful engagement of the underlying learning processes.
The ABCD and ABCD matrix
By describing the seven aspects of behavior change (target behavior, sub-behaviors, determinants, sub-determinants, applications, conditions for effectiveness and behavior change principles) we have defined the causal-structural chain underlying behavior change interventions (Crutzen & Peters, Citation2019). Such chains show what an intervention entails and how it is hypothesized to lead to behavior change in terms of both causal (what influences what) and structural (what consists of what) terms. Every chain is self-sustaining: it always contains exactly seven links from behavior change principle through sub-determinants to target behavior. This means that if any of the seven links is broken, the intervention developer’s assumptions no longer provide a reason to assume that that specific chain will contribute to behavior change. A typical intervention often contains a multitude of such chains. On the one hand, this complexity or ‘high cumulative dose’ is advantageous; the more causal-structural chains an intervention consists of, the lower the impact of one or two faulty links. However, at the same time, the plethora of assumptions and information to document and keep track of can cause intervention developers and stakeholders to be overwhelmed when developing and reporting interventions. Therefore, in this article, we propose and show the potential of the use of Acyclic Behavior Change Diagrams (ABCDs). They are a visual representation of all causal-structural chains that express a set of important assumptions as to why and how an intervention would achieve behavior change. ABCDs are produced from ABCD matrices, which are machine-readable representations of those assumptions in a spreadsheet format. ABCDs show at a glance which causal-structural relationships underlie an intervention. Therefore, we expect ABCDs to contribute to the development and reporting of interventions in a transparent manner.
Example
Van Stralen et al. (Citation2008) report how they developed, implemented and evaluated a program to motivate older adults to initiate and maintain physical activity with the Intervention Mapping protocol. (Active plus; recognized as a theoretically sound intervention in the Dutch recognition system for health promotion interventions; Brug et al., Citation2010). Based on this description, we created an ABCD matrix and ABCD, thereby illustrating how ABCDs can be of use to report interventions in a clear, concise, and consistent manner. To ensure rightful representation, the corresponding author of Van Stralen et al. (Citation2008) was contacted to examine whether our ABCD reflected the part of the intervention that they described. This part, and therefore the focus of the ABCD matrix and ABCD, is the target behavior of initiating physical activity. Note that the same logic applies to other possible target behaviors of the at-risk group (e.g. maintaining physical activity) as well as other environmental agents that might be targeted in an intervention (e.g. health professionals, policy makers). The target behavior of initiating physical behavior has been divided into three sub-behaviors: ‘older adults monitor their recreational physical activity level’, ‘older adults indicate reasons to be physically active as recreation’, and ‘older adults identify solutions to take away the barriers to being physically active for recreation’ (note that these sub-behaviors are known as ‘performance objectives’ in the Intervention Mapping literature and the Active plus protocol; Bartholomew et al., Citation1998; Van Stralen et al., Citation2008). For the second sub-behavior, two sub-determinants were selected: ‘older adults list the personally relevant benefits of being sufficiently active’, and ‘older adults express a positive attitude about being sufficiently active’, falling under the determinants of awareness and attitude, respectively. Then, the behavior change principle ‘self-monitoring’ was used to create a practical application for the first sub-determinant in the form of ‘self-complete logbooks to monitor own physical activity behavior in last week’ based on which rewarding feedback was given, taking into account the conditions for effectiveness that ‘the monitoring must be of the specific behavior (i.e. not of the physiological state or health outcome). The data must be interpreted and used. The reward must be reinforcing to the individual’. The second sub-determinant, ‘tailored feedback and argumentation’ has been used to create the practical application of ‘computer tailored feedback in text on perceived positive and negative consequences of physical activity. New arguments to change opinions are provided in text’, taking into account the conditions for effectiveness ‘Feedback needs to be individual, follow the behavior in time, and be specific’.
