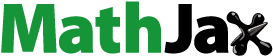
Abstract
In this paper, litchi freshness rapid non-destructive evaluating method using electronic nose (e-nose) and non-linear stochastic resonance (SR) was proposed. EN responses to litchi samples were continuously detected for 6 d Principal component analysis (PCA) and non-linear stochastic resonance (SR) methods were utilized to analyze EN detection data. PCA method could not totally discriminate litchi samples, while SR signal-to-noise ratio (SNR) eigen spectrum successfully discriminated all litchi samples. Litchi freshness predictive model developed using SNR eigen values shows high predictive accuracy with regression coefficients R2 = 0 .99396.
Introduction
Litchi (Litchi chinensis Sonn) belongs to subtropical fruit. Its main produce areas in China include Guangxi, Guangdong, Fujian, Hainan, Taiwan, etc. This fruit is well known for its abundant nutrition (such as vitamin, sugar, amino acid, protein, etc), delicious taste and the function of beauty as well as anticancer effect.Citation1-3 After harvest, litchi still precedes metabolism reactions by consuming its internal nutrition substances. What's more, microbial growth and multiplication also influence litchi's inner structure. Fruit quality declines even decay, leading to food safety risks.Citation4,5 With the improvement of living standard, people's requirements for fruit quality highly improved. Neog et al as well as Ruenroengklin et al successfully examined litchi's physicochemical indexes (such as PPO specific activity, total phenols, pericarp browning, etc) to monitor litchi's freshness change using a UV-VIS spectrometer.Citation6,7 Liang et al determined litchi's browning and decay rate by traditional measuring method.Citation8 Sun et al analyzed the contents of major PPO substrates for enzymatic browning using HPLC and measured total phenol content using a UV spectrophotometer to obtain litchi fruit's quality change.Citation9 However, these methods cover some severe drawbacks, such as long detecting period, strict operating requirements, high detecting cost, etc.
E-nose concept is firstly proposed in 1982. E-nose imitates human's olfactory function and measures samples with sensor array combined with pattern recognition system. In the past 2 decades, it has been widely applied in food area, such as pork meat TVB-N evaluation,Citation10 non-destructive red onion flavor evaluation,Citation11 pork adulteration analysis in minced mutton,Citation12 cherry tomato juices adulteration detection,Citation13 discrimination of Chinese green tea,Citation14 etc. The species and content of volatile substances in fruits and vegetables are extremely different at different ripen period. Hence, the method based on e-nose is hopeful to realize the assessment of sample's ripeness and freshness by detecting and analyzing its volatile substances using some pattern recognition approaches. Previous studies have reported some successful applications about e-nose in fruit freshness determination.Citation15-17
In this paper, litchi freshness rapid non-destructive evaluating method using e-nose was studied. PCA method could not discriminate all litchi samples with different storage time, while SNR spectrum successfully discriminated all samples. And litchi freshness predictive model was built by linear fitting Max-SNR eigen values. The proposed model presents high predicting accuracy with high regression coefficients R2 = 0.99396.
Results and Discussions
E-nose measurement result
E-nose sensor original responses to litchi samples are displayed in . The volatile gases existing in the headspace of samples are inhaled into EN gas chambers and sensed by the functional materials settled in gas sensors. All sensors' initiative responses to litchi samples are close to zero. Sensors' responses gradually increase and finally reach their stable values. S1, S4 and S3 have big response values, and their final steady values are about 0.80, 0.60 and 0.40 V, respectively. S5 and S6 have a similar response value about 0.25 V, while the rest 3 sensors (S2, S7 and S8) present low response values near zero.
PCA analysis result
The PCA analysis result of litchi samples is displayed in . Five sensors including S1, S3, S4, S5 and S6 were chosen and their final stable response values were set as eigen values to represent litchi sample's freshness. The first principal components (PC1) and the second principal components (PC2) capture 82.22% of data variance. It is apparent that this method can well classify litchi samples in the first 2 d However, it cannot clearly discriminate litchi samples in the following 4 d Hence, the PCA method is not suitable for quantitative discrimination toward litchi samples.
SR analysis result
Litchi sample's SNR spectrum analysis result is shown in . Samples' initial SNR values are in the range from −77.5 to −62.5 dB and their Max-SNR values range between −59.5 to −55.0 dB. Eigen peak appears at the stimulating noise intensity of 100 or so. Some derivative vales arise before the formation of eigen peaks. After that, each SNR value gradually decreases with the increase of stimulating noise intensity and finally reaches their stable value about -85.0 dB. So, litchi samples under different storage days can be clearly discriminated using Max-SNR values.
For the further step, a litchi freshness predictive model using Max-SNR values as eigen values is built and EquationEq. (1)(1)
(1) is obtained by linear-fitting. The result with regression coefficients R2 = 0.99396 is displayed in , which indicates the proposed predictive model can successfully discriminate all litchi samples under different storage days. Some samples may bring about minor errors.
