ABSTRACT
Although some progress has been made in the molecular biological detection of major depression disorder (MDD), its specificity and accuracy are still insufficient. This study is aimed to find hub genes, which could contribute to MDD related suicide and provide potential therapeutic targets for diagnosis and treatment. We downloaded RNA expression and clinical information from Gene Expression Omnibus (GEO) Dataset. Then, weighted gene co-expression network analysis (WGCNA) was applied to find core modules. Logistic regression was performed to identify the independent risk factors, and a scoring system was constructed based on these independent risk factors. As a result, a total of 16487 genes were selected to further conducted WGCNA analysis. We found that tan and green functional modules were exhibited high correlation with suicide behavior. 309 genes were identified in tan modules that were the strongest positively correlated with suicide behavior. Functional analysis in tan module indicated that activation of enzymes including nitric-oxide synthase and endoribonuclease, estrogen signaling pathway, glucagon signaling pathway, and legionellosis pathway were most enriched in MDD. Furthermore, we applied protein–protein interaction (PPI) analysis to select the hub genes and 10 genes were found in the core area of network. Then, we identified three-gene base independent risk signature by logistic regression model, including HSPA1A, RASEF, TBC1D8B. In conclusion, our study suggests that the tan module genes are closely related to suicide behaviors, which is mainly caused by multiple signaling pathway activation. The three-genes-based signature could provide a better efficacy to predict suicidal behavior in MDD patients.
Introduction
Major depression disorder (MDD) is an affective psychiatric syndrome with depression of mood, lack of interest, which was also accompanied by anxiety, cognitive impairment, psychomotor disorders, or even suicide attempt [Citation1]. The pathogenesis of this disease is relatively complicated. It is generally considered to be related to genetic, gender, neuroendocrine, psychosocial environment, immunity, and intestinal microbes [Citation2,Citation3]. The pre-clinical diagnosis is mainly based on the symptom of patients description, mental state examination, and clinical behavior observation [Citation4]. For the treatment of MDD, the most commonly used method was to give selective serotonin reuptake inhibitors [Citation5]. However, more than one-third of patients were found resistant to this drug, which limited the use of the drugs [Citation6].
A recent research demonstrated that the number of deaths caused by suicide around the world was about 785000 in 2016, with an incidence of 10.6/100000 population [Citation7]. Despite the personal, social, and culture factors, depression is considered the leading cause of suicide behavior, which contributes to almost half of the individual deaths in developed countries [Citation8,Citation9]. These results suggested an urgent situation to develop and explore more efficient therapeutic and diagnosis targets for prevention of suicidal behavior in MDD patients.
Recently, with the development of microarray and next-generation sequence (NGS), various of genes were identified and selected for diagnosis and treatment of many human diseases, including cancer, immune diseased, and so on. Meanwhile, as an emerging interdisciplinary subject, bioinformatics analysis was widely used for differential expressed gene selected, network construction, functional analysis, which could facilitate the mining of molecular mechanism of diseases and the development of potential target for therapy. For example, Zhao et al. [Citation10] analyzed and compared the data of oral squamous cell carcinoma and normal tissues through bioinformatics methods, and screened out the prognosis-related genes including plasminogen activator urokinase (PLAU), claudin8 (CLDN8) and cyclin-dependent kinase inhibitor 2A (CDKN2A), providing important guidance for the personalized and precise treatment of oral squamous cell carcinoma. The results of bioinformatics analysis by Wang et al. showed that miR-34a expression was regulated by genes such as serpin family E member 1(SERPINE1), Kruppel like factor4(KLF4), and semaphorin 4B (SEMA4B), which in turn affected the development of colorectal cancer and provided diagnostic and therapeutic research for colorectal cancer [Citation11]. As for mental disorder, it was also reported the whole genome-wide research on common variants enhancing our understanding of many diseases, such as MDD [Citation12]. Pantazatos et al. applied with RNA-sequence analysis for exploration of whole-exome gene and exon expression in non-psychiatric controls and DSM-IV major depressive disorder suicides. They identified 35 differentially expressed genes and found that ATPase activity and astrocytic cell functions contribute to MDD and suicide [Citation13]. However, many of these researches neglected the high interconnection between genes that have similar expression patterns and only pay attention to the identification of differentially expressed genes. Recently, a systems biology algorithm of weighted gene co-expression network analysis (WGCNA) has been widely used to evaluate the association between genes sets and clinical traits by constructing a scale-free gene co-expression network. Meanwhile, there are still many unexplored genes and mechanisms underlying it needed to be discovered. In this study, the WGCNA-based bioinformatics method was used to deeply mine and analyze the MDD chip expression profile data, in order to find candidate genes that are convenient for detection of MDD and provide new targets for the early diagnosis and treatment.
