ABSTRACT
Immunotherapy has become a cornerstone for the treatment of renal cell carcinoma. Nevertheless, some patients are resistant to immune checkpoint inhibitors. The possibility to identify patients who cannot benefit from immunotherapy is a relevant clinical challenge. We analyzed the association between several radiomics features and response to immunotherapy in 53 patients treated with checkpoint inhibitors for advanced renal cell carcinoma. We found that the following features are associated with progression of disease as best tumor response: F_stat.range (p < .0004), F_stat.max (p < .0007), F_stat.var (p < .0016), F_stat.uniformity (p < .0020), F_stat.90thpercentile (p < .0050). Gross tumor volumes characterized by high values of F_stat.var and F_stat.max (greater than 60,000 and greater than 300, respectively) are most likely related to a high risk of progression. Further analyses are warranted to confirm these results. Radiomics, together with other potential predictive factors, such as gut microbiota, genetic features or circulating immune molecules, could allow a personalized treatment for patients with advanced renal cell carcinoma.
Introduction
Treatment options for metastatic renal cell carcinoma (RCC) are increased during the last years, allowing a relevant survival advantage.Citation1 After a long time characterized by tyrosine kinase inhibitors (TKIs) only, the treatment algorithm has been dramatically changed from the introduction of immune checkpoint inhibitors (ICIs).Citation2,Citation3 More recently, combinations of TKIs and ICIs have been demonstrated to improve the clinical outcome.Citation4–6 The benefit of ICIs has also been shown in adjuvant setting.Citation7 Therefore, immunotherapy has gained a fundamental role in the treatment of RCC, in different stages of disease and in various clinical settings. Indeed, an ICI can be employed as adjuvant therapy, in first-line metastatic disease associated with TKI or in subsequent lines of metastatic RCC. Furthermore, the combination of anti-PD-1 and anti-CTLA-4 can be considered for intermediate-poor risk patients with advanced disease.Citation3
Although the robust data supporting ICIs, no predictive factor is available to select patients who can benefit more from immunotherapy.Citation8 In addition, selected patients with favorable prognosis according to the International metastatic RCC database consortium (IMDC) could benefit from TKI.Citation9 Therefore, the possibility to choose the best therapeutic option for every patient has relevant clinical value.
In the recent years, many advances have occurred in the field of omics sciences, investigating several aspects of cell biology, growth pathways and treatment efficacy.Citation10 Among omics sciences, radiomics represents a measure of quantitative parameters extracted from medical images.Citation11 These features can be useful to define the tumor heterogeneity.Citation12–14 Emerging data highlight the role of radiomics in predicting the efficacy of immunotherapy in different tumors.Citation15–18
In this study, we evaluated the texture parameters of CT scans in patients affected by metastatic renal cell carcinoma treated with ICIs in order to explore the correlation between the radiomics features and the response to immunotherapy.
Patients and methods
Medical records of all patients with advanced renal cell carcinoma who underwent immunotherapy at Fondazione Policlinico Universitario Agostino Gemelli IRCCS were reviewed. Patients were considered if they met the following criteria: age ≥ 18 y, prevalent clear cell component, measurable disease according to Response Evaluation Criteria in Solid Tumors (RECIST) version 1.1, performance status (Eastern Cooperative Oncology Group) 0–2, no central nervous system metastases.
Staging Computed Tomography (CT) examinations were performed using a multi-detector CT (MDCT) scanner with 64 rows (Light Speed VCT XT, GE Healthcare Medical Systems, USA). The same acquisition protocol was used for all the CT series according to our institutional practice. Gross tumor volumes (GTVs) were manually contoured on portal phase of the baseline CT images using a dedicated software (Eclipse, Varian Medical Systems, USA). Response was evaluated on the images according to RECIST version 1.1.
Progression-free survival (PFS) was calculated from the first day of immunotherapy administration to progression or death for any cause. Survival was calculated from the first day of immunotherapy to death for any cause. PFS and overall survival (OS) were evaluated with the Kaplan–Meier method. The differences between patients’ subgroups were analyzed with the Log-rank test.
