Abstract
Updated household socio-economic information is necessary for planning the delivery of municipal services, particularly for cities in third world countries. The repetitive coverage of satellite imagery provides a possibility for sourcing and frequently updating information on household socio-economic conditions in urban landscapes. This paper examines the potential use of satellite imagery in inferring urban household socio-economic variables, using two high-resolution images of 2001 and 2010. Manual image interpretation was employed in deducing selected socio-economic variables that are utilised in census enumerations in South Africa, at four suburbs in Mafikeng. Of the three socio-economic variables that were examined (type of main dwelling, toilet facilities, and energy source for cooking), type of dwelling could more readily be deduced from the high-resolution imagery. Identified change in number of formal and informal houses indicated potential of satellite imagery in monitoring third world setting urban sprawl and the associated growth in informal settlements due to migration, among other factors. Satellite imagery appears useful as a supplementary source of socio-economic data to municipal authorities, for periods between regular censuses.
1. Introduction
In many third world cities, populations grow rapidly (McGill, Citation1994), often at unpredictable rates due to the diverse socio-economic causes that include high birth rates, migration, and economic factors, among others (Cohen, Citation2006). Migration driven by poverty and political strife can contribute to the growth of these city populations (Hatton & Williamson, Citation2011). Some of the political strife and poverty that can fuel migration into a given city may have origins beyond the borders of the country. Socio-economic data on the rapidly changing urban populations are important for municipal authorities to plan the delivery of services. Therefore, there is need for supplementary sources to enable frequent updates of data about the socio-economic conditions of urban households such as housing, in addition to the fixed-interval censuses. Satellite imagery could provide some of the required data at more frequent intervals than censuses, given the improvements in the ability of satellite sensors to detect and map spatial detail (i.e. spatial resolution) and frequency of imaging (i.e. temporal resolution). In this paper, this potential utility of satellite imagery is examined, using a town in South Africa.
South Africa underwent political change in the early to mid 1990’s (Crush & Dodson, Citation2007). The changes have resulted in migration into urban areas from the rural “homelands” where people were confined by state legislation under the segregative Apartheid system (Crush & Dodson, Citation2007), in search of employment (Hunter & Posel, Citation2012). The migrants have also originated from other African countries (Crush & Dobson, Citation2007; Klotz, Citation2012). One result of the migrations to urban areas has been the expansion of informal (unplanned) settlements (Hunter & Posel, Citation2012); as well large households as relatives in the urban areas accommodate the migrants, given the traditional extended family system of native (African) communities in South Africa. South African urban areas are, therefore, faced with the challenges of managing increasing informal settlements, giving rise to the difficulty of provision of timely and reliable data on the nature of the settlements as well as the associated socio-economic household data (Busgeeth, van den Bergh, Whisken, & Brits, Citation2008).
Socio-economic data are often provided by periodic ground-based (door to door) surveys. In South Africa, the state statistics agency Statistics South Africa (StatsSA) collects data on household socio-economic conditions from the public through national censuses that are conducted every 10 years. StatsSA also collects data through annual surveys such as the General Household Survey and the Labour Force Survey. In 2007, StatsSA introduced a mini census, the Community Survey 2007, aimed at obtaining socio-economic data at municipal level across the country (StatsSA, Citation2007). These fixed frequencies of provision of household socio-economic data point to the need for additional means of inferring socio-economic status of households, given the rapidity with which urban demographics can change in third world urban centres. The repetitive coverage of satellite imaging systems provides a possibility for obtaining some of the required information, which makes Remote Sensing and Geographic Information Systems (GIS) potentially useful.
Remote sensing and GIS have been widely accepted and utilised in the provision of timely information needed in urban planning and urban analysis for decision-making (Baud, Kuffer, Pfeffer, Sliuzas, & Karuppannan, Citation2010; Bishop et al., Citation2000; Jacquin, Misakova, & Gay, Citation2008; Longley, Citation2002; Martinuzzi, Gould, & González, Citation2007). Such urban planning and analysis needs remotely sensed data, which have the advantage of being cost-effective, commercially available, and not so time consuming (Hofmann, Strobl, Blaschke, & Kux, Citation2008; Schmitt, Sulzer, & Schardt, Citation1998; Tatem, Noor, & Hay, Citation2004; Ünsalan & Boyer, Citation2005). The bulk of urban remote sensing studies have mainly focused on the analysis of landscape spatial patterns as a result of urban sprawl (e.g. Durieux, Lagabrielle, & Nelson, Citation2008; Jacquin et al., Citation2008; Ji, Ma, Twibell, & Underhill, Citation2006), spatial patterns of human activities, and physical aspects of the environment (Miller & Small, Citation2003). A lot of the urban remote sensing studies tend to be based in wealthy western countries where cities are relatively well planned (Busgeeth et al., Citation2008). There are, therefore, fewer such studies relating to developing countries. Urban areas in developing countries are characterised by larger populations (Bishop et al., Citation2000) and outcrops of informal settlements that are densely populated and have smaller houses (Baud et al., Citation2010).
