ABSTRACT
Sepsis, resulting from a harmful or damaging response to infection, is a complex and severe disease that causes high mortality. Three independent expression profiles of miRNA – GSE94717, GSE149764, and GSE101639 – were collected and integrated to analyze miRNAs associated with sepsis. One miRNA, miR-223-3p, was detected significantly downregulated in patients with sepsis. The upregulated miR-223-3p target genes in patients with sepsis were enriched in central carbon metabolism associated with HIF-1 signaling and galactose metabolism. Specially, three HIF-1 signaling genes – hypoxia-inducible factor 1-alpha (HIF1A), hexokinase 2 (HK2), and MAP kinase-interacting serine/threonine-protein kinase 1 (MKNK1) – were found significantly upregulated in patients with sepsis. Additionally, MKNK1 expression was downregulated in septic responders to early therapeutic treatments. Neutrophils were significantly accumulated in patients with sepsis and decreased in responders after therapy; MKNK1 was significantly positively correlated with neutrophils. Our findings indicate MKNK1, one targets of miR-223-3p, might be involved in sepsis via regulating the neutrophils abundance by mediating the expression inflammation factors.
1 Introduction
Sepsis, a systemic inflammation usually caused by infection, is accompanied by clinical diseases, such as severe burns, acute illness, and surgical operation, and causes high mortality rates in intensive care units (ICUs) [Citation1,Citation2]. This syndrome has a high incidence, remains a crucial public health issue, and is one of the primary causes of death [Citation1]. Despite the advancements in medical care, sepsis, usually associated with acute organ failure, remains an acute disease that causes considerable economic loss [Citation3]. The World Health Assembly and World Health Organization consider sepsis a global health priority and appealed to the whole world to focus on the prevention, diagnosis, and support of sepsis in 2017 [Citation4]. Li and his team have performed population-level research on the mortality related to sepsis in China; they found that the standardized sepsis-related death rate in China is high (66.7 deaths per 100,000 population) [Citation5]. Therefore, more studies and a deeper knowledge of sepsis are needed, especially to better identify patients with sepsis and assess the outcome of prognosis.
MicroRNAs (miRNAs), small evolutionarily conserved non-coding RNAs containing 20–25 nucleotides, silence post-transcriptional genes via inhibiting the translation process of messenger RNA (mRNA) or decreasing transcript levels of mRNA [Citation6]. Several miRNAs, crucial regulators of biological processes and molecular regulation, have been reported to be involved in immune inflammatory pathologies and sepsis [Citation7–9]. A recent publication reported downregulation of Klf4 (Kruppel-like factor 4) mediated by rno-miR-146b-5p in development of intestinal injury in intestinal sepsis, indicating the critical role of miRNAs in regulating sepsis by mediating the expression of target genes [Citation10]. MiRNAs primarily regulate innate immunity via Toll-like receptor signal transduction pathways [Citation11]. miR-223-3p is a granulocyte-enriched miRNA in various tissues, particularly in whole blood. Researchers have reported the essential role of miR-223-3p as a regulator in multiple diseases, such as cancer, and acute and chronic liver injury [Citation12,Citation13]. Franz, et al. have reported the myeloid-specific miR-223-3p as a negative regulator mediating the activity of NOD-, LRR-, and pyrin domain-containing protein 3 inflammasome [Citation14]. Additionally, miR-223-3p may be considered a biomarker for sepsis or a mediator of kidney injury and myocardial depression in patients with sepsis [Citation15–17]. The molecular biological mechanism of miR-223-3p in mediating the process of sepsis, however, is limited and much needs to be done to narrow the knowledge gap.
Hypoxia-inducible factor 1 (HIF-1), a crucial transcription factor, regulates organs or tissues facing low oxygen stress [Citation18]. Clinical conditions, metabolism, and cytokines influence HIF expression. Some studies have reported that HIF expression is significantly changed in patients with sepsis compared to that in healthy controls; Textoris and his coworkers found that the expression of HIF-1 is significantly upregulated in patients with septic shock [Citation19], while Schäfer et al. found an inverse correlation between decreased expression of HIF-1 and the severity of illness [Citation20]. HIF is also considered a potential target in the medical care of sepsis; 5,7-dihydroxy-8-methoxyflavone partly prevents sepsis-related acute lung injury by inhibiting the accumulation of HIF-1α [Citation21]. Similarly, treating lipopolysaccharide-induced septic mice with statin and simvastatin protects against liver dysfunction in the early stages of sepsis by decreasing transcript levels of HIF-1α [Citation22]. The possibility of specific inhibitors of HIF, such as miRNAs, need to be explored as therapeutic targets in sepsis.
