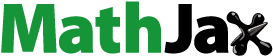
ABSTRACT
Studies on spatial structure features and their influence are increasingly receiving great attention from scholars and policymakers as they play an essential role in any geographical area’s planning and economic development. Therefore, this study analyses the specific impact of spatial structure on labour income in a typical case in Vietnam. Using instrumental variables combined with two-stage least squares regression analysis (TSLS), the results from the study show the specific effects of spatial structure on labour income. Urbanization externalities are reflected in the size of the urban population, which positively impacts the labour income in the region. In contrast, the population dispersion in the decentralized model brings negative impacts. Besides, when the spatial structure of localities tends to be highly polycentric, it will have a negative impact on labour income; however, this effect is dominated by the size of the urban population.
1. INTRODUCTION
Labour income has long been considered one of the critical indicators to assess the development of the economy of a specific country or region. In addition, labour income is also a core indicator showing the living standards of residential areas. With a higher income, people can improve living conditions and have better access to basic needs such as food, healthcare, education and entertainment (Cong & Van, Citation2021; Singh et al., Citation2022). Besides, income disparity is also a problem that receives excellent attention on a global scale when it is directly related to the process of wealth division and social inequality (Du et al., Citation2022). Because of the above reasons, labour income has long been a hot topic, receiving the attention of researchers and policymakers (Cong & Van, Citation2021; Ravallion, Citation2014).
According to neoclassical economic theory, the marginal value of labour factors determines the income level. Many related studies have been developed on this point of view, one of which is Mincer’s study. By emphasizing the role of experience and human capital, Mincer (Citation1974) proposed a fundamental function that helps determine labour income. From this function, Card (Citation1999) and Heckman et al. (Citation2006) have introduced proxies derived from individual characteristics such as education level, experience, gender, race and marital status to analyse more closely the impact of these factors on income. It cannot be denied that individual factors play an important role in determining labour income. However, it is clear that if any individual is in a social environment, they will be affected by the local characteristics of where they live. Therefore, individual factors are not all in the analysis of income differences (Cortes & Tschopp, Citation2020; Ferreira et al., Citation2022; Fontes et al., Citation2010). Although some studies use regional characteristics as explanatory variables for local labour income, surprisingly most of them have just stopped at analysing non-spatial factors such as fixed capital investment rate, government spending, foreign direct investment (FDI) inflows, etc. (Liu & Yin, Citation2010; Pandya, Citation2010). Spatial factors, especially the organization of spatial structure, have been ignored in the analysis of labour income in previous studies. At the same time, the New Economic Geography theories suggest that research on economic activity cannot ignore the external impacts of space. This raises the question of whether spatial structure affects labour income in geographical areas. Furthermore, this is also the goal that this study wants to achieve. In fact, many studies have demonstrated the relationship between spatial structure and productivity (Barde, Citation2010; Meijers & Burger, Citation2010; Ouwehand et al., Citation2022). Income, wages and productivity are closely related. The change in productivity is the driving force for the change in wages (Mincer, Citation1974), while wages are generally the main component of income. However, spatial factors clearly impact many aspects of generating income for workers, not simply wages. In particular, in many areas with a high percentage of self-employed or workers with income diversity, the study of wages does not seem to be sufficiently meaningful, while total income can provide a more comprehensive picture of an individual’s standard of living.
Vietnam, a typical developing country, is going through a rapid development process with a significant change in per capita income from US$94.56 in 1989 to US$2700 in 2019.Footnote1 Besides, the spatial structure of localities has also marked changes along with the urbanization process. If the proportion of the population living in urban areas in 1989 was 19.89%, after 30 years this nearly doubled and reached 36.62%.Footnote2 Although the effect of spatial structure on changes in labour income has been studied relatively commonly in Europe and the United States, where the spatial structure is established with a high degree of urbanization, similar studies for Asian countries seem to be ignored, especially for countries in the process of urbanization. With the above characteristics, Vietnam is an ideal case study for analysing the impact of spatial structure on labour income. Research results enrich the understanding of scientific aspects and provide statistical evidence for policymakers in planning and development orientation for some geographical regions, especially in developing countries.
The remainder of the paper is structured as follows. Section 2 reviews the related underlying theories. Research methods and data are described in section 3. Research results and discussion will be presented in sections 4 and 5, respectively. Section 6 concludes.