Integrating remotely sensed data with ancillary data from other sources can enhance the applicability of Remote Sensing in determining the nature of urban environments for use in policy formulation and research (Miller & Small, Citation2003). Early work by Rindfuss and Stern (Citation1998) indicated the benefits and challenges of integrating social data and remotely sensed data. However, even as far back as the late 1990’s, the availability of high spatial resolution satellite imagery in the future was seen as an avenue to permit the extraction of household socio-economic information (Cowen & Jensen, Citation1998). Avelar, Zah, and Tavares-Corrêa (Citation2009) integrated GIS with very high spatial resolution satellite imagery to identify socio-economic classes in Lima, Peru, based on characteristics of dwelling buildings and other physical neighbourhood elements that were analysed using supervised image classification techniques. Mertens, Sunderlin, Ndoye, and Lambin (Citation2000) showed that integrating remotely sensed data and household survey data allowed for identification of causal relationships and the processes of land cover change in south Cameroon.
The current study builds on the existing body of knowledge in the application of remote sensing in urban studies by assessing its utility as source of information on household socio-economic status. In particular, the study aimed at establishing which indicators of household socio-economic conditions could be inferred from satellite images. High-resolution satellite imagery was used as test case, in a developing country context.
2. Materials and methods
2.1. Study area
The study is set in Mafikeng, South Africa (Figure (a)). As a western-style urban settlement, Mafikeng traces its origins to the beginnings of the British colonial era of the late nineteenth and early twentieth centuries in Africa. Mafikeng is now the administrative seat of South Africa’s North West Province. Although the eastern part of the province is relatively wealthy due to the Platinum mining industry, Mafikeng is less endowed with mineral wealth. The local economy within the town is supported mainly by the retail industry, while the areas surrounding the town are predominantly agricultural.
Figure 1. Location of Mafikeng in South Africa (a), in Mafikeng Local Municipality (b) and (c) location setting of suburbs utilised in the study.
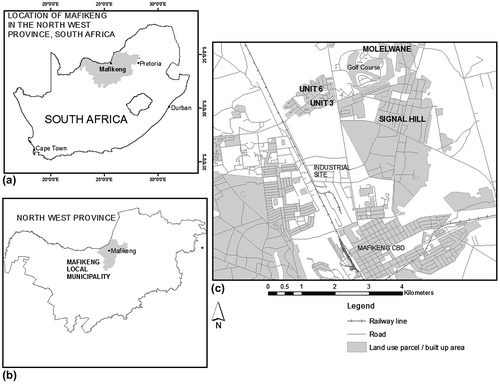
In Table , indicative demographic data for Mafikeng Local Municipality are summarised. StatsSA demographic data are available at local municipality level subdivided into wards, with none at town or suburb levels. Mafikeng Local Municipality extends beyond the actual town of Mafikeng (Figure (b)), into surrounding subsistence and commercial farm land. The commercial farms are considerably wealthy and, therefore, the data in Table do not fully indicate the levels of poverty in the town. From 242,193 in the 1996 census, the 2001 census established the population of Mafikeng Local Municipality as 259,502 (and 290,228 by 2007; StatsSA, Citation2007). Results from the 2011 census were not yet available at the time of this study.
Table 1. Selected indicative socio-economic data for Mafikeng Local Municipality in 2001 and 2007.
