ABSTRACT
Background
Due to the COVID-19 pandemic many employees perform under increasingly digital conditions. Enabling home office environments became mandatory for companies wherever possible in consideration of the ongoing pandemic. Simultaneously, studies reported on digital stress. The current literature lacks rigorous research into digital stress on psychosomatic outcomes, emotions, and disease. Therefore, we endeavor to understand how digital stress developed over the course of the pandemic and if it predicts differences in negative emotions and physical complaints in the home office setting.
Methods
To answer the research question, we conducted an online survey among 441 employees in 2020 and 398 employees in 2022 from three municipal administrations in Germany, who were working from home at least occasionally. We used a cluster analysis to detect digitally stressed employees. Regression analyses were performed on digital stress, negative emotions, and physical complaints.
Results
The analysis revealed an increase from 9 to 20% in digital stress, while negative emotions and physical complaints did not show evident differences. In the multivariate model, we observe a change in the proportion of digitally stressed employees between 4 and 17%, while the control variables explain around 9%.
Conclusions
Digital stress did not significantly affect either negative emotions or physical complaints. However, digital stress appeared to exert a more substantial predictive influence on negative emotions. The study emphasizes rising digital stress, which contradicts a positive adaption to the digital working conditions within the observed period. The psychosomatic relations are low or lagged. Further research investigating digital stress and countermeasures, especially to understand how to prevent harmful long-term effects such as distress resulting from working from home conditions, is needed.
Background
As one of the many knock-on effects of the COVID-19 pandemic, society at large will and is facing challenges linked to increased psychological stress and social isolation (Bouziri et al., Citation2020). In response to government restrictions, many employers have extemporaneously relocated their employees’ office workstations to home offices (Herrmann & Frey Cordes, Citation2020). Beyond Germany, the rapid mandated transition to a home office setup became a normalized measure to combat the ongoing pandemic (German Federal Government, Citation2022).
Flexible forms of work, which lead to an overlap between home and workplace, are associated with giving employees more resources for health-promoting behavior on the one hand, but, on the other hand propagate psychological stress factors (Beermann et al., Citation2018). There might be individual advantages for the organization of work, such as the elimination of commuting or improvement of time management, flexibility and autonomy. Moreover, employees who work occasionally in home office are more satisfied with their ergonomics and report less musculoskeletal complaints than employees working solely on site (Kettschau et al., Citation2023). Still, the communication within companies lacks clear structures for working from home (Bölsch-Peterka et al., Citation2022). The elongated work day, which arises with blurred work-life boundaries that can come with home office, results in an increase in work-related impacts on health (Gimpel et al., Citation2020; Samek Lodovici et al., Citation2021).
Working from home during the pandemic
Home office is defined as a workplace in one's own home that is equipped with the necessary telecommunication technology (Duden, Citation2018). The general trend toward Work 4.0 and the adaptation of the working world due to structural changes, such as globalization and digitization, drive the spread of mobile work, teleworking, and the use of home offices (Rieke et al., Citation2020). In 2019, 12.8% of the workforce worked in a home office, compared to 21.0% in the first year of the pandemic. In 2021, 24.8% of all employees in Germany worked at least occasionally in a home office, and as many as 36% in the public administration sector (German Federal Statistical Office, 2021).
Home office work can be implemented as mobile working or telecommuting, defined by contractual agreements and regulations of the workplace ordinance (§ Citation2 ArbStättV, Citation2022). Telework is accompanied by ‘permanently installed computer workstations in the private area of the employee’ at contractually agreed weekly working hours (§ Citation2 ArbStättV, Citation2022). In contrast, mobile working has not yet been defined in a legally binding way in Germany but typically describes a location-independent work activity of at least ten hours per week that is not tied to a fixed workplace, such as the home (German Parliment, Citation2017). Because of the rapid implementation of home office protocols for many sectors and employees, it can be assumed that hardly any contractual amendments have been made for the home office (Samek Lodovici et al., Citation2021). Due to the COVID-19 pandemic, most workers engage in some form of hybrid working, a mixture of telecommuting and mobile work. The workplace is relocated (in line with telecommuting) to the employee's home, but without a contractual agreement and accompanying legal obligations. In this context, working from home has become established and, after prior coordination with the company, encompasses temporary or occasion-related activity in the private sphere (§ Citation2 ArbStättV, Citation2022; German Parliament, Citation2017). In the following, this particular form is assumed to be the home office setting, i.e. working from home (WFH).
Barone Gibbs et al. (Citation2021) found that transitioning to remote work led to increased sedentary behavior on non-work days and a decline in quality of life and overall workers health. From another vantage, Niebuhr et al. (Citation2022) reveal that the functionality of technical equipment in employees’ home offices positively impacts their health, including work ability, stress-related symptoms, and job satisfaction, which emphasizes the potential of legal regulations for WFH mentioned in the prior paragraph. The weekly amount of WFH influences stress-related symptoms, i.e. a higher amount of weekly WFH working hours, is associated with more symptoms. Barone Gibbs et al. (Citation2021) discovered that workers who consistently worked remotely experienced higher levels of stress. While constant WFH has a negative impact on mental well-being, no difference was found when considering those who work from home on occasion. The ‘always remote’ group also reported decreased physical functioning (Barone Gibbs et al., Citation2021); rather recommending a hybrid model (partly working from home and going to the office).
Xiao et al. (Citation2021) reported that 73.6% of respondents encountered new mental health issues with a further 64.8% reporting physical health issues. In addition to an increase in depressive symptoms during pandemic-induced remote work, office workers reported musculoskeletal pain in the neck, shoulders, wrists, upper back, and hips/thighs (Majumdar et al., Citation2020). These findings were consistent with Seva et al. (Citation2021), who found that a majority of workers experienced lower back pain (74.1%), neck pain (67.9%) and shoulder pain (67.3%). WFH had a detrimental impact on the physical complaints experienced by certain participants, specifically those who were already dealing with issues such as back pain and neck pain (Moretti et al., Citation2020). 38.1% of participants reported worsened lower back pain, and 50% reported worsened neck pain since starting WFH. Workers without musculoskeletal pain reported significantly higher job satisfaction. Men, middle-aged individuals and those commuting to different municipalities particularly benefit from WFH, illustrating the advantages of WFH for certain groups (Denzer & Grunau, Citation2021). However, individuals who extensively utilize information and communication technology are more likely to engage in cognitively demanding tasks and experience frequent interruptions, both of which have been linked to elevated levels of reported headaches and eyestrain (Samek Lodovici et al., Citation2021; Vargas Llave et al., Citation2020).
Conversely, the perception of increased autonomy has positive effects on employees’ overall job satisfaction (Giovanis & Ozdamar, Citation2021). As we can see, similar associations apply to WFH that we already know from traditional models; mental strain results from the interaction of job demands and job decision latitude (Karasek, Citation1979). The lack of ergonomic equipment and insufficient space (for work) at home are associated with increased job demands.