In this study, we aimed to analyze co-regulated septic miRNAs with three independent miRNA expression profiles; additionally, target genes of co-regulated sepsis-related miRNAs were collected with public miRNA–mRNA interaction database; function analysis and expression of target genes were performed and examined to explore critical target genes regulated by miRNA associated with sepsis.
2 Methods and materials
2.1 Data processing
To analyze the differentially expressed miRNAs (DEmiRNAs) related to sepsis, we search GEO database with keywords including ‘sepsis’ and ‘septic’. Considering the limited sequencing experiments of miRNAs associated with sepsis, three matched septic miRNA expression and related clinical matrices were downloaded: GSE94717, with three healthy controls, six patients with sepsis, and six sepsis-induced patients with acute kidney injury (not used in this research); GSE149764 with eight healthy controls and 10 patients with sepsis; and GSE101639 with three normal controls, three patients with sepsis, and three patients with septic shock (not used in this analysis). Experiments with large samples (>30) were collected for test and validation process. An expression matrix associated with sepsis (GSE100159 containing 12 healthy controls and 35 patients with sepsis) was obtained to explore differentially expressed septic genes. To validate the collected candidate genes, another sepsis matrix, GSE13904, including 18 normal controls and 32 patients with sepsis was downloaded and analyzed; children with systemic inflammatory response syndrome (SIRS) and septic shock were not used in the validation process. To examine changes of targeted genes during early therapy response, GSE110487, a research on differentially expressed genes (DEGs) of two septic groups with different responses to early medical care, was collected and analyzed.
The expression matrix was normalized using the ‘quantile’ method via limma package [Citation23]; further, the matrix of gene information using official annotation files provided by submitters was integrated, and included: GSE94717, Exiqon miRCURY LNA microRNA Array, seventh-generation REV – hsa, mmu, & rno (miRBase v18.0) for GSE94717; GPL18573, Illumina NextSeq 500 (Homo sapiens) for GSE149764; GPL18058, Exiqon miRCURY LNA microRNA array, seventh generation (miRBase v18, condensed Probe_ID version) for GSE101639; GPL6884, Illumina HumanWG-6 v3.0 expression bead chip for GSE100159; GPL570, Affymetrix Human Genome U133 Plus 2.0 Array for GSE13904; GPL10999, Illumina Genome Analyzer IIx (Homo sapiens); and GPL16791, Illumina HiSeq 2500 (Homo sapiens) for GSE110487.
2.2 DEmiRNAs and DEGs analysis
The ‘sepsis-control’ contrast matrix was constructed and DEmiRNAs and DEGs were analyzed. The parameters, including |log2FC| > 1 and an adjusted P < 0.05, were used to identify DEmiRNAs and DEGs. All expression data of miRNAs were sorted based on log2FC and saved for co-regulated miRNA analysis.
2.3 Co-regulated miRNA analysis
The RobustRankAggreg package was used to perform integrative analysis of expression data obtained from the DEmiRNAs analysis [Citation24]. Two parameters |log2FC| > 1 and an adjusted Pe < 0.05 were used to classify statistically coexpressed miRNAs.
2.4 Candidate septic genes targeted by miR-223-3p
To obtain miR-223-3p target genes associated with sepsis, the miR-223-3p-bound genes were predicted using starBase v2.0, a web tool providing RNA–RNA and protein–RNA interaction networks from large-scale cross-linking immunoprecipitation sequencing data; the top 300 targets were collected and used for further analysis. Statistically upregulated expressed septic genes, observed from GSE100159, and the top 300 predicted targets were integrated to screen septic genes captured by miR-223-3p; overlapping genes were reserved for downstream analysis.
