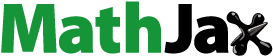
ABSTRACT
This study investigates the military outlays effects on human capital development in sub-Saharan Africa. By using panel data covering 44 countries from 1995 to 2020, a system Generalized Method of Moments is adopted as the estimation technique of a dynamic panel model. Findings show that military outlays have a significant positive effect on education spending in sub-Saharan Africa. However, the sensitivity analysis reports a mitigated result, highlighting the diversity of evidence on the ‘guns better than butter’ literature. While military outlays positively affect education expenditures in central and southern African sub-regions, they crowd-out the public spending allocated to education development in Sahel & Lake Chad area, western and eastern sub-regions. These results, therefore, suggest that policy makers should promote the approach which considers defense as a component of socioeconomic development by regulating the perfect ratio of military spending that meets their needs.
1. Introduction
In their popular book entitled Introduction to Modern Economics edited in 1973, Robinson and Eatwell (Citation1973) assert that ‘it is impossible to analyze economic problems without considering the role of arms spending’. This standpoint highlights the paramount role of military spending in economic development. Therefore, the socioeconomic effects of military outlays are an important issue for developing countries, such as sub-Saharan Africa (SSA, henceforth) countries. These countries are dealing with poverty, radicalization and violent extremism.Footnote1 The security challenges involve a strong militarization, thus increasing military expenditures at the expense of health or education spending. Due to budgetary trade-off, high defense outlays are afforded at the expense of spending in social program. According to Robinson (Citation1971), military outlays monopolize resources that could have met basic needs such as education and health.Footnote2
On a conceptual view, defense spending is the share separated by states from their national income in order to provide its security against internal and external threats. Thus, armed conflict can affect the composition of government spending (Gupta, Verhoeven, and Tiongson Citation2002). Stiglitz and Boadway (Citation1994) define human capital as the stock of accumulated skills and experiences that make workers more productive. The concept of Human Capital Development (HCD, henceforth) has been actively developed by the United Nations since the end of the twentieth century, the main principle of which is to expand the opportunities of each person to realize their potentials and aspirations, to live a healthy, full-fledged and creative life (Sagikyzy, Shurshitbay, and Akhmedova Citation2021). In HCD, education and health are essential. The importance of investing in education and health is well appreciated and understood in economies having the objective of attaining sustainable growth. HCD remains the centerpiece of any meaningful economic growth and development program that may be pursued by any nation.
From a historical perspective, defense spending is sometimes seen as a windfall or as a burden for economic development (Schméder Citation2013). This debate traditionally opposes Smithians to Keynesians. In his essay: ‘The Wealth of Nations’, Smith (1776) writes ‘the first duty of the sovereign which is that of protecting the society from the violence and invasion of other independent societies can only be performed only by means of a military force’. Thus, military spending incurred in preparing the military force in time of peace, and employing it in time of war, is essential in ensuring national security, which is a necessary condition for development and economic growth. War appears to be the only legitimate excuse for creating a fiscal deficit. Public spending therefore generates potential ills, the worst of which is an increase in public debts, which weighs on future generations. Moreover, the Keynesian theory considers that public expenditure has positive economic effects, which are linked, in particular to their multiplier effects on the economy, to the capacity that they offer to governments in the regulation of local activity (Keynes Citation1939).Footnote3 A war accompanied by the spending of supplying, ammunition and services relating to the setting up of a country on a war footing makes the depression disappear and brings prosperity (Kalecki Citation1943).
The effects of military spending are obvious facts. Krugman (Citation2011) argues that military spending creates jobs when the economy is depressed: ‘spending on bombs is as good as spending on public parks’. Beyond their stimulating effects on employment, military spending, although insensitive to the economy, generate positive effects on exports. However, this military KeynesianismFootnote4 is not unanimous (Melman Citation1971; Gold Citation2005; Swanson Citation2012). In SSA countries, the military outlays trends raise complex questions regarding development, security and governance. The data on military outlays trend are alarming. According to Stockholm International Peace Research Institute (SIPRI), military outlays increased from 2011 to 2020 in African countries where several armed conflicts are ongoing. In the Sahel region,Footnote5 the biggest increases in military spending were made by Mali (339%), Niger (288%), and Burkina Faso (238%) in fighting terrorist networks. In the other countries, military outlays increased from 2010 to 2020 by 276% in Somalia, 190% in Mozambique, 52% in Uganda and 25% in Kenya. Over the past 32 years (1988–2020), all 54 African countries have spent around 892 billion, whereas the SSA region has spent about 515 billion.
Nevertheless, education outcomes at the glance show evidence of low enrollment and completion rates for all education levels (Barro and Lee Citation2016; Farayibi and Folarin Citation2021). From 2000 to 2019, the average school completion rate for the primary stood at 16.7%, 9.7% for secondary and 1.53% for the tertiary. Within this same period, the average years of school completed were 3.37% for primary, 1.27% for secondary and 0.08% for tertiary education (WDI, Citation2020). These figures might explain the high rate of out-of-school children, which undermine the stock of human capital in the region. If public expenditures on education seem to be the biggest challenge in this region, high military expenditure and ongoing conflicts in SSA could account for the diversion of resources that would have been used for social welfare financing. From the above considerations, especially theoretical debate and empirical facts, the question then remains what is the effect of military outlays on education spending in SSA? Addressing this question will give a new lease to effective policymaking in SSA.
The main objective of this study is to examine the effect of military outlays on education spending. The previous studies related to military outlays effects have currently focused on three broad themes. One group of studies analyzed the relationship between military outlays and economic growth since the pioneering work of Benoit (Citation1978) and other authors (Ali and Dimitraki Citation2014; Churchill and Yew Citation2018). The second group has focused on the relationship between military outlays and human development, such as education and health (Kentor and Jorgenson Citation2017; Fan, Liu, and Coyte Citation2018; Coutts et al. Citation2019; Luqman and Antonakakis Citation2021; Wang Citation2022). The third group has concentrated on military spending and physical capital or Research & Development nexus (Flamm Citation1988; Gold Citation1991). Overall, there are several aspects of this field of research that warrant further exploration. Following the current studies, the main hypothesis is that military outlays exert a differentiated effect on education development in SSA. Hence, the contribution of this paper is twofold. First, we provide new cross-country evidence on the budgetary trade-off between military outlays and human capital spending. This subject matter is still far from being over. Second, our results constitute a significant departure from previous studies by highlighting the fact that the crowding-out effect is statistically more specific to some African sub-regions. This analysis is relevant due to the fact that some fragile and state-based conflicts are located in SSA.
The rest of the paper proceeds as follows. In section two, we review the results from the literature on the guns-and-butter trade-off. In section three, we present some stylized facts on human capital development in the SSA region. In Section four, we discuss the data to be used and econometric methodology. In section five, we present the empirical findings with insights into the possible explanations for the findings. Finally, the paper closes with a discussion of policy implications and future research.
