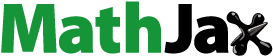
ABSTRACT
Clinical trials that study immunogenicity of combination vaccines often have less power than desirable. To make up for the reduction in statistical power at the study level, researchers have to increase the study sample size. To study immunogenicity variables, we used the geometric mean concentration of immune response after vaccination as immunologic endpoint and compared 3 sample size calculation methods: the “Inflation factors” method, the “Incrementing” method, and the “Bonferroni correction” method when there are multiple continuous co-primary endpoints. The parameters were set according to the actual situation of the use of combination vaccines and the simulation results were used as reference. The present study demonstrates that all 3 methods are applicable when the effect size of each endpoint is similar and the endpoints are at most weakly correlated, but when there is a true difference in effect sizes among endpoints, the “Incrementing” method has the best performance.
Introduction
Combination vaccinesCitation1,Citation2, which are composed of 2 or more inactivated organisms, live organisms or purified antigens, have been extensively used in recent decades as they offer protection against multiple diseases or several subtypes of bacteria or viruses and help simplify the current immunization scheduleCitation3. Owing to the multiple components in these vaccine products, they require multiple co-primary immunogenicity endpoints; for example, pneumococcal vaccination often requires 13 or 23 co-primary immunogenicity endpoints. Other multi-strain vaccines include those for human papillomavirus, rotavirus, influenza, meningococcus and poliomyletitis. Accordingly, the quality of such products might be determined by several parametersCitation4. To demonstrate the efficacy of these vaccinesCitation5, regulatory agencies often require all endpoints to be significant at the prespecified significance level. Therefore, the hypotheses regarding each serotype or component are referred to as co-primary endpoints. When the purpose of the trial is to ascertain the effectiveness of a vaccine on all of the designated co-primary variables, there is no need to adjust type I error rate. However, as described in “ICH Harmonised Tripartite Guideline for Statistical Principles Clinical Trials E9”Citation6 and “Committee for Human Medicinal Products Guideline on Multiplicity Issues in Clinical Trials”Citation7, the influence of co-primary endpoints on type II error rate should be thoroughly consideredCitation8,Citation9. To compensate for the loss of study-wide statistical power, researchers should increase the sample size. The natural question is, “what is the appropriate sample size?”
The Center for Drug Evaluation and Research (CDER) Guidance for Industry for the Evaluation of Combination Vaccines for Preventable DiseasesCitation1 recommends that the sample size required for each endpoint should be calculated separately and the largest sample size should be selected as the sample size for the overall trial. This method of calculating sample size is extensively accepted and used currently. By using this method, the loss of overall power may not be so severe when the endpoints are correlated or widely differ in effect size and variability estimates. When the endpoints are entirely independent, the overall power to attain success will be reduced to the the number of co-primary endpoints power of the prespecified study-wide statistical power. Where there are 4 mutually independent endpoints, if the type II error rate is 0.2 for each endpoint, then the overall power will be 0.84 =0.41. Thus, the likelihood of the study failing to support a conclusion of a favorable vaccine effect when such an effect exists would be 59.04%. Data extracted from previously published studies suggest that the high and low correlation levels between co-primary endpoints can range from 0.2 to 0.8 in clinical trialsCitation9. In vaccine trials, since the range of correlations between immunogenicity endpoints is still not clear, detailed discussion based on a wide range of correlation is worthwhile. Therefore, we investigated the actual data of phase II and phase III vaccine clinical trials for the purpose of summarizing the relevant patterns between endpoints and revealing the impact that multiple co-primary immunogenicity endpoints have on sample size and overall power.
Inflation of the Type II error rate in trials based on multiple co-primary endpoints has been studiedCitation8,Citation10–Citation15. Xiong et al.Citation10 explored sample size calculation in a study with 2 normally distributed continuous endpoints. Sozu et al.Citation11 extended the sample size calculation formula to a study with 3 correlated endpointsCitation8,Citation12. Sugimoto et al.Citation15 took correlations between endpoints and the ratios of effect size in multiple co-primary outcomes into consideration. These previous reports mostly focused on superiority trials with 2 or 3 co-primary endpoints. For non-inferiority clinical trials of combination vaccines when there are more than 2 endpoints, there are some other methodsCitation16,Citation17 which might be more practical. Julious et al.Citation17 provided a method of calculating sample size required for the entire study by multiplying the sample size for a single endpoint by the inflation factor. This method extends the results provided by Sankoh that explains both the number of comparisons and the correlations between endpoints for the correction of Type II error rate from five endpoints to seven endpoints. Varga et al.Citation16 presents another method which allocates more power to the groups that may have larger standardized effect sizes based on prior information. The premise of this method is the independence of endpoints. Besides these, “Bonferroni correction” is still the most popular approach used for correcting the error rate.