2.5 Gene function enrichment analysis of candidate septic genes
The functional analysis provided the global roles of the gene set. The database for annotation, visualization and integrated discovery (DAVID, https://david.ncifcrf.gov/) provides systematic and comprehensive information of genes. Gene function analysis was performed using DAVID based on 22 overlapping genes. In addition, three classes, biological process (BP), molecular function (MF), and cell component (CC)) were depicted using R package in circle and bar plots. Because KEGG enrichment analysis offers detailed pathway information for exploring the potential metabolism of gene clusters, KEGG analysis was conducted via DAVID using candidate genes. The web-based search tool for the retrieval of interacting genes/proteins (STRING, https://string-db.org/) supplies interaction information among proteins. Protein–protein interactions were performed using STRING based on candidate genes. The software Cytoscape (https://cytoscape.org/) is an efficient Java software used to visualize networks and integrate data, while molecular complex detection (MCODE), a high-efficient plugin of Cytoscape, helps researchers explore gene clusters in complex networks. Gene clusters were identified using MCODE via the ‘Degree’ method with default parameters.
2.6 Validation of candidate key genes
To validate crucial septic target genes of miR-223-3p, the expression of target genes was examined using the sepsis matrix (GSE13904) and target gene expression was compared between sepsis responders and sepsis nonresponders in early therapeutic treatments. To examine the specificity and sensitivity of crucial septic target genes in order to distinguish groups (patients with sepsis vs. healthy controls, sepsis responders vs. nonresponders), the area under the curve (AUC) of the receiver operating characteristic (ROC) curve was analyzed.
2.7 Immune infiltration analysis
To explore the different immune cells’ distribution between patients with sepsis and healthy controls or sepsis with/without response to therapy, we checked the fraction of 22 immune cells using a web-based tool CIBERSORTx (https://cibersortx.stanford.edu/), which estimates the immune cell abundances according to different sample groups. The fraction of 22 immune cell types were visualized using a heatmap and boxplot. We used ggplot to analyze and visualize the correlation between crucial genes and different immune cells.
2.8 GSEA of MKNK1
To examine the enriched pathways of MKNK1, patients with sepsis were divided into two groups according to the median expression of MKNK1. The GSEA was conducted via clusterProfiler package [Citation25]. The P-value was set 0.05, other parameters were set by default.
2.9 Statistical analysis
The significance of DEGs was calculated with limma package [Citation23]; significance of GSEA was calculated with clusterProfiler package [Citation25]; P-value of co-regulated miRNAs was calculated and adjusted with Bonferroni correction method in R (https://www.r-project.org/).
3 Results
3.1 Co-regulated septic miRNAs
After normalization ()), one coexpressed miRNA, miR-223-3p, was identified based on DEmiRNAs and co-regulation analysis. miR-223-3p expression was statistically decreased in GSE94717, while a tendency of decreased miR-223-3p expression was observed in GSE101639 and GSE149764 ()), though it was not significantly different.
3.2 DEGs related to sepsis
A total of 865 upregulated and 1030 downregulated genes were obtained in GSE100159; the top 20 genes of each set were visualized using a heatmap plot (). Considering that there was a negative relationship between the expression of miRNAs and their target genes, the 865 upregulated genes ()) were utilized for integrative analysis.
Figure 2. Heatmap of differentially expressed genes (DEGs). The top 20 upregulated and downregulated genes, examined in GSE100159, are visualized in the heatmap plot. The X-axis indicates samples, while the Y-axis represents genes. Gene expression is ranked from dark blue (low expression) to red (high expression) color. Samples are divided into two groups: patients with sepsis (pink) and healthy controls (light blue)
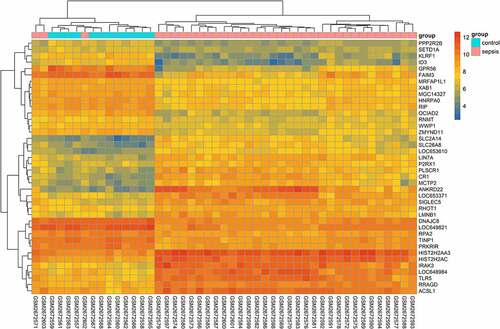
3.3 Functional analysis of septic miR-223-3p target genes
Considering the negative correlation of miRNAs and target genes, we mainly collected upregulated DEGs. A total of 22 overlapping septic upregulated genes were obtained in GSE100159, targeted by miR-223-3p (), ). These overlapping genes were enriched in biological processes (BP), such as the metabolic process of reactive oxygen (GO:2000378), immunity pathways like the interferon (IFN)-γ signaling pathway (GO:0060333) and target of rapamycin (TOR) signaling (GO:0032007); cellular components (CC) like the cytosol (GO:0005829), Golgi membrane (GO:0000139), and endoplasmic reticulum membrane (GO:0005789); and molecular functions (MF), such as GTP binding (GO:0005525), GTPase activity (GO:0003924), and Hsp90 protein binding (GO:0051879), as depicted by a circle plot ()) and a bar plot ()). The candidate genes were part of five KEGG pathways (); three pathways were statistically enriched, including central carbon metabolism associated with cancer (hsa05230), HIF-1 signaling (hsa04066), and galactose metabolism (hsa00052).