We only described two causal-structural pathways in the previous paragraphs, but it might have become clear already that such an intervention description is not ideal in terms of clarity and readability. ABCD matrices offer a standardized and machine-readable way to include this information. While we recommend publishing such matrices in machine-readable format (comma separated files,.csv, OpenDocument files,.ods, or Office Open XML,.xlsx; the benefit of this is explained in the next section), as an illustration we include an excerpt in .
Table 1. ABCD matrix of two of the causal-structural chains comprising Active plus.
While the ABCD matrix is optimized to be read by machines, the ABCD that can be produced from it is however human-readable. It clearly visualizes the seven columns of the ABCD matrix, thereby providing a clear overview of all relations and underlying assumptions of the intervention (). All cells with the same content are merged to be represented by the same element, with the only exception being the determinants. They are only merged within the corresponding sub-behaviors since determinants that determine different sub-behaviors may be labeled as the same psychological construct (e.g. attitude) but represent different aspects of human psychology in reality (Crutzen & Peters, Citation2019).
Figure 1. ABCD for (parts of) the intervention Active plus (Van Stralen et al., Citation2008). The dashed boxes and red lines represent the pathways that were discussed in the text. Note. Each box represents one of the seven columns in the ABCD matrix. The lines with the fork-shaped ends in between behavior change principles and application stand for ‘implemented in’. Applications are manifest constructs and have, in the tradition of drawing pathways in structural equation modeling (SEM; Stein et al., Citation2012), been placed in rectangles. In turn, the determinants have been placed in ovals as they are latent constructs (again in the tradition of SEM). The lines with three types of arrowheads represent three relationship types: regular arrowheads represent causal relationships, points represent structural relationships, and forks represent that applications implement a BCP. The shape of the boxes for sub-determinants has been chosen to distinguish them from the rest, as they are less likely to be conceptualized as a latent construct on their own, instead being closer to what in SEM is called an indicator.
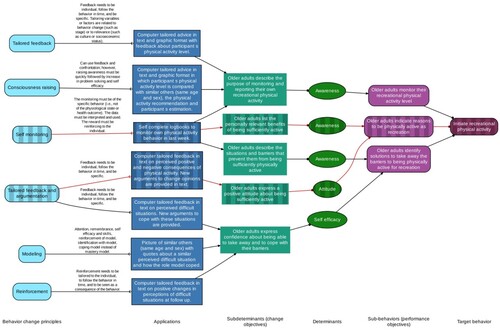
Why?
As previously mentioned, we believe that ABCDs are helpful in creating a clear and concise visualization of interventions. Besides that, there are several other, related advantages, that we would like to address here. First, as ABCDs offer an overview of all elements and underlying assumptions of an intervention, they are helpful in making implicit assumptions explicit, and in checking whether all relevant (sub)determinants of behavior are targeted or whether there are missing links. In this way, they help keep track of and manage the intricacies underlying complex interventions. Second, ABCDs facilitate reflecting on, discussing, and communicating the assumptions underlying an intervention. A clear visualization enables team members and other stakeholders, such as the executive intervention producers or target population members, to get a better grasp of the intervention and makes it possible to discuss those assumptions during the development stage and improve the intervention.
Third, ABCDs support thorough intervention evaluation. Because ABCDs explicitly list all psychological constructs that each intervention targets, they function as a blueprint outlining exactly what to measure in evaluations to identify which parts of the intervention worked well and which would benefit from an update. Ideally, an evaluation is designed to measure change in all sub-determinants, determinants, and sub-behaviors, so that it is clear which links may be responsible for broken chains. However, many situations are not ideal, and in such cases ABCDs can help justify and document decisions as to which elements are included and omitted in an evaluation.