Here, the weighted gene co-expression network analysis (WGCNA) method was used to find hub genes that are correlated with suicide behavior, which could provide new therapeutic targets for the diagnosis and individualized treatment of the MDD.
Methods and materials
Microarray data download and preprocessing
Two MDD cohorts of GSE101521 and GSE102556 datasets were downloaded from GSE database (http://www.ncbi.nlm.nih.gov/geo/) including microarray RNA expression profiles and clinical data, and then normalized between different arrays by R ‘sva’ package. The GSE101521 dataset includes 30 MDD patients and 29 normal control patients. The GSE102556 datasets contains 263 MDD patients from six different brain regions. We choose brain region of prefrontal cortex in both datasets to analyze the key genes. According to the annotation information on the respective platforms, the probes were converted into corresponding gene symbols. Then, we normalized the two cohort data by R ‘sva’ package and intersect the same gene. After intersection preprocessing, a total of 16487 genes were ready for further WCGNA analysis.
Construction of weighted gene coexpression networks
WGCNA package(v1.68) downloaded from Bioconductor (http://bioconductor.org/biocLite.R) was used for co-expression analysis. Firstly, we used the Pearson correlation analysis to evaluate the co-expression relationships in all datasets by soft threshold method, which could facilitate determine the connection strengths to establish a weighted network. Based on the topological overlapping dissimilarity of network connection strengths, the adjacent matrix was converted to a topological overlapping matrix(TOM). Then, each TOM was hierarchically clustered via the flashClust function in R. To obtain the correct module number and clarify gene interaction, we set the restricted minimum gene number to 100 for each module and used a threshold of 0.25 to merge the similar modules. The regression relationship between clinical data and gene expression was based on the P-value. The statistical significance was calculated as P values (P < 0.05). Once the modules of interest were selected, gene significance (GS) and module membership (MM) were determined for each important gene, and thresholds of cor. gene MM > 0.8 and cor. gene GS > 0.5 was established to screen key genes in each module.
Functional enrichment analysis of genes in key modules
We further applied function enrichment analysis including gene ontology (GO) enrichment and Kyoto Encyclopedia of Genes and Genomes (KEGG) pathway. To evaluate the biological process, molecular functions, and cellular components associated with the key module genes, we performed the GO enrichment analysis and visualization by ‘clusterProfiler’ and ‘ggplot2’ package. Also, KEGG pathway enrichment analysis was carried out by ‘clusterProfiler’ package to elucidate potential pathway regulated by key module genes. P-value < 0.05 and adjust P-value < 0.05 were defined as the cutoff criteria.
Integration of genetics and highly connected hubs in modules
The STRING database was applied to identify potential interactions among the key module genes. The protein–protein interaction networks (PPIs) with a confidence score ≥ 0.4 were reserved and further imported to Cytoscape (v3.7.0) for constructing the PPI network of key module genes. Moreover, to detect hub clustering modules in the PPI network, we performed module analysis utilizing ‘cytoHubba’ app with MCC method in Cytoscape.