Table 1. Overlapping septic gene expression. The table lists the expression information of 22 upregulated target genes in GSE100159 that are associated with sepsis
Table 2. The Kyoto Encyclopedia of Genes and Genomes (KEGG) pathways of targeted genes. The table shows the KEGG functional analysis of miR-233-3p target genes constructed using the database for annotation, visualization and integrated discovery (DAVID)
3.4 PPIs of miR-223-3p targets associated with sepsis
Interactions of protein genes, forming a molecular machine, play a critical role in regulating sepsis. The interactions among several genes, such as HK2, Ras-related protein Rab-33B (RAB33B), HIF1A, and Ras-related protein Rab-1A (RAB1A) were identified (). One of which, HK2, a key regulator of glucose metabolism pathways, was considered a candidate hub gene.
3.5 Validation of crucial septic miR-223-3p target genes
The expression of target genes was examined in GSE13094; a total of 102 downregulated genes and 276 upregulated genes were obtained in GSE13094; the top 20 genes of each set were depicted in the heatmap plot (). One of the target genes – MKNK1 – was statistically upregulated in patients with sepsis in GSE13904 ()) and its AUC was 0.979 ()). The expression of HK2 and HIF1A ()) was upregulated, though not significantly; their AUC scores were 0.921 and 0.902 ()), respectively. MKNK1 expression in patients with sepsis that responded to early (48 h) therapeutic treatment was tremendously downregulated ()); its AUC score was 0.748 ()).
Figure 5. Heatmap of differentially expressed genes (DEGs) in GSE13904. The heatmap shows the expression of the top 20 upregulated and downregulated genes. The X-axis and Y-axis indicate samples and genes, respectively. The color gradation represents the expression of genes – from dark blue (low expression) to red (high expression). The two groups are annotated using two colors: pink for patients with sepsis and light blue for healthy controls. To clarify the heatmap, gene expression has been transformed using the log2 (1+ expression) method
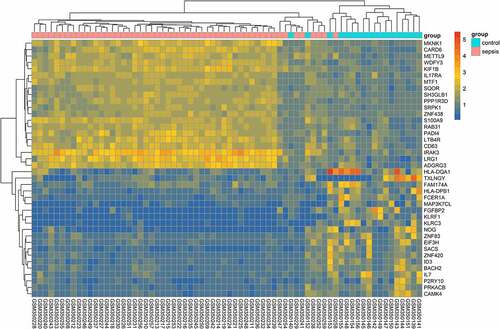
Figure 6. Crucial target genes expression level and receiver operating characteristic (ROC) curve. A depict the gene expression of hypoxia-inducible factor 1-alpha (HIF1A), MAP kinase-interacting serine/threonine-protein kinase 1 (MKNK1), and hexokinase 2 (HK2); B plots the expression of MKNK1 before and after early therapy treatment; C represent the area under the curve (AUC) of the ROC curve for HIF1A, MKNK1, and HK2 to divide subjects into patients with sepsis and healthy controls; D depicts the discriminability of responders and nonresponders of patients with sepsis
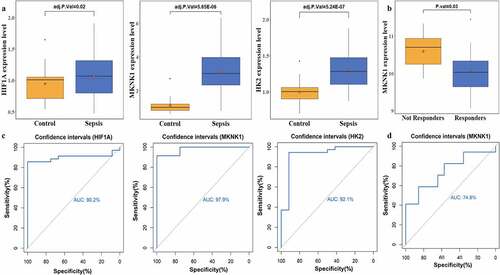
3.6 Immune infiltration analysis
To examine the different immune cell distribution between patients with sepsis and healthy controls, we estimated the immune cell abundances. Different type of immune cells showed different levels of correlation ()). MKNK1 showed higher correlation with neutrophils (). T cells regulatory (Tregs), monocytes, macrophages M0, and neutrophils immune cells were extremely significantly accumulated ()).