The radiomics analysis was performed with Moddicom, an R library compliant with the Image Biomarker Standardization Initiative.Citation19,Citation20 From all the contoured GTVs, 231 radiomics features were extracted, belonging to three main families: morphological (related to the shape of the region of interest – ROI – e.g.: volume, surface vs volume ratio, etc.), histogram-based (related to the distribution of the gray-level histogram, e.g.: mean, skewness, kurtosis, etc.) and textural (related to the visible texture of the tumor, e.g.: large zone high gray level emphasis, gray level non-uniformity, etc.).
Considering the limited sample size, in order to provide a more robust statistical test, a univariate non-parametric analysis (Mann–Whitney test) and a cross-correlation measure based on the Pearson correlation coefficient were preferred over the fitting of a parametric model (such as a proportional hazard model). Finally, the two most significant and not cross-correlated features were used to build a space to plot the distribution of the cases. All the shown p.values are the raw p.values (uncorrected) to provide transparent results. In the univariate analysis, we explored the entire set of the covariates instead of limiting the investigation on a restricted set of selected features.
The study was conducted in accordance with the Declaration of Helsinki. Informed consent was obtained from the subjects involved in the study.
Results
Fifty-three patients who started immunotherapy from September 2017 to September 2020 were considered, 32 males and 21 females. Median age was 66.8 y (range 36–87). Twenty patients received the combination nivolumab + ipilimumab in first-line setting, and 33 patients were treated with nivolumab after 1 or 2 previous systemic treatments. Patients’ characteristic are reported in .
Table 1. Patients’ characteristics.
PFS of patients treated with ICIs in first-line setting was 9.5 months () and in second/third-line setting was 7.0 months ().
Considering the whole population, 18 patients (34%) achieved an objective response (complete response and partial response) to checkpoint inhibitors, 17 (32) a disease stabilization, while 18 patients (34%) had progression as best response. In first-line setting, response rate was 50% (10 out of 20 patients) and disease control (complete response, partial response and stable disease) was 80%. In second/third line, response rate was 24.2% (8 out of 33 patients) and disease control 57.6%. Responses are described in . Survival of patients with disease control was 32.4 months, while survival of patients with progression was 11.7 months (p = .008, HR 0.41, 95% CI 0.18–0.92 – ). Considering the population receiving ICIs as first-line treatment, median survival was not reached for patients with disease control and 7.2 months for patients reporting a progression as best response (p = .06, HR 0.28, 95% CI 0.07–1.16 – ). Among the patients treated with ICIs in second-third line, the subjects obtaining a disease control had a median survival of 32.4 months, while the patients with disease progression had a survival of 11.7 months (p = .04, HR 0.44, 95% CI 0.19–1.00 – ).
Figure 2. Survival of patients with clinical benefit (solid line) or progression (dashed line). CR: complete response, PR: partial response; SD: stable disease; PD: progression of disease.
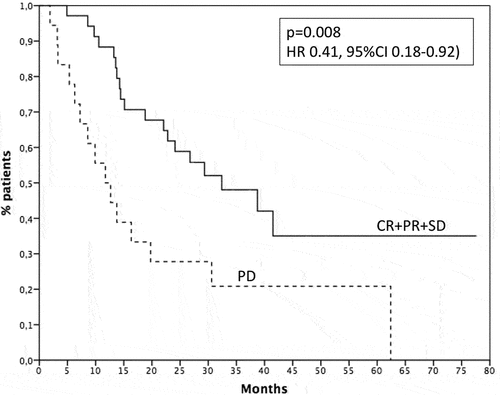
Figure 3. Survival of patients with clinical benefit (solid line) or progression (dashed line) in first (A) and second/third line setting (B). CR: complete response, PR: partial response; SD: stable disease; PD: progression of disease.
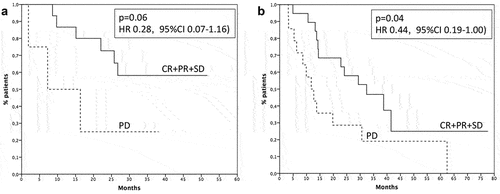
Table 2. Response rate.