2. Guns versus butter literature: bedfellows or antagonists between military spending and education development
The widespread public view is that in a region renowned for protracted terrorism, policy makers have prioritized military and security expenditures, which has led to the subsequent neglect of social welfare spending, thereby increasing poverty and inequalities. The argument is that, overall military outlays compete with civilian expenditures for overall government spending (Deger Citation1985; Gifford Citation2006).Footnote6 The existence of a trade-off between guns and butter asserting that spending on defense comes at the expense of the social welfare expenditures (Russett Citation1969; Peroff and Podolak-Warren Citation1979; Deger Citation1985; Apostolakis Citation1992). Given the nature of military expenditure as a sunk cost, outlays on industrial investment, education, and health have been suggested as better investment options in the guns versus butter literature (Bullock and Firebaugh Citation1990). Like American economies, many regions and countries have attracted attention from authors’ explorers of the guns-butter trade-off. However, their findings are equivocal. While Sandler and Hartley (Citation1995) showed from the results of 25 research studies conducted from 1970 to 1993 that the defense spending impacts are differentiated, Dunne and Uye (Citation2009) came to a conclusion after the analysis of 103 research studies that defense outlays would have a negative or unclear impact on the national economy. From the empirical view, the debate on guns versus butter highlights four possible categories of contradictory results: one depressing thanks to the crowding-out, one stimulatory thanks to the multiplier, ambiguous effect, and no trade-off evidence.
Firstly, scholars have established evidence of the crowding-out effect of military spending on the social sectors (Apostolakis Citation1992; Ozsoy Citation2002; Blomberg, Hess, and Athanasios Citation2004; Bove, Efthyvoulou, and Navas Citation2017; Fan, Liu, and Coyte Citation2018). The social sectors absorb a non-negligible part of the State’s resources and any increase in the shares of other sectors, such as the army is only possible at the expense of health and education given the budgetary constraint. The pioneering work by Russett (Citation1969) reports that military spending in the US can crowd-out education and healthcare expenditures; while Dabelko and McCormick (Citation1977) confirmed the existence of crowding-out effect for several developing countries over the period 1950–1972 and Apostolakis (Citation1992) for 19 Latin American countries. Adeola (Citation1996) reports a crowding-out of military outlays for a sample of 60 developing countries in 1985; and Abdul, Mohd, and Dewi (Citation2015) show that military spending created a threat to the educational system for five countries in South Asia between 1980 and 2013. Fan, Liu, and Coyte (Citation2018) found that increased military spending has a significant negative impact on both the publicly financed and total health expenditures of 197 countries over the period 2000–2013. These findings strongly support the crowding-out hypothesis whereby increased military outlays reduce the capacity of the government to spend on health. More recently, Luqman and Antonakakis (Citation2021) examined the relationship between military expenditure, human development, and economic growth in Pakistan for the period from 1965 to 2016. Applying the Quantile Autoregressive-Distributed Lag method, findings confirm a negative association between human capital development and military outlays in Pakistan. By using the system generalized methods of moments estimations, Ikegami and Wang (Citation2022) find that military spending has a significant crowding-out effect on domestic government health spending in 116 countries (including a subsample of 87 non-OECD countries) over the period 2000–2017. The less well-off countries stand to suffer more than wealthy ones from the crowding-out effect.
Secondly, in line with military Keynesianism, a set of studies confirm the complementarity hypothesis (bedfellow) between social and military outlays (Harris and Pranowo Citation1988; Kollias and Paleologou Citation2011; Lin, Ali, and Lu Citation2015). These studies contrast with the butter-versus-guns theory. Military spending can enhance better education, health and nutrition, which are all important factors that can raise income and productivity levels (Looney Citation1990). In addition, they highlight the indirect effect of military spending on social sectors via investment, growth, quality of training in the army and especially technological innovations. Military spending on research & development increases the productivity of the civilian sector by generating new infrastructure and advanced technology. For example, Lin, Ali, and Lu (Citation2015) through the panel Generalized Method Of Moments (GMM) found a significant positive impact of military outlays on health-care and education spending in a sample of 29 OECD countries over the period 1988–2005. This positive crowding-out effect implies that an increase in military spending also promotes health and education spending, suggesting a complementary relationship between these variables. The main reason for this relationship is the general demand increase created by the generosity of governments for defense, as well as the demand for health caused by military reasons. Moreover, in line with military Keynesian doctrine, increased military demand for goods and services is generated directly by government spending, which induces a multiplier effect of general consumer spending and, therefore, increases in education and health spending.
Thirdly, another group of studies generates ambiguous effects of military spending. According to Ali (Citation2011), Yildirim and Sezgin (Citation2002), Frederiksen and Looney (Citation1994), Peroff (Citation1976) and Caputo (Citation1975), the effect depends on the social welfare indicator. Caputo (Citation1975), for instance, reports a complementary relationship in which defense and welfare spending increase or decrease simultaneously. According to the research, US defense spending is shown to be positively correlated with healthcare spending but not related to education spending. In Australia and Sweden, defense spending always correlates positively with education and healthcare expenditures. In Britain, defense spending is shown to squeeze out resources from education but has no significant impact on healthcare. Yildirim and Sezgin (Citation2002) find out that in Turkey, although the trade-off is negative between defense and health, there is complementarity between defense and education spending. Ali (Citation2011) shows that in Egypt, military spending crowds out health spending but not education and food subsidies. The effect on health expenditure is, therefore, opposite to that on education, so that by combining education and health as social expenditure, the overall effect is very small. According to Zhang et al. (Citation2016), results from developed economies and newly emerging economies are also conflicting. Using a panel co-integration analysis over two time periods, 1998–2011 and 1993–2007, they show that military spending enhances social welfare expenditures in G7 countries (i.e. the US, Japan, Germany, the UK, France, Italy, Canada), while the effect is ambiguous in BRICS (i.e. Brazil, Russia, India, China, South Africa). Wang (Citation2022) examines defense-education and defense-healthcare relations in the US and Britain. He discovers that defense welfare trade-off is more likely to occur between defense and education spending than between defense and healthcare spending in the US and Britain.
Fourthly, scholars report no evidence for guns versus butter trade-offs. The share of the budget allocated to defense and social sectors depends on different factors (Russett Citation1982; Davis and Chan Citation1990; Xu et al. Citation2018; Coutts et al. Citation2019). The research of Clayton (Citation1976) affirmed that in the United States, from 1900 to 1975, there has not been a significant correlation between defense and social spending since the Korean conflict, but large wars did have a notable negative impact on welfare spending. Peroff and Podolak-Warren (Citation1979) found that in the United States from 1929 to 1974 evidence points to no trade-off between federal health and defense in allocations or final expenditures during war or peace, although a trade-off existed between the private health and defense sectors only in terms of capital investment in health. Domke, Eichenberg, and Kelleher (Citation1983), Frederiksen and Looney (Citation1994), Ali (Citation2011) could not detect any kind of trade-off relation in military and health spending. Coutts et al. (Citation2019) ran panel data from 1995 up to the start of the Arab Spring in 2011 through some detailed statistical models to explore the trade-off between spending on military and on welfare – health, in this case – of 18 different MENA nations from 1995. They found no statistically significant evidence that increased military spending had an impact on health investment. Contrary to previous evidence from MENA region (Bauwens Citation2012), they found that military expenditure did not induce cuts to healthcare in the Middle East and North Africa. Biscione and Caruso (Citation2021) found that the once-lagged military outlays-total government expenditure ratio has no significant effect on current health expenditure in a sample of 26 transition countries over the period 1990–2015.