2. LITERATURE REVIEW
The impact of spatial structure on labour income is ordinarily presented in two types: agglomeration economies and regional linkage. First, the spatial structure shows the current state of the agglomeration economies. At the same time, agglomeration economies will bring certain advantages to enterprises to improve productivity by cutting costs of moving goods, allocating labour effectively and knowledge spillover (Ahlfeldt et al., Citation2015; Cong, Citation2022; Glaeser, Citation2010). Second, the spatial structure provides information about the relationship between geographical areas, predominantly urban and rural areas. This linkage is essential in raising labour income based on each geographical area’s characteristics and functions (Truong Cong, Citation2021). Although spatial structure has been mentioned many times in geo-economic studies, the representation of spatial structure has never been straightforward (Van Meeteren et al., Citation2016). They require answering questions about the degree of population dispersion and uniform population distribution between cities (Anas et al., Citation1998). Meijers and Burger (Citation2010) proposed the contrast aspects of the spatial structure for this problem as monocentric versus polycentric, dispersion versus concentration. These aspects were then commonly combined with urbanization externalities to represent the spatial structure of a particular geographical area (Ouwehand et al., Citation2022; Sun & Lv, Citation2020).
2.1. Urbanization externalities and labour income
Urbanization externalities and their effects on economic activity have been discussed extensively in the previous literature. Empirical studies have provided concrete evidence of the benefits of urban size or density (Meijers & Burger, Citation2010). It can be seen that urbanization externalities are considered economic externalities, which help businesses take advantage of the agglomeration economy (Ciccone, Citation2002; Graham et al., Citation2010). These externalities are related to diverse and abundant labour resources, adequate public utilities, good infrastructure, crowded institutions, research facilities and universities (Dogaru et al., Citation2016; Meijers & Burger, Citation2010). With the above advantages, enterprises in urban areas can easily increase output and productivity (Ouwehand et al., Citation2022), thereby increasing the income level of labour (Tabuchi & Yoshida, Citation2000). According to Combes et al. (Citation2008), spatial wage disparities can be attributed to spatial differences in the skill composition of the workforce, non-human endowments and local interactions. The difference in individual skills plays an important role, and the interaction effect is mainly driven by the density of local employment. Workers with better labour market characteristics tend to focus on the larger, denser and more skilled local labour market. Similarly, Glaeser and Mare (Citation2001) show a cross-effect between urban residence and experience. The bulk of the urban wage premium comes from an interaction between urban residence and skill accumulation. Cities seem to speed wage growth. In addition, market potential (Fingleton & Longhi, Citation2013), market concentration (Thoresson, Citation2021) and market access (Fallah et al., Citation2011) will also affect labour income.
2.2. Polycentric–monocentric dimension and labour income
While the urbanization externalities are related to the benefits from the size of the city, the polycentric–monocentric dimension is related to the impact of the difference in size between cities (Meijers & Burger, Citation2010). The monocentricity or polycentricity is essentially a convergence of other concepts used to describe a dimension of spatial structure (Burger & Meijers, Citation2012; Liu et al., Citation2016). These concepts were previously expressed in terms of describing ‘multi-core city areas’, ‘network cities’, ‘city networks’ or ‘polynucleated metropolitan regions’ (Batten, Citation1995; Batty, Citation2001; Camagni & Salone, Citation1993; Dieleman & Faludi, Citation1998; Glaeser et al., Citation2016). This spatial dimension is well documented and described in detail in some countries with dynamic economic activity, such as the United States (Angel & Blei, Citation2016; Arribas-Bel & Sanz-Gracia, Citation2014), Japan (Phelps & Ohashi, Citation2020) and China (Liu & Wang, Citation2016). In addition, the hierarchy of cities is considered to have a strong relationship with the wage gap by city size (Soto & Paredes, Citation2016). In large cities where land is scarce, workers demand higher wages to compensate for higher house prices (Roback, Citation1982). However, this wage will decrease gradually with distance to the urban centres (Partridge et al., Citation2009). Effects from the polycentric–monocentric dimension are also found in productivity studies (Li & Liu, Citation2018; Meijers & Burger, Citation2010; Ouwehand et al., Citation2022; Wang et al., Citation2019), traffic congestion (Li et al., Citation2019), retail amenities (Burger et al., Citation2014) and innovation capacity (Li & Du, Citation2022). According to Glaeser et al. (Citation2016) and Meijers et al. (Citation2016), municipalities, residents and businesses can benefit from mutual spillovers and take advantage of ‘borrowed size’ from neighbouring municipalities. In essence, the impact of the polycentric–monocentric dimension on income is also derived from the characteristics of the agglomeration economy, but this aspect has much to do with overcoming negative aspects of the agglomeration economy (Ouwehand et al., Citation2022). The disadvantages of agglomeration, such as pollution, noise levels, congestion, rising land prices and additional costs brought about by stiff competition, become more extensive problems for individual municipalities with a high degree of concentration, but less for the scattered small-scale urban networks (Parr, Citation2004). Empirical evidence also supports the view that a more regionalized structure is increasingly associated with an agglomeration advantage (Capello & Camagni, Citation2000; Sassen, Citation2007). The reason for this problem is that the agglomeration advantage is likely to be replaced by a network (Johansson & Quigley, Citation2003). In addition, according to Fingleton and Longhi (Citation2013), the income of labour is not only affected by the market potential at the labour’s family location but also by the surrounding locations; therefore, the polycentric–monocentric dimension is an interesting problem in the analysis of labour income.
