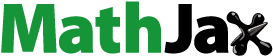
ABSTRACT
Worldwide, it is widely recognized that violent conflicts constrain economic growth and development. Thus, it is necessary to research and analyse the main causes of violence and their relationship with development. This paper analyses how violence has affected economic development in the Mexican context during the 2000–2015 period. It takes into account that in the last decade, this country has had increased levels of violence, especially since the ‘war on drugs’ against drug trafficking was promulgated in 2006. This study uses different econometric models that apply the assessments of the panel unit root and cointegration tests and panel cointegration estimation. The results indicate that the tests used to validate the data allow the panel data cointegration relationships to be calculated. They demonstrate the existence of a long-run relationship between violence and economic development. Specifically, the results take into account that gross domestic product per capita and public investments have inverse relationships with the homicide rate, whereas poverty, unemployment, illiteracy and the Gini index have direct relationships with the homicide rate. Moreover, violence is strongly related to the deterrence variables. The findings of this study demonstrate that drug trafficking explains the increased violence in Mexico. Thus, it is important to develop and formulate adequate policies to control this situation, take measures to control violence starting with its root causes and promote social and economic development with a strategy to strengthen legal activities in society.
1. Introduction
Violence is considered one of the main risks to economic growth and development, especially in emerging and developing countries. It generates higher insecurity, discourages investments and the creation of entrepreneurship and new businesses, deteriorates institutional structures, and increases the costs of doing business (Herrera and Martinez Citation2022; World Bank Citation2015; Gagne Citation2015). This reality implies the importance of analysing the relationship between violence and economic growth (World Bank Citation2015; Gagne Citation2015).
Countries’ recurrent violence in the short and long terms undermines economic growth and development. It is difficult to measure the economic costs of the effects of conflict on economic activity, infrastructure, employment, internal and foreign investments, new businesses and entrepreneurship, higher levels of poverty and inequality and decreases in health and the welfare of society. Moreover, violence is generated especially in fragile nations, which feeds a negative cycle composed of conflict, economic instability, poverty, and injustice. This reality makes it important to design, formulate and apply adequate policies to prevent violence and conflict and to minimize their effects on economic and human development (Muñiz, Fuerte, and Méndez Citation2022; Muller and Tobias Citation2016).
Based on the World Economic Forum, Schippa (Citation2016) estimated that for 2015, the global costs of violent conflicts were $13.6 trillion in terms of purchasing power parity (PPP), which equates to 13.3% of global gross domestic product (GDP). Additionally, the average level of global peacefulness deteriorated by 0.3% in the 2022 Global Peace Index (GPI), mainly in terms of the political terror scale, neighboring country relations, the intensity of internal conflict, the number of refugees and political instability. Although small, this deterioration continues a long-standing trend, with the GPI deteriorating in eleven of the past 14 years (Institute for Economics & Peace Citation2022). These facts suggest that many of the world's regions are spending too much on conflict and war and too little on peace, thus making it important to change the trend and dynamic (Schippa Citation2016).
The economic impacts of violence are related to the duration and intensity of violence. The costs of violence can be divided in the following manner (Schippa Citation2017; Muller and Tobias Citation2016): (i.) The direct costs are accounting losses that appear in the present year and are related to public expenditures on the military, the judicial system, healthcare and the police. (ii.) The indirect costs are economic losses that are incurred as a consequence of conflict being prolonged within the present year, such as decreased productivity, lost economic output due to murder, pain and trauma resulting from violence and the reduction in economic growth when conflict is prolonged.
Collier (Citation1999) developed a study on conflict and the economic effects that have had relevant impacts in recent decades. The results indicated that economic growth and development are disturbed by the following: (i.) the deterioration of resources, (ii.) damage to infrastructure, (iii.) changes in the social order, (iv.) budgetary changes, (v.) dissaving and (vi.) the decrease in foreign investment. The first five of these effects influence economic development only during violent episodes, whereas the last continues to affect economic development after the restoration of peace. These elements are important because they allow for the analysis of specific contexts since a conflict depending on time can generate higher or lower impacts on economic growth (Collier Citation1999 and Brück and De Groot Citation2013). In other words, long conflicts are generally followed by an increase in economic growth, whereas short conflicts can reduce economic growth rates over a longer time, which is known as a legacy effect (Collier Citation1999 and Brück and De Groot Citation2013).
The objective of this study is to empirically analyse and evaluate the relationship between violence and economic and social development in Mexico, taking into account the increase in drug trafficking. Therefore, the research question that guides this study is the following: How can violence derived from drug trafficking undermine economic and social development, and what are the respective connections?
This study contributes to the economic and development literature by presenting empirical evidence on the relationship between violence and crime. It does so by using different approaches, including economic, social and drug control variables, and by showing how these interrelations can affect economic performance and development in an emerging economy such as Mexico.
Mexico is selected in this study because in recent decades, this country has experienced an increase in violence and crime, especially after 2007 when its homicide rate and the absolute number of homicides grew more than those of any other Western country. This growth has been attributed to drug trafficking and organized crime. In the last five years, there was an average of 23,293 homicides per year, which is more than 55 people per day or just over two people every hour (Calderon, Rodriguez, and Shirk Citation2018). These data demonstrate the importance of analysing how violence can affect economic performance in Mexico.