Establishing of scoring system
Firstly, we randomly separated all patients to train dataset and test dataset by ‘caret’ package in R with the proportion of train dataset 60%. Independent risk genes were identified by logistic regression analysis. A scoring system based on independent risk genes was established by Least absolute shrinkage and selection operator(LASSO) regression. The receiver operating characteristic (ROC) curve was carried out using the ‘pROC’ package in R to evaluate the reliability of the scoring system. Subsequently, the risk score and the clinicopathological characteristics of MDD patients were analyzed, and the scoring system was visualized by forest chart and nomogram through the ‘rms’ package in R.
Results
Identification of intersection genes among two GEO cohort
After downloaded the row data from GEO website, we applied annotation and batch normalization by the ‘sva’ package in R. There were 51123 genes in GSE101521 and 57774 genes in GSE102566 dataset. Then, we intersected the GSE101521 and GSE102566 datasets and a total of 16487 same genes were found exist in both cohorts for further WGCNA analysis.
Weighted co-expression network construction and key module identification
To identify the potential mechanisms by which these genes facilitate the progression of major depression disorder, the WGCNA analysis we applied to construct a scale-free co-expression network. After determining the optimal parameter (β = 19), the WGCNA algorithm was used to convert correlation coefficient to adjacent coefficient (,)). Then, we clustered the samples and delete the discrete samples ()). After that, we set the restricted minimum gene number for each module and used a threshold of 0.25 to merge the similar modules. As is shown in ), we finally obtained nine gene modules according to these assumptions. The hierarchical clustering of module hub genes that summarize the modules yielded in the clustering analysis, which combined the similar modules ()). The detailed information of these modules is as follows: there were 526 genes in black module, 1993 genes in blue module, 1398 genes in brown module, 893 genes in green module, 6394 genes in gray module, 1602 genes in magenta module, 309 genes in tan module, 2437 genes in turquoise module, 939 genes in yellow module.
Figure 1. Determination of soft-threshold power and clustering of samples and identification of gene expression modules. (a) Analysis of the scale-free fit index for various soft-threshold powers(β). (b) Analysis of the mean connectivity for various soft-threshold powers. (c) Clustering dendrogram of genes based on a dissimilarity measure. (d) Clustering dendrogram of genes based on a dissimilarity measure. The sample clustering was based on the expression data with variances ranked in top 10,000 in MDD samples. (e) The hierarchical clustering of module hub genes that summarize the modules yielded in the clustering analysis. (f) The TOM heatmap indicated the relationship between co-expression genes
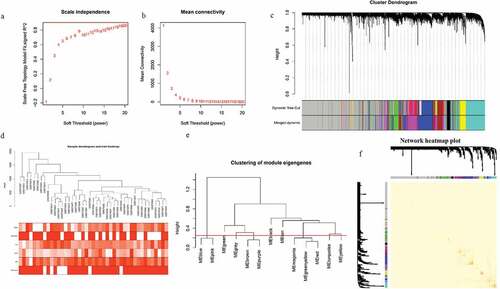
Identification of key modules highly correlated with suicide behavior
The TOM heatmap ()) indicated the interactions between the genes of nine modules. As shown in ), the tan module exhibited positive association with suicide behavior with correlations close to 0.63, while green module showed negative association with suicide behavior with 0.45 correlations. In the tan module, we filtered 93 key genes that were consistent with the criterion of MM > 0.8 and GS > 0.5, which indicated that these key genes were highly correlated with suicide behavior in MDD patients ()). Furthermore, we also demonstrated the connectivity of eigengenes and suicide behavior by the heatmap plot. The results indicated that four pairs of modules exhibited highly connection, which were turquoise and yellow, tan and suicide, magenta and brown, black and turquoise ()). Taken together, tan module was finally selected for further analysis.