Figure 7. Distributions of immune cells with sepsis. A indicated the correlation map of 22 immune cells; B represented the correlation of three targeted genes and immune cells with different distribution between sepsis and healthy people. C and D showed the heatmap and boxplots of immune cells in people with sepsis (red) and healthy controls (blue)
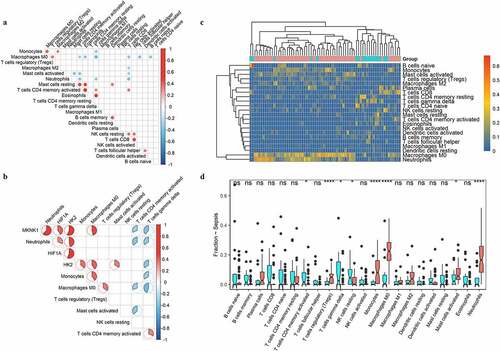
The immune cells distribution between sepsis with/without response to therapy showed that neutrophils and monocytes had significant accumulation (). MKNK1 showed a significant correlation with neutrophils (cor = 0.526, p = 0.002) ()). GSEA showed that MKNK1 significantly enriched in cell cycle and ribosome pathways ()).
Figure 8. Immunity analysis in sepsis under therapy. The distributions of immune cells among septic samples with (red) or without (blue) response to therapy were depicted with heatmap (a) and boxplots (b). The correlation of MKNK1 expression and fraction of neutrophils was visualized with scatter plot (c); top 3 enriched pathways obtained from GSEA were showed in D
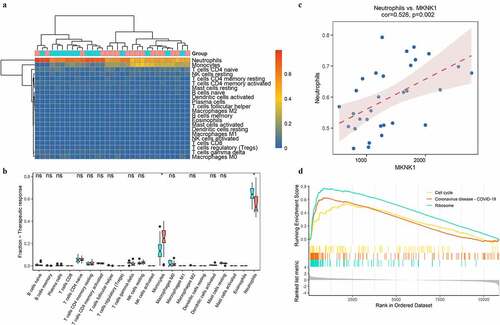
4 Discussion
Our study found that the expression of one miRNA, miR-223-3p, was inhibited in patients with sepsis; the miR-223-3p expression significantly decreased in GSE94717 ()). A trend for decreased miR-223-3p expression was also observed in patients with sepsis in two other sepsis experiments: GSE101639 ()) and GSE149764 ()), even though it was not significant. Previous studies have found that inflammation, myocardial dysfunction, and mortality, induced by sepsis, are stimulated and irritated in mice with miR-223-3p deletion [Citation17]. Zhang et.al observed the differential expression of miR-223-3p in septic myocardial depression [Citation26]. Benz and his coworkers, however, found there’s no significant correlation between circulating miR-223 and cecal pole ligation and puncture (CLP)-induced septic mice[Citation27]. Another team over-expressed miR-223 in mice and found that it could remit LPS-induced sepsis mouse model [Citation28]. These indicate the complication of sepsis and various roles of miR-223 in different sepsis. Based on the integration of the top 300 predicted target genes and upregulated genes in patients with sepsis in GSE100159, 22 overlapping target genes were identified (), ), including solute carrier family 36 member 1 (SLC36A1), MKNK1, acyl-CoA synthetase long-chain family member 3 (ACSL3), RAB1A, HK2, ubiquitin-protein ligase E3 component n-recognin 1 (UBR1), SEC24 homolog D, COPII coat complex component (SEC24D), lamin B receptor (LBR), membrane bound O-acyltransferase domain containing 2 (MBOAT2), guanylate-binding protein 1 (GBP1), speckled protein of 100 kDa (SP100), ubiquitin thioesterase OTU1 (YOD1), HIF1A, KH domain containing RNA binding (QKI), zinc finger DHHC-type palmitoyltransferase 20 (ZDHHC20), activated leukocyte cell adhesion molecule (ALCAM), Jun dimerization protein 2 (JDP2), phosphoglucomutase 2 (PGM2), sperm-specific antigen 2 (SSFA2), solute carrier family 16 member 3 (SLC16A3), cut-like homeobox 1 (CUTL1), and RAB33B.