Mental strain and digital stress
Mental stress is defined as the external influences that affect a person psychologically (DIN EN ISO, 10075-Citation1 Citation2018; Wieland & Hammes, Citation2014). Mental (or syn. psychological) strain is the corresponding immediate effect of mental stress within the individual, i.e. internally. Both terms are formulated neutrally for the workplace setting, because it does not have exclusively negative or exclusively positive effects (DIN EN ISO, 10075-Citation1 Citation2018), (Hammes & Wieland, Citation2017). Mental stress and strain unleash a chain of effects internally that is also dependent on the characteristics of the affected individual (Hammes & Wieland, Citation2017). The effects can include, on the one hand, stimulating effects, such as further development of personal skills or health, and, on the other hand, impairing effects, such as the occurrence of psychosomatic illnesses or early retirement (Hammes & Wieland, Citation2017). Acute, stimulating stress has a positive function, referred to as eustress (e.g. motivation, feeling of happiness), while stress that is perceived as unpleasant or overwhelming has a negative function, known as distress (e.g. despair, pain). Distress brings with it a strongly increased tension in the body and consequently can lead to a decrease in attention span and performance (Meunier et al., Citation2022). When maintained permanently, chronic stress is accompanied by further effects harmful to health. After headaches and eyestrain, technology use has the strongest associations with high stress levels which manifest as physical symptoms (Messenger et al., Citation2017).
Digital stress or synonymous technostress (internationally the latter, but in Germany digital stress is more widely used) occurs in individuals who are involved with digital technologies. It refers to the difficulty of dealing with new technologies in a healthy way, which leads to stressful experiences (Gimpel et al., Citation2018). Those affected are more likely to suffer from accompanying symptoms such as headaches, fatigue and exhaustion, and sleep disturbances (Gimpel et al., Citation2018). Digital stress is negatively related to well-being, health, and work ability (Gimpel et al., Citation2019).
When Gimpel et al. (Citation2018) examined ‘digital stress in Germany’, they concluded that digital stress is more pronounced among younger workers. Other studies specify that older workers seem to be influenced more by technostress, while younger individuals are more vulnerable to overload (The mental health of workers in the digital era, Citation2022). Wrede et al. (Citation2021) show that within the German public administration setting (among others) older age, feeling overwhelmed, as well as resignation, are significant risk factors for digital stress. While at the beginning of the two phase study, a minority of employees across the municipalities used home office, the proportion rose to 76.4 percent during the pandemic (Claassen et al., Citation2022). In the first phase of survey results, Wrede et al. (Citation2021) found that about 10 percent of employees are digitally stressed. Since individual digital competence and functioning technology is mandatory to work from home, digital stress is a potential disruptive factor that we need to keep an eye on. Digital stress factors are changing; for example, a lack of a sense of achievement or the omnipresence of technology are perceived as more stressful, while factors attributable to inexperience with information technology are decreasing (Gimpel et al., Citation2020).
With regard to gender, it was found that women worked in more digitalized workplaces and concurrently experienced a higher level of digital stress than men (Gimpel et al., Citation2018). Broadly, males and females present some differences in terms of how they relate to to technology, with the latter more susceptible to technostress (Gravelling et al., Citation2020). Consequently, ‘zoom fatigue’ is significantly more prevalent among female workers (Fauville et al., Citation2021). Following Ragu-Nathan et al. (Citation2008), perceived digital stress decreases with level of education and job experience. Lower socioeconomic status environments are assumed to be more stressful and reduce individuals’ reserve capacity to manage stress, thereby increasing vulnerability to negative emotions and cognitions (Gallo & Matthews, Citation2003). In Korea, excessive information received through cell phone usage has been linked to heightened negative emotions, including anger and anxiety (Lee et al., Citation2016). Being young, female and having a mental illness diagnosis were risk factors for increased negative emotions at the beginning of the pandemic in Australia (Rossell et al., Citation2021). In Germany, emotional demands due to work, as well as the number of social conflicts decreased. However, personal demands were found to be increasing; in particular, financial concerns, quantitative private (e.g. household) and emotional demands in general (Gimpel et al., Citation2020). Even before the pandemic, digital stress has been associated with psychological outcomes such as burnout, depression, anxiety, and the perceived social pressure to constantly be available or connected, communication overload, and demonstrating proficiency in internet multitasking (Reinecke et al., Citation2017).
In a recent scientific review, technostress related to WFH has been examined. Of most of the studies investigating psychological well-being, only two focused on the physical well-being of remote workers, while three investigated both (Gualano et al., Citation2023). 78.9% of the studies included in this review outlined high technostress levels in remote workers (higher in female and older workers). Majumdar et al. (Citation2020) observed a decline in sleep duration during remote work among Indian office workers potentially due to increased screen time, while Schmitt et al. (Citation2021) observed an increase in overload due to the use of text-based tools among German office workers. Molino et al. (Citation2020) found higher levels of technostress among remote workers across multiple industries. Oksanen et al. (Citation2021) revealed that in Finland 17.1% of workers experienced a significant increase in technostress, while 69.7% reported little or no change, and 13.2% reported a decrease. Young age acted as a protective factor against technostress, while victimization from cyberbullying at work, using social media for work communications, and neuroticism were predictors of higher technostress (Gualano et al., Citation2023).
Psychosomatics; the relationship of stress, negative emotions, and disease
Early research already indicates associations between psychosocial factors and physical load manifested in work-related musculoskeletal complaints. Monotonous work, high perceived workload, and time pressure appear to be related to the occurrence of musculoskeletal symptoms, while low job control and insufficient social support from colleagues are positively associated with musculoskeletal disorders (Bongers et al., Citation1993). Perceived stress may serve as an intermediary in this relationship. A theoretical model proposes that psychosocial work factors, such as work pressure and lack of control, which have the potential to induce stress, may also be linked or associated with ergonomic factors such as force, repetition, and posture (Carayon et al., Citation1999). Physical ergonomic factors and psychosocial work factors need to be examined simultaneously to gain a comprehensive understanding of the causes of risk factors.
The concept of psychosomatics emphasizes psyche and soma, mind and body as interconnected, where mental health, illness, and behavior are linked to physical well-being and social conditions (Rensing et al., Citation2006). In 1895, Freud (Wörterbuch der Psychotherapie, Citation2000) expounded upon the concept of ‘conversion’, suggesting that unresolved psychological conflicts can manifest as physical symptoms. However, this does not imply a direct causal relationship between mental disorders and physical diseases. Allostasis describes the reciprocal process between psychological and physiological conditions, maintaining homeostasis and adaptive responses to stress (Dragano, Citation2007; Sterling & Eyer, Citation1988). The sympathetic-adrenal medullary system and hypothalamic–pituitary–adrenal axis play key roles in stress response, releasing hormones to increase energy supply and initiate necessary changes. Prolonged exposure to stress can disrupt adaptive compensation, leading to psychosomatic processes and pathological conditions affecting various organ systems. Stress-induced diseases, such as hypertension, can be triggered by factors like depression and workload. Continuous release of stress hormones contributes to allostatic load and disease development (McEwen, Citation2010; Sterling, Citation2012). A crucial factor in this link between stress and illness is negative emotions, especially anger (Myers, Citation2014).
Research demonstrates increased (pandemic-related) strains under increasingly digital working conditions in the home office. Findings underscore the potential importance of psychosocial factors and stress in relation to e.g. musculoskeletal health, but conclusive evidence is lacking. No evidence on the impact of specifically digital stress (external) on emotions and disease (internal) exists for the setting at the national level. By considering the interplay between these factors, insights into the complex etiology enable the development of effective interventions to prevent and manage associated disorders. Building upon the work of Wrede et al. (Citation2021), within this study, we contribute to examining the impact of digital stress among German municipal administration workers working in home office from 2020 to 2022, with emphasis on the psychosomatic triangulation illustrated in . Based on the prior mentioned theory, we assume that the employees’ experience of digital stress increased and, through mental strain, is associated with negative emotions and physical complaints.
Figure 1. Hypothesis model.