Four adjacent residential areas in Mafikeng were selected for the study: Molelwane, Signal Hill, Unit 3, and Unit 6 (Figure (c)). These adjacent residential areas were particularly ideal for the study because they depict a transition from high-density informal to affluent, planned residential areas. Unit 6 and Unit 3 are high- and middle-income suburbs, respectively, with low-density brick structure housing consisting of large town houses, flats, and coloured roof-tile individual houses. Roof tiles and their associated colours, therefore, can indicate household income status in the study area. Molelwane and Signal Hill are low-income suburbs, with a mixture of low- and medium-density housing. Due to the low-income levels, the houses in Molelwane and Signal Hill are small to medium in size. Some of the houses in Molelwane and Signal Hill, called “shacks”, have walls built from tin-sheet material as opposed to bricks as is the case in high-income neighbourhoods. Some building structures in these suburbs are used for commercial purposes as motor repair shops, truck service centres, textile shops, and other similar uses.
2.2. Selection of satellite image data
High spatial resolution of about 5 m was required for purposes of discriminating individual house structures using spatial socio-economic variables such as those in Table . Sensors with such high spatial resolution only became available around the year 2000 with the advent of the new generation of high spatial resolution sensors (Ikonos, OrbView, QuickBird, SPOT 5; Lillesand, Keifer, & Chapman, Citation2008). We targeted images dated as close as possible to the 2001 StatsSA census, and then newer imagery thereafter close to the 2011 census (i.e. to approximate the 10-year period of the StatsSA census period). For analysing the 2001 urban situation, a November 2001 QuickBird multispectral image with 2.62 m spatial resolution was obtained. For this image, only the green (0.466–0.620 μm; band 1), red (0.59–0.71 μm; band 2), and near-infrared (0.715–0.918 μm; band 3) bands were subsequently utilised.
Systéme Pour l’Observation de la Terre 5 (SPOT 5) imagery with high (5 m) spatial resolution is more readily available in South Africa and would have been preferred for this first date of analysis, but SPOT 5 was only launched in 2002. For the date close to the 2011 census, two SPOT 5 images of the area acquired on 14 April 2010 (scene reference K/J 137/402) were obtained from the Council for Scientific and Industrial Research Satellite Application Centre, South Africa. The images were a 2.5 m panchromatic (sensitive in the 0.48–0.71 μm range) and a 10 m multispectral image (sensitivity in green; 0.50–0.59 μm, red; 0.61–0.68 μm, and near infrared; 0.78–0.89 μm). These images were selected particularly on the basis of high spatial resolution. On a high spatial resolution of an urban area, identification of individual houses is made possible (Jacquin et al., Citation2008). Pan-sharpening, a spatial enhancement technique that utilises both the panchromatic and the multispectral images, was then employed in order to generate a multispectral image at 2.5 m spatial resolution (details in image pre-processing section). Because only spatial detail enabling the identification of building structures was important, the differences in spatial (2.62 m vs. 2.5 m) and spectral resolutions (i.e. the spectral ranges of the sensors’ bands) were judged not to introduce much error to the analysis.
2.3. Ancillary socio-economic data
Socio-economic survey data of the Mafikeng Local Municipality were obtained from the state statistics agency StatsSA. The data were obtained for purposes of comparison with the socio-economic information that could be inferred from the satellite image data. Within constraints of availability, the data were for 2001 and 2007. The 2001 data were from the 2001 census, while the 2007 data were obtained by StatsSA as part of the Community Survey 2007. The socio-economic variables selected from the data were income levels, type of dwelling, source of energy, and toilet facilities. Type of dwelling, source of energy, and toilet facilities were assessed in terms of extent to which they could be inferred on the high spatial resolution imagery. Income level on the other hand was a mere indicator, as it influences the affordability of the other three variables by households.
2.4. Image pre-processing
ERDAS Imagine 2010 was used for image processing. Image pre-processing involved geometric registration, pan-sharpening, and subset extraction. Geometric registration was necessary in order to align the SPOT 5 images (i.e. the panchromatic with the multispectral image) as well as to make all the images conform to a common projection. The Universal Transverse Mercator (UTM) WGS 84 projection was used. In the process, a number of ground control points that were widely spread within the image scenes were collected from the field using a hand-held global positioning system with an accuracy of 4 m, and subsequently utilised in the rectification process. The nearest neighbour resampling algorithm was utilised, and sub-pixel root mean square error achieved for each image.
The three bands on the multispectral image that had a spatial resolution of 10 m (i.e. the bands sensitive to green, red, and near-infrared energy) were pan-sharpened to produce a 2.5 m multispectral image using the 2.5 m SPOT 5 panchromatic image. The procedure was necessary in order to produce an image at a spatial resolution that was better than 10 m for the multispectral image. Although the panchromatic image was sufficient on the basis of its high (2.5 m) spatial resolution, the depiction of green, red, and near-infrared energy in separate bands on the multispectral image was important for assessing the households on the basis of colour. The pan-sharpening procedure has successfully been employed in a number of studies to enhance spatial detail (e.g. Baud et al., Citation2010; Durieux et al., Citation2008).