The GO enrichment analysis showed that the candidate genes primarily participate in reactive oxygen and immunity pathways, such as IFN-γ and TOR signaling pathways ()). The KEGG analysis indicated three pathways: central carbon metabolism associated with cancer, HIF-1 signaling, and galactose metabolism (). Three genes involved in HIF-1 signaling and regulating hypoxia (low oxygen) were interesting. Previous studies have examined HIF-1 expression using whole blood samples and found that HIF-1 is significantly stimulated in patients with septic shock [Citation19]. In this study, the upregulation of three HIF-1 signaling genes – HIF1A, HK2, and MKNK1 – were similarly detected in patients with sepsis in two independent experiments (GSE100159 and GSE13904), and they had good AUC scores of 0.902, 0.921, and 0.979, respectively, using ROC analysis ()). Peyssonnaux and Fitzpatrick have found that the expression of HIF-1 influences the clinical features of sepsis, such as tachycardia, hypotension, and hypothermia via changing proinflammatory cytokines [Citation29,Citation30]. Kiss et al. have found that loss of PHD3, an enzyme inhibiting HIF, leads to an irresistible innate immune response and causes significantly higher mortality rate in mice with abdominal sepsis [Citation31]. Several studies have reported that HIF may be considered a potential therapeutic target; medical elements like Edaravone, 5,7-dihydroxy-8-methoxyflavone, and simvastatin influence the expression of HIF-1α and mediate organs in response to sepsis [Citation21,Citation22,Citation32].
Despite the development of medical care, sepsis remains one of the major causes leading to death in ICUs. Pittet, et al. have found that almost all patients in the surgical intensive care unit are accompanied by SIRS, a whole-body reaction, many of which may be equipped with infection-positive diseases (i.e., sepsis) [Citation33]. Biomarker-guided therapy, usually used in complex diseases, such as cancer and chronic heart failure, also has drawn the attention of researchers in sepsis [Citation34]. The performance of the three HIF-signaling genes was evaluated in response to early therapeutic treatment of patients with sepsis in the ICU. One of them, MKNK1, was significantly downregulated in septic shock responders treated within 48 h after ICU admission ()) and had good specificity and sensitivity in distinguishing between responders and nonresponders with septic shock having received early medical care ()); however, there was no change in the expression of HIF-1A and HK2. Given the different roles of HIF signaling in different cell types and the time-dependent aspects after treatment, further work is required to examine the potential of HIF as a biomarker in patients with sepsis with early treatment. The performance of three HIF-1 signaling genes in evaluating the outcomes of therapy in sepsis was examined using two experiments profiling the expression of the transcriptome (GSE54514 and GSE63042) of survivors and non-survivors of patients with sepsis; no statistical changes were identified. Similarly, Textoris et al. have reported the independence of the expression of HIF-1 and outcomes of patients with sepsis [Citation19]. Höcker and his coworkers have further announced that the expression of HIF-1α cannot alter 30-day mortality in severe sepsis [Citation35].
Neutrophils and monocytes were significantly accumulated in patients with sepsis vs. healthy controls or patients with sepsis with response vs. those without response to therapy (). The previous research showed that neutrophils are important players in the outcome of sepsis, as the neutrophil antibacterial arsenal contributes to tissue damage and the development of organ dysfunction during sepsis [Citation36]. Wang et al. found significant downregulation of miR-223(3p) and miR-223(5p) in hearts of mouse with sepsis which aggravates the inflammation induced by sepsis [Citation17]. Previous research found that expression of MKNK1, involved in mRNA translational regulation was upregulated in patients 25 to 48 hours before sepsis conversion which boosts the formation of eIF-4 F, a critical trait in initiation of translation [Citation37]. MKNK1 was reported engaged in genesis of pro-inflammatory mediators; function experiment showed that the expression of MKNK1 is crucial element in the accumulation of genes involved in inflammation such as IL6, C-X-C motif chemokine ligand 8 (CXCL8), and prostaglandin-endoperoxide synthase 2 (PTGS2) [Citation38]. MKNK1 was significantly positively associated with neutrophils () and upregulated in patients with sepsis compared to healthy controls ()). These indicate that potential role of MKNK1 in boosting the accumulation of neutrophils which contributes to the aggravation of inflammation caused by downregulation of miR-223-3p. Compared with patients without response to therapy, MKNK1 expression was significantly down-regulated in responders with sepsis under medical treatments ()); proportion of neutrophils was significantly decreased in patients with response to therapy ()) which indicates role of MKNK1 in regulating inflammation status under therapy and potential of MKNK1 as indicator representing the effects of medical treatments for patients with sepsis. Our results provide a novel inflammation-related networks miR223-3p, MKNK1, and neutrophils in sepsis.