This paper proceeds as follows. The first section is the introduction, which describes the main study and the objectives of this research. The second section presents the literature review of this study. The third section presents the specifications of our model, the empirical strategy and the data description. The fourth section explains the main results and provides a discussion. Finally, the paper concludes by discussing the relevance of the results and exploring future avenues of research.
2. Literature review
The economic impact of violence on the global economy in 2021 was $16.5 trillion in terms of PPP, which is equivalent to 10% of the world’s economic activity (gross world product) or $2,117 per person. Moreover, violence has an important effect on the world’s economic performance. For example, for the ten countries most affected by violence, the average economic impact of violence was equivalent to 34% of GDP, compared to 3.6% in the countries least affected by violence (Institute for Economics & Peace Citation2022).
Different studies have demonstrated several relationships between violence and economic aspects. For example, Camacho and Rodriguez (Citation2013) studied the causal effects of violence on firms’ exit using a fixed-effect estimation method that determined that violence especially affects younger and smaller enterprises that are important for increasing equality and development in emerging economies. Shemyakina (Citation2011) evaluated the impacts of violence on educational outcomes using an empirical approach and found that violence is significantly and negatively related to schooling results, especially for girls. Caudillo and Torche (Citation2014) investigated the effects of violence on failing a grade in elementary school by applying different causal inference techniques. Their results suggested that violence could affect early educational achievements, which in turn could affect human capital formation and economic welfare. Amodio and Di Maio (Citation2014) evaluated the relationship between violence and firm production using an econometric model. Their findings suggested that violence damages the most productive sectors of the economy and generates negative effects on growth and the population. Cook (Citation2014) determined the relationship between violence and economic activity by assessing the production of patents through a historical exercise. The findings showed that the production of patents is higher when violence declines and that conflict may have effects on the development of quality inventions and economic growth over time. Driffield, Jones, and Crotty (Citation2013) evaluated the determinants of a business strategy to invest in violent zones through a standard model of international business and a probit model. They found that countries with low institutionality and conflict problems are less attractive places for foreign investment. Diallo (Citation2018) examined the connection between internal armed conflicts, criminality, terrorism, political violence and economic growth, proposing a fully-micro-
founded endogenous economic growth model. The findings showed that an increase in internal violence has a strong negative impact on economic growth and that a reduction in internal violence promotes saving, investment and growth. The United Nations Development Programme (UNDP) (Citation2021) determined that violence is a causal factor propelling the high-inequality, low-growth trap and that the persistence of violence generates a vicious circle promoting inequality and slow growth. This reality requires the adoption of policies that promote a peaceful solution to conflicts considering that progress in combating violence may pave the way towards more equal, more productive and more peaceful societies. De Groot et al. (Citation2022) estimated the average consequence of various dimensions of conflicts on yearly GDP growth using different quantitative techniques. They found that conflict-induced global GDP loss gives valuable insights into the structure of the economic burden of violent conflict and the need for the international community to make additional efforts regarding conflict resolution and peacekeeping. These studies show the importance of continuing to analyse the effects of violence on economic growth and development based on different approaches, especially for countries or regions that have suffered from increased conflict and violence in recent decades.
In the Mexican context, different studies have investigated the connection between violence and economic growth and development in the following ways. (i.) The first is the relationship between the labor market and violence. Some studies, such as Coronado and Saucedo (Citation2018), have evaluated the effects of violence generated by drug trafficking on employment using an econometric model and system-generalized method of moments, finding that violence due to drug trafficking has a negative influence on employment. BenYishay and Pearlman (Citation2013) analysed the connection between trends in violence measured by homicides and working hours by applying a fixed-effect model and instrumental variable regressions. They found that the intensification of violence through homicides has negatively impacted labor market activities. Liu, Fullerton, and Ashby (Citation2013) studied the impact of the labor market and deterrence variables on different Mexican crime rates through a panel data model. They found that rising average wages, federal police forces, and incarceration rates reduced violent crime rates in Mexican states. (ii.) The second is the relationship between geography and crime. Some studies, such as Gonzalez (Citation2021) on conservation and violence associated with the Mexican drug war, and Basu and Pearlman (Citation2017) have determined the impacts of violence on migration at the municipal and state levels using an empirical analysis, finding little support for the notion that homicides related to the war on drugs increased domestic migration. Buchelia, Fontenla, and James (Citation2021) estimated the direct effects of return migration in the context of Mexico, a traditionally migrant country that has suffered record levels of violence in the past decade. Rios (Citation2014) generated an estimated migration pattern as a consequence of drug-related violence by applying a linear regression model. The results indicated that migration outflows are higher in places with higher drug-related violence and crime. (iii.) The third is the effects of violence on the economy. Various authors have indicated that drug trafficking has increased violence and deteriorated some economic indicators in this country. For example, Rios (Citation2017) analysed the causal effects of violence on the diversity of local economies through a panel fixed-effect model. The results showed that higher violence reduced economic diversification, increased industrial concentration and decreased economic complexity. Robles, Calderón, and Magaloni (Citation2015) determined the economic costs of drug-related violence by applying two empirical strategies. They found that violence has a negative effect on the economy and development. Enamorado, López-Calva, and Rodriguez-Castelan (Citation2013) assessed the impacts of violence on development patterns and growth through a regional convergence analysis, finding that violence related to drug trafficking resulted in negative growth. Zepeda and Perez (Citation2022) found that an increase in the number of years of schooling in Mexico is correlated with a decline in homicide rates and that sustained development policies can be a pacifying mechanism in the country.