2.3. Dispersed–centralized dimension and labour income
Dispersed–centralized dimension of spatial structure refers to the situation in which the population lives spatially dispersed with a pattern of non-concentration or concentration into urban areas (Meijers & Burger, Citation2010; Ouwehand et al., Citation2022). The spatial structure exists in a dispersed form, which can lead to the consumption of more land, infrastructure and other types of costs (Meijers & Burger, Citation2010). Meanwhile, cities of different sizes all play essential roles in the economy. Even medium-sized cities are considered economic centres; they connect small cities and rural areas to an increasingly diverse range of goods and services, promoting job creation in those areas (Berdegué et al., Citation2015; Soto & Paredes, Citation2016). In addition, the impact of this dimension on income is most evident in the relationship between urban and rural areas. When the spatial structure is more concentrated, which means the formation of more urban areas or larger urban areas, then scattered rural areas adjacent to urban areas will enjoy certain benefits from these urban areas. Urban impacts on neighbouring areas are expressed through labour, capital, goods and information flows, which help rural areas increase incomes and improve productivity (Bac, Citation2022; Truong Cong, Citation2021). Empirical evidence for labour income variation near urban areas has been found in China (Duvivier et al., Citation2013), Japan (Tabuchi, Citation2019), Rwanda (Nilsson, Citation2019) and Brazil (Ferreira & Lanjouw, Citation2001).
3. METHODOLOGY AND DATA
3.1. Measurement of spatial structure
As mentioned above, this study analyses the impact of spatial structure on income based on urbanization externalities with two dimensions: polycentric–monocentric and dispersed–centralized (), in which the urban population simply measures urbanization externalities in localities (Ciccone, Citation2002; Meijers & Burger, Citation2010; Ouwehand et al., Citation2022). For the two rest dimensions, there is still no consensus on how to measure them in the relevant literature (Ouwehand et al., Citation2022). In fact, there is a great debate about the polycentric–monocentric approaches. It is due to two distinct perspectives on understanding and measuring polycentricity (Burger & Meijers, Citation2012; Green, Citation2007). In this, a morphological approach centring on nodal features and a functional approach focused on the relations between centres. Morphological and functional polycentricity do not necessarily change in one direction (Chen et al., Citation2021; Veneri & Burgalassi, Citation2012). According to Burger and Meijers (Citation2012), most regions tend to be morphologically polycentricity rather than functionally polycentricity. The difference is primarily explained by the size, external connectivity and degree of self-sufficiency of a region’s principal centre. This is also consistent with the results of Yue et al. (Citation2019) that the development of functional polycentricity is generally slower than the morphological polycentricity. Because of this, the polycentric–monocentric dimension can be measured through various methods such as the ‘Exploratory spatial data analysis (ESDA-based) approach’ (Li & Du, Citation2022), ‘Threshold-based methods’ (Taubenböck et al., Citation2017) and ‘hierarchical clustering method’ (Roth et al., Citation2011). However, in cases where it is necessary to use instrumental variables to solve the endogeneity problem, especially using similar variables in the past, the above measures become less effective (Ouwehand et al., Citation2022). Recently, some scholars have proposed a measure by calculating the ‘slope of the regression line of the rank–size distribution’ for the polycentric–monocentric aspect. This measure also meets the requirements in analysis using instrumental variables (Burger et al., Citation2014; Ouwehand et al., Citation2022).