As far as we know, there is no previous report on sample size calculation for trials with multiple co-primary endpoints considering the nature of immunogenicity variables. In our study, we used the geometric mean concentration (GMC) of immune response after vaccination as the immunologic endpoint and discussed the following 3 methods for sample size calculation: the “Inflation factors” method, the “Incrementing” method, and the “Bonferroni correction” method.
Methods
Considering a non-inferiority vaccine trial, the objective is to determine whether the efficacy of the experimental vaccine is not worse than that of the active comparator concerning the null hypothesis. When GMC is the outcome measured in a randomized clinical vaccine trial, then the parameter of interest is the ratio of geometric means. The trial is considered to have shown benefit for all components of the vaccine if the lower limits of the confidence interval for the ratio of the experimental vaccine group to the control vaccine group is greater than the pre-specified non-inferiority margin for all endpoints.
We start with some notation. Suppose there are K (≥ 2) co-primary outcomes in a study with nT subjects in the experimental group and nC subjects in the active control group. Let V denote the post-vaccination humoral and cellular immunogenicity value, ln(Vi) (i = 1. K) are normally distributed with mean μi, variance σi2, and GMC =. The means μTi, μCi, the variances σ2Ti, σ2Ci, and the correlation coefficients ρTij, ρCij (i = 1. K, j = 1. K, i ≠ j) among endpoints are specified in advance. The vector of log-transformed immunogenicity response Yj = (Yj1, … YjK)T (j = 1. n) obeys K-variate normal distributionsCitation8,Citation18 with mean vector E[Y] = μ = (μ1. μK)T, and common covariance matrix Σ, i.e.,
where
For a single endpoint, the null (H0) and alternative (H1) hypotheses take the following forms:
H0: The experimental vaccine is inferior to the control vaccine (μT-μC≤-d).
H1: The experimental vaccine is non-inferior to the control vaccine (μT-μC >-d).
When defining the null and alternative hypotheses, d is known as the non-inferiority limit. The lower limit of the confidence interval for the difference between μT and μC should be greater than -d, which is the same as requiring the lower limit of the confidence interval for the ratio of the geometric means and
greater than λ, where d = ln(1/λ). A commonly used marginCitation19-Citation22 for the ratio is ln(1/2), which means that the experimental vaccine be at least half as efficacious as the control vaccine.
We first introduce the sample size calculation procedures we used for the non-inferiority trials and then describe the design schemes that assume the endpoints of interest to be following lognormal distribution. All the calculations and simulations were conducted using SAS (ver 9.4). The related SAS code to implement the “Incrementing” method is provided in Supplementary 3.
Sample size calculation procedure
For a trial with a single endpoint, many methods can be used to calculate the sample size for non-inferiority parallel group trials with normal dataCitation23,Citation24. Among them, the most commonly used formula is proposed by Julious et al.Citation23 as,
Here, r is the allocation ratio between groups (nT = rnC), μ is the mean, σ2 is the variances, d is the non-inferiority limit, and Z1-α/2 and Z1-β are the corresponding values for type I and type II error risks α and β, respectively, assuming a standard normal distribution.
From formula (1), if we set the allocation ratio to 1 for maximum study power, the factors that determine the sample size can be summarized as type I and II error rates, non-inferiority limit, and the effect sizes. Generally, the non-inferiority limit and the type I and II error rates are fixed. Factors that can have a direct effect on sample size are and the variance
2. For trials with multiple co-primary endpoints, denote by cij the sample size ratio of the sample size calculated by formula (1) for endpoint i to that for endpoint j (i = 1… K, j = 1… K, i ≠ j), which is,
Here, we are interested in performing hypothesis tests on the difference in GMC between the experimental arm and the control arm. Assuming equal variance and identical samples of the 2 arms, let diff = μT-μC. According to formula (1), cij could be simplified as,
Because different study endpoints may choose different non-inferiority thresholds, the choice of dj and di must always be justified on both clinical and statistical grounds.