Applying these advantages to the example of Van Stralen et al. (Citation2008), using ABCDs might have helped in specifying intervention contents. For example, creating the ABCD revealed that the description of Active plus was not entirely complete in how the parameters for the BCP ‘self-monitoring’ were applied in the intervention. This BCP requires that the ‘data must be interpreted and used’ but in our view, the description of the intervention did not elaborate on how this was done. Using ABCDs might also have been helpful in specifying sub-determinants into more concrete ones that allow for more targeted (and potentially more effective) intervention elements. For example, when discussing this ABCD with team members and stakeholders, the question could have arisen as to what exactly were the personally relevant benefits referred to in the sub-determinant ‘older adults list the personally relevant benefits of being sufficiently active’. After all, developing an application communicating those benefits inevitably requires a concrete list. Based on this discussion, that sub-determinant could have been specified as: ‘older adults state that physical activity reduces the chance of cardiovascular disease’.
The fourth benefit, briefly described in the introduction section, concerns the machine-readability of the ABCD matrices underlying ABCDs. Human coding of the assumptions underlying an intervention based on publications, intervention manuals, and intervention content is error-prone, costly, and often yields incomplete descriptions (Albrecht et al., Citation2013; De Bruin et al., Citation2021; Kok & Mesters, Citation2011; Schaalma & Kok, Citation2009). Although ABCD matrices do not cover all aspects of an intervention, they do cover a set of important basic assumptions (what does an intervention target and with what?), and the benefit of this simplicity is that they are simple to use and do not require specific software. Since using ABCDs during the intervention design and development stages is beneficial on its own, the underlying ABCD matrix could be seen as an effort-free advantageous by-product by the time the intervention is completed. Namely, there are no additional costs to transparently publishing the causal-structural chains that are assumed to underlie an intervention. In systematic reviews, the ABCD matrices can then be imported directly into databases with negligible costs, whilst disclosing complete and accurate data.
As a fifth benefit, we believe the ABCD approach fits well with other initiatives to gain a deeper understanding and synthesis of the literature on active intervention elements. For example, over the past years, Michie et al. (Citation2020) have been working on the behavior change intervention ontology; a database using artificial intelligence that will contain detailed information on active ingredients of behavior change interventions, being able to provide intervention developers with insights on which elements have been proven to be most effective in the past. So, when an intervention developer has collected elements based on their search in the ontology database, they will have to plan the intervention, either with the Intervention Mapping framework, the Behavior Change Wheel or any other framework. Whichever framework is used, the ABCD will help the developer in visualizing the intervention and to get a clear understanding of the (sometimes implicit) assumptions and causal-structural relations underlying the intervention. The intervention developer can then put the ABCD into their report, enabling others to get a clear picture of that specific intervention. Of course, the findings from this report can also be integrated in the ontology, contributing to the insights on active intervention ingredients on an aggregate level. At the level of a specific intervention, we believe that the use of ABCDs leads to more transparent reporting of the active ingredients of interventions.
How?
Creating an ABCD can be achieved in three ways, all using Free/Libre Open Source Software. First, the behaviorchange::abcd() function in the behaviorchange package can be used in R (i.e. using script). Second, Jamovi offers the behaviorchange module, which offers the possibility of simultaneously making an ABCD matrix and an ABCD (i.e. using a point-and-click interface). For an extensive explanation on how to create an ABCD with either R or Jamovi, please see chapter 9 in the Book of Behavior Change (Crutzen & Peters, Citation2019). For now, we will focus exclusively on the third, and in our eyes, easiest way of creating an ABCD: a web application that can be found on https://a-bc.eu/apps/abcd, and does not require any software installation.
The first step in creating an ABCD with the app is to specify an ABCD matrix. This can be done in the app itself, but we recommend using your preferred spreadsheet editor (e.g. if working in a team, Google Sheets can be a convenient way to collaborate). In the app’s ‘data’ tab, the link to a Google Sheet can be pasted (if its sharing settings make it viewable with the link), and the information can be directly imported. Alternatively, a spreadsheet can be uploaded in.xlsx or.csv format, or one can manually specify the ABCD matrix in the app. ABCD matrices that are imported from Google Sheets or from an uploaded file will be transformed to a standardized ABCD matrix. At this point, it is still possible to edit the ABCD matrix in the app. After the ABCD matrix has been created, the second step is to click on the button labeled ‘generate plot’, which will turn the ABCD matrix into an ABCD. The generated ABCD can be downloaded and saved in different formats. The SVG format, for example, can be useful when one wants to make changes to the ABCD’s appearance (e.g. adding color with Inkscape, like was done in for the paths that are described in more detail in the main text).