The 2001 image was then subset from the UTM-projected scene, to extract the area covering the four residential areas (Figure (a)). The pan-sharpened SPOT 5 multispectral image was similarly subset (Figure (b)).
Figure 2. Image subsets of the study area showing the residential suburbs utilised: (a) 2001 QuickBird 2.62 m resolution image (RGB: 321) (b) 2010 SPOT 5 HRG pan-sharpened (to 2.5 m resolution) image (RGB:321). The ground photo inset (Photo A, located as indicated on the 2001 image) shows a corrugated iron dwelling structure (shack) in the foreground, a pit latrine (background, centre left), brick dwelling structures (background, centre-right) and electricity power lines as indicator of source of energy for the brick structure houses. These were some of the socio-economic variables that were being inferred on the high-resolution images in (a) and (b). The slight difference in spatial extent of the images in the south-eastern portion, due to frame edge of the SPOT image in (b), did not affect the analysis since the built-up section of the Signal Hill suburb was contained in full by both images.
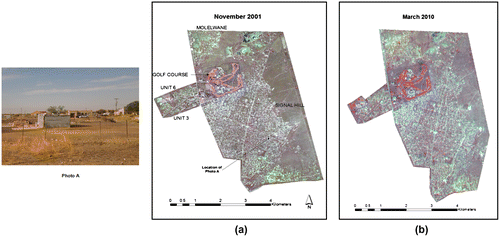
2.5. Inferring household socio-economic conditions on imagery
From the spectrum of socio-economic variables that StatsSA utilises in census and community survey enumerations, we targeted the variables that have a spatial component. We judged such variables as being likely to be identified on high spatial resolution satellite imagery. We, therefore, sought to infer three of the socio-economic variables in Table on the high spatial resolution images, with the exception of household income which (having no spatial characteristic) cannot easily be quantified directly from an image. The variables we sought to infer, therefore, were type of dwelling, source of energy, and toilet facilities. These were inferred for each of the four suburbs under study by placing shapefiles demarcating the suburb extents (as in Figure (c)) on the image subsets in Figure (a) and (b) and studying the image scene enclosed by the respective suburb boundaries, as illustrated in Figure . Three analysts independently made the respective inferences, and their independent results were collated in order to arrive at a value. For the inferences, we employed visual interpretation using image interpretation elements such as feature size, shape, pattern, texture, tone (colour), location, and association. The theory of these elements is explained in remote sensing texts (e.g. Lillesand et al., Citation2008). They were utilised as follows for the three variables under study:
Figure 3. Illustration of interpretation of type of main dwelling (brick houses on separate stands (plots) and blocks of flats) for the suburbs Unit 3 and part of Unit 6 (see Figure (c) for location) on the 2001 image in Figure (a) (with healthy vegetation depicted as green). The houses and blocks of flats were recognised on the basis of interpretation elements like tone (colour), shape, size (see text for explanation).
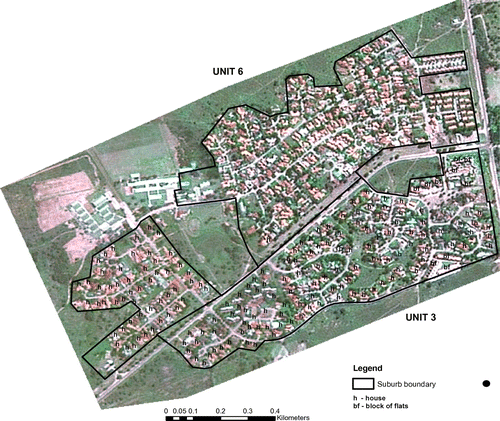
2.5.1. Type of main dwelling
The building material for a dwelling structure (particularly tin material) was discernible on the high spatial resolution images in Figure , at high magnification. Though not clear at the scale of the images in Figure (a) and (b), at magnified scales in the ERDAS viewer, the individual houses could be discerned and counted.
The high reflectance from the tin dwelling structures (shacks) gave them a light tone that differentiated them from the tile and asbestos roofing of brick houses.
At high scale, it was possible to tell a house on a separate stand (plot) on the basis of the shape of the plot (yard; see Photo A in Figure ) as well as tone (colour) of roofs (Figure ) and asphalt.