To calculate the sample size for trials with 2 co-primary endpoints, the “Jumping method”Citation25 is frequently used. With this method, the conjunctive power is (1-β1)*(1-β2), if 1-β1 and 1-β2 represent the 2 power values assigned to the 2 endpoints. The sample size is iterated from small to large and the power of the 2 endpoints is calculated using the same sample size. The smallest sample size that makes the conjunctive power meet expectations is the sample size of the entire study. For trials with more than 2 co-primary endpoints, an improved method, the “Incrementing” method can be adoptedCitation16. With the assumption that the endpoints are mutually independent, this method assigns the power of to each endpoint and calculates the corresponding sample sizes. The smallest sample size among endpoints is used as the initial value for the iteration and the power for each endpoint is recalculated. The sample size is increased by one and the power for each endpoint is recalculated if the overall power does not reach the expected value. The iteration stops when the overall power is no less than 1-β,
This design assigns a larger power to an endpoint that has a larger effect size, which provides an efficient way of using the sample size. Another popular approach is to use Bonferroni correctionsCitation26. In order to control the family-wise type II error rate, the type II error rate for each endpoint is corrected as β/K. Although this method is conservative especially when the comparisons are correlated, it is simple and practical. The third approach of calculating the sample size for trials with multiple co-primary endpoints is provided by Julious et al.Citation17,Citation23. When the multiple co-primary endpoints are potentially correlated, the sample size for a single endpoint in clinical trials with continuous co-primary endpoints is increased by using the “Inflation factors” method. This method is an extension of the result of SankohCitation27, which considers the number of comparisons and the correlations between endpoints for the type II error rate as follows,
where,
and k is the number of comparisons, ρ is the correlation coefficient between the comparisons, β is the prespecified type II error rate, βk is the value to calculate the sample size for each arm as described in methods for single endpoint, and the values for c are described by Julious, where K ≤ 7. Thus, the increase in sample size for must-win trials compared with a single endpoint trial can be described as,
The “Inflation factor” method uses a single correlation coefficient instead of the correlation coefficient matrix between the endpoints. This assumption of equal correlation may not be true; however, it represents a pragmatic solution to the problem if the correlations are not known to differ greatly. Supplementary Table 2 shows that the coefficient of variation of the correlations between the endpoints is no more than 33%. In practice, it might be difficult to set a detailed correlation matrix before the study; therefore, a minimum estimate of correlation between endpoints should be chosen to be conservative.
Table 1. Sample size estimated to acquire an overall power of 80% or 90% at significant level α = 0.05 (2-tailed) by simulation. The proportion of sample size decreases as the correlation coefficient increases compared to r = 0 were provided in parentheses. For example, when cij = 1, r = 0.3 and K = 2, the proportion of sample size decreases was 100%×(45–44)/45 = 2.22%.
Table 2. Sample size calculated by these three methods and by simulation to acquire an overall power of 80% or 90%. The number of endpoints were 2, 5, 10 and 20, the correlation between endpoints were 0, 0.3, 0.6 and 0.9 and the cij were 1, 1.5 and 3, respectively. The ratio of the difference between sample sizes calculated by these three methods and the sample size estimated by simulation compared to the sample size estimated by simulation were provided in parentheses.
Table 3. Factors considered in the sample size calculation by these mehtods.
Simulation strategy and methods
In order to validate the methods mentioned above and to gain further insight into statistical power and correlation, the sample size calculated was compared to the sample size obtained by simulation, used as a reference. For simplicity, the allocation ratio r is set to 1 by assuming nT = nc, which is the most common case.
Simulation methods
The following steps were involved in the simulation of sample size:
Step 1: The log-transformed immunogenicity response mean vector E[Y] = μ = (μ1…μk)T, common covariance matrix Σ, and an initial sample size n is used to generate K sets of normally distributed data of 2 groups of subjects.
Step 2: Based on the simulation data, the hypothesis of non-inferiority is further tested by ANOVA and the lower limit of the 95% confidence of the difference between 2 groups is obtained.
Step 3: If all lower limits of the 95% confidence of the K co-primary endpoints are greater than the pre-defined non-inferiority limit, then the trial is marked as “success”, otherwise, the trial is labeled as “failure”.
Step 4: Steps 1–3 are repeated 1000 times and the number m of “successes” is counted.
Step 5: For the sample size as n, the overall power is m/1000.
Step 6: The sample size is slightly increased and steps 1–5 are repeated until the maximum sample size is reached.