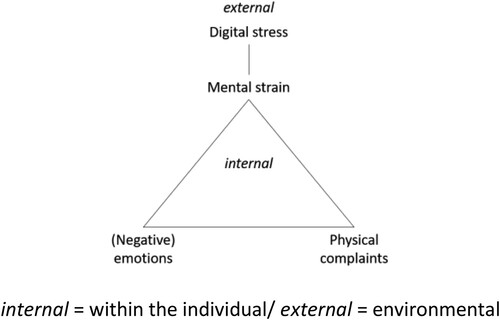
Thus, the study at hand aims to answer the following question: How has digital stress developed over the course of the pandemic? Does digital stress (DS) predict differences in negative emotions (NE) and physical complaints (PC) in the home office setting?
Methods
The data for this research study was derived from a survey conducted in two phases. The survey was part of the project ‘Health and Digital Change’ (GudW), which was supported by the digital model regional funding framework established by the Ministry of Economic Affairs, Innovation, Digitalization, and Energy of the State of North Rhine-Westphalia (NRW MWIDE). The study underwent an assessment to ascertain its compliance with the ethical guidelines stipulated in the Declaration of Helsinki for conducting medical research involving human subjects, as well as the applicable data protection regulations. Notably, it received a positive ethical evaluation and was granted approval by the Ethics Committee of the University of Witten/Herdecke, assigned with reference number 158/2020.
Data collection
Employees from three municipal administrations in North Rhine-Westphalia were surveyed using an online tool. The same survey was conducted twice, i.e. in 2020 (W1) and repeated in 2022 (W2). Working from home at least occasionally, was the inclusion criterion for the population of this study. Given the comparison between W1 and W2 and that the investigation occurred on the departmental level, the data was therefore aggregated accordingly. All employees in departments of the administration offices with ongoing digital implementation processes received an invitation link via their internal email system. Thus, participants were voluntarily engaged for approximately 15 min and their explicit consent was acquired for the purposes of scientific data processing and subsequent publication. In the event of no response within a two-week period, a single reminder was dispatched. To address privacy concerns, the invitation link directed participants to an external survey platform, specifically using the ‘LimeSurvey’ software, which was hosted by Witten/Herdecke University.
The formation of the survey's variables was guided by the constructs of mental stress and new ways of working outlined in the Joint German Occupational Safety and Health Strategy (Beck et al., Citation2017). The survey was designed within the steering committee and in consultation with all project partners on the basis of a (mental) risk assessment. The items’ formulations subordinated to the survey's variables were finalized in a group discussion in coordination with two project leaders of each of the participating municipal administrations, who were either specialists in occupational medicine or occupational health managers. In addition to questions on the type of employment, assessments were obtained on working conditions and work-related satisfaction, separately for both home office and on-site activities. In addition, questions were asked about working methods and tools related to digitalization, as well as questions about the assessment of ongoing digitalization initiatives. Other elements of the questionnaire looked at leadership and digital leadership, typical stress factors at the workplace, mental stress and physical and emotional complaints. For better comparability and identification of the relevant influencing factors, basic demographic information was requested.
Seven items represent digital stress (DS). The survey items primarily assess agreement using a scale ranging from 1 to 4, inquire about the level of perceived stress. For the data analysis, the items related to digitization were not combined into a single score due to the absence of a standardized and validated instrument for digital stress. Instead, these items were treated as distinct clustering variables. The variable, its corresponding question formulations and response coding are presented in .
Table 1. Items representing digital stress.
The eight emotions sought after are consistently formulated negatively; negative emotions (NE): sad, helpless, anxious, frustrated, angry, enraged, resigned, and overwhelmed (). For physical complaints (PC), we chose five symptoms of disease surveyed for the analysis. We selected less severe psychosomatic complaints, as they are more likely to vary within the observed period (not including joint pain, heart problems, hypertension, visual disturbances; see ). The variables PC and NE are measured in a four-point scale: almost never (1) – rarely (2) – frequently (3) – almost always (4). NE holds the average number of nominated negative emotions from the eight items, cutoff is value 3 (frequently). Simultaneously, PC shows the number of nominated physical complaints on average, cutoff is value 3 again to reflect the negative connotation. Age, gender, and income group were included as control variables. The cutoff within income group is through the German pay scale ‘E13/A13’, which equals more than 4.000 Euro gross income per month. The variables were not used ordinally but dichotomized in order to fit the further equations. The researchers determined variables and items for the analysis as well as the cut-off points in a discussion.
Table 2. Included variables.
Data analysis
The dataset has been cleansed of missing data. DS was operationalized by a hierarchical agglomerative cluster analysis using complete linkage to divide the dataset into a risk and a non-risk group (Kaufman & Rousseeuw, Citation2005). A distance-based method (discovery of structure in data sets) that groups together objects that have a smaller distance (higher similarity) to each other than to other objects, was used. In agglomerative clustering methods, each object first forms a cluster and then the clusters already formed are gradually combined into larger ones until all objects belong to one cluster (‘bottom-up’). The distance matrix was based on Euclidean distances, called the L2 norm. To validate the quality of the cluster analysis, a scree plot was used. Subsequently, descriptive analysis based on quantity (N), arithmetic mean (M), or proportion (%) + - standard deviation (SD) 2020 vs. 2022, respectively, was performed.
Furthermore, to examine the relationship between the variables, multivariate first-difference Ordinary Least Square (OLS) regression was employed in this study. Due to different variables selected as the outcome variable multivariate analysis was performed. To adress the problem of omitted variables in the two phase data, the first-differenced estimator was used. To determine the regression that best fits the data, the OLS method seeks to minimize the sum of squared residuals, which are the disparities between the observed values of the outcome variable and the predicted values obtained from the model (Wooldridge, Citation2007). OLS was chosen as the method, because it is has lowest sampling variance among unbiased estimators.
The following regressions were calculated:
DS as the outcome variable predicted by the control variables age, gender and income.
NE as the outcome variable predicted by the control variables age, gender and income.
NE as the outcome variable predicted by the independent variable of DS and the control variables age, gender and income.
PC as the outcome variable predicted by the control variables of age, gender, and income.
PC as the outcome variable predicted by the independent variable of DS and the control variables of age, gender, and income.
The five models were compared using the adjusted coefficient of determination, R². Because R-squared always increases as more predictors are added to a model, the adjusted R-squared can indicate how useful a model is, adjusting for the number of predictors in a model. For comparison of the models (e.g. to assess the independent contribution of (change in) DS to the prediction of (change in) NE or PC above and beyond the contribution of the (changes in) control variables, ΔR² is used and tested. Adjustment is made for the number of explanatory variables in the regression model, i.e. ‘penalizing’ for increasing number of variables. To avoid problems of multiple testing for multiple outcomes (i.e. α-error inflation), statistical tests are omitted in favor of 95 percent confidence intervals of the regression coefficients. The analysis was performed using R-Studio version 2022.02.2 (package prcr/olsrr).
Ethics statement
The study was conducted in accordance with the Declaration of Helsinki and was approved by an Institutional Review Board/Ethics committee. See details under Methods. The study received an exemption from an Institutional Review Board/Ethics committee.
Results
Data is available for 36 departments in 2020 (W1) and 35 departments in 2022 (W2), respectively. If only those departments are considered that were represented in both years with participating employees in data protection-compliant case numbers (n ≥ 5), 34 departments remain for the overall analysis. The total number of cases in sufficiently sized departments then includes n = 441 employees in 2020 and n = 398 employees in 2022.
Compared to the first survey, 37 percent of the respondents in the repeat survey are male and around 63 percent identify as female or gender-diverse. The proportion of men among the respondents thus decreased by 4.5 percentage points from 2020 to 2022. The respondents are 44.6 years old, so the average age has decreased almost one year compared to the sample in the first phase of the survey (2020: 45.5 years). The proportion of higher-income earners rose from 9.4–9.8 percent, which represents a slight increase (see ).