Overall, this literature review loudly voiced the importance of discussions about the impact of military outlays. These inconclusive findings suggest the need for further research.
3. Human capital development: sub-Saharan Africa’s greatest challenge
Human capital accumulation can be stimulated in developing countries through public education expenditures as well as government spending on health and other social services. Clearly, governments are the most important agencies in this area and can do much more than private enterprises could ever hope to achieve. In the third-world countries, especially in Africa, governments continue to allocate, on average, over 5% of their GDP to defense outlays. Generally, the patterns of military expenditures in SSA have changed over the past three decades (see ).
The patterns of military outlays show an upward trend from 1964 to 2020. They have been generally increasing in SSA since the 1990s, despite numerous development challenges in the region. The security crises faced by some countries in the region in recent decades have led to a very significant increase in military spending by countries in the region. For example, the outbreak of the war in Mali in 2012 led to a tripling of the budgetary effort in favor of its defense in the following years. The spread of the Malian crisis to Burkina Faso explains the doubling of Burkinabe military spending in 2016.
However, military outlays as a proportion of GDP (military burden) decreased in the past decades in SSA and in the Fragile and Conflict-Affected Countries (FCAC) group. In SSA countries, rankings of military expenditures as a percentage of GDP provide a basis for identifying the nations that have chosen to spend a large amount on the military and those that have not. Aside from this observed general pattern, there are substantial variations in both military spending (absolute values) and military burden (defined as a share of military expenditures to GDP) across countries, across sub-regions, and over time. The top 10 of countries with higher average military burden between 1990 and 2020 are Angola, Burundi, Zimbabwe, Sudan, Ethiopia, Botswana, Mauritania, Namibia, Lesotho and Chad. By sub-region, Mauritania has the highest average military burden (3.2% of GDP) in West Africa, followed by Chad in Central Africa. In addition, Angola has the highest average military burden in the Southern Africa sub-region (6.9%), followed by Zimbabwe (3.6%) in Eastern Africa.
Although there is a great deal of variation among the regions, the same general pattern of military expenditures is observed in West Africa, Eastern Africa, Central Africa and Southern Africa (). However, the combined average military expenditure of the G5 Sahel countries, Central African countries or Lake Chad countries remains relatively very low in terms of volumes. In the ‘Regional Economic Outlook’ report on Sub-Saharan Africa (IMF Citation2019), the International Monetary Fund finds that an increase in conflict intensity is, on average, linked to a 9% increase in real military budget expenditure (around 0.6% of GDP) and a decline in real investment spending of around 9%.
The demographic shifting of different world regions illustrates how sub-Saharan Africa’s population is younger than any other in the world. While 26% of the world’s population is aged 0–14 years, the equivalent for Africa was 43%. Similarly, 15–24-year-old make up 16% of the total world population, while sub-Saharan Africa comprise 20%, according to the UN Population Division report in 2017. Therefore, the provision of education and employment opportunities appear to be another challenge in SSA. Over the last three decades, average public spending on education in Africa has increased since 1990.
Table 1. Military outlays (% of GDP) per sub-region in Sub-Saharan Africa.
Overall, SSA spends, on average, up to 5.17% of its gross domestic product each decade on education, which seems equal to OECD members at 5.22% but second to European Union at 5.4% (see ). Furthermore, the average growth rate of school enrollment for the decade 2010–2020 has decreased relative to 2000–2010. Therefore, the weak governance, which is reflected in weak institutional structures and the lack of accountability on the part of government officials in the continent, has undermined most of the government expenditure policies and has weakened transmission channels of government education spending on educational outcomes in SSA countries. Government spending on education is inequitable and is sometimes inefficient. Countries such as Lesotho (12.4% of GDP), Burundi (8.3% of GDP), Botswana (7.9% of GDP), Swaziland (7.8% of GDP) and Comoros (7.6% of GDP) are the top five in terms of allocating public spending on education ().
Table 2. Trends of education spending in percentage of GDP (average values).
Table 3. Top 10 education spenders in sub-Saharan Africa.
Despite the fact that governments continue to prioritize spending toward primary education, certain countries in SSA spend more on secondary or postsecondary education than on primary education. It is evident that the poorest and most disadvantaged are still unlikely to complete a full cycle of primary education. Even when they do, their chances of transitioning into secondary education are much lower than their more advantaged counterparts. This can impede some countries to achieve the Sustainable Development Goal 4 targets by 2030.
highlights the allocation of education expenditure in SSA countries by education level (primary, secondary, tertiary) in 2018. Many countries in SSA have taken very important steps to make sure that all school-age children get universal primary education. According to a UNICEF report, about 15 African countries have abolished payment of school fees in primary schools since the year 2000. Certain countries that cannot provide every child with primary education invest more in tertiary education. By shifting more resources to lower levels of education, governments might stimulate more household spending for higher education, without threatening tertiary education development.
4. Model specification, data description and estimation technique
This section presents the econometric model, data description and variables, and the estimation technique.
4.1. Econometric model
This paper adopts an augmented Mintz and Huang’s model specification. Following Russett (Citation1982), Mintz and Huang (Citation1991) propose a linear relationship between changes in education expenditures (and changes in growth (
, military spending (
, government revenue (
and school enrollment (
. Beyond these variables, population growth rate (
, Gini coefficient (
and governance effectiveness (
are taken into account. These scholars hypothesize a negative nexus between military and education spending. The education spending function is given by the following equation:
(1)
(1) Wooldridge (Citation2013) indicated that endogenous models are highly dependent on the past and its accumulative process. Hence, Dynamic Panels (DyPa) allow including an endogenous structure into the model through instrumental variables (Baltagi Citation2008). The DyPa model resulting from Equation (1) is specified as follows:
(2)
(2) where
and
are respectively education and military expenditures as a share of GDP.
represents a SSA country;
the time series.
is the country-specific fixed effects;
is the error term;
is the coefficient of the lagged dependent variable;
is a coefficient vector.
is a vector of control variables (
,
,
,
,
). EquationEquation (2)
(2)
(2) represents the main tool for understanding both the direct and indirect effects of military outlays. Indeed, a statistically significant and positive
parameter implies the indirect effect of military outlays on education spending. For example, if a crowding-out effect on investment exists a higher military burden will reduce education spending through lower growth rate. In addition, a significant negative
parameter capturing the direct effect means that the higher the military outlays the lower the resources available for enhancing education spending.
is a one-way error component model:
; where
and
independent of each other and among themselves. The specification of the DyPa presents some characteristics such as the autocorrelation due to the lagged term of dependent variable and the individual effects capturing the country heterogeneity. The inclusion of the autoregressive term
is justified because education financing is dynamic; the previous education spending influences its present level (Manuelli and Seshadri Citation2014). According to Baltagi (Citation2008), Ordinary Least Square (OLS) estimator is biased and inconsistent while the fixed effect estimates are biased.