In this study, the maximum value of the sample size calculated by formula (1) for each endpoint when the significance level is α and the predetermined power of each study is 1−β was used as an initial value in simulation. The sample size calculated with Bonferroni corrected 1-βK (βK = β/K) was used to determine the maximum sample size required to achieve the required overall power 1-β. Note that we have to repeatedly generate simulation data and testing hypothesis in order to understand the performance of these methods in different situations; at least 100 repeats for each situation should be used to endure accuracy. We have used 1000 simulations to achieve appropriate precision.
Design scheme
In order to capture the nature of immunogenicity variables and design the parameters for simulation, we analyzed the raw data of 4 combination vaccine trials. GMC, standard deviation of GMC of each immunologic endpoint in the experimental group and the control group, and the Pearson correlation coefficient among endpoints from these trials were calculated. Considering the practicality of vaccine clinical trials, the non-inferiority limit was set to 0.693 (ln(1/2)). The mean μCi was set to 3.5, and the variance σ2Ci was 1. Two-sided test was used and the family-wise type I error rate (α) was 0.05. According to the number of endpoints (K), the correlation coefficient (ρ), the sample size ratio (cij), and the type II error rate (β), there were 96 (4*4*3*2) ways of setting the parameters in this simulation study. The numbers of endpoints were taken as 2, 5, 10, and 20, corresponding to the number of actual combinations or multivalent vaccine immunogenic outcomes. The correlation coefficient between 2 different endpoints varied from 0, 0.3, and 0.6 to 0.9 as no correlation, low correlation, moderate correlation or high correlation, respectively. We assumed that cij = 1, 1.5, or 3. cij = 1 represents the case where the power for each endpoint is equal. When cij is greater than 1, the effect size of one endpoint out of K is less than that of the other endpoints. When cij = 1, μTi = μCi, diffi = 0. When cij = 1.5, for i = 1,…, K-1, μTi = μCi, diffi = 0; for i = K, μTK = 3.37, μCK = 3.5, diffK = -0.127. When cij = 3, for i = 1… K-1, μTi = μCi, diffi = 0; for i = K, μTK = 3.21, μCK = 3.5, diffK = -0.293. The family-wise type II error rate β values were set to 0.1 and 0.2.
We performed a simulation study for all of the 96 combinations of parameters described above and plotted the behavior of the overall power of different settings with increasing sample size. The “Incrementing”, “Bonferroni correction”, and the “Inflation factors” methods were used to calculate the sample size for each of these 96 cases. The results were compared with the simulation results. A deviation of more than 5% might suggest that the method is inappropriate for this situation.
Results
Behavior of overall power
We illustrated the behavior of overall power for 2, 5, 10, and 20 co-primary endpoints. Parameter cij was set to 1, 1.5, and 3. The correlation coefficient was set to 0, 0.3, 0.6, and 0.9. Two-sided tests were used, and the significance level α was 0.05. Parameter μCi was set to 3.5, and the variance σ2Ci was equal to 1. shows the overall power per group obtained by performing 1000 simulations at different sample sizes. illustrates the sample size calculated to acquire an overall power of 80% or 90%.
Figure 1. The behavior of overall power with the increasement of sample size of different settings for cij and correlation coefficient (r). The type I error rate was 0.05. The numbers of endpoints were 2, 5, 10 and 20 in each set. The range of the horizontal coordinate axis X were 20–95, 35–110 and 65–140, respectively. The sample size shown in the figure is the required sample size for a single group.
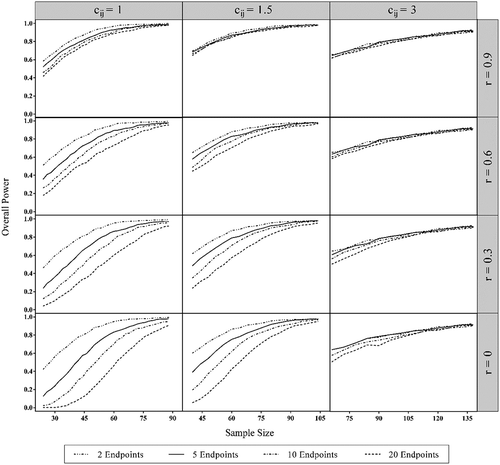
The graph clearly shows that the greater the number of co-primary endpoints, the larger is the sample size required to reach the desired overall power. also shows that the larger the correlation coefficient, the closer are the curves of different co-primary endpoints when cij is fixed. For example, when cij = 1, to acquire an overall power of 80%, trials having 20 co-primary endpoints required 33 more cases per group than trials with 2 endpoints when r = 0; however, it can be as low as 11 when r = 0.9. Moreover, as the ratio of the sample size between different endpoints increases, the curve of overall power with more co-primary endpoints approaches the curve with less co-primary endpoints. Numerically, for the cases where the endpoints are independent from each other (r = 0), 33,27 or 11 more subjects were required for trials with 20 co-primary endpoints than that for trials with 2 co-primary endpoints to obtain an overall power of 0.8 when cij was 1,1.5 or 3, respectively.