Development of digital stress, negative emotions, and physical complaints
The M and SD of the variables, the clustering was based on, can be found in . The clustering in the repeated survey W2 was done with the same method and according to the same equation as in W1 (Wrede et al., Citation2021). They reveal ambiguous time-dependent changes, stating no clear image, with no change in one direction or the other evident of the individual variables; assessed negative impact on health, constant screen work and availability among others (see ).
Table 3. Comparison of M + - SD per item used for cluster analysis between years.
The scree plots in confirm a two cluster solution in both years. The difference between the number of cases to be clustered and the fusion step, after which the distance between two observations (‘height’ on the Y-axis) increases abruptly, representing the optimal number of clusters.
Figure 2. Scree plot for the digitally stressed group 2020 and 2022.
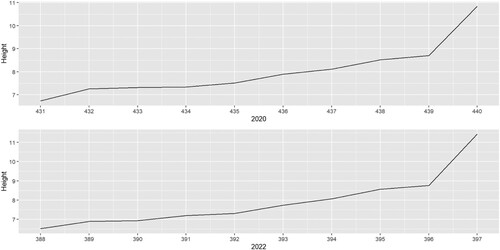
The average percentage of digitally stressed employees in a department, which is calculated in proportion to the remaining employees, has increased significantly from 8.5–20.2 percent (). At the same time, the variance of digital stress (DS) has also increased. Both, the average number of negative emotions (NE) in a department and their variance, have increased slightly between 2020 and 2022. The average number of physical complaints (PC) has increased only marginally. The average age in years has decreased slightly, while the average proportion of men has decreased noticeably. The proportion of employees in pay group E13/A13 or higher has increased marginally, while its variance has increased noticeably.
Table 4. Comparison of proportion (DS, Gender and Income) or quantity (NE and PC) or mean (Age) and SD per included variable between years.
In summary, the descriptive analyses suggest an increase of DS, NE, and PC, even if not consistently remarkable throughout the variables. Simultaneously, the difference between the most and least burdened employees in terms of the investigated conditions, seems to increase. Employees in the sample of W2 are younger, even more likely to be female than in W1. The difference between earning more and earning less increased, even though the probability within the samples to earn more than 4.000 Euro per month are marginally higher in 2022.
Impact of digital stress on negative emotions and physical complaints
demonstrates the outcomes for the multivariate analysis. As we have seen already in the descriptive analyses, the proportion of digitally stressed employees also increased significantly from 2020 to 2022 in the multivariate OLS regression model of first differences. With 95 percent probability, the change in the proportion is between four and 17 percent. Age, gender and income group can only explain around nine percent (of the variance) of this change.
Table 5. First-difference OLS regression models.
The multivariate regression model continues to show a very weak increase in the average number of NE (regression constant/intercept) when controlling for age, gender, and income. None of the variables show a significant effect (all confidence intervals include zero). Overall, only a small proportion of the change in the average number of NE in a department can be explained. If the risk factor DS is added to the regression model, the sign of the intercept changes. This means that a part of the influence on NE, which could not be attributed to the endogenous variables of the regression model previously, can now be attributed to the factor DS. However, this influence is not significant due to the overall small change in the occurrence of NE. The average number of PC decreases only marginally even after controlling for age, gender, and income group. Again, none of these variables shows a significant influence. The variance explained is low overall.
The inclusion of DS as a further regression variable does not change the aforementioned results. For the last two regression models ΔR² is two percent, which shows that the set of predictor variables does not explain much of the variation in the response variable, adjusted for the number of predictors in the model. The adjusted R-squared indicates that the fit of the regression models with the selected number of predictor variables, is not optimal.
Discussion
In this study, digital stress effects have been examined based on descriptive and multivariate regression analysis, illustrating the increasing amount or levels of digital stress for employees working in home office at least occasionally.
The study design and multivariate analysis enable reliable results, e.g. in terms of causation. In contrast to the previously published cross-sectional study based on the first survey in the project ‘Health and Digital Transformation’ (Wrede et al., Citation2021), the repeat survey avoided the risk of a transmitted variable bias. The study at hand extends the insights from the previous study with the longitudinal perspective on the development of digital stress. However, no evidence has been found for the impact of digital stress on emotions and physical complaints within the study period.
It is noticeable that the individual cluster variables show an inhomogeneous picture with regard to a change over time. At the same time, however, the proportion of digitally stressed employees has increased significantly. This can be explained by the fact that the cluster analysis was carried out at the level of all employees, but the regression analysis is at the department level. Because the average was calculated for all departments regardless of their size in order to achieve a more even representation of the population in terms of activity profiles, etc., the influence of larger departments is truncated here in contrast to the individual data analysis (i.e. cluster analysis).
The fact that the proportion of the risk cluster with regard to digital stress in 2020 is slightly lower than in (Wrede et al., Citation2021), is probably, in addition to the below mentioned reasons, due to the fact that here we filtered for employees who were already at least occasionally in the home office in 2020. In addition, the values of those employees who were in a department with fewer than five respondents were dropped.
While the cluster analysis shows an increase in digitally stressed employees from 8.5% in 2020 to 20.2% in 2022, thus 11.7% difference, the multivariate model confirms a change in the proportion (4-17%). Only around nine percent of this change can be traced back to the surveyed socio-demographic control variables, which indicates more influencing factors. Overall physical and mental well-being are associated with exercise, nutrition, communication with coworkers, children at home (incl. pandemic-induced homeschooling), distractions while working, work hours, workstation set-up and satisfaction with workspace conditions (incl. functionality of the technology), as determining factors affecting individual well-being during pandemic-induced WFH (Xiao et al., Citation2021). The wide range of influencing factors and complexity of the observed timespan marked by all the circumstances of the pandemic, home office and the digital working conditions, which are difficult to distinguish from each other, might also explain the poor fit model eventually revealed by quite low values of adjusted R-squared in this study. The poor fit model might be attributed to non-linear relationships between variables, which may suggest that alternative analytic approaches might yield more accurate insights. Yet to mention that in a corresponding multivariate study on the national level (examining the relationship between digital tools and cognitive overload among office workers during the pandemic) models including more emotional or sensational variables, such as ‘loss of joy’ or ‘tension’, achieve lower R-squareds than models including stress-related ‘demands’ and ‘worries’ (Schmitt et al., Citation2021).
We suspect a withdrawal from pervasive home office use after the pandemic. Nevertheless, the attempt of an approach for the consolidation of home offices supported through legal obligations, is important. Despite all the damage caused by SARS-CoV-2, the circumstances provided the opportunity to investigate and gain knowledge for future developments globally. These findings can serve as an orientation to guide digital implementation processes at workplaces, i.e. with regard to prevention.
Embedded in prior work: digital stress
The proportion of digitally stressed employees in this study increased even after controlling for a change in the composition of departments in terms of age, gender, and income level. Partly, this can be related to a pandemic-related expansion of digital communication. For instance, meetings that previously took place in person have been digitized, triggering the discussion of being ‘always-on’ (Messenger et al., Citation2017; Samek Lodovici et al., Citation2021). The benefits of in-person social activities at work as well as outside from work, e.g. team events and occupational health interventions, were also eliminated due to WFH mandates. The increased variance of digital stress could indicate that the pandemic effect shows different effects depending on the prior exposure to digital work conditions, and possibly hits the ‘weakest’ particularly hard. By that, a majority is not affected by digital stress initially, however, a few are possibly hit early and intensively. This would correspond to the vulnerability aspect of the diathesis-stress model (Ingram & Luxton, Citation2005).