(1)
(1)
In this research, PCA and SR analysis methods were used to analyze the detected e-nose data. The result indicated that PCA could not discriminate all litchi samples with different storage days. SNR spectrum eigen values calculated by SR successfully discriminated all litchi samples. The rapid predictive model about litchi freshness built by linear-fitting Max-SNR eigen values shows a satisfying result with prediction accuracy R2 = 0.99396.
Materials and methods
Litchi samples
Litchi samples were purchased from Gouzhuang fruit whole market (Hangzhou, China). Samples (nearly the same in weight, size, and ripeness) without any freshness pretreatment were selected for the experiments. All litchi samples were stored in an ice bubble chamber. And all measurements were performed at room temperature.
Methods
E-nose detection
E-nose structure is displayed in . It consists of 3 main parts: data acquisition, modulating and transmitting unit (U1); sensor array and chamber unit (U2); power and gas supply unit (U3). E-nose consists of 8 M.O.S gas sensors with different sensitive species. The selectivity toward volatile compound classes of M.O.S sensors is indicated by the supplier: S1 (TGS-825, hydrogen sulfide), S2 (TGS-821, hydrogen), S3 (TGS-826, ammonia), S4 (TGS-822, ethanol, methylbenzene, xylene gas), S5 (TGS-842, hydrocarbon component gas), S6 (TGS-813, methane, propane, and butane), S7 (TGS-2610, propane, butane), S8 (TGS-2201, nitrogen oxides). Each sensor is installed in independent chamber to avoid cross-influence of gas flow.
Figure 1. (a) E-nose sensor original responses; (b) PCA analysis result; (c) SNR spectrum analysis result; (d) Litchi freshness predictive model.
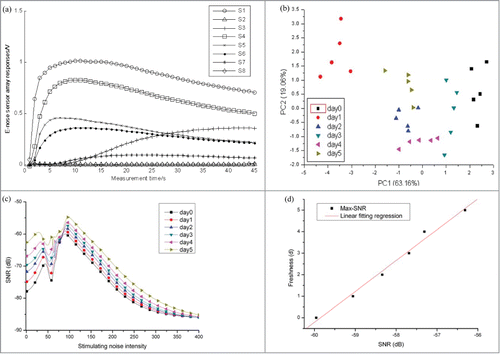
In e-nose detection, each sample was placed into a 50 mL air-tight vial, and sealed with sealing membrane. The vials were equilibrated for 30 min at room temperature. Turned on e-nose power, and started washing pump and valve 2. The sampling pump and valve 1 remained off. The air was filtered by active carbon to obtain zero gas. Sensor array were recovered by zero gas. When sensors' responses returned to the baseline, washing pump and valve 2 were shut off. Then sampling pump and valve 1 were turned on. The gases in sample's headspace were inhaled into gas sensor chambers by sampling pump at a flux speed of 400 mL/min for 40s. E-nose measurement interval was 0.05 s. E-nose real-time responses to Litchi samples were recorded. When measurement was over, gas sensors were recovered by zero gas at a flux speed of 1000 mL/min for 600s, waiting for the next measurement.
SR
SR is a typical non-linear model and proposed by Benzi for the explanation for Earth climate periodic changes.Citation18,19 SR phenomenon has 3 elements: a bistable system, a coherent input, and a noise source, which can be described as:(2)
(2)
Where is the position of the Brownian particle,
is the time, M and D are adjustable parameters,
denotes an input signal
and intrinsic noise
,
is the external noise, and
is the simplest double-well potential with the constants
and
characterizing the system.
(3)
(3)
Eq. (2) can be written as(4)
(4)
The minima of are located at
, where
. A potential barrier separates the minima with the height given by
. The barrier top is located at
. When three elements of SR interact coherently, the potential barrier can be reduced and the Brownian particle may surmount the energy barrier and enter another potential well. The intensity of signals will increase, which makes it possible that the weak signal can be detected from noise background.
Suppose the input signal is , where
is signal intensity,
is signal frequency.
is external noise intensity. SNR is the common quantifier for SR and it can be approximately described as:
(5)
(5)
Conclusions
Litchi (Litchi chinensis Sonn) freshness rapid non-destructive evaluating method based on e-nose and non-linear stochastic resonance was proposed in this research. PCA method cannot discriminate all litchi samples with different qualities, while SR SNR spectrum successfully discriminated all samples. Litchi freshness rapid evaluating model was built by linear fitting regression of Max-SNR values. The developed model exhibited high evaluating accuracy for litchi samples with regression coefficients R2 = 0 .99396. The method proposed in this research exhibits many unique characteristics including rapid response, high accuracy, low cost, etc.
Disclosure of Potential Conflicts of Interest
No potential conflicts of interest were disclosed.
Funding
This work is financially supported by National Natural Science Foundation (No. 81000645), and project supported by Key Laboratory of Forestry Intelligent Monitoring and Information Technology of Zhejiang Province.
References
- Zeng XA, Chen XD, Qin FGF, Zhang L. Composition analysis of litchi juice and litchi wine. International J Food Engineering 2008; 4:1556-3758; http://dx.doi.org/10.2202/1556-3758.1379.