Regarding the specific contents of the ABCD matrix, this article does not prescribe methods to identify (sub-)behaviors and (sub-)determinants or to select behavior change principles. Being a generic tool, ABCDs can be used in the context of different intervention planning frameworks, each with their own ideas on how to engage in several planning aspects, for example regarding how to select and formulate (sub)determinants. Within the Intervention Mapping framework, behavior change methods are mapped on determinants, meaning that a suitable method is chosen based on the decision of which determinants need to be targeted (Kok et al., Citation2016). BCTs, on the other hand, have been mapped on mechanisms of action (Carey et al., Citation2019; Connell et al., Citation2019). So, when using ABCDs during development of an intervention using the Behavior Change Wheel, the names of the columns in the ABCD can be changed from ‘behavior change method’ to ‘behavior change technique’ and from ‘determinant’ to ‘mechanism of action’ and can then be used to get a clear overview of the intervention. Another example is the use of Core Processes – a helpful and systematic approach to answer questions relevant to problem definition and solution using expert knowledge, empirical evidence and theory, and collecting additional data (Ruiter & Crutzen, Citation2020). This approach can also be used to answer planning questions such as ‘What are the determinants of X’ (see, for example, Nalukwago et al., Citation2018). The identified determinants can then be used to complete the ABCD matrix.
Anecdotal evidence
First experiences with the use of ABCDs in systematic behavior change were gained both in research and in education and have been shown to be promising. Berninger et al. (Citation2020) used ABCDs in developing a computer-based intervention to reduce sedentary behavior among office employees (please note that the appearance of the ABCD used in this paper is somewhat different from the current lay-out, yet the underlying assumptions and elements are exactly the same). ABCDs have also been used in the context of the evaluation of the Dutch sexual health website Sense.info (Metz et al., Citation2021). The content of Sense.info’s chlamydia page was put in an ABCD to get a clear overview of all elements and how they were intended to be used to lead to behavior change. The subsequent evaluation of the actual use of the intervention benefited from the structure that the ABCD offered. In education, ABCDs were used in intervention design courses both in psychology and health science programs. Students used ABCDs when developing intervention ideas, and this helped them cover all relevant links of the causal-structural chain.
Conclusion
This paper introduced ABCDs in order to achieve clearer and more transparent development, evaluation, and reporting of behavior change interventions. This is highly relevant given the oftentimes expressed concerns about suboptimal reporting of interventions. Although our examples were mainly derived from research in health psychology and health promotion, we believe ABCDs can be used for behavior change interventions in general.
Ethics statement
Ethics approval was not required as this study is a description of a tool and no primary data collection has taken place.
Prior versions
Preprints of this article are available online at https://psyarxiv.com/mzc4u/ (https://doi.org/hrz4).
Data, materials, and online resources
The web application to create an ABCD can be found at https://a-bc.eu/apps/abcd.
The spreadsheet file containing the ABCD matrix of Active plus can be found in the Open Science Framework repository at https://osf.io/epbm4/.
Open Scholarship
This article has earned the Center for Open Science badges for Open Data and Open Materials through Open Practices Disclosure. The data and materials are openly accessible at https://osf.io/epbm4/.
Acknowledgements
We would like to thank Luke McGuinness for coding the ABCD Shiny App, Maartje van Stralen for corroborating the ABCD reflecting part of the Active plus intervention, and Lior Weinreich for proofreading the manuscript.
Disclosure statement
No potential conflict of interest was reported by the author(s).