Likewise in other pandemic-related studies, the usage of information technology, i.e. social media, is associated with more pronounced psychological strain (Bendau et al., Citation2021). Participants with pre-existing fears seem to be vulnerable for mental distress related to more immoderate media consumption. The adverse mental health effects associated with the use of new technological devices are primarily influenced by the manner in which these technologies are utilized. Prolonged usage emerges as a significant contributing factor to the development of technostress and techno-addiction (Gravelling et al., Citation2020). It should be emphasized that working with technology is not harmful per se, but the way the technology is used, can cause adverse or potentially harmful effects.
The risks to worker health primarily revolve around psychosocial factors, particularly concerning the absence from the employer's premises and limited social interactions with colleagues, which can lead to feelings of isolation. Additionally, extended managerial monitoring, constant availability expectations, and blurred boundaries between private life and work can contribute to psychosocial health and safety issues (Cabrell & Graveling, Citation2019). Intensified interference of work and home life, social isolation and loneliness, might have additionally reduced the employees’ capacity to handle changes and related increase of mental strain in the digital working context. Prior studies have recognized digital stress as a significant issue (Gimpel et al., Citation2018). Regarding the increasing specialization of technology in work environments, occupational health practitioners should consider evaluating digital stress as part of medical surveillance, particularly for at-risk individuals such as workers utilizing information and communication technologies.
Embedded in prior work: negative emotions and physical complaints
An increase in negative emotions and physical complaints cannot be confirmed and explained by digital stress in the multivariate model. In contrast to digital stress, these are outcomes of stress, whereas a time-lagged development cannot be ruled out, even though longer time already passed between the surveys. The health consequences of digital stress and the differentiation from ‘analog stress’ thus remain under-researched.
Meanwhile, studies in other settings found evidence via biometric markers for stronger stress responses in the face-to-face environment. Gellisch et al. (Citation2022) indicate that the transfer of a course to an online learning environment is associated with decreased sympathetic and enhanced vagal cardiovascular influences with lower cortisol concentrations in healthy medical students. Participants engaged in face-to-face learning showed significantly higher cortisol concentrations, but, increased sympathetic activation correlated with the discrete positive emotion of enjoyment (which enhances learning) exclusively within the analog course (Gellisch et al., Citation2022). Such specifications do not exist for the workplace setting to date.
Shahwan et al. (Citation2022) and Tahernejad et al. (Citation2022) have been investigating ergonomics to address and identify the causes of musculoskeletal pain in office workers. The setup of the workstation plays a significant role in musculoskeletal pain prevention, and factors such as ergonomic chairs, adjustable workstations, and suitable mice or screens are essential in ensuring proper ergonomics. It is important to evaluate home workstations to ensure that these meet appropriate safety standards, i.e. to reduce and prevent physical complaints.
In the hypothesis model illustrated in , there is a line between negative emotions and physical complaints as well, but due to converging evidence and the already prevailing complexity we did not examine this relation within the study project. Research drawing on theories from psychological disciplines, i.e. psychoneuroimmunology seemingly completes the triangle as it has identified relationships between negative emotions with inflammation, the vital process involved in infection clearance and wound healing (Gouin et al., Citation2015). Depression, sadness and anxiety appear to interact in predicting basal levels of inflammation. For instance, anger and hostility have been associated with increased inflammation (Graham et al., Citation2006; Marsland et al., Citation2008). Stress through negative emotions can promote a state of chronic low-grade inflammation, which has been associated with detrimental health outcomes. Although some studies suggest that inflammation may have a causal pathophysiological role, other evidence suggests that increased inflammation may reflect simply the presence of risk factors or represent a marker of disease activity (Gouin et al., Citation2015). It remains to be noted that due to ommitting the investigation of the relationship between emotions and physical complaints in this study, eventually a multivariable, i.e. multiple linear instead of a multivariate analysis has been conducted (Bunce et al., Citation2017).
Additionally, considering that the emotions in our study were all being formulated negatively, it should be kept in mind, that negative emotions cannot be stated as negative solely. In this study, we referred to chronic negative emotions by cutting off the variable at value 3, i.e. all participants who experienced the surveyed negative emotions and physical complaints more than frequently. Other findings suggest that accepting negative emotional experiences predicts positive effects, such as decreased depressive symptoms (Shallcross et al., Citation2010), which moves the regulation of emotions into the center of attention (McLean & Foa, Citation2017). When not regulated properly, negative emotions can create biological harm on the body that can increase risk for morbidity and mortality (Renna, Citation2021). In a longitudinal experience-sampling study across the adult life span, it was found that not only were frequent experiences of mixed emotions (co-occurrences of positive and negative emotions) strongly associated with relatively good physical health, but that increases of mixed emotions over many years attenuated typical age-related health declines (Hershfield et al., Citation2013). Recent findings also point to the emotional benefits associated with promoting positive emotions, such as gratitude and kindness, during the COVID-19 pandemic (Datu et al., Citation2022). A strategy of ‘taking the good with the bad’ may benefit health outcomes, emphasizing the complex interplay between positive and negative emotions and how this interplay affects physical well-being, which requires further investigation.
Limitations and future research
As the survey items were formulated in cooperation with practitioners from municipal administrations, no standardized instruments were utilized in this study. Hence, the reliability and validity of the results could be limited.
Further, it is important to note that the respondents self-assessed themselves using a mostly four-point Likert scale. Although less common than scales with an odd number of points, even-pointed Likert scales are frequently employed to elicit a non-neutral decision from respondents (Allen & Seaman, Citation2007). This potential bias was mitigated by allowing respondents the option to skip questions or choose not to answer.
Moreover, the sample may not fully represent home office settings due to two factors that could introduce selection bias: (i) the voluntary nature of participation and (ii) the requirement to include only employees from departments undergoing digital implementation processes. Consequently, it would be intriguing to determine the proportion of digitally stressed employees working from home in other countries and various economic sectors through representative surveys.
Eventually, a decrease in the number of cases of about 50 in the dataset should be noted. Following the official reception of Ukrainian refugees, there was also additional administrative work apportioned or delegated to employees included in this sample. Upon further inquiry, this resulting lack of time was cited by the project partners as by far the most frequent reason for lack of follow-up with the study. However, this would rather affect the presumably more stressed employees, which would lead to an underestimation of the stated increase of digital stress.
Due to personnel fluctuation and non-response (not the least for the prior mentioned reasons) it cannot be assumed that the study groups are stable over time. The same employees who participated in the first phase did not necessarily participate in the second phase, presumably because retirements and/or new hires took place, amongst other reasons. The nearly identical average age confirm that the samples are not fully dependent (). For this reason, the calculated standard errors should be viewed with caution. If, e.g. individuals were employed for only a few days in the repeated measurement, this would likely lead to less digital stress, negative emotions, and physical complaints. So, on the one hand, the sample composition has changed, and on the other hand, the total number of cases has decreased. The number of cases in a few departments has fallen below the threshold of n < 5 defined as relevant for data protection. Nevertheless, it is assumed that a departmental culture anchored in the company is stable over time, which is why we calculated departmental averages. Still, if the exact same sample of employees was used twice, then it would be more meaningful to predict changes in an outcome variable based on changes in predictors.
Further research should aim to test the time-sensitive delays to adopting or adjusting for psychosomatic outcomes, and, further investigate on mediators, as well as resources for and consequences of digital stress via complex multivariate causal models. Due to the subjective composition of the phenomenon, qualitative research methods might be even more informative to derive preventive measures for individual workplaces and its employees working from home.