4.2. Data sources and description
We use annual data that cover 44 countriesFootnote7 from 1995 to 2020. With a large number of individuals and a short period of time, we have the most common type of data in Dynamic Panel analysis. Human capital development is the dependent variable. It is often proxied by some variables such as school enrollment (Ouedraogo et al. Citation2021), Internet users per 100 people (Tsaurai Citation2020) and education expenditure (Deger Citation1985; Jurado-Sanchez and Jimenez-Martin Citation2014; Xu et al. Citation2018). Following Looney (Citation1990), we assume that public spending in education is a crucial proxy of human capital formation. From both theoretical framework and previous empirical literature review, seven variables were identified as determining factors of human capital development at an aggregate level in SSA: military outlays is the ratio of military expenditures to GDP; economic growth rate measured by an annual percentage growth rate of GDP at market prices; government revenues is the ratio of total tax revenue to GDP. Tax revenue refers to compulsory transfers to the central government for public purposes; gross school enrollment; government effectiveness which captures perceptions of the quality of public services, the quality of the civil service and the degree of its independence from political pressures; Gini coefficient, which measures household income inequality with a potential range of values from zero to 100 (Galbraith Citation2009; Kentor, Jorgenson, and Kick Citation2012); and population growth rate. In order to diagnose the multicollinearity issues, the pairwise bivariate correlations for all variables included in the model were carried (see Appendix 2). The results of correlation test show that military outlays are negatively correlated to education spending. Moreover, the correlation coefficients between different pairs of variables are significant but very low or not significant, implying the multicollinearity neglect in the model to be estimated. Descriptive statistics are presented in and the definition and sources of variables are in Appendix 3.
Table 4. Descriptive statistics for variables.
From the descriptive statistics table, the standard deviations for edu, grow and grev show that they have changed greatly between 1995 and 2020. The Shapiro and Wilk test (Swilk test) indicates that the variables edu, mex, geff, enrol and gini all have p-values less than .10, indicating that they were not normally distributed, while the variables, grev, grow, and pop all had p-values greater than .10 implying that these variables are normally distributed (Shapiro and Wilk Citation1965). To solve the problem of missing data, some countries that have no observations for the dependent variables were removed.
Before estimating the Dynamic Panel model, for scrutinizing the stochastic properties of the variables, we implement the Hadri Lagrange multiplier (LM) unit root test for panel data. It is carried out with the null hypothesis of stationarity in all units against the alternative of a unit root in all units. According to Hadri (Citation2000), this test takes into account both serial correlation and cross-sectional dependency through the implementation of an AR-based bootstrap, compared to the standard unit root tests (Augmented Dickey and Fuller (ADF), Levin, Lin and Chu (LLC)). The standard unit root tests do not result very powerfully against relevant alternatives and fail to reject the null hypothesis for many economic series.
4.3. Estimation technique: system Generalized Method of Moments
Several studies with varying objectives and types of data have employed distinctive estimators to analyze relationships between military spending and human capital development. These include Two or Three Stage Least Square (Ozsoy Citation2002; Fan, Liu, and Coyte Citation2018), Generalized Method of Moment (Davis and Chan Citation1990; Lin, Ali, and Lu Citation2015; Coutts et al. Citation2019), Seemingly Unrelated Regression (Ali Citation2011), and panel structural VAR (Blomberg, Hess, and Athanasios Citation2004). We need in this study to use an efficient estimation method. In order to estimate this dynamic panel model, the study employs the system Generalized Method of Moments (GMM) estimator proposed by Blundell and Bond (Citation1998), which is considered to be more efficient in the case of micro panel (a large number of cross-sectional units observed (44 countries) over relatively short time periods (26 years)). According to Labra and Torrecillas (Citation2018), when T is small, the difference-GMM estimator could be substantially biased. In the relevant literature, it has been established that Gross Domestic Product and education spending have a bi-causal relationship, which leads to the endogeneity bias. Furthermore, military spending as government expenditures is a potential endogenous variable due to the simultaneity with education expending. A well-suited technique to deal with such an endogeneity issue is the GMM methodology. The system GMM estimator allows us to solve the problem of weak instruments by combining moment conditions for the model in first-differences with those for the model in levels (Greene Citation2003). In addition, this estimator is capable of correcting for unobserved country heterogeneity, errors due to measurement, omitted variable bias and likely endogeneity problems, which often affect finding robustness (Arellano and Bover Citation1995; Blundell and Bond Citation1998).
The lagged dependent variable is correlated with the disturbance term (since is a function of
), even if the
is not serially correlated. Inspired by Kripfganz (Citation2016), we use, in the case of this study, a group of lagged terms of variables as instruments for the corresponding variables. In order to check if the number of instruments is adequate and it does not produce overidentification, we perform the Sargan and Hansen tests (Hansen Citation1982). These tests are carried out and the autocorrelation test is applied to check the existence of second-order autocorrelation. Two steps estimators are more efficient and recommendable (Labra and Torrecillas Citation2018) because it uses the heteroscedastic weight matrix in this type of estimation.
5. Estimation, interpretation and discussion of results
The unit test results show that all the variables with the exception of education, government revenue and gross school enrollment are stationary at levels (Appendix 4). Education, government revenue and school enrollment became stationary after first differencing. In regards to the diagnostic tests, results report the presence of first-order autocorrelation, but no second-order autocorrelation. The Sargan test conducted rejected the null hypothesis of the validity of instruments. In the estimation process, the number of countries exceeds the number of instruments, indicating the absence of a problem of instrument proliferation (Roodman Citation2009). Overall, these findings imply that the models are well-specified. and display the estimation results of military outlays effects on education capital development in SSA and in sub-regions, respectively.
Table 5. Estimated effects of military expenditures on education development in SSA.
Table 6. Estimated effects of military outlays on education spending in sub-regions.
The lagged effect of education spending (measured as a percentage of GDP) on itself is dominant, which amounts to approximately a 98.5% increase in this year’s spending for a percent increase in last year’s public expenditures in education (model I). This also means that education spending stimulates itself and perpetuates itself relatively. In line with the empirical literature, scholars like Imana (Citation2017) similarly found that real GDP and education-lagged expenditure were significant in affecting public education expenditure in Kenya.
The results show that growth has a positive and significant effect on education spending. Thus, an increased economic growth rate leads to an improvement in government expenditures on education in SSA. This result conformed to the a priori expectation. The coefficient value is 0.2155, implying that 1% increase in GDP growth rate resulted in a 21.55% increase in education spending. This suggests that public spending on education responds to prevailing economic conditions in the SSA countries. It supports the findings of Hanushek (Citation2013) that economic growth is a driver of human capital development. Like this finding, a study by Jurado-Sanchez and Jimenez-Martin (Citation2014) concluded that one standard deviation change in growth has a positive impact on education expenditure change. The positive relationship reported in this study agrees with the previous findings of Oseni et al. (Citation2020), who reported similar conclusions for SSA, notwithstanding the different objectives aim by the present study. While growth seems to contribute strongly to education financing in central and southern African sub-regions, the growth rate had a strong and negative impact on the proportion of funds allocated to education in Western and Eastern African sub-regions.