Additional information
Funding
References
- Abraham, C., Johnson, B. T., de Bruin, M., & Luszczynska, A. (2014). Enhancing reporting of behavior change intervention evaluations. Journal of Acquired Immune Deficiency Syndromes, 66(3), S293–S299. https://doi.org/10.1097/QAI.0000000000000231
- Abraham, C., & Michie, S. (2008). A taxonomy of behavior change techniques used in interventions. Health Psychology, 27(3), 379–387. https://doi.org/10.1037/0278-6133.27.3.379
- Albrecht, L., Archibald, M., Arseneau, D., & Scott, S. D. (2013). Development of a checklist to assess the quality of reporting of knowledge translation interventions using the workgroup for intervention development and evaluation research (WIDER) recommendations. Implementation Science, 8(1), 52. https://doi.org/10.1186/1748-5908-8-52
- Bartholomew, L. K., Parcel, G. S., & Kok, G. (1998). Intervention mapping: A process for developing theory- and evidence-based health education programs. Health Education and Behavior, 25(5), 545–563. https://doi.org/10.1177/109019819802500502
- Berninger, N. M., ten Hoor, G. A., Plasqui, G., Kok, G., Peters, G.-J. Y., & Ruiter, R. A. C. (2020). Sedentary work in desk-dominated environments: A data-driven intervention using intervention mapping. JMIR Formative Research, 4(7), e14951. https://doi.org/10.2196/14951
- Black, N., Johnston, M., Michie, S., Hartmann-Boyce, J., West, R., Viechtbauer, W., Eisma, M. C., Scott, C., & Bruin, M. (2020). Behaviour change techniques associated with smoking cessation in intervention and comparator groups of randomized controlled trials: A systematic review and meta-regression. Addiction, 115(11), 2008–2020. https://doi.org/10.1111/add.15056
- Brug, J., van Dale, D., Lanting, L., Kremers, S., Veenhof, C., Leurs, M., van Yperen, T., & Kok, G. (2010). Towards evidence-based, quality-controlled health promotion: The Dutch recognition system for health promotion interventions. Health Education Research, 25(6), 1100–1106. https://doi.org/10.1093/her/cyq046
- Carey, R. N., Connell, L. E., Johnston, M., Rothman, A. J., de Bruin, M., Kelly, M. P., … Michie, S. (2019). Behavior change techniques and their mechanisms of action: A synthesis of links described in published intervention literature. Annals of Behavioral Medicine, 53(8), 693–707. https://doi.org/10.1093/abm/kay078
- Cialdini, R. B. (2008). Influence: Science and practice. Allyn & Bacon.
- Connell, L. E., Carey, R. N., de Bruin, M., Rothman, A. J., Johnston, M., Kelly, M. P., … Michie, S. (2019). Links between behavior change techniques and mechanisms of action: An expert consensus study. Annals of Behavioral Medicine, 53(8), 708–720. https://doi.org/10.1093/abm/kay082
- Craig, P., Dieppe, P., Macintyre, S., Michie, S., Nazareth, I., & Petticrew, M. (2008). Developing and evaluating complex interventions: The new medical research council guidance. BMJ, 337, a1655. https://doi.org/10.1136/bmj.a1655
- Crutzen, R., & Peters, G.-J. Y. (2018). Evolutionary learning processes as the foundation for behaviour change. Health Psychology Review, 12(1), 43–57. https://doi.org/10.1080/17437199.2017.1362569
- Crutzen, R., & Peters, G.-J. Y. (2019). The Book of Behavior Change (1st ed.). https://doi.org/10.5281/zenodo.3570967
- Crutzen, R., Peters, G.-J. Y., & Noijen, J. (2017). Using confidence interval-based estimation of relevance to select social-cognitive determinants for behaviour change interventions. Frontiers in Public Health, 5, 165. https://doi.org/10.3389/fpubh.2017.00165