5 Conclusion
In conclusion, miR-223-3p was detected significantly downregulated in patients with sepsis. Three miR-223-3p target genes involved in HIF-1 signaling (HIF1A, HK2, and MKNK1) were found significantly upregulated, which could be used to distinguish patients with sepsis from healthy controls. Neutrophils significantly positively correlated with MKNK1, were significantly accumulated in patients with sepsis vs. healthy controls or sepsis with response compared to those without response to therapy. MKNK1, negatively regulated by miR-223-3p, boosted the expression of inflammation-related factors causing accumulation of neutrophils which leads to aggravation of inflammation in patients with sepsis.
Disclosure of potential conflicts of interest
No potential conflict of interest was reported by the author(s).
Availability of data and material
The datasets used and/or analyzed during this study are available from the corresponding author upon reasonable request.
Authors’ contributions
Mingmin Fu conducted most of the analysis of data. Mingmin Fu and Kai Zhang wrote and revised the manuscript; All authors read and approved the final manuscript.
References
- Fleischmann C, Scherag A, Adhikari NK, et al. Assessment of global incidence and mortality of hospital-treated sepsis. Current estimates and limitations. Am J Respir Crit Care Med. 2016;193:259–272.
- Cohen J. The immunopathogenesis of sepsis. Nature. 2002;420:885–891.
- Tiru B, DiNino EK, Orenstein A, et al. The economic and humanistic burden of severe sepsis. Pharmacoeconomics. 2015;33:925–937.
- Reinhart K, Daniels R, Kissoon N, et al. Recognizing Sepsis as a Global Health Priority - A WHO Resolution. N Engl J Med. 2017;377:414–417.
- Weng L, Zeng XY, Yin P, et al. Sepsis-related mortality in China: a descriptive analysis. Intens Care Med. 2018;44:1071–1080.
- Guo H, Ingolia NT, Weissman JS, et al. Mammalian microRNAs predominantly act to decrease target mRNA levels. Nature. 2010;466:835–840.
- Liu G, Abraham E. MicroRNAs in immune response and macrophage polarization. Arterioscler Thromb Vasc Biol. 2013;33:170–177.
- Essandoh K, Li Y, Huo J, et al. MiRNA-mediated macrophage polarization and its potential role in the regulation of inflammatory response. Shock. 2016;46:122–131.
- Chen L, Xie W, Wang L, et al. MiRNA-133a aggravates inflammatory responses in sepsis by targeting SIRT1. Int Immunopharmacol. 2020;88:106848.
- Tong L, Tang C, Cai C, et al. Upregulation of the microRNA rno-miR-146b-5p may be involved in the development of intestinal injury through inhibition of Kruppel-like factor 4 in intestinal sepsis. Bioengineered. 2020;11:1334–1349.
- O’Neill LA, Sheedy FJ, McCoy CE. MicroRNAs: the fine-tuners of Toll-like receptor signalling. Nat Rev Immunol. 2011;11:163–175.
- Regouc M, Belge G, Lorch A, et al. Non-coding microRNAs as novel potential tumor markers in testicular cancer. Cancers (Basel). 2020;12:749.
- Jimenez Calvente C, Del Pilar H, Tameda M, et al. MicroRNA 223 3p negatively regulates the NLRP3 inflammasome in acute and chronic liver injury. Mol Ther. 2020;28:653–663.
- Bauernfeind F, Rieger A, Schildberg FA, et al. NLRP3 inflammasome activity is negatively controlled by miR-223. J Iimmunol. 2012;189:4175–4181.
- Zhang W, Jia J, Liu Z, et al. Circulating microRNAs as biomarkers for Sepsis secondary to pneumonia diagnosed via Sepsis 3.0. BMC Pulm Med. 2019;19:93.
- Colbert JF, Ford JA, Haeger SM, et al. A model-specific role of microRNA-223 as a mediator of kidney injury during experimental sepsis. Am J Physiol Renal Physiol. 2017;313:F553–F9.
- Wang X, Huang W, Yang Y, et al. Loss of duplexmiR-223 (5p and 3p) aggravates myocardial depression and mortality in polymicrobial sepsis. Biochim Biophys Acta. 2014;1842:701–711.