The estimated coefficients of relationship between military outlays and education spending in SSA reveal mixed results in line with the diversity of evidence in the literature on the guns versus butter dilemma. Generally, in SSA countries, results show the non-existence of budgetary trade-offs between defense and education spending. Military outlays have a strong and direct positive impact on the proportion of funds allocated to education development. Indeed, a unit increase in military burden would increase education spending in the percentage of GDP by 9.8% in the current period. Similarly, there is a complementarity between military outlays and education spending in central and southern sub-regions. Confirming the military Keynesianism thesis in SSA, this result is in conformity with the finding of Lin, Ali, and Lu (Citation2015), who showed the complementarity between military and education spending in OECD countries; Moreover, it remains consistent with other studies such as Hess and Mullan (Citation1988). However, education development suffers in some sub-regions (models II–VI) due to high military burdens. In Western, Eastern and Sahel & Lake Chad sub-regions, other things being equal, military outlays appear to depress directly the propensity to allocate funds to education. There exists a negative and significant relationship between military and education spending. These crowding-out effects remain in accordance with certain findings evidenced by Fan, Liu, and Coyte (Citation2018), Luqman and Antonakakis (Citation2021), and Wang (Citation2022).
However, the indirect effect does not coincide with a direct effect of military outlays. Overall, in SSA (model I), there is a positive relationship between growth and education spending, implying that if there is crowding-out effect of military burden on investment, military outlays will decrease education spending through a lower economic growth rate. An increase in defense outlays crowds out investment, which in turn, reduces economic growth, thereby diminishing the capacity of governments to allocate more funds to welfare programs. This indirect link is also evidenced in central and southern Africa. This result concords with the results reported by authors like Mintz and Huang (Citation1991) in the USA case from 1953 to 1987. For other sub-regions, Western and Eastern Africa, if military burden involves a budgetary crowding-out effect on investment, the decrease in growth does not lead to a decrease in education expenditures.
Again, the result indicates a positive and significant relationship between government revenue and education spending. Total government revenues refer to all tax and non-tax revenues collected by governments, including grants, while total government expenditures are all operational and development expenditures incurred by the government in a particular financial period (Nyamongo, Sichei, and Schoeman Citation2007). Imbalances between government expenditures and revenues will translate into a budget deficit or surplus. Therefore, we support that government revenues positively affect education expenditure. In line with the Friedman’s (Citation1978) tax-and-spend hypothesis, the increase in tax revenues lead to an increase in government spending. Results that confirm this hypothesis show that 1% increase in government revenue resulted in 1.6% increase in education spending in SSA. Similar findings about the positive relationship were found in different sub-regions. Like this finding, a study by Manage and Marlow (Citation1986) concluded that revenue causes government spending in the USA. It suggests finally that government revenue has a positive contribution to education development. In addition, results show that in SSA, increasing school enrollment has a negative influence on education expenditure.
Government effectiveness, which reflects the perceptions of citizens on different aspects of the measures taken by the Government (quality of the public services, implementation and conducting of public policies, political pressures and the trustworthiness of the government), is important aspect of the quality of public expenditures. The weak governance, weak institutional structures and the lack of accountability on the part of government officials can undermine government spending policies. As shown in (model I), the government effectiveness effect on education spending in SSA is not significant. Nevertheless, in the countries of Sahel and Chad Lake area (model II), this variable has a significant and positive effect on education development: 1% increase in the perception’s degree of government effectiveness results in 16.34% increase in education spending. A similar finding is found in Southern Africa sub-region (model VI in ). When the government is effective, there is a greater chance that public spending will be better allocated and educational policies will be better formulated and implemented in order to develop human capital. For example, an effective government can finance educational infrastructure and provide facilities by building and maintaining well-equipped infrastructure at all levels of education. In other sub-regions, especially Western Africa (model III) and Eastern Africa (model V), results show a negative and significant relationship between government effectiveness and education spending. Government effectiveness may vary depending on various factors, such as the quality of governance. When security issues are preeminent in the public spending policy, improving government effectiveness will privilege defense spending and therefore decrease education expenditures.
Theoretically, the relative distribution of income within society affects government spending on education. The findings suggest that countries with higher income inequality are likely to spend more on education. A significant and positive relationship between income inequality and education spending means that an increase in the income inequality index leads to a rise in education spending. Similarly, this positive relationship can be verified in some sub-regions, especially in western and central Africa. In line with the conclusion of Gioacchino, Sabani, and Usai (Citation2020), the allocation of education spending depends strongly to the inclusiveness of the education system.
In line with a priori expectation, the population growth rate has a positive and significant effect on education spending in SSA and within sub-regions, except in the Eastern Africa sub-region (model V). In SSA (model I), for example, results show that 1% increase in population growth rate resulted in a 9.7% increase in education spending. This finding suggests that as the total population and even the number of children are growing, the school enrollment increases; therefore, they may need additional resources to be able to cope with the growing number of children who are of school age. The overpopulation increases education costs. As evidenced by Verbina and Chowdhury (Citation2004) in Russia, high population density allowed a lower cost of education provision due to developed infrastructure and economies of scale; or by Grob and Wolter (Citation2005) in Switzerland, the share of the elderly population exhibited that demographic changes give rise to competition for public financial resources between elderly and younger age population. However, in countries where the share of public expenditures allocated to education is stable over time, the total population growth and, therefore, a larger number of school-going children probably reduces their per capita spending on education. Demographic factors seem to have been overlooked in the government’s education allocation decisions.
6. Conclusion
The purpose of this study was to investigate the effects of military outlays on human capital development across countries in sub-Saharan Africa. By using a dynamic panel model analysis from 1995 to 2020, a system Generalized Method of Moments was adopted as the estimation technique in this study. For robustness analysis, we conduct the sensitivity analysis by estimating the military burden effect on education spending by Africa sub-region. Findings showed the mixed results in line with the diversity of evidences on the ‘guns versus butter dilemma’ literature.
In sub-Saharan Africa countries, the results showed the non-existence of budgetary trade-offs between defense and education spending. Military outlays have a significant positive effect on education spending, confirming the complementarity hypothesis (bedfellow) between welfare and military expenditures. Similarly, there is a positive relationship between military outlays and education spending in central and southern sub-regions. However, in Sahel & Lake Chad area, Western and Eastern sub-regions, the military burden appears to depress directly the propensity to allocate funds to education. There exists a negative and significant relationship between military and education spending. Furthermore, military expenditures indirectly contribute to education development through economic growth in SSA and in many sub-regions, especially in Central and Southern Africa. For other sub-regions, western and Eastern Africa, the negative effect operates indirectly through growth, given that increased defense outlays crowd-out investment, which, in turn, reduces economic growth, thereby diminishing the capacity of governments to allocate more funds to educational programs.