- De Bruin, M., Black, N., Javornik, N., Viechtbauer, W., Eisma, M. C., Hartman-Boyce, J., Williams, A. J., West, R., Michie, S., & Johnston, M. (2021). Underreporting of the active content of behavioural interventions: A systematic review and meta-analysis of randomised trials of smoking cessation interventions. Health Psychology Review, 15(2), 195–213. https://doi.org/10.1080/17437199.2019.1709098
- Kelly, M. P., & Barker, M. (2016). Why is changing health-related behaviour so difficult? Public Health, 136, 109–116. https://doi.org/10.1016/j.puhe.2016.03.030
- Kok, G., Gottlieb, N. H., Peters, G.-J. Y., Mullen, P. D., Parcel, G. S., Ruiter, R. A. C., Fernández, M. E., Markham, C., & Bartholomew, L. K. (2016). A taxonomy of behavior change methods: An intervention mapping approach. Health Psychology Review, 10(3), 297–312. https://doi.org/10.1080/17437199.2015.1077155
- Kok, G., & Mesters, I. (2011). Getting inside the black box of health promotion programmes using intervention mapping. Chronic Illness, 7(3), 176–180. https://doi.org/10.1177/1742395311403013
- Lewin, K. (1936). Principles of topological psychology. McGraw-Hill. https://doi.org/10.1037/10019-000
- Metz, G., Roosjen, H., Zweers, W., & Crutzen, R. (2021). Evaluating use of web-based interventions: An example of a Dutch sexual health intervention. Health Promotion International, https://doi.org/10.1093/heapro/daab190
- Michie, S., Richardson, M., Johnston, M., Abraham, C., Francis, J., Hardeman, W., Eccles, M. P., Cane, J., & Wood, C. E. (2013). The behavior change technique taxonomy (v1) of 93 hierarchically clustered techniques: Building an international consensus for the reporting of behavior change interventions. Annals of Behavioral Medicine, 46(1), 81–95. https://doi.org/10.1007/s12160-013-9486-6
- Michie, S., West, R., Finnerty, A. N., Norris, E., Wright, A. J., Marques, M. M., … Hastings, J. (2020). Representation of behaviour change interventions and their evaluation: Development of the upper level of the behaviour change intervention ontology [version 2; peer review: 2 approved]. Wellcome Open Research, 5(123), https://doi.org/10.12688/wellcomeopenres.15902.2
- Nalukwago, J., Alaii, J., van den Borne, B., Mukisa Bukuluki, P., & Crutzen, R. (2018). Application of core processes for understanding multiple concurrent sexual partnerships among adolescents in Uganda. Frontiers in Public Health, 6, 371. https://doi.org/10.3389/fpubh.2018.00371
- Peters, G.-J. Y. (2014). A practical guide to effective behavior change: How to identify what to change in the first place. European Health Psychologist, 16(4), 142–155. https://doi.org/10.31234/osf.io/hy7mj
- Ritchie, H., & Roser, M. (2019). Causes of death. Our world in data. https://ourworldindata.org/causes-of-death
- Ruiter, R. A. C., & Crutzen, R. (2020). Core processes: How to use evidence, theories, and research in planning behavior change interventions. Frontiers in Public Health, 8. https://doi.org/10.3389/fpubh.2020.00008
- Schaalma, H., & Kok, G. (2009). Decoding health education interventions: The times are a-changin. Psychology & Health, 24(1), 5–9. https://doi.org/10.1080/08870440801995802
- Stein, C. M., Morris, N. J., & Nock, N. L. (2012). Structural equation modeling. In R. C. Elston, J. M. Satagopan, & S. Sun (Eds.), Statistical human genetics (Vol. 850, pp. 495–512). Humana Press. https://doi.org/10.1007/978-1-61779-555-8_27
- Van Stralen, M. M., Kok, G., de Vries, H., Mudde, A. N., Bolman, C., & Lechner, L. (2008). The active plus protocol: Systematic development of two theory- and evidence-based tailored physical activity interventions for the over-fifties. BMC Public Health, 8, 399. https://doi.org/10.1186/1471-2458-8-399