Conclusions
Digital stress increased noticeably for employees in a working from home setting. This cannot be confirmed for psychosomatically related negative emotions and physical complaints. The results reveal just a marginal increase of these variables, whereas the impact of digital stress on emotions and physical complaints is insignificant. However, this study adds insights to the current research on stress and especially a delineation of the phenomenon of digital stress, which is still being explored in its infancy. The findings highlight the importance of addressing digital stress in occupational health assessments to better support workers in managing the potential adverse effects of technology use in the workplace. Moreover, stakeholders who design workplaces, aiming for undistracted work and employment, should take these insights into account.
Data accessibility
The dataset supporting the conclusions of this article is included within the article. Data available on reasonable request through the corresponding author SW.
Informed consent
Informed consent was obtained from all individual participants included in the study.
Acknowledgements
Author contributions: SW particularly contributed to the study conception and design. Project management, material preparation, data collection and analysis were performed by KC, DRdA, JK, and HCB supervised the project. The first draft of the manuscript was written by SW and all authors commented on previous versions of the manuscript. All authors read and approved the final manuscript.
Disclosure statement
No potential conflict of interest was reported by the author(s).
Additional information
Funding
References
- § 2 ArbStättV – Einzelnorm, 2022 [Internet]. Retrieved September 19, 2022, from https://www.gesetze-im-internet.de/arbst_ttv_2004/__2.html
- The mental health of workers in the digital era: How recent technical innovation and its pace affects the mental well-being of workers [Internet] [cited 2022 Oct 4].
- Allen, I. E., & Seaman, C. A. (2007). Likert scales and data analyses. Quality Progress, 40, 64–65. http://rube.asq.org/quality-progress/2007/07/statistics/likert-scales-and-data-analyses.html
- Barone Gibbs, B., Kline, C. E., Huber, K. A., Paley, J. L., & Perera, S. (2021). COVID-19 shelter-at-home and work, lifestyle and well-being in desk workers. Occupational Medicine, 71(2), 86–94. https://doi.org/10.1093/occmed/kqab011
- Beck, O., Berger, S., Breutmann, N., Fergen, A., Gregersen, S., Morschhäuser, M. et al. (2017). Empfehlungen zur Umsetzung der Gefährdungsbeurteilung psychischer Belastung. Arbeitsschutz in der Praxis. Hg. v. Leitung des GDA-Arbeitsprogramms Psyche. Bundesministerium für Arbeit und Soziales. Berlin. https://www.bra.nrw.de/system/files/media/document/file/broschuere-empfehlung-gefaehrdungsbeurteilung.pdf.
- Beermann, B., Amlinger-Chatterjee, M., Brenscheidt, F., Gerstenberg, S., Niehaus, M., & Wöhrmann, A. M. (2018). Orts- und zeitflexibles Arbeiten: Gesundheitliche Chancen und Risiken. Bundesanstalt für Arbeitsschutz und Arbeitsmedizin.
- Bendau, A., Petzold, M. B., Pyrkosch, L., Mascarell Maricic, L., Betzler, F., Rogoll, J., Große, J., Ströhle, A., & Plag, J. (2021). Associations between COVID-19 related media consumption and symptoms of anxiety, depression and COVID-19 related fear in the general population in Germany. European Archives of Psychiatry and Clinical Neuroscience, 271(2), 283–291. https://link.springer.com/article/10.1007s00406-020-01171-6
- Bölsch-Peterka, R., Krowicki, M., Schmidtke, A., & Böckelmann, I. (2022). Durchführung von digitalen Arbeitssituationsanalysen für die mobil-flexible Arbeit zur Erhebung von psychischen Belastungsfolgen: Befragung von Mitarbeiter*innen einer öffentlichen Verwaltung zu Homeoffice – Erfahrungen während der SARS-CoV-2-Pandemie [Carrying out digital work situation analyses for mobile flexible work to ascertain the consequences of mental stress]. Zentralblatt für Arbeitsmedizin, Arbeitsschutz und Ergonomie, 72(3), 115–128. https://link.springer.com/article/10.1007s40664-022-00460-2
- Bongers, P. M., Winter, C. d., Kompier, M. A., & Hildebrandt, V. H. (1993). Psychosocial factors at work and musculoskeletal disease. Scandinavian Journal of Work, Environment & Health, 19(5), 297–312. https://doi.org/10.5271/sjweh.1470
- Bouziri, H., Smith, D. R. M., Descatha, A., Dab, W., & Jean, K. (2020). Working from home in the time of COVID-19: How to best preserve occupational health? Occupational and Environmental Medicine, 77(7), 509–510. https://oem.bmj.com/content/oemed/77/7/509.full.pdf
- Bunce, C., Czanner, G., Grzeda, M. T., Doré, C. J., & Freemantle, N. (2017). Ophthalmic statistics note 12: Multivariable or multivariate: What's in a name? British Journal of Ophthalmology, 101(10), 1303–1305. https://doi.org/10.1136/bjophthalmol-2017-310846
- Cabrell, D., & Graveling, R. (2019). Health and safety in the workplace of the future. European Parliament. https://www.europarl.europa.eu/RegData/etudes/BRIE/2019/638434/IPOL_BRI
- Carayon, P., Smith, M. J., & Haims, M. C. (1999). Work organization, job stress, and work-related musculoskeletal disorders. Human Factors: The Journal of the Human Factors and Ergonomics Society, 41(4), 644–663. https://pubmed.ncbi.nlm.nih.gov/10774134/
- Claassen, K., Rodil dos Anjos, D., Kettschau, J. P., Wrede, S. J. S., & Broding, H. C. (2022). Gesundheit und digitaler Wandel: Abschlussbericht. https://www.uni-wh.de/fileadmin/user_upload/03_G/07_Humanmedizin/Gesundheit_und_digitaler_Wandel_E-Paper.pdf
- Datu, J. A. D., Valdez, J. P. M., McInerney, D. M., & Cayubit, R. F. (2022). The effects of gratitude and kindness on life satisfaction, positive emotions, negative emotions, and COVID-19 anxiety: An online pilot experimental study. Applied Psychology: Health and Well-Being, 14(2), 347–361. https://doi.org/10.1111/aphw.12306
- Denzer, M., & Grunau, P. (2021). The impacts of working from home on individual health and well-being: Discussion paper [Internet] Retrieved October 3, 2022, from https://download.uni-mainz.de/RePEc/pdf/Discussion_Paper_2106.pdf
- Deutscher Bundestag. (2017). Homeoffice Duden.de, 2018 Apr 27. Retrieved September 21, 2022, from https://www.duden.de/rechtschreibung/Homeoffice
- DIN EN ISO 10075-1. (2018). Ergonomische Grundlagen bezüglich psychischer Arbeitsbelastung -Teil 1: Allgemeine Aspekte und Konzepte und Begriffe. Beuth Verlag GmbH https://doi.org/10.31030/2654667.
- Dragano, N. (Ed.) (2007). Arbeit, Stress und krankheitsbedingte Frührenten: Zusammenhänge aus theoretischer und empirischer Sicht. VS Verlag für Sozialwissenschaften.
- Duden, 2018. [Internet]. Retrieved September 21, 2022, from https://www.duden.de/rechtschreibung/Homeoffice.