Traditional dwellings (huts) are round in shape, with thatched roofing that have a distinctive dull tone.
Blocks of flats could be identified on the basis of size and shape: they were larger than the ordinary house on a separate yard (Figure ) and also more elongated (rectangular). The occurrence of large asphalt surfaces, interpreted as car parks, next to these structures also gave further clues to their identities. However, it was not possible to count the number of households in a block of flats or a semi-detached house and, therefore, no counts of these sub-categories were possible.
Backyard residential quarters can, in theory, be identified on the basis of their location next to the larger main house. They can be differentiated from pit latrines on the basis of size; they are larger than the backyard pit latrine. We, therefore, included this variable initially on this theoretical basis.
Other types of dwellings (caravan, boat, tent, etc.) could not be identified by image interpretation.
2.5.2. Toilet facilities
Pit latrines could be identified visually on the images as being the extra small structures beside the main house dwelling structure. Their absence in the large, low-density neighbourhoods indicated the use of flush toilets. However, it was not possible to tell whether the flush toilet facilities were of septic tank or sewerage connection systems. No other categories of toilet facility as in Table (i.e. chemical, bucket, etc.) could be identified on the images.
2.5.3. Household source of energy
We sought to identify electricity as a source of household energy by identifying the power lines (see Photo A in Figure ), as well as solar panels as dark tone square/rectangular features either on rooftops or beside the dwelling structures. This proved challenging in reality, because the power lines could not easily be discerned. The solar panel structures were very rare.
The interpretation was then validated by visits to 75 randomly selected locations, and established to be accurate for 91% of the locations. The field coordinates of the locations were generated within ERDAS, as a pure random sample of buildings on the respective classified images that had buildings and asphalt as classes, among other classes (healthy vegetation, dry vegetation, bare dry surface, water, as the other classes).
3. Results
Table shows the socio-economic variable data that were inferred from the satellite images on the two dates in 2001 and 2010. The results can be analysed per suburb, given the differences in rates of change between the formal suburbs (Unit 3 and Unit 6) and the two suburbs of semi-informal origin (Signal Hill and Molelwane).
Table 2. Socio-economic data for the four suburbs under study (Unit 3, Unit 6, Signal Hill, and part of Molelwane) inferred from the high spatial resolution imagery of 2001 and 2010 utilised in the study (Figures (a) and (b)).
3.1. Type of main dwelling
For Unit 3, a formal and affluent suburb, 112 houses on separate plots (stands) were identified on the 2001 image. This number stayed the same on the 2010 image. For Unit 6, also a formal and quite affluent suburb, the number increased from 260 in 2001 to 278 in 2012 (an increase of 7%). As is evident in Figures (a) and (b) and 3, Unit 6 had vacant portions of land in 2001, on which new housing had developed by 2010. However, being a formal and affluent suburb, this growth in housing was restricted to within the boundaries of the suburb. There were no traditional huts or “shacks” in these affluent suburbs on the image dates. The number of blocks of flats was established to have been 10 and 55, respectively, for Unit 3 and Unit 6 on both image dates. Semi-detached houses proved difficult to separate from detached houses and, therefore, could not be identified on the images for any of the suburbs.
For the semi-informal suburb of Signal Hill, the number of houses on separate plots was established as 117 on the 2001 and 398 on the 2010 image, an increase of 240%. In the portion of Molelwane covered by the images, the change that was established in number of houses was from 106 on the 2001 to 191 on the 2010 image (an increase of 80%). The increase in number of formal brick houses at Signal Hill was accompanied by a large decrease in number of informal dwellings (shacks), from 668 to 479 (−28%). For the portion of the images covering Molelwane, however, a 126% increase in number of shacks was established, from 43 to 97. In reality, however, the decrease in number of shacks at Signal Hill masks the fact that there was an increase in the total number of residential units (formal brick houses and shacks combined), from 785 on the 2001 to 877 on the 2010 image, an increase of about 12% (Figure ). This increase indicates an expansion of this settlement due to new informal residential structures. For the portion of Molelwane covered by the images, there was also an increase in the total number of residential units (formal brick houses and shacks combined), from 149 to 288.
Figure 4. Illustration of changes in number of residential units for the semi-informal suburbs of Signal Hill and Molelwane as established from the 2001 and 2010 high spatial resolution satellite images in Figure . The figures for Molelwane are for the section covered by the images only, since some parts of this suburb were not covered by the images.