Figure 2. Identification of modules associated with the clinical traits of MDD and functional analysis. (a) heatmap of the association between MES and clinical traits of MDD. Tan module showed high correlation with suicide trait. (b) The connectivity of eigengenes and suicide behavior by the heatmap plot. (c) The GC and MM analysis showed key genes in green and tan modules. (d) GO and KEGG functional annotation genes in the purple module. The y-axis shows the GO and KEGG terms, and x-axis shows the gene counts of each term
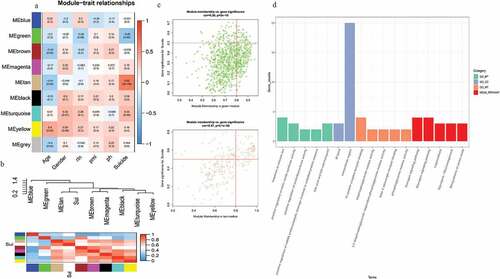
Functional enrichment analysis of genes in modules of interest
GO results indicated that genes in tan module were mainly enriched in the activation of enzymes including nitric-oxide synthase and endoribonuclease. Besides, response to calcium ion, positive regulation of mRNA endonucleolytic cleavage involved in unfolded protein response, transportation of bile acid and bile salt were also involved in tan module genes. According to KEGG analysis, we found that estrogen signaling pathway, glucagon signaling pathway, legionellosis, gluconeogenesis, and biosynthesis of amino acids were the top 5 pathways involving in tan module genes. There are four genes enriched in estrogen signaling pathway, which were heat shock protein family A member 1B(HSPA1B), heat shock protein family A member 1A(HSPA1A), GNAS complex locus(GNAS), estrogen receptor 1(ESR1). These results suggested that suicide behavior in MDD might closely relate to hormone regulation and glucose and amino acid metabolism ()).
PPI network construction and analysis of selected modules
The protein–protein interaction networks (PPIs) of whole module genes in tan module were constructed, respectively, by STRING database ()). Then the downloaded data were visualized by Cytoscape software and analyzed by ‘cytoHubba’ application ()). We intersected the 30 hub genes with 93 key genes sorted by GS and MM. Ten hub genes were found including mab-21 like 3(MAB21L3), transmembrane protein 212(TMEM212), TBC1 domain family member 8B (TBC1D8B), transmembrane protein 45A(TMEM45A), RAS and EF-hand domain-containing (RASEF), NEDD4 E3 ubiquitin protein ligase(NEDD4), fibrous sheath interacting protein 2(FSIP2), CYSLTR1, HSPA1B, and HSPA1A ()).
Figure 3. PPI networks of hub genes in tan module. (a) All genes in tan module were analyzed in PPI network. (b) Hub genes in tan module were identified via ‘cytohubba’ method. (c) Overlap between hub genes and key genes in tan module. Group 1 represented key genes in tan module selected by GS and MM. Group 2 represented hub genes selected by ‘cytohubba’
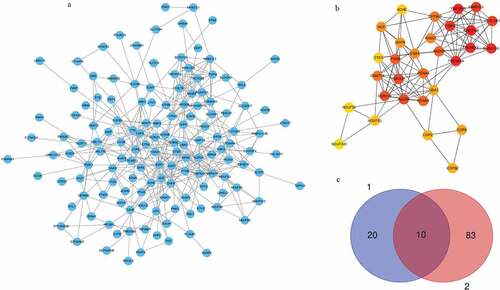
Scoring system construction
After ten hub genes were identified, we applied logistic regression and LASSO analysis to establish prediction model. After deleted highly irrelevant genes, a total of three suicide-related genes in train dataset were selected in LASSO model, including HSPA1A, RASEF, and TBC1D8B ()). The riskscore = −2.648 (Intercept) + HSPA1A-expression*0.0273 + RASEF-expression*0.531 + TBC1D8B-expression*0.310. The area under the curve (AUC) of this scoring system in train dataset and test dataset were 0.929 and 0.702, respectively ()). Then, we combined the risk-score and clinical features of each patient and found that risk-score is an independent factor to predict the probability of suicide behavior () upper panel). Moreover, we then constructed a risk factor-based nomogram to further generate a more accurate evaluation system () lower panel).