Several radiomics features were associated with the progression of disease as best response: F_stat.range (p < .0004), F_stat.max (p < .0007), F_stat.var (p < .0016), F_stat.uniformity (p < .0020), F_stat.90thpercentile (p < .0050). In particular, F_stat.var and F_stat.max are poorly correlated with each other and are eligible for further qualitative bivariate investigations. The spatial distribution of the GTV in the bivariate space is shown in . F_stat.max and F_stat.var represent the highest value and the variance, respectively, in the distribution of intensity of the voxels within the ROI. Regarding F_stat_range and F_stat.uniformity, the former is the difference between the maximum and the minimum voxel value and the latter is a measure of the uniformity of the gray-level histogram. F_stat.90thpercentile indicates the 90th percentile of the gray intensity value.Citation20
Figure 4. a–b: the boxplots represent the distribution of F_stat_var and F_stat.Max between the two cohorts (DC: patients with disease control; PD: patients with progression of disease). c: Pearson correlation coefficients between the most significant features. d: the distribution of the cases (patients with disease control in blue, patients with progression of disease in red) in a space built with F_stat.Var on the x-axis and F_stat.Max on the y-axis.
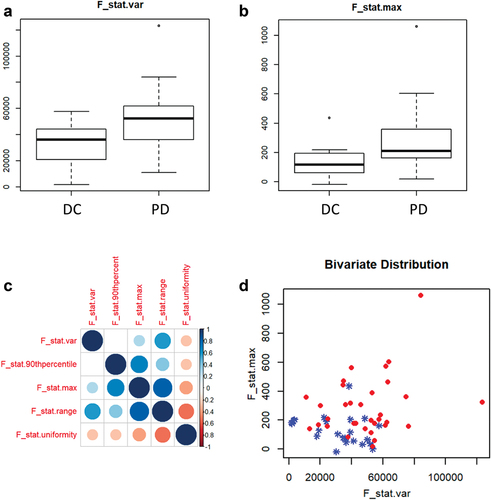
Discussion
Immune checkpoint inhibitors have become a milestone for the treatment of RCC. These agents demonstrated an improved clinical outcome in several studies. The anti-PD-1 nivolumab allowed a longer overall survival compared to everolimus in previously treated advanced RCC patients with fewer grade 3–4 adverse events.Citation2 The association of nivolumab and ipilimumab as first metastatic systemic therapy showed a longer OS and a higher response rate than sunitinib in intermediate-poor risk patients.Citation3 Of note, nivolumab + ipilimumab demonstrated a remarkable response rate and a survival advantage in patients with sarcomatoid histology.Citation21 Furthermore, the addition of anti-PD-1 to a TKI showed a benefit compared to sunitinib. The combination of pembrolizumab and axitinib allowed a 47% of reduction in death risk and 31% in disease progression compared to TKI alone.Citation4 In the CLEAR study, an improved PFS in favor of pembrolizumab-lenvatinib instead of sunitinib has been reported.Citation5 The CheckMate 9ER trial investigated the combination of nivolumab and cabozantinib versus sunitinib. It has been observed a superiority of the combination arm in terms of both OS and PFS.Citation6 ICIs can change the natural course of RCC also in adjuvant setting. Indeed, pembrolizumab showed the ability to decrease the risk of recurrence after nephrectomy.Citation7 Several further clinical trials have been conducted or are ongoing to test ICIs in combinations or as single agents in various settings of RCC. Therefore, it is probable that ICIs will increasingly become part of the treatment options for RCC.
Although more therapeutic alternatives are available, we cannot select patients who can benefit more from immunotherapy. Therefore, no predictive factor can be considered to establish if intermediate-poor patients should be candidate to anti-PD-1 plus TKI or anti-PD-1 plus anti-CTLA-4 combination. Similarly, we cannot identify patients resistant to immunotherapy, for whom other treatment options should be preferred. This is also the case of radically resected RCC patients. In this setting, it is crucial to employ an active treatment, avoiding adverse events of ineffective therapies.
Many studies have been conducted with the aim to identify predictive factors for immunotherapy efficacy.Citation22 None of them have been validated for routine use.