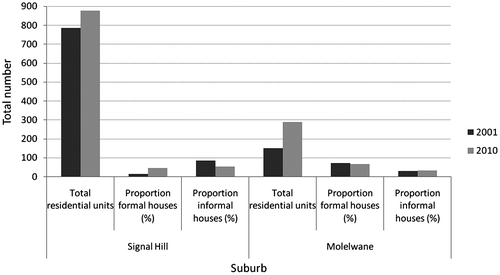
Backyard informal dwellings (shacks) could not be distinguished from non-backyard shacks for either Signal Hill or Molelwane. Other types of dwelling (boat, caravan, tent, etc.) were also not discernible on the images.
3.2. Toilet facilities
Whereas pit latrines could easily be identified and counted on the images, sewerage-connected and septic tank flush toilets could only be deduced. Field visits to Unit 3 and Unit 6 indicated that the houses in these affluent suburbs generally have flush toilets, but whether septic tank or sewerage connected was more difficult to establish. However, there was evidence of a sewerage system in these suburbs during the field visits. Therefore, the total number of houses on separate plots that was established for Unit 3 and Unit 6 also gave the total number of sewerage-connected flush toilets (see Table ). For Signal Hill, 354 pit latrines were counted on the 2001 and 111 on the 2010 image (against totals of 785 and 877 housing units, respectively). This 69% reduction mirrors the increase in number of formal houses on separate stands, with formal houses utilising septic tank systems. The corresponding numbers of pit latrines for the portions of the images covering Molelwane were established as 29 and 53 for 2001 and 2010, respectively. The increase is a reflection of the increase in number of informal dwelling structures (shacks) in the area.
3.3. Household source of energy
Identifying electricity lines (depicted on Photo A in Figure ) and solar panels proved difficult at the spatial resolution of the images that were used. However, most households in South Africa, including rural villages, are electrified owing to a vigorous government policy of electrification (Bekker, Eberhard, Gaunt, & Marquard, Citation2008). Therefore, all formal brick houses that were identified on the images, both in the affluent and the semi-informal suburbs Signal Hill and Molelwane, were inferred to have electricity (Table ).
4. Discussion and conclusion
A lot of migration into urban centres from the villages that constituted the majority of the settlements in the homelands under the Apartheid system in South Africa has occurred since the system ended in 1994. Growth in the size of the informal settlements that have sprung up in urban centres is one of the consequences of the migrations. The migrants often have little to no formal income, and can only afford to construct the informal dwellings made out of corrugated iron, called shacks (see Photo A in Figure ). Mafikeng is experiencing this phenomenon, and the results from this study indicate this, although only for a section of the town.
The rate at which informal housing springs up in many urban areas in South Africa often outstrips the abilities of the local authorities to provide services such as running water and refuse collection and disposal. For Signal Hill, a large low-income suburb that emerged originally as in informal settlement, this study established an estimated growth rate of about 12% in the number of housing units (formal and informal combined) in 9 years (2001–2010). In a municipality such as Mafikeng Local Municipality where the proportion of the population with no income actually increased from 27.6 to 63% between 2001 and 2007 (Table ), such rates of increase in urban housing put added social and economic pressures on the urban landscape, for example, increases in vices such as crime. Municipality budgets are unlikely to cope with such rapidity of change, because the municipalities often work on annual budgets and 5–10-year development plans.
The trends in the socio-economic variables that were inferred from the satellite images of 2001 and 2010 somewhat fit in the overall scenario at local municipality level established from the data in Table for the period 2001–2007 using data from the state statistics agency StatsSA. For the whole of Mafikeng Local Municipality, the number of informal dwellings (shacks) increased from 6.4 to 11.7% in the seven years. For the sections of the suburbs covered by the images in this study, the total number of shacks decreased from a total of 711 on the 2001 to 576 on the 2010 image (for all suburbs in Table ). The decrease is because Signal Hill and Molelwane, which are developing and relatively new suburbs that emerged as informal settlements, are now being formalised. This was judged from the fact that municipal services such as schools and tarred roads have been constructed over the years. As a result, brick houses are replacing shacks and pit latrines are gradually being replaced by flush toilets. At the level of Mafikeng Local Municipality, however, new informal houses (the shacks) are emerging on the urban fringe due to continued migration into the urban area. This partly accounts for the decrease in the proportions of households living in the formal housing categories of house/brick structure on separate plot, block of flats, and semi-detached house between 2001 and 2007 in Table . Although the actual number of housing units in these respective categories may not have decreased between 2001 and 2007, the rise in number of households due to migration into the urban fringe in Mafikeng could have lowered the actual proportions (percentages) of households in the respective categories between 2001 and 2007. The increase in number of non-ventilated pit latrines between 2001 and 2007 in Mafikeng Local Municipality (Table ) can also be attributed to the increase in number of informal dwellings.