In line with these findings, government and policy makers should revise the approach, which consists of considering defense expenditures as sovereignty spending for an approach that considers defense as a component of socioeconomic development. Furthermore, it is urgent in some SSA countries that government authorities and public institutions should engage in a dialogue as to public investment budget execution in order to avoid the crowding-out effect of military burden on social welfare development. The governmental authorities should regulate the perfect ratio of guns and butter that meets their needs which means the ratio of defense goods to civilian goods.
Acknowledgements
The authors would like to thank reviewers who took the time to give comments and suggestions to improve this paper. Their insights have proved invaluable.
Disclosure statement
No potential conflict of interest was reported by the author(s).
Notes
1 There were at least 15 countries with active armed conflicts in sub-Saharan Africa in 2019: Burkina Faso, Burundi, Cameroon, Central African Republic, Chad, Democratic Republic of Congo, Ethiopia, Kenya, Mali, Mozambique, Niger, Nigeria, Somalia, South Sudan and Sudan.
2 Robinson believes that in the long run, the loss due to the arms race is literally incalculable because we cannot know benefits that would have flowed from using these intellectual and material resources for constructive purposes.
3 During the 1930s, the idea gradually arose that in order to reduce unemployment and overcome the economic depression, aggregate demand had to be increased through a public expenditure policy.
4 See Cypher (Citation2015) for the conceptual development of military Keynesianism.
5 Sahel is an extremely poor region that stretches from Senegal to Sudan and Eritrea.
6 For instance, Wolpin (Citation1986) has shown that health, education and social welfare tend to decrease as military influence increases.
7 The list of these countries is displayed in Appendix 1.
References
- Abdul, R., S. Z. Mohd, and A. S. Dewi. 2015. “Effect of Conflict and Military Expenditure on School Performance in South Asia.” International Journal of Humanities and Social Science 5 (12): 99–107.
- Adeola, F. O. 1996. “Military Expenditures, Health and Education: Bedfellows or Antagonists in Third World.” Armed Forces & Society 22 (3): 441–467. doi:10.1177/0095327X9602200306.
- Ali, H. E. 2011. “Military Expenditures and Human Development: Guns and Butter Arguments Revisited: A Case Study from Egypt.” Peace Economics, Peace Science and Public Policy 17 (1): 1–19. https://doi.org/10.2202/1554-8597.1240.
- Ali, M., and O. Dimitraki. 2014. “Military spending and economic growth in China: a regime-switching analysis.” Applied Economics 46 (28): 3408–20.
- Apostolakis, B. E. 1992. “Warfare-Welfare Expenditure Substitution in Latin America, 1953–87.” Journal of Peace Research 29 (1): 85–98. doi:10.1177/0022343392029001007.
- Arellano, M., and O. Bover. 1995. “Another Look at the Instrumental Variable Estimation of Error Components Models.” Journal of Economics 68 (1): 29–51. doi:10.1016/0304-4076(94)01642-D.
- Baltagi, B. 2008. Econometric Analysis of Panel Data. Hoboken, NJ: John Wiley and Sons.
- Barro, R. J., and J. Lee. 2016. “A New Data Set of Educational Attainment in the World, 1950–2010.” NBER Working Paper No.15902.
- Bauwens, M. A. 2012. “Guns Versus Butter: A Budgetary Trade-Off in the MENA Region.” Master Thesis, Lund University.
- Benoit, E. 1978. “Growth and Defense in Developing Countries.” Development and Cultural Change 26: 271–80. https://doi.org/10.1086/451015
- Biscione, A., and R. Caruso. 2021. “Military Expenditures and Income Inequality: Evidence from a Panel of Transition Countries (1990–2015).” Defence Peace Economics 32: 46–67. doi:10.1080/10242694.2019.1661218.
- Blomberg, S. B., G. D. Hess, and O. Athanasios. 2004. “The Macroeconomic Consequences of Terrorism.” Journal of Monetary Economics 51: 1007–1032. doi:10.1016/j.jmoneco.2004.04.001.
- Blundell, R., and S. Bond. 1998. “Initial Conditions and Moment Restrictions in Dynamic Panel Data Models.” Journal of Economics 87 (1): 115–143. doi:10.1016/s0304-4076(98)00009-8.
- Bove, V., G. Efthyvoulou, and A. Navas. 2017. “Political Cycles in Public Expenditure: Butter vs Guns.” Journal of Comparative Economics 45: 582–604. doi:10.1016/j.jce.2016.03.004.
- Bullock, B., and G. Firebaugh. 1990. “Guns and Butter? The Effects of Militarization on Economic Growth and Social Development in the Third World.” Journal of Political and Military Sociology 18: 231–236.
- Caputo, D. A. 1975. “New Perspective on the Public Policy Implications of Defence and Welfare Expenditures in Four Modern Democracies: 1950–1970.” Policy Sciences 6 (4): 423–446. doi:10.1007/BF00142383.
- Churchill, S. A., and S. L. Yew. 2018. “The effect of military expenditure on growth: an empirical synthesis.” Empirical Economics 55 (3): 1357–87. https://doi.org/10.1007/s00181-017-1300-z.
- Clayton, J. L. 1976. “The Fiscal Limits of the Warfare-Welfare State: Defense and Welfare Spending in the United States Since 1900.” Western Political Quarterly 29 (3): 364–383. doi:10.1177/106591297602900304.
- Coutts, A., A. Daoud, A. Fakih, W. Marrouch, and B. Reinsberg. 2019. “Guns and Butter? Military Expenditure and Health Spending on the eve of the Arab Spring.” Defence and Peace Economics 30 (2): 227–237. doi:10.1080/10242694.2018.1497372.
- Cypher, J. M. 2015. “The origins and evolution of military Keynesianism in the United States.” Journal of post-Keynesian Economy 38 (3): 449–76.
- Dabelko, D., and J. McCormick. 1977. “Opportunity Cost of Defence: Some Cross-National Evidence.” Journal of Peace Research 14 (2): 145–154. doi:10.1177/002234337701400204.
- Davis, D. R., and S. Chan. 1990. “The Security-Welfare Relationship: Longitudinal Evidence from Taiwan.” Journal of Peace Research 27 (1): 87–100. doi:10.1177/0022343390027001008.
- Deger, S. 1985. “Human Resources, Government Education Expenditure, and the Military Burden in Less Developed Countries.” Journal of Developing Areas 20 (1): 42–43.
- Domke, W. K., R. C. Eichenberg, and C. M. Kelleher. 1983. “The Illusion of Choice: Defence and Welfare in Advanced Industrial Democracies 1948–1978.” American Political Science Review 77 (1): 19–35. doi:10.2307/1956009.
- Dunne, J. P., and M. Uye. 2009. Military Spending and Development. London: Global Arms Trade.