Figure 4. Establish the scoring system to predict suicide behavior in MDD patients. (a) Optimal candidate key genes selected by AUC measurement. (b) ROC curve of train and test dataset. (c) forest plot showed risk-score was independent risk factor for prediction (upper panel). Nomogram of a scoring system was showed in lower panel
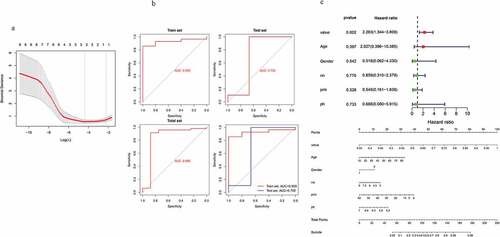
Discussion
Depression, a serious and common mental illness, has now aroused widespread concern and has become a widespread public health problem. Although some progress has been made in the treatment of depression, there are still many MDD unique pathophysiological characteristics that need to be further studied [Citation14,Citation15].
The mechanisms of depression are complicated. The currently recognized mechanisms include: neurotransmitter hypothesis, neurotrophic factor hypothesis, and inflammatory immune hypothesis. The research on MDD biomarkers is also mainly carried out around these hypotheses, involving research in neurochemistry, neuroimaging, genetics, epigenetics, and genomics [Citation16,Citation17]. For example, the neurotransmitter hypothesis theory believes that depression is mainly due to intersynaptic monoamine transmitters (such as serotonin, norepinephrine, dopamine, etc.) [Citation18] and receptors (such as NMDA receptors, Glutamate receptors, etc.) [Citation19,Citation20]. Meanwhile, the ‘neurotrophic factor hypothesis’ focuses on the aberrant expression level of brain-derived neurotrophic factor (BDNF) and vascular endothelial growth factor (VEGF) [Citation21].
In recent years, microarray and high-throughput sequencing technology has become a widely used technology to identify special targets for the treatment of many diseases [Citation22]. In our previous study, we used the RNA-Seq analysis identified a serious of differently expressed genes that could contribute to cancer stem cell properties in esophageal squamous cell carcinoma [Citation23]. In the treatment of MDD, this method is also widely used. Through the original data of GSE98793, a recent study found that genes of MDD were extensively enriched in the immune response against infection, suggesting that the target of immune system regulation should be used to treat MDD [Citation24].
In this study, we first applied the WGCNA method to identify MDD signatures related to suicidal behavior. First, we downloaded two datasets of GSE101521 and GSE102566 in the GEO database. After deleting the data with not available (NA), we found 16487 genes after taking the intersection and batch normalization. We then performed WGCNA analysis on these co-expressed genes and identified 9 modules. We correlated these modules with clinical traits and found that tan module genes are highly related to suicide behavior. Subsequently, we conducted GO enrichment and KEGG pathway analysis for tan module genes and found that the function of tan module is mainly related to activation of enzymes including nitric-oxide synthase and endoribonuclease, estrogen signaling pathway, glucagon signaling pathway, and legionellosis pathway. Through PPI network analysis, we identified 10 hub genes in the tan module. Through GO enrichment and Pathway analysis, these hub genes are mainly enriched in positive regulation of protein catabolic process, interspecies interaction between organisms, regulation of protein catabolic process, positive regulation of catabolic process.
Although in the past few decades, bio-amine drugs including serotonin and norepinephrine have become widely used in the treatment of depression. Recently, there is an increasing evidence that other biological systems may also contribute to the pathophysiology and treatment of MDD, such as the role of nitric oxide (NO). At present, some studies have shown that NO levels in patients with MDD have significantly altered, and NO signaling pathway regulators have antidepressant effects in MDD patients or animals [Citation25]. In addition, the NO signaling pathway may potentially regulate the inflammatory pathways of various mental disorders such as MDD. Exploring the key molecules of the NO pathway can provide an important target for the treatment of MDD [Citation26]. In our study, we identified core tan module highly related to suicide behavior of MDD patients by WGCNA. Genes in tan module were mainly enriched in activation of nitric-oxide synthase, which suggesting the tan module genes could play an important role in MDD treatment.