The results from this study indicate that remotely sensed imagery can serve as data source for inferring socio-economic variables of a spatial nature in urban areas in the developing country context, as has been established by similar studies (e.g. Busgeeth et al., Citation2008; Sudhira, Ramachandra, & Jagadish, Citation2004). In the South African context, data on the type of dwelling can potentially be obtained from high spatial resolution satellite images by the municipal authorities using relatively inexpensive manual image interpretation techniques as employed in this study. The data can enable the planning of municipal services without utilising data from the previous census that can underestimate the demand for the service in question, or having to wait for the next census for more updated data. A plausible limitation is that the number of households per dwelling cannot readily be deduced. In many third world cities, the high cost of living can result in multiple households per dwelling. In this study, this limitation is demonstrated by the difficulty in determining the number of households in a block of flats or a semi-detached house. In some developing countries, the cost of satellite images and the associated hardware and software can be a hindrance.
High spatial resolution images from orbiting environmental satellites, such as those utilised in this study, are increasingly being available since the last decade. Local authorities in third world countries need to take advantage of this availability, for purposes of obtaining inferences on socio-economic conditions in urban areas that are experiencing rapid demographic change. Manual interpretation techniques appear workable for providing data for inferences on spatial aspect-related socio-economic variables, if supported by knowledge of the local contexts of the urban landscape.
Acknowledgements
The authors acknowledge financial support from North West University (NWU), who granted a postgraduate bursary to Gaolatlhe Motholo tenable at the Mafikeng campus of NWU.
References
- Avelar, S., Zah, R., & Tavares-Corrêa, C. (2009). Linking socioeconomic classes and land cover data in Lima, Peru: Assessment through the application of remote sensing and GIS. International Journal of Applied Earth Observation and Geoinformation, 11, 27–37.10.1016/j.jag.2008.05.001
- Baud, I., Kuffer, M., Pfeffer, K., Sliuzas, R., & Karuppannan, S. (2010). Understanding heterogeneity in metropolitan India: The added value of remote sensing data for analyzing sub-standard residential areas. International Journal of Applied Earth Observation and Geoinformation, 12, 359–374.10.1016/j.jag.2010.04.008
- Bekker, B., Eberhard, A., Gaunt, T., & Marquard, A. (2008). South Africa’s rapid electrification programme: Policy, institutional, planning, financing and technical innovations. Energy Policy, 36, 3125–3137.10.1016/j.enpol.2008.04.014
- Bishop, I., Escobar, F., Karuppannan, S., Suwarnarat, K., Williamson, I., Yates, P., & Yaqub, H. (2000). Spatial data infrastructure for cities in developing countries: Lessons from the Bangkok experience. Cities, 17, 85–96.10.1016/S0264-2751(00)00004-4
- Busgeeth, K., van den Bergh, F., Whisken, J., & Brits, A. (2008). Potential application of remote sensing in monitoring informal settlements in developing countries where complimentary data does not exist. In L. Liu, X. Li, K. Liu, & X. Zhang (Eds.), Geoinformatics 2008 and joint conference on GIS and built environment: Classification of remote sensing images (pp. 314–328). Johannesburg: Planning Africa Conference.
- Cohen, B. (2006). Urbanization in developing countries: Current trends, future projections, and key challenges for sustainability. Technology in Society, 28, 63–80.10.1016/j.techsoc.2005.10.005
- Cowen, D. J., & Jensen, J. R. (1998). Extraction and modelling of urban attributes using remote sensing technology. In D. Liverman, E. F. Moran, R. R. Rindfuss, & P. C. Stern (Eds.), People and pixels (pp. 164–188). Washington, DC: National Academy Press.
- Crush, J., & Dodson, B. (2007). Another lost decade: The failures of South Africa’s post-apartheid migration policy. Tijdschrift voor Economische en Sociale Geografie, 98, 436–454.10.1111/tesg.2007.98.issue-4
- Durieux, L., Lagabrielle, E., & Nelson, A. (2008). A method for monitoring building construction in urban sprawl areas using object-based analysis of SPOT 5 images and existing GIS data. ISPRS Journal of Photogrammetry & Remote Sensing, 63, 399–408.