Comparison of methods
We used numerical examples to compare the 3 sample size calculation methods mentioned in the previous section. shows the sample sizes calculated using the “Incrementing” method, “Bonferroni correction” method, “Inflation factors” method, and sample size obtained by simulation. shows the factors considered in the sample size calculation by these methods. Considering the situation where the ratio of the sample size at each endpoint is the same (cij = 1), the difference between the sample size calculated by the “Inflation factors” method and the reference estimate obtained via simulation did not exceed ± 7%. The sample size obtained using the “Incrementing” method was similar to that obtained using the “Bonferroni correction” method, which did not exceed ± 9% compared with the simulation results when r ≤ 0.3. In particular, the sample sizes obtained using the “Incrementing” method and the sample size obtained using the “Bonferroni correction” method were above the simulation results when K > 2 and r > 0.3. Next, considering the case where the theoretical sample size ratio between one endpoint and the other endpoints is 1.5 (cij = 1.5), the sample size calculated by the “Bonferroni correction” method and the “Inflation factors” method was at least 14% more than the simulation result. Meanwhile, when the correlation between the endpoints was small (≤ 0.3), the sample size calculated by the “Incrementing” method did not exceed ± 6% of the sample size acquired by simulation. As the number of co-primary endpoints and the correlation between endpoints increased, the sample size calculated by the “Incrementing” method gradually exceeded the sample size required calculated by simulation. Finally, when the theoretical sample size ratio between one endpoint and the other endpoints was 3 (cij = 3), the results obtained using the “Incrementing” method were within ± 6% of the simulation results. However, the sample sizes calculated using the “Inflation factors” method or the “Bonferroni correction” method were at least 19.15% or 35.79% more than that calculated by simulation in order to achieve an overall power of 0.8.
Overall, all 3 methods were applicable when the effect size of each endpoint was similar and there was no or weak correlation between each endpoint (r ≤ 0.3); when the correlation between endpoints is strong (r ≥ 0.6), the “Inflation factors” method considers the influence of correlation between endpoints. When the effect size of individual endpoints is significantly smaller than that of other endpoints (cij ≥ 1.5), the “Incrementing” method allocates more power to this endpoint, and thus, requires a smaller sample size to achieve the desired overall power. Moreover, when the gap of effect size between the endpoints is relatively large (cij ≥ 1.5), the impact of the correlation on the overall power is almost negligible. In an intermediate case, the sample size under each method can be calculated separately, and the largest sample size can be considered.
Discussion
This article describes 3 sample size calculation methods that are appropriate for vaccine clinical studies having multiple continuous co-primary endpoints. The inflation of type II error rate in combination vaccine trials with multiple co-primary endpoints has been recognizedCitation7,Citation28–Citation32. Calculating the sample size required for each endpoint separately and selecting the maximum value as the sample size for the entire trial often underestimate the demands of sample sizeCitation1. A robust method is required for sample size calculation of vaccine trials when there are multiple co-primary endpoints. Most methods in the literature are limited to trials with either 2 or 3 co-primary endpoints or superiority trialsCitation12,Citation14,Citation15,Citation17; however, combination vaccines could have as many as 23 co-primary endpointsCitation33 and are often designed to be non-inferiority trials especially for generic products. Other methods, such as the “Bonferroni correction” method, although easy to implement in practice, tend to generate conservative sample sizes. Therefore, we have examined 3 ways of calculating the sample size for combination vaccine trials. We first introduced cij to indicate the ratio of sample sizes required for each endpoint separately in non-inferiority clinical trials. As cij could be simplified as, when it takes a value of 1, the effect sizes are expected to be similar across endpoints and the “Inflation factors” method is relatively straightforward. When cij was set to 1.5 or 3, the sample size calculated by the “Incrementing” method was closer to the simulation result than that calculated by the other methods. In addition, it is noteworthy that the simulation study was based on the assumption that the effect size of a single endpoint is less than that of the other endpoints, which is a theoretical situation. In fact, the immunogenicity results among endpoints will be moderate; therefore, the results of other approaches will be less conservative.