- Fan, H., W. Liu, and P. C. Coyte. 2018. “Do Military Expenditures Crowd out Health Expenditures? Evidence from Around the World, 2000–2013.” Defence and Peace Economics 29 (7): 766–779. doi:10.1080/10242694.2017.1303303.
- Farayibi, A. O., and O. Folarin. 2021. “Does Government Education Expenditure Affect Educational Outcomes? New Evidence from sub-Saharan African Countries.” African Development Review 33 (3): 546–559. doi:10.1111/1467-8268.12588.
- Flamm, K. 1988. Creating the Computer: Government, Industry and High Technology. Washington, DC: Brookings Institution.
- Frederiksen, P. C., and R. E. Looney. 1994. “Budgetary Consequences of Defense Expenditures in Pakistan: Short-Run Impacts and Long-Run Adjustments.” Journal of Peace Research 31 (1): 11–18. doi:10.1177/0022343394031001002.
- Friedman, M. 1978. “The Limitations of Tax Limitation.” Policy Review 5: 7–14.
- Galbraith, J. 2009. “Inequality, Unemployment and Growth: New Measures for Old Controversies.” The Journal of Economic Inequality 7: 189–206. doi:10.1007/s10888-008-9083-2.
- Gifford, B. 2006. “Why No Trade-off Between Guns and Butter? Armed Forces and Social Spending in the Advanced Industrial Democracies, 1960–1993.” American Journal of Sociology 112 (2): 473–509. doi:10.1086/506416.
- Gioacchino, D. D., L. Sabani, and S. Usai. 2020. “Why Education Expenditures Differ Across Countries? The Role of Income Inequality, Human Capital, and the Inclusiveness of the Education System.” Working Papers – Economics wp2020_15.rdf.
- Gold, D. 1991. “Military R&D a Poor Scapegoat for Flagging Economic.” Bulletin of the Atomic Scientists 47 (1): 38–43. doi:10.1080/00963402.1991.11459935.
- Gold, D. 2005. “Does Military Spending Stimulate or Retard Economic Performance? Revisiting an Old Debate.” International Affairs Working Paper 1, 20p.
- Greene, W. H. 2003. Econometric Analysis. 5th ed. Upper Saddle River, NJ: Prentice Hall.
- Grob, U., and S. C. Wolter. 2005. “Demographic Change and Public Education Spending: A Conflict Between Young and Old?” CESifo Working Paper, No. 1555, Munich. http://ideas.repec.org/p/ces/ceswps/_1555.html.
- Gupta, S., M. Verhoeven, and E. R. Tiongson. 2002. “The Effectiveness of Government Spending on Education and Health Care in Developing and Transition Economies.” European Journal of Political Economy 18 (4): 717–737. doi:10.1016/S0176-2680(02)00116-7.
- Hadri, K. 2000. “Testing for Stationarity in Heterogeneous Panel Data.” Econometrics Journal 3: 148–161. doi:10.1111/1368-423X.00043.
- Hansen, L. P. 1982. “Large Sample Properties of Generalized Method of Moments Estimators.” Econometrica 50 (4): 1029–1054. doi:10.2307/1912775.
- Hanushek, E. A. 2013. “Economic Growth in Developing Countries: The Role of Human Capital.” Economics of Education Review 37: 204–212. doi:10.1016/j.econedurev.2013.04.005.
- Harris, G., and M. K. Pranowo. 1988. “Trade-Offs Between Defence and Education Health Expenditures in Developing Countries.” Journal of Peace Research 25 (2): 165–177. doi:10.1177/002234338802500205.
- Hess, P., and B. Mullan. 1988. “The Military Burden and Public Education Expenditures in Contemporary Developing Nations: Is There a Trade-off?” Journal of Developing Areas 22: 267–283.
- Ikegami, M., and Z. Wang. 2022. “Does Military Expenditure Crowd out Health-Care Spending? Cross-Country Empirics.” Quality Quantity, 1–16. doi:10.1007/s11135-022-01412-x.
- Imana, D. K. 2017. “The Determinants of Public Education Expenditures: An Empirical Analysis of Changing Patterns and Growth of Public Expenditure on Education in Kenya.” Journal of Public Administration and Governance 7 (4): 1–18. http://www.macrothink.org/journal/index.php/jpag/article/view/11972. doi:10.5296/jpag.v7i4.11972.
- IMF. 2019. Regional Economic Outlook: Sub-Saharan Africa Recovery Amid Elevated Uncertainty Background Paper–Online Annexes & Statistical Tables. Washington, DC: International Monetary Fund.
- Jurado-Sanchez, J., and J.-A. Jimenez-Martin. 2014. “Guns, Economic Growth and Education During the Second Half of the Twentieth Century: Was Spain Different?” ICAE WP # 1414, June.
- Kalecki, M. 1943. “Political Aspects of Full Employment.” Political Quarterly XIV (3): 322–331.
- Kentor, J., and A. Jorgenson. 2017. “Military Expenditures and Health: A Cross-National Study, 1975–2000.” International Journal of Sociology and Social Policy 37 (13/14): 755–772. doi:10.1108/IJSSP-01-2017-0004.
- Kentor, J., A. Jorgenson, and E. Kick. 2012. “The new military and income inequality: A cross national analysis.” Social Science Research 41: 514–526. https://doi.org/10.1016/j.ssresearch.2011.12.005.
- Keynes, J. 1939. “Will Rearmament Cure Unemployment?” The Listener, June, Collected Works, 21.
- Kollias, C., and S.-M. Paleologou. 2011. “Budgetary Trade-Offs Between Defence, Education and Social Spending in Greece.” Applied Economics Letters 18 (11): 1071–1075. doi:10.1080/13504851.2010.524606.
- Kripfganz, S. 2016. “Quasi-maximum Likelihood Estimation of Linear Dynamic Short-T Panel-Data Models.” Stata Journal 16 (4): 1013–1038. doi:10.1177/1536867X1601600411.
- Krugman, P. 2011. “Bombs, Bridges and Jobs.” New York Times, October 30.
- Labra, E., and C. Torrecillas. 2018. “Estimating Dynamic Panel Data. A Practical Approach to Perform Long Panels.” Revista Colombiana de Estadística 41 (1): 31–52. doi:10.15446/rce.v41n1.61885.
- Lin, E. S., H. E. Ali, and Y. L. Lu. 2015. “Does Military Spending Crowd out Social Welfare Expenditures? Evidence from a Panel of OECD Countries.” Defence Peace Economics 26: 33–48. doi:10.1080/10242694.2013.848576.
- Looney, R. E. 1990. “Militarization, Military Regimes, and the General Quality of Life in the Third World.” Armed Forces1 Society 17: 127–139. doi:10.1177/0095327X9001700107.
- Luqman, M., and N. Antonakakis. 2021. “Guns Better Than Butter in Pakistan? The Dilemma of Military Expenditure, Human Development, and Economic Growth.” Technological Forecasting & Social Change 173: 121–143. doi:10.1016/j.techfore.2021.121143.