Recently, it was reported that the incidence of MDD in female was higher than that of male, indicating the role of estrogen in regulation of MDD [Citation27]. Both human and animal models confirm this theory that block estrogen signaling pathway might be a potential therapeutic target for the treatment of MDD [Citation28,Citation29]. In our study, we identified that estrogen signaling pathway was also enriched in tan module genes, which showed strong correlation with suicide behavior.
According to the PPI network analysis from the tan module, we identified ten hub genes including MAB21L3, TMEM212, TBC1D8B, TMEM45A, RASEF, NEDD4, FSIP2, CYSLTR1, HSPA1B, and HSPA1A. Among these genes, HSPA1A and HSPA1B were clustered to estrogen signaling pathway. HSPA1A, HSPA1B, NEDD4 was also enriched to the biological process of positive regulation of protein catabolic process, interspecies interaction between organisms, regulation of protein catabolic process, and positive regulation of catabolic process via GO analysis. For example, it was reported that NEDD4, an E3 ubiquitin-protein ligase, was suppressed in depression patients and impedes offspring dendrite development and maternal immune activation [Citation30]. Having a deep insight into these hub genes would provide with novel therapeutic target for the treatment of MDD and prevent suicidal behavior.
After ten hub gene were identified, we further applied logistic regression analysis to construct a three-gene-based prediction model in facilitating suicide behavior prediction of MDD patients. The results indicated that this model has an excellent prediction probability in both train and test datasets. Moreover, the risk predictor could serve as an independent risk factor compared with other clinical characteristics. To conclusion, this study is a first attempt to explore the suicide-related genes in MDD patients by WGCNA and subsequently construction of a prediction model and using key genes as potential biomarker.
There were also some shortcomings and limitations in our study. First of all, the results in this study were based on WGCNA data mining and haven’t further proved on experiments. Secondly, the number of samples used in this study needs to be further increased. We believed experiments for validation of our results and exploration of the deep mechanisms in vivo and in vitro will be further applied in future.
Authors’ contributions
ZY and SY-Z conceptualized and designed the article. ZY and WL analyzed and interpreted the data. ZY and WL drafted of the article. ZY, SY-Z, WYJ, LZQ were responsible for critical revision of the article for important intellectual content.
Data availability statement
The datasets analyzed during the present study are available from CEO database (http://www.ncbi.nlm.nih.gov/geo/).
Disclosure statement
We declare that we do not have any commercial or associative interest that represents a conflict of interest in connection with the work submitted.
References
- Yang L, Zhao Y, Wang Y, et al. The effects of psychological stress on depression. Curr Neuropharmacol. 2015;13:494–504.
- Ogłodek E, Szota A, Just M, et al. The role of the neuroendocrine and immune systems in the pathogenesis of depression. Pharmacol Rep. 2014;66:776–781.
- Weightman MJ, Air TM, Baune BT. A review of the role of social cognition in major depressive disorder. Front Psychiatry. 2014;5:179.
- Fan HM, Sun XY, Guo W, et al. Differential expression of microRNA in peripheral blood mononuclear cells as specific biomarker for major depressive disorder patients. J Psychiatr Res. 2014;59:45–52.
- Kitahara Y, Ohta K, Hasuo H, et al. Chronic fluoxetine induces the enlargement of perforant path-granule cell synapses in the mouse dentate gyrus. PloS One. 2016;11:e0147307.
- Duman RS, Aghajanian GK. Synaptic dysfunction in depression: potential therapeutic targets. Science. 2012;338:68–72.
- Turecki G, Brent DA, Gunnell D, et al. Suicide and suicide risk. Nat Rev Dis Primers. 2019;5:74.
- Cavanagh JT, Carson AJ, Sharpe M, et al. Psychological autopsy studies of suicide: a systematic review. Psychol Med. 2003;33:395–405.
- Arsenault-Lapierre G, Kim C, Turecki G. Psychiatric diagnoses in 3275 suicides: a meta-analysis. BMC Psychiatry. 2004;4:37.
- Zhao X, Sun S, Zeng X, et al. Expression profiles analysis identifies a novel three-mRNA signature to predict overall survival in oral squamous cell carcinoma. Am J Cancer Res. 2018;8:450–461.