These studies in both the international and Mexican contexts show that there is still scarce quantitative and empirical research supporting the relationship between violence and economic development and its social dimensions.
In Mexico, the longer-term trends indicate a marked deterioration in peacefulness in the last decade due to high levels of corruption, poor governance and drug trafficking. The economic impact of violence in Mexico is estimated to be 4.9 trillion pesos (US$243 billion) in constant 2021 terms, which is equivalent to 20.8% of Mexico’s GDP. On a per capita basis, the economic impact of violence was 38,196 pesos (US$1,884), which is approximately 2.5 times the average monthly salary (Institute for Economics & Peace Citation2022). These facts show the importance of analysing the relationship between violence and economic development in Mexico.
3. Model specification and the empirical strategy
This section presents the econometrics models, data and variables used in this research with the aim of understanding how violence affects economic development. Mexico during the 2000–2015 period is used as a case study.
3.1 Econometric models and estimation methods
The panel cointegration model estimations involved the following steps: the panel unit root and cointegration tests and the panel cointegration estimation. These steps guarantee the reliability, consistency and robustness of the results.
3.1.1 Panel unit root tests
We need to check that all variables are integrated to the same order. To do so, we use panel unit root tests following Levin, Lin, and Chu (Citation2002), Im, Pesaran, and Shin (Citation2003), Breitung (Citation2000), Maddala and Wu (Citation1999) and Choi (Citation2001).
Levin, Lin, and Chu (Citation2002)
Levin, Lin and Chu consider the following basic augmented Dickey – Fuller (ADF) specification:
The method described in Levin, Lin, and Chu (Citation2002) derives the estimates of α from the proxies for
and
, which are standardized and free of autocorrelations and deterministic components. The test shows that under the null hypothesis, a modified t statistic for the resulting
is asymptotically normally distributed.
where
is the standard t statistic for
,
is the estimated variance of the error term η,
is the standard error of
, and
Im, Pesaran, and Shin (Citation2003)
Im, Pesaran, and Shin (Citation2003) start by postulating a separate ADF regression for each cross-section:
Once they estimate the separate ADF regressions, the average of the t statistics for
from the individual ADF regressions
is as follows:
The Im, Pesaran, and Shin (Citation2003) test statistic requires the specification of the number of lags and the specification of the deterministic component for each cross-sectional ADF equation. The test may choose to include individual constants or to include individual constant and trend terms.
Breitung (Citation2000)
The Breitung test accepts that there is a common unit root process such that ρi is identical across cross-sections. Breitung considers the following basic ADF specification:
The ADF model for panel data usually includes two assumptions. The autoregressive portion (and not the exogenous components) is removed when constructing the standardized proxies. The test specifies that the proxies are transformed and detrended.
The persistence parameter α is estimated from the pooled proxy equation:
Breitung demonstrates that under the null hypothesis, the created estimator is asymptotically distributed as a standard normal distribution.
Maddala and Wu (Citation1999)
They suggest the use of a test that is constructed by combining the ρ-values of the test statistic for a unit root in each cross-sectional unit. The Maddala and Wu statistic is specified as follows:
The
test is distributed as a chi square with 2N degrees of freedom under the hypothesis of cross-sectional independence.
is the ρ-value of the unit root test.
Choi (Citation2001)
Choi (Citation2001) suggests the following standardized statistic:
3.1.2 Panel cointegration tests
Pedroni (Citation1999, Citation2004) proposes two types of cointegration tests. First, the panel tests are based on the within-dimension approach, which includes four statistics: the panel ν, panel ρ, panel Phillips – Perron (PP), and panel ADF statistics. The within tests (panel cointegration statistics) are as follows.
Panel ν statistic: This is a non-parametric test with a variance ratio type.
Panel ρ statistic: This is a non-parametric test with a PP ρ statistic type.
Panel t statistic: This is a non-parametric test with a PP t statistic type.
Panel t statistic: This is a parametric test with an ADF t statistic type.
These statistics basically pool the autoregressive coefficients across different Mexican states for the unit root tests on the estimated residuals. These statistics take into account the common time factors and heterogeneity across states. Additionally, the group tests are based on the between-dimension approach, which includes three statistics: the group ρ, group PP, and group ADF statistics. The between tests (group mean panel cointegration statistics) are as follows.
Group ρ statistic: This is a non-parametric test with a PP ρ statistic type.
Group t statistic: This is a non-parametric test with a PP t statistic type.
Group t statistic: This is a parametric test with an ADF t statistic type.