- Manage, N., and M. L. Marlow. 1986. “The Causal Relation Between Federal Expenditures and Receipts.” Southern Economic Journal 52 (3): 617–629. doi:10.2307/1059261.
- Manuelli, R. E., and A. Seshadri. 2014. “Human Capital and the Wealth of Nations.” American Economic Review 104 (9): 2736–2762. doi:10.1257/aer.104.9.2736.
- Melman, S. 1971. Pentagon Capitalism. The Political Economy of War. New York: McGraw-Hill.
- Mintz, A., and C. Huang. 1991. “Guns Versus Butter: The Indirect Link.” American Journal of Political Science 35 (3): 738–757. doi:10.2307/2111564.
- Nyamongo, M. E., M. M. Sichei, and N. J. Schoeman. 2007. “Government Revenue and Expenditure Nexus in South Africa.” South African Journal of Economic and Management Sciences 10 (2): 256–268. doi:10.4102/sajems.v10i2.586
- Oseni, I. O., S. O. Akinbode, D. A. Babalola, and S. B. Adegboyega. 2020. “Government Spending and School Enrolment in sub-Saharan Africa: A System GMM Approach.” Journal of Economics & Management 40 (2): 91–108. https://doi.org/10.22367/jem.2020.40.05.
- Ouedraogo, I., H. Ngoa Tabi, H. Atangana Ondoa, and A. N. Jiya. 2021. “Institutional Quality and Human Capital Development in Africa.” Economic Systems 46 (1). doi:10.1016/j.ecosys.2021.100937.
- Ozsoy, O. 2002. “Budgetary Trade-Offs Between Defense, Education and Health Expenditures: The Case of Turkey.” Defence and Peace Economics 13 (2): 129–136. doi:10.1080/10242690210968.
- Peroff, K. K. 1976. “The Warfare-Welfare Tradeoff: Health, Public Aid, and Housing.” Journal of Sociology and Social Welfare 4: 366–381.
- Peroff, K. K., and M. Podolak-Warren. 1979. “Does Spending on Defence Cut Spending on Health? A Time-Series Analysis of the U.S. Economy 1929–74.” British Journal of Political Science 9 (1): 21–40. doi:10.1017/S0007123400001605.
- Robinson, J. 1971. Economic Heresies. London: Macmillan.
- Robinson, J., and J. Eatwell. 1973. Introduction to Modern Economics. Paris: McGraw-Hill.
- Roodman, D. 2009. “How to do Xtabond2: An Introduction to Difference and System GMM in Stata.” Stata Journal 9 (1): 86–136. doi:10.1177/1536867X0900900106.
- Russett, B. M. 1969. “Who Pays for Defence?” American Political Science Review 63 (2): 412–426. doi:10.2307/1954697.
- Russett, B. M. 1982. “Defence Expenditures and National Well-Being.” American Political Science Review 76 (4): 767–777. doi:10.2307/1962969.
- Sagikyzy, A., M. Shurshitbay, and Z. Akhmedova. 2021. “Upbringing and Education as Factors of Human Capital Development.” Adam alemi 2 (88): 18–25. doi:10.48010/2021.2/1999-5849.03.
- Sandler, T., and K. Hartley. 1995. Defence Economics. Oxford: Oxford Uniersity Press.
- Schméder, G. 2013. “Le casse-tête économique des dépenses militaires: Le cas des États-Unis.” Innovations 3 (42): 17–38. doi:10.3917/inno.042.0017.
- Shapiro, S. S., and M. B. Wilk. 1965. “An Analysis of Variance Test for Normality.” Biometrika 52: 591–611. doi:10.1093/biomet/52.3-4.591.
- Stiglitz, J. E., and R. W. Boadway. 1994. Economics and the Canadian Economy. New York: W.W. Norton.
- Swanson, D. 2012. The Military Industrial Complex at 50, ebook.
- Tsaurai, K. 2020. “Determinants of Human Capital Development in Africa.” International Journal of Economics and Business Administration VIII (4): 377–391. doi:10.35808/ijeba/594.
- Verbina, I., and A. Chowdhury. 2004. “What Determines Public Education Expenditures in Russia?” The European Bank for Reconstruction and Development 12 (3): 489–508. DOI: 10.1111/j.0967-0750.2004.00190.x.
- Wang, Y. 2022. “Revisiting the Guns-Butter Tradeoff: A Wavelet Analysis of the US and Britain.” Policy Studies 00 (0): 1–25. doi:10.1080/01442872.2022.2076822.
- WDI 2020. World Development Indicators retrieved at https://databank.worldbank.org/source/world-development-indicators.
- Wolpin, M. 1986. Militarization, Internal Repression and Social Welfare in the Third World. London: Croom Helm.
- Wooldridge, J. 2013. Introductory Econometrics: A Modern Approach. 5 ed., South-Western, Australia.
- Xu, Y., H. L. Chang, C. W. Su, and A. Dumitrescu. 2018. “Guns for Butter? Empirical Evidence from China.” Defence and Peace Economics 29 (7): 809–820. doi:10.1080/10242694.2017.1293791.
- Yildirim, J., and S. Sezgin. 2002. “Defence, Education and Health Expenditures in Turkey, 1924–96.” Journal of Peace Research 39 (5): 569–580. doi:10.1177/0022343302039005004.
- Zhang, Y., X. Liu, J. Xu, and R. Wang. 2016. “Does Military Spending Promote Social Welfare? A Comparative Analysis of the BRICS and G7 Countries.” Defence and Peace Economics 28 (6): 686–702. doi:10.1080/10242694.2016.1144899.
Appendices
Appendix 1. List of countries in the sample
Sub-Saharan Africa: Angola, Benin, Botswana, Burkina Faso, Burundi, Cabo Verde, Cameroon, Central African Republic, Chad, Congo Democratic Republic, Congo, Equatorial Guinea, Ethiopia, Gabon, Gambia, Ghana, Guinea, Guinea Bissau, Ivory Coast, Kenya, Lesotho, Liberia, Madagascar, Malawi, Mali, Mauritania, Mauritius, Mozambique, Namibia, Niger, Nigeria, Rwanda, Senegal, Seychelles, Sierra Leone, Somalia, South Africa, Sudan, Swaziland, Tanzania, Togo, Uganda, Zambia, Zimbabwe.
Sahel and Lake Chad: Burkina Faso, Cameroon, Chad, Mali, Mauritania, Nigeria, Niger.
Central Africa: Angola, Burundi, Cameroon, Chad, Central African Republic, Congo, Congo Democratic Republic, Equatorial Guinea, Gabon.
West Africa: Benin, Burkina Faso, Cabo Verde, Gambia, Ghana, Guinea, Ivory Cost, Liberia, Mali, Nigeria, Niger, Sierra Leone, Senegal, Togo.
East Africa: Ethiopia, Kenya, Madagascar, Mauritius, Rwanda, Sierra Leone, Somalia, Sudan, Tanzania, Uganda.
South Africa: Botswana, Lesotho, Malawi, Mozambique, Namibia, Seychelles, South Africa, Swaziland, Zambia, Zimbabwe.