- Fauville, G., Luo, M., Queiroz, A. C. M., Bailenson, J. N., & Hancock, J. (2021). Nonverbal mechanisms predict zoom fatigue and explain Why women experience higher levels than Men. SSRN Electronic Journal, https://doi.org/10.2139/ssrn.3820035
- Gallo, L. C., & Matthews, K. A. (2003). Understanding the association between socioeconomic status and physical health: Do negative emotions play a role? Psychological Bulletin, 129(1), 10–51. https://doi.org/10.1037/0033-2909.129.1.10
- Gellisch, M., Wolf, O. T., Minkley, N., Kirchner, W. H., Brüne, M., & Brand-Saberi, B. (2022). Decreased sympathetic cardiovascular influences and hormone-physiological changes in response to COVID-19-related adaptations under different learning environments. Anatomical Sciences Education, 15(5), 811–826. https://anatomypubs.onlinelibrary.wiley.com/doi/10.1002ase.2213
- German Federal Government. (2022). Homeoffice überall da, wo es möglich ist [Internet]. Retrieved September 19, 2022, from https://www.bundesregierung.de/breg-de/themen/coronavirus/verordnung-zu-homeoffice-1841120
- German Federal Statistical Office (2022): Ein Viertel aller Erwerbstätigen arbeitete 2021 im Homeoffice [Internet]. Retrieved September 21, 2022, fromHYPERLINK “https://www.destatis.de/DE/Presse/Pressemitteilungen/Zahl-der-Woche/2022/PD22_24_p002.html” https://www.destatis.de/DE/Presse/Pressemitteilungen/Zahl-der-Woche/2022/PD22_24_p002.html
- German Parliament. (2017). Telearbeit und Mobiles Arbeiten: Voraussetzungen, Merkmale und rechtliche Rahmenbedingungen [Internet] Retrieved September 19, 2022, from https://www.bundestag.de/resource/blob/516470/3a2134679f90bd45dc12dbef26049977/WD-6-149-16-pdf-data.pdf.
- Gimpel, H., Bayer, S., Lanzl, J., Regal, C., Schäfer, R., & Schoch, M. (2020). Digitale Arbeit während der COVID-19-Pandemie: Eine Studie zu den Auswirkungen der Pandemie auf Arbeit und Stress in Deutschland. Augsburg: Projektgruppe Wirtschaftsinformatik des Fraunhofer FIT. https://doi.org/10.24406/FIT-N-618361
- Gimpel, H., Lanzl, J., Manner-Romberg, T., & Nüske, N. (2018). Digitaler Stress in Deutschland. Eine Befragung von Erwerbstätigen zu Belastung und Beanspruchung durch Arbeit mit digitalen Technologien. https://www.boeckler.de/pdf/p_fofoe_WP_101_2018.pdf
- Gimpel, H., Lanzl, J., Regal, C., Urbach, N., Wischniewski, S., Tegtmeier, P., Kreilos, M., Kühlmann, T., Becker, J., Eimecke, J., & Derra, N. D. (2019). Gesund digital arbeiten?! Eine Studie zu digitalem Stress in Deutschland. Augsburg: Projektgruppe Wirtschaftsinformatik des Fraunhofer FIT. https://doi.org/10.24406/fit-n-562039
- Giovanis, E., & Ozdamar, O. (2021). Implications of COVID-19: The effect of working from home on financial and mental well-being in the UK. International Journal of Health Policy and Management, https://www.ijhpm.com/article_4036_5d224430a356fb41aa1f82cc87583e37.pdf
- Gouin, J.-P., Hantsoo, L. V., & Kiecolt-Glaser, J. K. (2015). Stress, negative emotions, and inflammation. In J. Decety, & J. T. Cacioppo (Eds.), The Oxford handbook of social neuroscience (pp. 814–829). Oxford University Press.
- Gravelling, R. (2020). The mental health of workers in the digital era. How recent technical innovation and its pace affects the mental well-being of workers. European Parliament. https://data.europa.eu/doi/10.2861/986378
- Graham, J. E., Robles, T. F., Kiecolt-Glaser, J. K., Malarkey, W. B., Bissell, M. G., & Glaser, R. (2006). Hostility and pain are related to inflammation in older adults. Brain, Behavior, and Immunity, 20(4), 389–400. https://doi.org/10.1016/j.bbi.2005.11.002
- Gualano, M. R., Santoro, P. E., Borrelli, I., Rossi, M. F., Amantea, C., Daniele, A., & Moscato, U. (2023). TElewoRk-RelAted stress (TERRA), psychological and physical strain of working from home during the COVID-19 pandemic: A systematic review. Workplace Health & Safety, 71(2), 58–67. https://doi.org/10.1177/21650799221119155
- Hammes, M., & Wieland, R. (2017). Von Wirkungen auf Ursachen schließen – Psychische Beanspruchung und die Gefährdungsbeurteilung. Innsbruck Journal Psychologie des Alltagshandelns, 19–32. http://www.allgemeine-psychologie.info/wp/wp-content/uploads/2019/03/02_hammes_wieland.pdf
- Herrmann, M., & Frey Cordes, R. (2020). Homeoffice im Zeichen der Pandemie: Neue Perspektiven für Wissenschaft und Praxis?. IUBH Internationale Hochschule. https://www.econstor.eu/bitstream/10419/217267/1/1698345356.pdf.
- Hershfield, H. E., Scheibe, S., Sims, T. L., & Carstensen, L. L. (2013). When feeling bad can be good: Mixed emotions benefit physical health across adulthood. Social Psychological and Personality Science, 4(1), 54–61. https://doi.org/10.1177/1948550612444616
- Ingram, R. E., & Luxton, D. D. (2005). Vulnerability-Stress models. In B. L. Hankin, & J. R. Z. Abela (Eds.), Development of psychopathology: A vulnerability-stress perspective (pp. 32–46). Sage Publications.
- Karasek, R. A. (1979). Job demands, job decision latitude, and mental strain: Implications for job redesign. Administrative Science Quarterly, 24(2), 285. http://www.jstor.org/stable/2392498
- Kaufman, L., & Rousseeuw, P. J. (2005). Finding groups in data: An introduction to cluster analysis. Wiley.
- Kettschau, J., Claassen, K., Rodil dos Anjos, D., Wrede, S. J. S., & Broding, H. C. (2023). The influence of occasional and frequent work from home on musculoskeletal complaints. WORK: A Journal of Prevention, Assessment & Rehabilitation, 75(3), 1051–1057. https://doi.org/10.3233/WOR-220228
- Lee, A. R., Son, S.-M., & Kim, K. K. (2016). Information and communication technology overload and social networking service fatigue: A stress perspective. Computers in Human Behavior, 55, 51–61. https://yonsei.elsevierpure.com/en/publications/information-and-communication-technology-overload-and-social-netw
- Majumdar, P., Biswas, A., & Sahu, S. (2020). COVID-19 pandemic and lockdown: Cause of sleep disruption, depression, somatic pain, and increased screen exposure of office workers and students of India. Chronobiology International, 37(8), 1191–1200. https://doi.org/10.1080/07420528.2020.1786107
- Marsland, A. L., Prather, A. A., Petersen, K. L., Cohen, S., & Manuck, S. B. (2008). Antagonistic characteristics are positively associated with inflammatory markers independently of trait negative emotionality. Brain, Behavior, and Immunity, 22(5), 753–761. https://doi.org/10.1016/j.bbi.2007.11.008
- McEwen, B. S. (2010). Stress: Homeostasis, rheostasis, allostasis and allostatic load. In L. R. Squire (Ed.), Encyclopedia of Neuroscience. (pp. 557–561). Academic Press.
- McLean, C. P., & Foa, E. B. (2017). Emotions and emotion regulation in posttraumatic stress disorder. Current Opinion in Psychology, 14, 72–77. https://doi.org/10.1016/j.copsyc.2016.10.006
- Messenger, J., Llave Vargas, O., Gschwind, L., Böhmer, S., Vermeylen, G., & Wilkens, M. (2017). Working anytime, anywhere: The effects on the world of work. Publications Office of the European Union.