3.1.3 Panel cointegration estimation
Although the cointegration methodology proposed by Pedroni allows us to test the presence of long-run relationships, it is unable to provide an estimation under an error correction model framework. To estimate a long-run relationship among variables under a panel setting in the presence of cointegration, several estimators are proposed: dynamic ordinary least squares (DOLS), the pool mean group (PMG), ordinary least squares (OLS), and fully modified OLS (FMOLS). In this investigation, we use the DOLS estimator. Kao and Chiang (Citation2000) demonstrated that both OLS and FMOLS have a small sample bias and that the DOLS estimator appears to outperform both estimators. Pedroni (Citation2001) has also created a between-dimension, group-means panel DOLS estimator that includes parametric adjustments for endogeneity and serial correlation.
DOLS estimation (Kao and Chiang Citation2000)
The DOLS estimator of Kao and Chiang (Citation2000) is an extension of the estimator of Stock and Watson (Citation1993). To obtain an unbiased estimator of the long-run parameters, the DOLS estimator extends the static regression with the leads, lags, and contemporaneous values of the first-difference regressors. We use a fixed-effect panel model:
Kao and Chiang (Citation2000) assume that {
} is 1 × 1,
is a k x 1 vector of the slope parameters, {
} are the intercepts, and
is an error term. We assume that {
} is a k x 1 vector and an autoregressive process of the first-order difference:
The DOLS estimator is shown in the following expression:
Another important point is to guarantee the reliability of the results and to guarantee that the relationship between development and violence is not due to the period itself. For these reasons, this study differentiates the effects of the relationship between development and violence by taking two reference periods in a regression by sections to determine that the impacts are not due solely and exclusively to the reference period analysed. For the first period I, the variables are the homicide rate and GDP per capita during the 1991–1999 period. For the second period II, the same variables are considered during the 2000–2015 period.
First Period I:
Second Period II:
where Y = Homicide Rate
X = GDP per capita
,
= shocks in both regressions, piecewise regression
Full period
First period I
With this analysis, it can be concluded that for each period, the regressions are different for each intertemporal space using Chow's test to check the above estimates.
The Chow test indicated that the value of 36.5 is significant at 5% level. It can be concluded that the two regressions are different for each period, particularly for the – 2000–2015 period. For this reason, the study period is little affected by the variables of development and violence.
3.2 Data
The dataset consists of a panel of observations for the 31 Mexican states and the Federal District over the 2000–2015 period. The homicide rate of each state is the dependent variable. The data come from the National Institute of Statistics and Geography (INEGI), the National Council for the Evaluation of Social Development Policy (CONEVAL), the Mexican Ministry of Public Education (SEP), the Office of the Mexican Attorney-General (PGR), the Uniform Statistical System for Crime Analysis (SEUNAD), and the Secretariat of National Defense (SEDENA).
The explanatory variables are calculated from the information provided by the Mexican institutions that have the statistics, such as the level of economic development, poverty, inequality, the labor market, education and drugs. These indicators are used to answer the research question: How can violence derived from drug trafficking undermine economic and social development, and what are the respective connections? This question guides this study. To assess the development level of each state, the model uses the aggregate-level production per capita (GDPpc by state) and public investment in economic development from the INEGI. It also uses poverty (asset poverty) and inequality (Gini index) data based on the CONEVAL. The model uses education (illiteracy by state) data from the SEP. Additionally, it uses drug (psychotropic drug seizures and poppy gum seizures) data from the INEGI. Furthermore, it uses crime and captures based on the databases of the INEGI, SEUNAD and SEDENA. Finally, unemployment is the variable selected to define the labor market in the states, with data coming from the database of the INEGI.
shows the summary statistics of every variable used in this study. Cotte (Citation2011a, Citation2011b, Citation2012) demonstrated in the Colombian context the strong connection between poverty, inequality and labor market features and trends in violence. This supports the selection of these variables in this study to determine the different effects between violence and economic development.
Table 1. Summary statistics of the variables used in this study.
4. Results and discussion
In this section, the main results of the study are shown based on the requirements of the empirical strategy following the different stages described in the last section. The aim is to obtain robust results that allow us to analyse the relationship between violence and economic development in the Mexican context.
4.1 Panel unit root results
Since our database is composed of annual data from 2000 to 2015, we run panel data unit root tests on all variables. shows that among the five unit root tests mentioned (individual effects and linear trends), at least one exists that provides evidence of the non-stationarity of each variable. This allows us to continue with the next stage of the empirical strategy.
Table 2. Panel unit root test (individual effects and linear trends).
4.2 Panel cointegration results
The results of the cointegration analysis between violence and economic development are reported in . The four within-group tests were used. The panel tests were conducted using the within-dimension approach, including four statistics: the panel ν, panel ρ, panel PP, and panel ADF statistics. Three between-group tests were conducted to check whether the panel data are cointegrated. The group tests were based on the between-dimension approach, including three statistics: the group ρ, group PP, and group ADF statistics.
Table 3. Panel data cointegration tests.
shows that between the panel cointegration test and the group mean cointegration test of Pedroni (Citation1999), there is at least one that rejects the null hypothesis of no cointegration in all six models. This allows us to estimate the cointegration relationships of the panel data.
4.3 Panel estimation and empirical results
The strategy used to estimate a long-run relationship between violence and economic development is based on one estimator with error correction: DOLS. The DOLS model that is used is as follows:
presents the results from the DOLS estimates of the cointegration relationships. The estimated coefficients for several of the variables were statistically significant for all states of Mexico. The DOLS results confirm the existence of a long-run relationship between violence and economic development.