Based on the assumption that the effect sizes among the endpoints are roughly equal, numerous studies have been conducted; however, our analysis of the actual data found that there is a gap in immunogenicity results among the serotypes or components of combination vaccines. cij is not equal to 1 at most times. Supplementary Table 1 shows that the cij between strain A1 and strain A3 stimulated to 5.80 in the influenza A subunit vaccine trial; the cij between serotype M9V and M18C in a 23-valent pneumococcal polysaccharide vaccine trial was 2.44; the maximum value of cij among the immunogenicity endpoints of the Adsorption Free Pertussis Combined Vaccine trial was 2.3. For such trials, the “Incrementing” method can assign more statistical power to the endpoints having a larger effect size, thereby allowing the endpoint with a smaller effect size to have a lower power to achieve the desired overall power and significantly reduce the sample size. Although in the simulation study, we analyzed the behavior of sample size when the correlation among endpoints reaches 0.9, it is a rare situation in actual clinical trials. Correlation coefficients between each serotypes or components of combination vaccines do not exceed 0.6 according to Supplementary Table 2. Due to the presence of cross-immunizationCitation34, the body could develop immunity against another pathogen when vaccinated against a particular pathogen, resulting in a positive correlation among immunogenicity results. However, some bacteria or viruses do not have the cross-protection effect owing to the difference in evoking mechanism. Besides, there are many fluctuations during clinical trial conduct, and often the assumptions of treatment response are off the mark. In some cases, the correlation coefficient between the endpoints is unknown. The sample size calculation is based on the assumption that the endpoints are independent from each other. Considering the relatively small impact, for the trials with 2 or 5 co-primary endpoints, the influence of relevance on research results is negligible. However, when there are as many as 10 or more endpoints, a high correlation could cause the sample size calculated by the “Incrementing” method to slightly exceed the sample size required.
This study focused on the influence of the number of endpoints, the difference in immunological effect between the experimental group and the control group, and the correlation among the endpoints on the overall power for vaccine trials with multiple co-primary endpoints. For conservative considerations, the endpoints of each serotype of the control vaccine had the same level of immune response, and therefore, the non-inferiority margins for the different endpoints were the same. When the immune response levels of the various endpoints of the control group are different, we believe that different non-inferiority thresholds should be selected for different species. Although this does not affect the application of the 3 methods described in this paper, it affects the calculation of the parameter cij. For example, if we calculate the non-inferiority margin based on the mean differenceCitation35, the non-inferiority margin for effect retention is δ = -ε(C-P), where 1-ε is the desirable proportion of the control effect to be retained, C and P refer to the parameters of benefit for the control vaccine and the placebo, respectively. Thus, . The calculation of cij needs to fully consider the difference in immune response between the experimental group and the control group and the non-inferiority margin.
In this paper, 3 convenient methods were compared. Generally, all 3 methods were acceptable when the effect sizes among all endpoints were similar. The “Inflation factors” method was the only method that could take correlation into consideration. When there is a valid difference in effect sizes among endpoints, the “Inflation factors” method and the “Bonferroni correction” method would be conservative. Our work has been restricted to continuous endpoints in a non-inferiority clinical trial. However, this work serves as the basis for designing other types of randomized clinical trials with multiple co-primary endpoints, including superiority clinical trials. To focus on the effects of correlation on different methods, we only changed the log-transformed immunogenicity response for one endpoint in different settings; the picture on the ground is far more complicated. Moreover, the sample size calculation methods for multiple dichotomous co-primary endpoints still need further discussion since positive rates are often used as the primary endpoint in vaccine trials.
Disclosure of potential conflicts of interest
No potential conflict of interest was reported by the authors.
Supplemental Material
Download Zip (45.8 KB)Supplemental data
Supplemental data for this article can be accessed here.
Additional information
Funding
References
- U.S. Department of Health and Human Services, Food and Drug Administration, Center for Biologics Evaluation and Research. Guidance for industry for the evaluation of combination vaccines for preventable diseases: production, testing and clinical studies. 1997 April accessed 2017 Oct 21. https://wwwfdagov/downloads/biologicsbloodvaccines/guidancecomplianceregulatoryinformation/guidances/vaccines/ucm175909pdf 1997.