Our study aimed to investigate correlations between CT-based radiomics features and immunotherapy efficacy in RCC. An interesting association between some CT scan features and progression of disease in RCC patients treated with ICIs has been found. In particular, F_stat.var and F_stat.max are poorly correlated with each other and are used to build a space where the GTV associated to the different outcomes can be plotted. As shown in , the GTVs with a value of F_stat.var lower than 60,000 and F_stat.max lower than 300 are commonly associated with the absence of progression of disease, while the GTVs associated to this outcome are less clustered. According to this geometrical distribution, GTVs with high values of F_stat.var and F_stat.max (greater than 60,000 the former or greater than 300 the latter) are more probably associated to a high risk of progression of disease. Remarkably, we observed that some of the most human interpretable features correlate with the clinical outcome: F_stat.max, F_stat.var, F_stat_range, F_stat.uniformity and F_stat.90thpercentile. These radiomics features could be potentially useful for the interpretation of response to immunotherapy by trained radiologists. Despite the limitation of the sample size, we could also hypothesize that the features which are associated with disease progression during immunotherapy could be related to neoplastic tissues with low inflammatory infiltrate.Citation23–25 This aspect could justify the poor response to immunotherapy. The biological interpretation of the radiomics features is the focus of the current research.Citation26
Our results need to be confirmed with further investigations, due to the limited number of cases and the retrospective nature of the study. However, the possibility of extending these findings in clinical practice could allow to avoid ICIs in refractory patients, for whom other treatment options, such as TKIs, can lead to a longer OS. Indeed, in our study, patients resistant to ICIs reported shorter survival than patients with clinical benefit.
Basing on the above observations, it is reasonable to hypothesize that radiomics can help patients’ selection. Prospective clinical trials are needed to validate the role of the features which have been identified in our study. Taking together factors such as radiomics, circulating immune molecules,Citation27,Citation28 gut microbiota or molecular characteristics,Citation29,Citation30 a personalized treatment in RCC could be achieved.
Disclosure statement
The authors declare that they have no competing interest. ER had a role as consultant for MSD, Novartis, Pierre Fabre, Immunocore and Pfizer. GT had a role as consultant for BMS, MSD, Astra Zeneca, Servier, Merck Serono and Dompé. GS had a role as consultant for BMS.
Additional information
Funding
References
- Fluhrer H, Hutterer GC, Golbeck S, Stidl M, Niedrist T, Pichler R, Mischinger J, Seles M, Mannweiler S, Spiegelberg J, et al. Improved overall survival of metastatic renal cell carcinoma patients in the era of modern tyrosine kinase inhibitors and immune checkpoint inhibitors: results from a real-life, population-based Austrian study comprising three decades of follow-up. Ther Adv Med Oncol. 2022;14:17588359221134065. doi:10.1177/17588359221134065.
- Motzer RJ, Escudier B, McDermott DF, George S, Hammers HJ, Srinivas S, Tykodi SS, Sosman JA, Procopio G, Plimack ER, et al. CheckMate 025 investigators. Nivolumab versus everolimus in advanced renal-cell carcinoma. N Engl J Med. 2015;373(19):1803–6. doi:10.1056/NEJMoa1510665.
- Motzer RJ, Tannir NM, McDermott DF, Arén Frontera O, Melichar B, Choueiri TK, Plimack ER, Barthélémy P, Porta C, George S, et al. CheckMate 214 investigators. Nivolumab plus ipilimumab versus sunitinib in advanced renal-cell carcinoma. N Engl J Med. 2018;378(14):1277–90. doi:10.1056/NEJMoa1712126.
- Rini BI, Plimack ER, Stus V, Gafanov R, Hawkins R, Nosov D, Pouliot F, Alekseev B, Soulières D, Melichar B, et al. KEYNOTE-426 investigators. Pembrolizumab plus axitinib versus sunitinib for advanced renal-cell carcinoma. N Engl J Med. 2019;380(12):1116–27. doi:10.1056/NEJMoa1816714.
- Motzer R, Alekseev B, Rha SY, Porta C, Eto M, Powles T, Grünwald V, Hutson TE, Kopyltsov E, Méndez-Vidal MJ, et al. CLEAR trial investigators. Lenvatinib plus pembrolizumab or everolimus for advanced renal cell carcinoma. N Engl J Med. 2021;384(14):1289–300. doi:10.1056/NEJMoa2035716.
- Choueiri TK, Powles T, Burotto M, Escudier B, Bourlon MT, Zurawski B, Oyervides Juárez VM, Hsieh JJ, Basso U, Shah AY, et al. CheckMate 9ER investigators. Nivolumab plus cabozantinib versus sunitinib for advanced renal-cell carcinoma. N Engl J Med. 2021;384(9):829–41. doi:10.1056/NEJMoa2026982.