Table 4. Panel DOLS long-run estimates.
The estimations thus show that the development level of Mexican states negatively affects regional violence (i.e. a higher development level translates into less violence in each Mexican state) based on the DOLS model. The estimations of the economic variables assessed using GDP per capita and public investments in economic development are comparable. In the first case, the results indicate that a higher GDP per capita in a Mexican state leads to a lower homicide rate. These estimations coincide with Hart (Citation2015), Centrepiece (Citation2015), Cotte (Citation2011b) and Elgar and Aitken (Citation2010), who established that countries with a better GDP per capita have low homicide rates. The results of public investment in economic development show a negative relationship such that an increase in public investment in economic development generates a decrease in violence in a state.
Examining the estimations for social conditions, measured in terms of asset poverty and the Gini index, we find that these variables have a direct relationship with homicide rates in the states of Mexico. Therefore, high poverty levels and inequality in states are likely to cause higher rates of crime and violence. These estimations coincide with the results of Pridemore (Citation2011) and Roberts and Willits (Citation2014) in the context of the United States and Nadanovsky and Cunha-Cruz (Citation2009) in the context of developed and developing countries. The results for the labor market reveal a positive coefficient for the unemployment rate. That is, lower unemployment and higher employment generate lower incentives to participate in criminal activity. This result coincides with Hojman (Citation2004), Robinson et al. (Citation2009) and Cotte (Citation2011a).
The estimations of this analysis indicate that factors such as inequality, poverty, crime and unemployment influence violence in Mexican states and negatively impact economic and social development in Mexican states. This finding coincides with the results of several studies, such as Cotte (Citation2007), Darby, Li, and Muscatelli (Citation2004), and Ades and Chua (Citation1997). All of these social indicators are therefore essential in the formulation of strategies leading to growth and development, although the development of each state must also be taken into account. It is also necessary to consider key policies to increase economic growth and development with the objective of decreasing violence in the states of Mexico.
Violence is examined based on the homicide rate, and the estimations indicate that the deterrence variables and captures have negative effects on violence. These results show that violence is closely associated with the deterrence variables. Captures are determined by state presence, and violence is likely to be associated with critical variables such as a weak state presence and a lack of effective justice, which coincides with the Mexican case.
The results for education indicate that states with a more educated population have lower homicide rates. Illiteracy and low education among the poor population may generate unemployment, which in turn may increase the potential for crime and violence (Cole and Marroquin Citation2009). Moreover, the results concur with the country-level findings of Fajnzylber, Lederman, and Loayza (Citation2002) and Souza and Carvalho (Citation2006) for a Brazilian city. They indicate the importance of strengthening education and related policies to guarantee a decrease in violence, especially in developing countries, to offer more labor opportunities and adequate salaries as a strategy for promoting a peaceful society (Fajnzylber, Lederman, and Loayza Citation2002; Souza and Carvalho Citation2006).
The presence of drug trafficking explained the increase in violence in Mexico, especially because drug cartels have used their profits from this illegal business to violently continue their domination over the market despite the government's war on drugs (López Citation2016; Dell Citation2014; UNODOC Citation2011). Specifically, two associated factors have been described as contributing to this increase. First, the expansion of drug trafficking weakened law enforcement institutions and facilitated the diffusion of criminal know-how, thereby strengthening the initial effect of violence and increasing the level of violence in the 2000s. Second, the rivalry over the operation of drug markets and the essentially criminal nature of the drug business produced a rise in homicides. Illegal drugs, measured as poppy gum seizures and psychotropic drug seizures, have a negative and significant influence on violence. In the Mexican case, narcotics traffickers have originated a new scale of ideals for Mexican society. This practice is verified by the consumerism and the damage to institutional legitimacy shown in the crisis of state authority implemented by this country in the mid-2000s. This crisis gradually devastated all chances of institutional arbitration because drug traffickers have no institutional loyalties of any kind and validate that anyone can reach power through the use of violence, mainly due to the lack of management between municipal, state and federal policy agencies and state weakness, which generates circumstances in which violence is provoked and increased (Zepeda Citation2018; Benítez Citation2015; Durante and Gutierrez Citation2013).
5. Conclusions
This paper analysed the relationship between violence and economic development in the Mexican context during the 2000–2015 period. The results indicate that an empirical strategy is adequate to analyse the relationship that was proposed, and the estimations confirm the existence of a long-run relationship between violence and economic development. The development level of Mexican states negatively affects the situation of violence. This result suggests the importance of designing and applying new political instruments that make it possible to promote equitable development in Mexican regions based on their contexts and requirements.
Economic variables (GDP per capita and public investment) have inverse relationships with violence, thus indicating that a higher GDP per capita and public investment are associated with low homicide rates. In the case of social variables (asset poverty, Gini index and illiteracy), the study reveals a direct relationship with homicide rates, thus demonstrating that states with high poverty levels, low education and inequality have high levels of violence and crime.