- Hatton, T. J., & Williamson, J. G. (2011). Are third world emigration forces abating? World Development, 39, 20–32.10.1016/j.worlddev.2010.05.006
- Hofmann, P., Strobl, J., Blaschke, T., & Kux, H. J. (2008). Detecting informal settlements from QuickBird data in Rio de Janeiro using an object-based approach. In T. Blaschke, S. Lang, & G. J. Hay (Eds.), Object-based image analysis (pp. 531–553). New York, NY: Springer.10.1007/978-3-540-77058-9
- Hunter, M., & Posel, D. (2012). Here to work: The socioeconomic characteristics of informal dwellers in post-apartheid South Africa. Environment and Urbanization, 24, 285–304.10.1177/0956247811433537
- Jacquin, A., Misakova, L., & Gay, M. (2008). A hybrid object-based classification approach for mapping urban sprawl in periurban environment. Landscape and Urban Planning, 84, 152–165.10.1016/j.landurbplan.2007.07.006
- Ji, W., Ma, J., Twibell, R. W., & Underhill, K. (2006). Characterizing urban sprawl using multi-stage remote sensing images and landscape metrics. Computers, Environment and Urban Systems, 30, 861–879.10.1016/j.compenvurbsys.2005.09.002
- Klotz, A. (2012). South Africa as an immigration state. Politikon, 39, 189–208.10.1080/02589346.2012.683939
- Lillesand, T. M., Keifer, R. W., & Chapman, J. W. (2008). Remote sensing and image interpretation. New York, NY: Wiley.
- Longley, P. A. (2002). Geographical information systems: Will developments in urban remote sensing and GIS lead to ‘better’ urban geography? Progress in Human Geography, 26, 231–239.10.1191/0309132502ph366pr
- Martinuzzi, S., Gould, W. A., & González, O. M. R. (2007). Land development, land use, and urban sprawl in Puerto Rico integrating remote sensing and population census data. Landscape and Urban Planning, 79, 288–297.10.1016/j.landurbplan.2006.02.014
- McGill, R. (1994). Integrated urban management: An operational model for third world city managers. Cities, 11, 35–47.10.1016/0264-2751(94)90047-7
- Mertens, B., Sunderlin, W. D., Ndoye, O., & Lambin, E. F. (2000). Impact of macroeconomic change on deforestation in south Cameroon: Integration of household survey and remotely-sensed data. World Development, 28, 983–999.10.1016/S0305-750X(00)00007-3
- Miller, R. B., & Small, C. (2003). Cities from space: Potential applications of remote sensing in urban environmental research and policy. Environmental Science & Policy, 6, 129–137.10.1016/S1462-9011(03)00002-9
- Rindfuss, R. R., & Stern, P. C. (1998). Linking remote sensing and social science: The need and the challenges. In D. Liverman, E. F. Moran, R. R. Rindfuss, & P. C. Stern (Eds.), People and pixels (pp. 1–27). Washington, DC: National Academy Press.
- Schmitt, U., Sulzer, W., & Schardt, M. (1998). Analysis of settlement structure by means of high resolution satellite imagery. In D. Fritsch, M. Englich, & M. Sester (Eds.), Between visions and applications (Vol. 32, pp. 557–562). Stuttgart: ISPRS Commission IV Symposium on GIS.
- StatsSA. (2007). Community survey 2007: Statistical release basic results – municipalities. Pretoria: Statistics South Africa.
- Sudhira, H. S., Ramachandra, T. V., & Jagadish, K. S. (2004). Urban sprawl: Metrics, dynamics and modelling using GIS. International Journal of Applied Earth Observation and Geoinformation, 5, 29–39.10.1016/j.jag.2003.08.002
- Tatem, A. J., Noor, A. M., & Hay, S. I. (2004). Defining approaches to settlement mapping for public health management in Kenya using medium spatial resolution satellite imagery. Remote Sensing of Environment, 93, 42–52.10.1016/j.rse.2004.06.014
- Ünsalan, C., & Boyer, K. (2005). A system to detect houses and residential street networks in multispectral satellite images. Computer Vision and Image Understanding, 98, 423–461.10.1016/j.cviu.2004.10.006