- Choueiri TK, Tomczak P, Park SH, Venugopal B, Ferguson T, Chang YH, Hajek J, Symeonides SN, Lee JL, Sarwar N, et al. KEYNOTE-564 investigators. Adjuvant pembrolizumab after nephrectomy in renal-cell carcinoma. N Engl J Med. 2021;385(8):683–94. doi:10.1056/NEJMoa2106391.
- Tucker MD, Rini BI. Predicting response to immunotherapy in metastatic renal cell carcinoma. Cancers (Basel). 2020;12(9):2662. doi:10.3390/cancers12092662.
- Rossi E, Bersanelli M, Gelibter AJ, Borsellino N, Caserta C, Doni L, Maruzzo M, Mosca A, Pisano C, Verzoni E, et al. Combination therapy in renal cell carcinoma: the best choice for every patient? Curr Oncol Rep. 2021;23(12):147. doi:10.1007/s11912-021-01140-9.
- Wang C, Lye X, Kaalia R, Kumar P, Rajapakse JC. Deep learning and multi-omics approach to predict drug responses in cancer. BMC Bioinform. 2022;22(Suppl 10):632. doi:10.1186/s12859-022-04964-9.
- Gillies RJ, Kinahan PE, Hricak H. Radiomics: images are more than pictures, they are data. Radiology. 2016;278(2):563–77. doi:10.1148/radiol.2015151169.
- Eloyan A, Yue MS, Khachatryan D. Tumor heterogeneity estimation for radiomics in cancer. Stat Med. 2020;39(30):4704–23. doi:10.1002/sim.8749.
- Henry T, Sun R, Lerousseau M, Estienne T, Robert C, Besse B, Robert C, Paragios N, Deutsch E. Investigation of radiomics based intra-patient inter-tumor heterogeneity and the impact of tumor subsampling strategies. Sci Rep. 2022;12(1):17244. doi:10.1038/s41598-022-20931-z.
- Yang G, Nie P, Yan L, Zhang M, Wang Y, Zhao L, Li M, Xie F, Xie H, Li X, et al. The radiomics-based tumor heterogeneity adds incremental value to the existing prognostic models for predicting outcome in localized clear cell renal cell carcinoma: a multicenter study. Eur J Nucl Med Mol Imaging. 2022;49(8):2949–59. doi:10.1007/s00259-022-05773-1.
- Dercle L, Sun S, Seban RD, Mekki A, Sun R, Tselikas L, Hans S, Bernard-Tessier A, Mihoubi Bouvier F, Aide N, et al. Emerging and evolving concepts in cancer immunotherapy imaging. Radiology. 2022;306(1):210518. doi:10.1148/radiol.210518.
- Chen NB, Xiong M, Zhou R, Zhou Y, Qiu B, Luo YF, Zhou S, Chu C, Li QW, Wang B, et al. CT radiomics-based long-term survival prediction for locally advanced non-small cell lung cancer patients treated with concurrent chemoradiotherapy using features from tumor and tumor organismal environment. Radiat Oncol. 2022 Nov;17(1):184. doi:10.1186/s13014-022-02136-w.
- Yu Y, Bai Y, Zheng P, Wang N, Deng X, Ma H, Yu R, Ma C, Liu P, Xie Y, et al. Radiomics-based prediction of response to immune checkpoint inhibitor treatment for solid cancers using computed tomography: a real-world study of two centers. BMC Cancer. 2022;22(1):1241. doi:10.1186/s12885-022-10344-6.
- Sun R, Lerousseau M, Briend-Diop J, Routier E, Roy S, Henry T, Ka K, Jiang R, Temar N, Carré A, et al. Radiomics to evaluate interlesion heterogeneity and to predict lesion response and patient outcomes using a validated signature of CD8 cells in advanced melanoma patients treated with anti-PD1 immunotherapy. J Immunother Cancer. 2022;10(10):e004867. doi:10.1136/jitc-2022-004867.