The unemployment rate has a positive coefficient, thus suggesting that higher employment discourages participation in criminal and illegal activities. The institutions related to the deterrence variables and captures have inverse relationships with violence. This result indicates the importance of strengthening state presence and effective justice and decreasing corruption to decrease crime and violence. In this respect, Mexico should formulate new strategies to guarantee security and legality around the country.
The findings of this study are important for analysing and evaluating the importance of controlling violence and defining adequate policies to promote peace. This is especially true in countries with drug trafficking problems. In such countries, one of the most important challenges is to ensure order, provide security and guarantee equal opportunities for society, as doing so will allow future increases in economic development, productivity and the welfare of the population. It is key to continue conducting empirical studies that reveal other factors that can strengthen violence or decrease violence. These factors can be used as important inputs for policy makers and decision makers in the formulation of policies and programs to control drug trafficking, to progressively eliminate the root causes of violence through social and economic activities and to motivate legal activities and a peaceful society.
Disclosure statement
No potential conflict of interest was reported by the author(s).
References
- Ades, A., and H. Chua. 1997. “Thy Neighbor’s Curse: Regional Instability and Economic Growth.” Journal of Economic Growth 2: 279–304. doi:10.1023/A:1009782809329
- Amodio, F., and M. Di Maio. 2014. Making do with what you have: Conflict, arm performance and input misallocation in Palestine. Centro Studi Luca d'Agliano Development Studies HiCN Working Paper, (379). http://www.hicn.org/wordpress/wp-content/uploads/2012/06/HiCN-WP-179.pdf.
- Basu, S., and S. Pearlman. 2017. “Violence and Migration: Evidence from Mexico’s Drug War.” IZA Journal of Development and Migration, 7–18. doi:10.1186/s40176-017-0102-6.
- Benítez, R. 2015. “México 2015: El Leviatán contra los señores feudales: Retos a la seguridad.” In La multidimensionalidad de la seguridad nacional: Retos y desafíos de la región para su implementación, edited by Sonia Alda Mejías, and Susana de Sousa Ferreira, 201–230. Madrid: Instituto Uni- versitario General Gutiérrez Mellado.
- BenYishay, A., and S. Pearlman. 2013. Homicide and work: the impact of Mexico’s drug war on labor market participation. Working paper LACEA 2013. Vassar College. doi:10.2139/ssrn.2302437.
- Breitung, J. 2000. “The Local Power of Some Unit Root Tests for Panel Data.” In Nonstationary Panels, Panel Cointegration, and Dynamic Panels, Advances in Econometrics, 15, edited by B. Baltagi, 161–178. Amsterdam: JAI.
- Brück, T., and O. De Groot. 2013. “The Economic Impact of Violent Conflict.” Defence and Peace Economics 24 (6): 497–501. doi:10.1080/10242694.2012.723153.
- Buchelia, J., M. Fontenla, and B. James. 2021. “Return Migration and Violence.” World Development 116: 113–124. doi:10.1016/j.worlddev.2018.12.010
- Calderon, L., O. Rodriguez, and D. Shirk. 2018. Drug violence in Mexico. https://justiceinmexico.org/2018-drug-violence-mexico-report/.
- Camacho, A., and C. Rodriguez. 2013. “Firm Exit and Armed Conflict in Colombia.” Journal of Conflict Resolution 57 (1): 89–116. doi:10.1177/0022002712464848
- Caudillo, M., and F. Torche. 2014. “Exposure to Local Homicides and Early Educational Achievement in Mexico.” Sociology of Education 87: 89–105. doi:10.1177/0038040714523795
- CentrePiece. 2015. Crime and violence in Latin America and the Caribbean: towards evidence-based policies. http://cep.lse.ac.uk/pubs/download/cp461.pdf.
- Choi, I. 2001. “Unit Root Tests for Panel Data.” Journal of International Money and Finance 20: 249–272. doi:10.1016/S0261-5606(00)00048-6
- Cole, J., and A. Marroquin. 2009. “Homicide Rates in a Cross-Section of Countries: Evidence and Interpretations.” Population and Development Review 35: 749–776. doi:10.1111/j.1728-4457.2009.00307.x
- Collier, P. 1999. “On the Economic Consequences of Civil War.” Oxford Economic Papers 51: 168–183. doi:10.1093/oep/51.1.168
- Cook, L. 2014. “Violence and Economic Activity: Evidence from African American Patents, 1870-1940.” Journal of Economic Growth 19: 221–257. doi:10.1007/s10887-014-9102-z
- Coronado, R., and E. Saucedo. 2018. “Drug-related Violence in Mexico and its Effects on Employment.” Empirical Economics, doi:10.1007/s00181-018-1458-z.
- Cotte, A. 2007. Growth, inequality and poverty: an analysis of the violence in Colombia. Serie de Documentos en Economía y Violencia 003984. Centro de Investigaciones en Violencia, Instituciones y Desarrollo Económico (VIDE).
- Cotte, A. 2011a. “Socio-Economic Development and Violence: An Empirical Application for Seven Metropolitan Areas in Colombia.” Peace Economics, Peace Science and Public Policy 17 (1), doi:10.2202/1554-8597.1223.