- Yang ZY, Wang TD, Wang HC, Huang XM, Qin YH, Hu GB. Patterns of enzyme activities and gene expressions in sucrose metabolism in relation to sugar accumulation and composition in the aril of Litchi chinensis Sonn. J Plant Physiol 2013; 170:731-40; PMID:23499454; http://dx.doi.org/10.1016/j.jplph.2012.12.021.
- Lin S, He JR, Jiang YM, Wu FW, Wang H, Wu D, et al. Production of nigragillin and dihydrophaseic acid by biotransformation of litchi pericarp with Aspergillus awamori and their antioxidant activities. J Functional Foods 2014; 7:278-86; http://dx.doi.org/10.1016/j.jff.2014.02.001.
- Liu H, Song LL , You Y, Li YB, Duan XW, Jiang YM, et al. Cold storage duration affects litchi fruit quality, membrane permeability, enzyme activities and energy charge during shelf time at ambient temperature. Postharvest Biol Technol 2011; 60:24-30; http://dx.doi.org/10.1016/j.postharvbio.2010.11.008.
- Holcroft DM, Mitcham EJ. Postharvest physiology and handing of litchi. Postharvest Boil Technol 1996; 9:265-81; http://dx.doi.org/10.1016/S0925-5214(96)00037-3.
- Neog M, Saikia L. Control of post-harvest pericarp browning of litchi (Litchi chinensis Sonn). J Food Sci Technol 2010; 47:100-4; PMID:23572609; http://dx.doi.org/10.1007/s13197-010-0001-9.
- Ruenroengklin N, Sun J, Shi J, Xue SJ, Jiang YM. Role of endogenous and exogenous phenolics in litchi anthocyanin degradation caused by polyphenol oxidase. Food Chem 2009; 115:1253-6; http://dx.doi.org/10.1016/j.foodchem.2009.01.040.
- Liang YS, Chen NL, Ke LS. Influence of dipping in sodium metabisulfite on pericarp browning of litchi cv. Yu Her Pau (Feizixiao). Postharvest Biol Technol 2012; 68:72-7; http://dx.doi.org/10.1016/j.postharvbio.2012.02.005.
- Sun J, Xiang X, Yu CY, Shi JY, Peng HX, Yang B, Yang SY, Yang A, Jiang YM. Variations in contents of browning substrates and activities of some related enzymes during litchi fruit development. Scientia Horticulturae 2009; 120:555-9; http://dx.doi.org/10.1016/j.scienta.2008.12.006.
- Huang L, Zhao JW, Chen QS, Zhang YH. Nondestructive measurement of total volatile basic nitrogen (TVB-N) in pork meat by integrating near infrared spectroscopy, computer vision and electronic nose techniques. Food Chem 2014; 145:228-36; PMID:24128472; http://dx.doi.org/10.1016/j.foodchem.2013.06.073.
- Russo M, Sanzo RD, Cefaly V, Carabetta S, Serra D, Fuda S. Non-destructive flavour evaluation of red onion (Allium cepa L.) Ecotypes: An electronic-nose-based approach. Food Chem 2013; 141: 896-9; PMID:23790864; http://dx.doi.org/10.1016/j.foodchem.2013.03.052.
- Tian XJ, Wang J, Cui SQ. Analysis of pork adulteration in minced mutton using electronic nose of metal oxide sensors. J Food Engineering 2013; 119:744-9; http://dx.doi.org/10.1016/j.jfoodeng.2013.07.004.
- Hong XZ, Wang J. Detection of adulteration in cherry tomato juices based on electronic nose and tongue: Comparison of different data fusion approaches. J Food Engineering 2014; 126:89-97; http://dx.doi.org/10.1016/j.jfoodeng.2013.11.008.
- Huo DQ, Wu Y, Yang M, Fa HB, Luo XG, Hou CJ. Discrimination of Chinese green tea according to varieties and grade levels using artificial nose and tongue based on colorimetric sensor arrays. Food Chem 2014; 145:639-45; PMID:24128526; http://dx.doi.org/10.1016/j.foodchem.2013.07.142.
- Hui GH, Wu YL, Ye DD, Ding WW, Zhu LS, Wang LY. Study of peach freshness predictive metod based on electronic nose. Food Control 2012; 28:25-32; http://dx.doi.org/10.1016/j.foodcont.2012.04.025.
- Hong XZ, Wang J, Qi GD. E-nose combined with chemometrics to trace tomato-juice quality. J Food Engineering 2015; 149:38-43; http://dx.doi.org/10.1016/j.jfoodeng.2014.10.003.
- Torri L, Sinelli N, Limbo S. Shelf life evaluation of fresh-cut pineapple by using an electronic nose. Postharvest Biol Technol 2010; 56:239-45; http://dx.doi.org/10.1016/j.postharvbio.2010.01.012.
- Benzi R, Sutera A, Vulpiana A. The mechanism of stochastic resonance. J Phys 1981; 14:453-6; http://dx.doi.org/10.1088/0305-4470/14/11/006.
- Gammaitoni L, Hanggi P, Jung P, Marchesoni F. Stochastic resonance. Rev Modern Phys 1998; 70:223-87; http://dx.doi.org/10.1103/RevModPhys.70.223.