- Kaelin WG Jr., Ratcliffe PJ. Oxygen sensing by metazoans: the central role of the HIF hydroxylase pathway. Mol Cell. 2008;30:393–402.
- Textoris J, Beaufils N, Quintana G, et al. Hypoxia-inducible factor (HIF1alpha) gene expression in human shock states. Crit Care. 2012;16:R120.
- Schäfer ST, Frede S, Winning S; et al. Hypoxia-inducible factor and target gene expression are decreased in patients with sepsis: prospective observational clinical and cellular studies. Anesthesiology. 2013;118:1426–1436.
- Sun HL, Peng ML, Lee SS, et al. Endotoxin-induced acute lung injury in mice is protected by 5,7-dihydroxy-8-methoxyflavone via inhibition of oxidative stress and HIF-1alpha. Environ Toxicol. 2016;31:1700–1709.
- Yorulmaz H, Ozkok E, Erguven M, et al. Effect of simvastatin on mitochondrial enzyme activities, ghrelin, hypoxia-inducible factor 1alpha in hepatic tissue during early phase of sepsis. Int J Clin Exp Med. 2015;8:3640–3650.
- Ritchie ME, Phipson B, Wu D, et al. limma powers differential expression analyses for RNA-sequencing and microarray studies. Nucleic Acids Res. 2015;43:e47.
- Kolde R, Laur S, Adler P, et al. Robust rank aggregation for gene list integration and meta-analysis. Bioinformatics. 2012;28:573–580.
- Yu G, Wang LG, Han Y, et al. clusterProfiler: an R package for comparing biological themes among gene clusters. OMICS. 2012;16:284–287.
- Zhang TN, Yang N, Goodwin JE, et al. Characterization of circular RNA and microRNA profiles in septic myocardial depression: a lipopolysaccharide-induced rat septic shock model. Inflammation. 2019;42:1990–2002.
- Benz F, Tacke F, Luedde M, et al. Circulating microRNA-223 serum levels do not predict sepsis or survival in patients with critical illness. Dis Markers. 2015;2015:384208.
- Dang CP, Leelahavanichkul A, Mukhopadhyay P. Over-expression of miR-223 induces M2 macrophage through glycolysis alteration and attenuates LPS-induced sepsis mouse model, the cell-based therapy in sepsis. PloS One. 2020;15:e0236038.
- Peyssonnaux C, Cejudo-Martin P, Doedens A, et al. Cutting edge: essential role of hypoxia inducible factor-1alpha in development of lipopolysaccharide-induced sepsis. J Iimmunol. 2007;178:7516–7519.
- Fitzpatrick SF, Gojkovic M, Macias D, et al. Glycolytic response to inflammation over time: role of myeloid HIF-1alpha. Front Physiol. 2018;9:1624.
- Kiss J, Mollenhauer M, Walmsley SR, et al. Loss of the oxygen sensor PHD3 enhances the innate immune response to abdominal sepsis. J Iimmunol. 2012;189:1955–1965.
- He C, Zhang W, Li S, et al. Edaravone improves septic cardiac function by inducing an HIF-1alpha/HO-1 pathway. Oxid Med Cell Longev. 2018;2018:5216383.
- Pittet D, Rangel-Frausto S, Li N, et al. Systemic inflammatory response syndrome, sepsis, severe sepsis and septic shock: incidence, morbidities and outcomes in surgical ICU patients. Intensive Care Med. 1995;21:302–309.
- Reinhart K, Bauer M, Riedemann NC, et al. New approaches to sepsis: molecular diagnostics and biomarkers. Clin Microbiol Rev. 2012;25:609–634.
- Hocker A, Rabeling M, Bick A, et al. Hypoxia inducible factor-1 alpha and prolinhydroxlase 2 polymorphisms in patients with severe sepsis: a prospective observational trial. BMC Anesthesiol. 2016;16:61.
- Sonego F, Castanheira FV, Ferreira RG, et al. Paradoxical roles of the neutrophil in sepsis: protective and deleterious. Front Immunol. 2016;7:155.
- Johnson SB, Lissauer M, Bochicchio GV, et al. Gene expression profiles differentiate between sterile SIRS and early sepsis. Ann Surg. 2007;245:611–621.
- Liong S, Lappas M. Markers of protein synthesis are increased in fetal membranes and myometrium after human labour and delivery. Reprod Fertil Dev. 2018;30:313–329.