- Elsevier, Inc. “polyvalent vaccine”. Saunders comprehensive veterinary dictionary, 3 ed. 2007 accessed 2017 Nov. https://medical-dictionary.thefreedictionary.com/polyvalent+vaccine.
- Dodd D. Benefits of combination vaccines: effective vaccination on a simplified schedule. Am J Manag Care. 9;2003:S6–12.
- Berger RL. Multiparameter hypothesis testing and acceptance sampling. Technometrics. 1982;24:295–300. doi:10.2307/1267823.
- Chuang-Stein C, Stryszak P, Dmitrienko A, Offen W. Challenge of multiple co-primary endpoints: a new approach. Stat Med. 2007;26:1181–1192. doi:10.1002/sim.2604.
- ICH Expert Working Group. ICH harmonised tripartite guideline: statistical principles for clinical trials E9. International conference on harmonisation of technical requirements for registration of pharmaceuticals for human use. 1998 February 5 [ Accessed 2017 Mar 20. http://wwwichorg/fileadmin/Public_Web_Site/ICH_Products/Guidelines/Efficacy/E9/Step4/E9_Guidelinepdf.
- Committee for Medicinal Products for Human Use. Guideline on multiplicity issues in clinical trials (Draft). London: european medicines agency. 2016 December 15 Accessed 2018 Jan 12. http://wwwemaeuropaeu/docs/en_GB/document_library/Scientific_guideline/2017/03/WC500224998pdf.
- Sozu T, Sugimoto T, Hamasaki T,et al. Sample size determination in clinical trials with multiple endpoints. New York: Springer, 2015.
- Offen W, Chuang-Stein C, Dmitrienko A, Littman G, Maca J, Meyerson L, Muirhead R, Stryszak P, Baddy A, Chen K, et al. Multiple co-primary endpoints: medical and statistical solutions: A report from the multiple endpoints expert team of the pharmaceutical research and manufacturers of America. Drug Inf J. 2007;41:31–46. doi:10.1177/009286150704100105.
- Xiong C, Yu K, Gao F, Yan Y, Zhang Z. Power and sample size for clinical trials when efficacy is required in multiple endpoints: application to an Alzheimer’s treatment trial. Clin Trials. 2005;2:387–393. doi:10.1191/1740774505cn112oa.
- Sozu T, Kanou T, Hamada C, Yoshimura I. Power and sample size calculations in clinical trials with multiple primary variables. Jpn J Biometrics. 2006;27:83–96. doi:10.5691/jjb.27.83.
- Sozu T, Sugimoto T, Hamasaki T. Sample size determination in superiority clinical trials with multiple co-primary correlated endpoints. J Biopharm Stat. 2011;21:650–668. doi:10.1080/10543406.2011.551329.
- Sozu T, Sugimoto T, Hamasaki T. Sample size determination in clinical trials with multiple co-primary binary endpoints. Stat Med. 2010;29:2169–2179. doi:10.1002/sim.3972.
- Sozu T, Sugimoto T, Hamasaki T. Sample size determination in clinical trials with multiple co‐primary endpoints including mixed continuous and binary variables. Biometrical J. 2012;54:716–729. doi:10.1002/bimj.201100221.
- Sugimoto T, Sozu T, Hamasaki T. A convenient formula for sample size calculations in clinical trials with multiple co-primary continuous endpoints. Pharm Stat. 2012;11:118–128. doi:10.1002/pst.505.
- Varga Z, Tsang YC, Singer J. A simple procedure to estimate the optimal sample size in case of conjunctive coprimary endpoints. Biometrical J. 2016:n/a-n/a. doi:10.1002/bimj.201500231
- Julious SA, McIntyre NE. Sample sizes for trials involving multiple correlated must-win comparisons. Pharm Stat. 2012;11:177–185. doi:10.1002/pst.515.
- Nauta J. 2010. Statistics in clinical vaccine trials. New York: Springer Science & Business Media, 2010.
- Chen JJ, Yuan L, Huang Z, Shi NM, Zhao YL, Xia SL, Li GH, Li RC, Li YP, Yang SY, et al. Safety and immunogenicity of a new 13-valent pneumococcal conjugate vaccine versus a licensed 7-valent pneumococcal conjugate vaccine: a study protocol of a randomised non-inferiority trial in China. BMJ Open. 2016;6:e012488. doi:10.1136/bmjopen-2016-012488.