- Dinapoli N, Alitto AR, Vallati M, Gatta R, Autorino R, Boldrini L, Damiani A, Valentini V. Moddicom: a complete and easily accessible library for prognostic evaluations relying on image features. Annu Int Conf IEEE Eng Med Biol Soc. 2015 Aug;2015:771–74. doi: 10.1109/EMBC.2015.7318476. PMID: 26736376
- Zwanenburg A, Vallières M, Abdalah MA, Aerts HJWL, Andrearczyk V, Apte A, Ashrafinia S, Bakas S, Beukinga RJ, Boellaard R, et al. The image biomarker standardization initiative: standardized quantitative radiomics for high-throughput image-based phenotyping. Radiology. 2020 May;295(2):328–38. doi:10.1148/radiol.2020191145. Epub 2020 Mar 10. PMID: 32154773; PMCID: PMC7193906.
- Tannir NM, Signoretti S, Choueiri TK, McDermott DF, Motzer RJ, Flaifel A, Pignon JC, Ficial M, Frontera OA, George S, et al. Efficacy and safety of nivolumab plus ipilimumab versus sunitinib in first-line treatment of patients with advanced sarcomatoid renal cell carcinoma. Clin Cancer Res. 2021;27(1):78–86. doi:10.1158/1078-0432.CCR-20-2063.
- Pourmir I, Noel J, Simonaggio A, Oudard S, Vano YA. Update on the most promising biomarkers of response to immune checkpoint inhibitors in clear cell renal cell carcinoma. World J Urol. 2021;39(5):1377–85. doi:10.1007/s00345-020-03528-x.
- Aoude LG, Wong BZY, Bonazzi VF, Brosda S, Walters SB, Koufariotis LT, Naeini MM, Pearson JV, Oey H, Patel K, et al. Radiomics biomarkers correlate with CD8 expression and predict immune signatures in melanoma patients. Mol Cancer Res. 2021;19(6):950–56. doi:10.1158/1541-7786.MCR-20-1038.
- Ramlee S, Hulse D, Bernatowicz K, Pérez-López R, Sala E, Aloj L. Radiomic signatures associated with CD8+ Tumour-infiltrating lymphocytes: a systematic review and quality assessment study. Cancers (Basel). 2022;14(15):3656. doi:10.3390/cancers14153656.
- Wang JH, Wahid KA, van Dijk Lv, Farahani K, Thompson RF, Fuller CD. Radiomic biomarkers of tumor immune biology and immunotherapy response. Clin Transl Radiat Oncol. 2021;28:97–115. doi:10.1016/j.ctro.2021.03.006.
- Fournier L, Costaridou L, Bidaut L, Michoux N, Lecouvet FE, de Geus-Oei LF, Boellaard R, Oprea-Lager DE, Obuchowski NA, Caroli A, et al. European society of Radiology. Incorporating radiomics into clinical trials: expert consensus endorsed by the European society of radiology on considerations for data-driven compared to biologically driven quantitative biomarkers. Eur Radiol. 2021;31(8):6001–12. doi:10.1007/s00330-020-07598-8.
- Zizzari IG, Napoletano C, Di Filippo A, Botticelli A, Gelibter A, Calabrò F, Rossi E, Schinzari G, Urbano F, Pomati G, et al. Exploratory pilot study of circulating biomarkers in metastatic renal cell carcinoma. Cancers (Basel). 2020;12(9):2620. doi:10.3390/cancers12092620.
- Botticelli A, Pomati G, Cirillo A, Scagnoli S, Pisegna S, Chiavassa A, Rossi E, Schinzari G, Tortora G, Di Pietro FR, et al. The role of immune profile in predicting outcomes in cancer patients treated with immunotherapy. Front Immunol. 2022;13:974087. doi:10.3389/fimmu.2022.974087.
- Ianiro G, Rossi E, Thomas AM, Schinzari G, Masucci L, Quaranta G, Settanni CR, Lopetuso LR, Armanini F, Blanco-Miguez A, et al. Faecal microbiota transplantation for the treatment of diarrhoea induced by tyrosine-kinase inhibitors in patients with metastatic renal cell carcinoma. Nat Commun. 2020;11(1):4333. doi:10.1038/s41467-020-18127-y.
- McDermott DF, Huseni MA, Atkins MB, Motzer RJ, Rini BI, Escudier B, Fong L, Joseph RW, Pal SK, Reeves JA, et al. Clinical activity and molecular correlates of response to atezolizumab alone or in combination with bevacizumab versus sunitinib in renal cell carcinoma. Nat Med. 2018;24(6):749–57. doi:10.1038/s41591-018-0053-3.