- Cotte, A. 2011b. “Economic Development and Growth in Colombia: An Empirical Analysis with Super-Efficiency DEA and Panel Data Models.” Socio-Economic Planning Sciences 45: 154–164. doi:10.1016/j.seps.2011.07.003
- Cotte, A. 2012. “Estimating Effectiveness of the Control of Violence and Socioeconomic Development in Colombia: An Application of Dynamic Data Envelopment Analysis and Data Panel Approach.” Social Indicators Research 105: 343–366. doi:10.1007/s11205-010-9772-7
- Darby, J., C. Li, and V. Muscatelli. 2004. “Political Uncertainty, Public Expenditure and Growth.” European Journal of Political Economy 20: 153–179. doi:10.1016/j.ejpoleco.2003.01.001
- De Groot, O. J., C. Bozzoli, A. Alamir, and T. Brück. 2022. “The Global Economic Burden of Violent Conflict.” Journal of Peace Research 59 (2): 259–276. doi:10.1177/00223433211046823
- Dell, M. 2014. Trafficking Networks and the Mexican Drug War. https://scholar.harvard.edu/files/dell/files/121113draft_0.pdf.
- Diallo, I. 2018. How Internal Violence Lowers Economic Growth: A Theoretical and Empirical Study. MPRA Paper No. 88285. https://mpra.ub.uni-muenchen.de/88285/.
- Driffield, N., C. Jones, and J. Crotty. 2013. “International Business Research and Risky Investments, an Analysis of FDI in Conflict Zones.” International Business Review 22 (1): 140–155. doi:10.1016/j.ibusrev.2012.03.001
- Durante, R., and E. Gutierrez. 2013. “Fighting Crime with a Little Help from my Friends: Party Affiliation, Inter-Jurisdictional Cooperation and Crime in Mexico.” Sciences Po Publications 17.
- Elgar, F., and N. Aitken. 2010. “Income Inequality, Trust and Homicide in 33 Countries.” European Journal of Public Health 21: 241–246. doi:10.1093/eurpub/ckq068
- Enamorado, T., L. López-Calva, and A. Rodriguez-Castelan. 2013. Crime and Growth Convergence. Evidence from Mexico. Policy Research Working Paper 6730. http://documents.worldbank.org/curated/en/441081468299351843/pdf/WPS6730.pdf.
- Fajnzylber, P., D. Lederman, and N. Loayza. 2002. “What Causes Violent Crime?” European Economic Review 46: 1323–1357. doi:10.1016/S0014-2921(01)00096-4
- Gagne, D. 2015. Rising Violence Could Impact LatAm Economic Development: World Bank. https://www.insightcrime.org/news/brief/rising-violence-could-impact-latam-economic-development-world-bank/.
- Gonzalez, C. 2021. “Butterflies, Organized Crime, and “Sad Trees”: A Critique of the Monarch Butterfly Biosphere Reserve Program in a Context of Rural Violence.” World Development 142. doi:10.1016/j.worlddev.2021.105420.
- Hart, Rachel. 2015. An Analysis of Global Homicide Patterns. https://www.econ.berkeley.edu/sites/default/files/HART-Honors%20Thesis.pdf.
- Herrera, S., and C. Martinez. 2022. “Diversifying Violence: Mining, Export-Agriculture, and Criminal Governance in Mexico.” World Development 151. doi:10.1016/j.worlddev.2021.105769.
- Hojman, D. E. 2004. “Inequality, Unemployment and Crime in Latin American Cities.” Crime, Law and Social Change 41: 33–51. doi:10.1023/B:CRIS.0000015327.30140.8d.
- Im, K., H. Pesaran, and Y. Shin. 2003. “Testing for Unit Roots in Heterogeneous Panels.” Journal of Econometrics 115: 53–74. doi:10.1016/S0304-4076(03)00092-7
- Institute for Economics & Peace. Global Peace Index. 2022. Measuring Peace in a Complex World. http://visionofhumanity.org/resources.
- Kao, C., and M. H. Chiang. 2000. “On the Estimation and Inference of a Cointegrated Regression in Panel Data.” In Nonstationary Panels, Panel Cointegration and Dynamic Panels. Advances in Econometrics, 15, edited by B. H. Baltagi, 179–222. New York: Elsevier.
- Levin, A., C. Lin, and C. Chu. 2002. “Unit Root Tests in Panel Data: Asymptotic and Finite-Sample Properties.” Journal of Econometrics 108: 1–24. doi:10.1016/S0304-4076(01)00098-7
- Liu, Y., M. Fullerton, and N. Ashby. 2013. “Assessing the Impacts of Labour Market and Deterrence Variables on Crime Rates in Mexico.” Contemporary Economic Policy 31: 669–690. doi:10.1111/j.1465-7287.2012.00339.x.
- López, G. 2016. The war on drugs, explained. https://www.vox.com/cards/war-on-drugs-marijuana-cocaine-heroin-meth/war-on-drugs-problems.
- Maddala, G., and S. Wu. 1999. “A Comparative Study of Unit Root Tests with Panel Data and a new Simple Test.” Oxford Bulletin of Economics and Statistics 61: 631–652. doi:10.1111/1468-0084.0610s1631
- Muller, H., and J. Tobias. 2016. The Cost of Violence: Estimating the Economic Impact of Conflict. IGC Growth Brief Series 007. London: International Growth Centre.