- Diez-Domingo J, Gurtman A, Bernaola E, Gimenez-Sanchez F, Martinon-Torres F, Pineda-Solas V, Delgado A, Infante-Marquez P, Liang JZ, Giardina PC, et al. Evaluation of 13-valent pneumococcal conjugate vaccine and concomitant meningococcal group C conjugate vaccine in healthy infants and toddlers in Spain. Vaccine. 2013;31:5486–5494. doi:10.1016/j.vaccine.2013.06.049.
- Ofori-Anyinam O, Leroux-Roels G, Drame M, Aerssens A, Maes C, Amanullah A, Schuind A, Li P, Jain VK, Innis BL. Immunogenicity and safety of an inactivated quadrivalent influenza vaccine co-administered with a 23-valent pneumococcal polysaccharide vaccine versus separate administration, in adults ≥50 years of age: results from a phase III, randomized, non-inferiority trial. Vaccine. 2017;35:6321–6328. doi:10.1016/j.vaccine.2017.09.012.
- Song JY, Cheong HJ, Hyun HJ, Seo YB, Lee J, Wie S-H, Choi MJ, Choi WS, Noh JY, Yun JW, et al. Immunogenicity and safety of a 13-valent pneumococcal conjugate vaccine and an MF59-adjuvanted influenza vaccine after concomitant vaccination in ≥60-year-old adults. Vaccine. 2017;35:313–320. doi:10.1016/j.vaccine.2016.11.047.
- Julious, Steven A. Sample sizes for clinical trials. Boca Raton: Chapman and Hall/CRC, 2009.
- Julious SA. Sample sizes for clinical trials with normal data. Stat Med. 2004;23:1921–1986. doi:10.1002/sim.1783.
- Senn S, Bretz F. Power and sample size when multiple endpoints are considered. Pharm Stat. 2007;6:161–170. doi:10.1002/pst.301.
- Ludbrook J. Multiple comparison procedures updated. Clin Exp Pharmacol Physiol. 1998;25:1032–1037. doi:10.1111/j.1440-1681.1998.tb02179.x.
- Sankoh AJ, Huque MF, Dubey SD. Some comments on frequently used multiple endpoint adjustment methods in clinical trials. Stat Med. 1997;16:2529–2542. doi:10.1002/(SICI)1097-0258(19971130)16:22<2529::AID-SIM692>3.0.CO;2-J.
- Snapinn S. Some remaining challenges regarding multiple endpoints in clinical trials. Stat Med. 2017;36:4441–4445. doi:10.1002/sim.7390.
- Sankoh AJ, Li H, D’Agostino RB. Composite and multicomponent end points in clinical trials. Stat Med. 2017;36:4437–4440. doi:10.1002/sim.7386.
- Chuang-Stein C, Li J. Changes are still needed on multiple co-primary endpoints. Stat Med. 2017;36:4427–4436. doi:10.1002/sim.7383.
- U.S. Department of Health and Human Services, Food and Drug Administration, Center for Drug Evaluation and Research (CDER), Center for biologics evaluation and research (CBER). Guidance for industry: multiple endpoints in clinical trials (draft). January 2017 Accessed 2017. https://wwwfdagov/downloads/drugs/guidancecomplianceregulatoryinformation/guidances/ucm536750pdf.
- Hamasaki T, Evans SR, Asakura K. Design, data monitoring, and analysis of clinical trials with co-primary endpoints: A review. J Biopharm Stat. 2017:1–24. doi:10.1080/10543406.2017.1378668
- Jackson LA, El Sahly HM, George S, Winokur P, Edwards K, Brady RC, Rouphael N, Keitel WA, Mulligan MJ, Burton RL, et al. Randomized clinical trial of a single versus a double dose of 13-valent pneumococcal conjugate vaccine in adults 55 through 74years of age previously vaccinated with 23-valent pneumococcal polysaccharide vaccine. Vaccine. 2018;36:606–614. doi:10.1016/j.vaccine.2017.12.061.
- Smith JW, Glorioso JC. Effect of cross-immunization on monotypic antibody responses to herpes simplex virus types 1 and 2. J Immunol. 116;1976:898–903.
- Hung HM, Wang SJ, O’Neill R. A regulatory perspective on choice of margin and statistical inference issue in non-inferiority trials. Biometrical J Biometrische Zeitschrift. 2005;47:28–36. discussion 99-107.