- Meunier, S., Bouchard, L., Coulombe, S., Doucerain, M., Pacheco, T., & Auger, E. (2022). The association between perceived stress, psychological distress, and Job performance during the COVID-19 pandemic: The buffering role of health-promoting management practices. Trends in Psychology, 30(3), 549–569. https://link.springer.com/article/10.1007s43076-021-00136-5
- Molino, M., Ingusci, E., Signore, F., Manuti, A., Giancaspro, M. L., Russo, V., Zito, M., & Cortese, C. G. (2020). Wellbeing costs of technology Use during COVID-19 remote working: An investigation using the Italian translation of the technostress creators scale. Sustainability, 12(15), 5911. https://doi.org/10.3390/su12155911
- Moretti, A., Menna, F., Aulicino, M., Paoletta, M., Liguori, S., & Iolascon, G. (2020). Characterization of home working population during COVID-19 emergency: A cross-sectional analysis. International Journal of Environmental Research and Public Health, 17(17), 6284. https://doi.org/10.3390/ijerph17176284
- Myers, D. G. (2014). Emotionen, stress und gesundheit. In D. G. Myers (Ed.), Psychologie (3rd ed., pp. 495–550). Springer.
- Niebuhr, F., Borle, P., Börner-Zobel, F., & Voelter-Mahlknecht, S. (2022). Healthy and happy working from home? Effects of working from home on employee health and Job satisfaction. International Journal of Environmental Research and Public Health, 19(3), 1122. https://doi.org/10.3390/ijerph19031122
- Oksanen, A., Oksa, R., Savela, N., Mantere, E., Savolainen, I., & Kaakinen, M. (2021). COVID-19 crisis and digital stressors at work: A longitudinal study on the Finnish working population. Computers in Human Behavior, 122, 106853. https://doi.org/10.1016/j.chb.2021.106853
- Ragu-Nathan, T. S., Tarafdar, M., Ragu-Nathan, B. S., & Tu, Q. (2008). The consequences of technostress for End users in organizations: Conceptual development and empirical validation. Information Systems Research, 19(4), 417–433. https://doi.org/10.1287/isre.1070.0165
- Reinecke, L., Aufenanger, S., Beutel, M., Dreier, M., Quiring, O., Stark, B., Wölfling, K., & Müller, K. (2017). Digital stress over the life span: The effects of communication load and internet multitasking on perceived stress and psychological health impairments in a German probability sample. Media Psychology, https://www.semanticscholar.org/paper/Digital-Stress-over-the-Life-Span%3A-The-Effects-of-a-Reinecke-Aufenanger/7b5a7c2e63ef62ef1da79021743bc9019eb4e5e1
- Renna, M. E. (2021). A review and novel theoretical model of how negative emotions influence inflammation: The critical role of emotion regulation. Brain, Behavior, & Immunity - Health, 18, 100397. https://www.sciencedirect.com/science/article/pii/S2666354621002003
- Rensing, L., Koch, M., Rippe, B., & Rippe, V. (2006). Mensch im stress: Psyche, Körper, Moleküle. Elsevier GmbH.
- Rieke, K., Rübner, K., & Speth, F. (2020). Der Morgen danach. Corona als Katalysator für digitale Transformation. Bundesverband Digitale Wirtschaft. https://www.bvdw.org/fileadmin/bvdw/upload/publikationen/data_economy/BVDW_Leitfaden_Corona_als_Katalysator.pdf
- Rossell, S. L., Neill, E., Phillipou, A., Tan, E. J., Toh, W. L., van Rheenen, T. E., & Meyer, D. (2021). An overview of current mental health in the general population of Australia during the COVID-19 pandemic: Results from the COLLATE project. Psychiatry Research, 296, 113660. https://www.sciencedirect.com/science/article/pii/S0165178120333217
- Samek Lodovici, Manuela et al. (2021). The impact of teleworking and digital work on workers and society: Special focus on surveillance and monitoring, as well as on mental health of workers [Internet]. Retrieved September 27, 2022, from European Parliament https://www.europarl.europa.eu/RegData/etudes/STUD/2021/662904/IPOL_STU(2021.
- Schmitt, J. B., Breuer, J., & Wulf, T. (2021). From cognitive overload to digital detox: Psychological implications of telework during the COVID-19 pandemic. Computers in Human Behavior, 124, 106899. https://doi.org/10.1016/j.chb.2021.106899
- Seva, R. R., Tejero, L. M. S., & Fadrilan-Camacho, V. F. F. (2021). Barriers and facilitators of productivity while working from home during pandemic. Journal of Occupational Health, 63(1), e12242. https://doi.org/10.1002/1348-9585.12242
- Shahwan, B. S., D'emeh, W. M., & Yacoub, M. I. (2022). Evaluation of computer workstations ergonomics and its relationship with reported musculoskeletal and visual symptoms among university employees in Jordan. International Journal of Occupational Medicine and Environmental Health, 35(2), 141–156. https://doi.org/10.13075/ijomeh.1896.01822
- Shallcross, A. J., Troy, A. S., Boland, M., & Mauss, I. B. (2010). Let it be: Accepting negative emotional experiences predicts decreased negative affect and depressive symptoms. Behaviour Research and Therapy, 48(9), 921–929. https://doi.org/10.1016/j.brat.2010.05.025
- Sterling, P. (2012). Allostasis: A model of predictive regulation. Physiology & Behavior, 106(1), 5–15. https://doi.org/10.1016/j.physbeh.2011.06.004
- Sterling, P., & Eyer, J. (1988). Allostasis: A new paradigm to explain arousal pathology. In S. Fisher, & J. Reason (Eds.), Handbook of life stress, cognition and health (pp. 629–649). John Wiley & Sons.
- Tahernejad, S., Choobineh, A., Razeghi, M., Abdoli-Eramaki, M., Parsaei, H., Daneshmandi, H., & Seif, M. (2022). Investigation of office workers' sitting behaviors in an ergonomically adjusted workstation. International Journal of Occupational Safety and Ergonomics, 28(4), 2346–2354. https://doi.org/10.1080/10803548.2021.1990581
- Vargas Llave, O., Mandl, I., Weber, T., & Wilkens, M. (2020). Telework and ICT-based mobile work: Flexible working in the digital age. Publications Office of the European Union.
- Wieland, R., & Hammes, M. (2014). Wuppertaler Screening Instrument Psychische Beanspruchung (WSIB) – Beanspruchungsbilanz und Kontrollerleben als Indikatoren für gesunde Arbeit. Innsbruck Journal Psychologie des Alltagshandelns. http://www.allgemeine-psychologie.info/cms/images/stories/allgpsy_journal/Vol%207%20No%201/wieland_hammes_druck.pdf
- Wooldridge, J. M. (2007). Econometric analysis of cross section and panel data. MIT Pr.
- Wörterbuch der Psychotherapie. (2000). Springer.
- Wrede, S. J. S., Rodil dos Anjos, D., Kettschau, J. P., Broding, H. C., & Claassen, K. (2021). Risk factors for digital stress in German public administrations. BMC Public Health, 21(1), 2204. https://bmcpublichealth.biomedcentral.com/articles/10.1186s12889-021-12247-w
- Xiao, Y., Becerik-Gerber, B., Lucas, G., & Roll, S. C. (2021). Impacts of working from home during COVID-19 pandemic on physical and mental well-being of office workstation users. Journal of Occupational and Environmental Medicine, 63(3), 181–190. https://www.ncbi.nlm.nih.gov/pmc/articles/PMC7934324/