- Muñiz, V., P. Fuerte, and K. Méndez. 2022. “The Killing Fields. A Bayesian Analysis of Crop Eradication and Organized Crime Violence in Mexico.” Spatial Statistics 47), doi:10.1016/j.spasta.2021.100553.
- Nadanovsky, P., and J. Cunha-Cruz. 2009. “The Relative Contribution of Income Inequality and Imprisonment to the Variation in Homicide Rates among Developed (OECD), South and Central American Countries.” Social Science & Medicine 69: 1343–1350. doi:10.1016/j.socscimed.2009.08.013
- Pedroni, P. 1999. “Critical Values for Cointegration Tests in Heterogeneous Panels with Multiple Regressors.” Oxford Bulletin of Economics and Statistics 61: 653–670. doi:10.1111/1468-0084.61.s1.14
- Pedroni, P. 2000. “Fully Modified OLS for Heterogeneous Cointegrated Panels.” Advanced in Econometrics 15: 93–130. doi:10.1016/S0731-9053(00)15004-2
- Pedroni, P. 2001. “Purchasing Power Parity Tests in Cointegrated Panels.” Review of Economics and Statistics 83: 727–731. doi:10.1162/003465301753237803
- Pedroni, P. 2004. “Panel Cointegration: Asymptotic and Finite Sample Properties of Pooled Time Series Tests with an Application to the PPP Hypothesis: New Results.” Econometric Theory 20: 597–627. doi:10.1017/S0266466604203073
- Pridemore, W. 2011. “Poverty Matters: A Reassessment of the Inequality-Homicide Relationship in Cross-National Studies.” British Journal of Criminology 51: 739–772. doi:10.1093/bjc/azr019
- Rios, V. 2014. “The Role of Drug-Related Violence and Extortion in Promoting Mexican Migration: Unexpected Consequences of a Drug War.” Latin American Research Review 49 (3): 199–217. doi:10.1353/lar.2014.0038
- Rios, V. 2017. Crime and violence effects on economic diversity: The case of Mexico’s drug war. https://scholar.harvard.edu/files/vrios/files/crimeanddiver2017_v4.pdf.
- Roberts, A., and D. Willits. 2015. “Income Inequality and Homicide in the United States.” Homicide Studies 19: 28–57. doi:10.1177/1088767914557811
- Robinson, S., K. Thierfelder, V. Korman, M. Kearney, and G. Delfin. 2009. Wage Subsidy and Labor Market Flexibility in South Africa. World Bank Policy Research Working Paper No. 4871.
- Robles, G., G. Calderón, and B. Magaloni. 2015. The Economic Consequences of Drug Trafficking Violence in Mexico. https://cddrl.fsi.stanford.edu/sites/default/files/cddrl_robles_calderon_and_magaloni_2015_economic_consecuences_drug_trafficking_violence_mexico.pdf.
- Schippa, C. 2016. War costs us $13.6 trillion. So why do we spend so little on peace?. https://www.weforum.org/agenda/2016/06/the-world-continues-to-spend-enormous-amounts-on-violence-and-little-on-building-peace.
- Schippa, C. 2017. Conflict costs us $13.6 trillion a year. And we spend next to nothing on peace. https://www.weforum.org/agenda/2017/01/how-much-does-violence-really-cost-our-global-economy/.
- Shemyakina, O. 2011. “The Effect of Armed Conflict on Accumulation of Schooling: Results from Tajikistan.” Journal of Development Economics 95 (2): 186–200. doi:10.1016/j.jdeveco.2010.05.002
- Souza, E., and M. Carvalho. 2006. “The Panorama of Urban Violence in Brazil and its Capitals.” Ciência & Saúde Coletiva 11: 363–373. doi:10.1590/S1413-81232006000200014
- Stock, J. H., and M. W. Watson. 1993. “A Simple Estimator of Cointegrating Vectors in Higher Order Integrated System.” Economometrica 61: 783–820. doi:10.2307/2951763
- UNDP. 2021. Regional Human Development Report 2021. Trapped: high inequality and low growth in Latin America and the Caribbean. https://www.undp.org/latin-america/publications/regional-human-development-report-2021-trapped-high-inequality-and-low-growth-latin-america-and-caribbean.
- UNODOC. 2011. TransnaTional organized Crime in CenTral ameriCa and The Caribbean. https://www.unodc.org/documents/toc/Reports/TOCTASouthAmerica/English/TOCTA_CACaribb_cocaine_orgCrimegroups_violence.pdf.
- World Bank. 2015. Global Economic Prospects. Having Fiscal Space and Using it. https://www.worldbank.org/content/dam/Worldbank/GEP/GEP2015a/pdfs/GEP15a_web_full.pdf.
- Zepeda, R. 2018. “Seven Explanatory Approaches About the Increasing of Violence in Mexico.” Política y Gobierno 25: 185–211.
- Zepeda, R., and C. Perez. 2022. “Effects of Long-Term Development and Schooling Expansion on the Decline in Homicide Rates: Mexico from 1950 to 2005.” Journal of Crime and Justice, doi:10.1080/0735648X.2022.2050277.