- Wang T, Xu H, Liu X, et al. Identification of key genes in colorectal cancer regulated by miR-34a. Med Sci Monit. 2017;23:5735–5743.
- Wray NR, Ripke S, Mattheisen M, et al. Genome-wide association analyses identify 44 risk variants and refine the genetic architecture of major depression. Nat Genet. 2018;50:668–681.
- Pantazatos SP, Huang YY, Rosoklija GB, et al. Whole-transcriptome brain expression and exon-usage profiling in major depression and suicide: evidence for altered glial, endothelial and ATPase activity. Mol Psychiatry. 2017;22:760–773.
- Smith K. Mental health: a world of depression. Nature. 2014;515:181.
- Rotenstein LS, Ramos MA, Torre M, et al. Prevalence of depression, depressive symptoms, and suicidal ideation among medical students: a systematic review and meta-analysis. Jama. 2016;316:2214–2236.
- Strawbridge R, Young AH, Cleare AJ. Biomarkers for depression: recent insights, current challenges and future prospects. Focus (American Psychiatric Publishing). 2018;16:194–209.
- Schneider B, Prvulovic D. Novel biomarkers in major depression. Curr Opin Psychiatry. 2013;26:47–53.
- Kuffel A, Eikelmann S, Terfehr K, et al. Noradrenergic blockade and memory in patients with major depression and healthy participants. Psychoneuroendocrinology. 2014;40:86–90.
- Maletic V, Eramo A, Gwin K, et al. The role of norepinephrine and its α-adrenergic receptors in the pathophysiology and treatment of major depressive disorder and schizophrenia: a systematic review. Front Psychiatry. 2017;8:42.
- Chandley MJ, Szebeni K, Szebeni A, et al. Gene expression deficits in pontine locus coeruleus astrocytes in men with major depressive disorder. J Psychiatry Neurosci. 2013;38:276–284.
- van Diermen L, van den Ameele S, Kamperman AM, et al. Prediction of electroconvulsive therapy response and remission in major depression: meta-analysis. Br J Psychiatry. 2018;212:71–80.
- Bian Y, Yang L, Zhao M, et al. Identification of key genes and pathways in post-traumatic stress disorder using microarray analysis. Front Psychol. 2019;10:302.
- Zhao Y, Zhu J, Shi B, et al. The transcription factor LEF1 promotes tumorigenicity and activates the TGF-β signaling pathway in esophageal squamous cell carcinoma. J Exp Clin Cancer Res. 2019;38:304.
- Leday GGR, Vértes PE, Richardson S, et al. Replicable and coupled changes in innate and adaptive immune gene expression in two case-control studies of blood microarrays in major depressive disorder. Biol Psychiatry. 2018;83:70–80.
- Vaváková M, Ďuračková Z, Trebatická J. Markers of oxidative stress and neuroprogression in depression disorder. Oxid Med Cell Longev. 2015;2015:898393.
- Ghasemi M. Nitric oxide: antidepressant mechanisms and inflammation. Adv Pharmacol. 2019;86:121–152.
- Chhibber A, Woody SK, Karim Rumi MA, et al. Estrogen receptor β deficiency impairs BDNF-5-HT(2A) signaling in the hippocampus of female brain: A possible mechanism for menopausal depression. Psychoneuroendocrinology. 2017;82:107–116.
- Murakami G, Hojo Y, Ogiue-Ikeda M, et al. Estrogen receptor KO mice study on rapid modulation of spines and long-term depression in the hippocampus. Brain Res. 2015;1621:133–146.
- Mehta D, Newport DJ, Frishman G, et al. Early predictive biomarkers for postpartum depression point to a role for estrogen receptor signaling. Psychol Med. 2014;44:2309–2322.
- Hu Y, Hong XY, Yang XF, et al. Inflammation-dependent ISG15 upregulation mediates MIA-induced dendrite damages and depression by disrupting NEDD4/Rap2A signaling. Biochim Biophys Acta Mol Basis Dis. 2019;1865:1477–1489.