Figure 1. Dimensions in spatial structure.
Source: Adapted from Meijers and Burger (Citation2010).
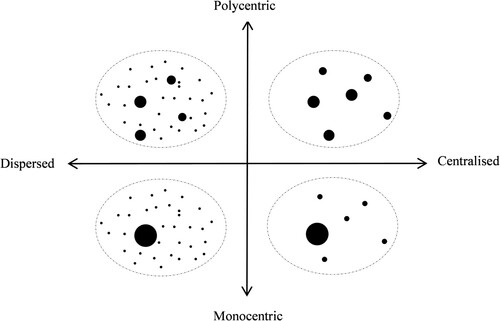
With the aim of an in-depth assessment of the effect of spatial structure on income, this study also uses instrumental variables to eliminate the problems of reverse causality and unobserved heterogeneity. From the theoretical background, the impact of spatial structure on labour income seems quite clear. However, labour income can affect the spatial structure in the opposite direction. For example, when income in an area increases, its jobs will become attractive and lead to a large number of workers from other areas moving to and changing the spatial structure of the area (Wang & Chen, Citation2019; Yu et al., Citation2019). Therefore, the degree of polycentricity in this study is measured by the slope of the regression line of the rank–size distribution. The specific way of measuring is shown in equation (1):
where the left-hand side represents the size of the urban population (u) in the area (r), and the right-hand side represents the rank of this urban size in the area.
The coefficient estimation results will represent the slope of the regression line of the rank–size distribution or the degree of polycentric–monocentric dimension in the area (r). The steeper regression line reflects the more unbalanced size between cities, the more hierarchical the distribution, and the more monocentric structure. In contrast, the flatter regression line reflects the more balanced size between cities or the lower hierarchy and a more polycentric structure. Another essential feature is that in this method of estimating the rank–size distribution, the coefficient always tends to slope from left to right; in other words, the estimated coefficient always has a negative sign. The coefficient estimation results, therefore, are also the degree of polycentricity of areas. In the calculation, areas with more cities usually show more polycentric (Burger & Meijers, Citation2012). Therefore, this study refers to the application of measures by Brezzi and Veneri (Citation2015) and Ouwehand et al. (Citation2022). Specifically, for areas with many urban areas, the degree of polycentricity or monocentricity will be only calculated through the three or four largest urban areas. Specific examples of the polycentric–monocentric dimension of areas are shown in , where the slope of the regression line of the relationship between rank and size is higher for Ninh Thuan than for Đăk Nong. This shows that Ninh Thuan province has a higher degree of monocentricity with the dominance of Phan Rang-Thap Cham, while Dak Nong has a higher degree of polycentricity with urban areas that are almost similar in size.
As for the decentralized–centralized dimension, there are different complex ways to capture this aspect of spatial structure (Ouwehand et al., Citation2022). However, a simple and common way to reflect this dimension is to use the proportion of the population that does not live in urban areas (Meijers & Burger, Citation2010; Ouwehand et al., Citation2022). This index will show the extent to which the population lives dispersed in space according to a decentralized pattern.
Figure 2. Rank–size distribution for Ninh Thuan (left) and Đăk Nong (right).
Source: Author’s calculations.
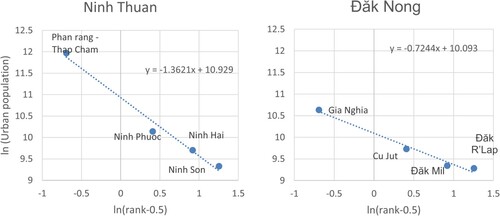
In addition to the effects from the assumed spatial aspects, the research model also adds several other factors, which have been found to affect labour income in previous studies. According to Cortinovis and Van Oort (Citation2019), human capital is essential in analysing the impact of economies of agglomeration. Ignoring this factor can lead to erroneous results in the estimate because the quality of labour is often proportional to the scale of economies of agglomeration. One of the ways to measure human capital is simply education level (Ouwehand et al., Citation2022). At the same time, much empirical evidence shows that education level is one of the crucial determinants of labour income (Acosta et al., Citation2019; Ferreira et al., Citation2022; Rinz, Citation2022). In addition, the concentration of different industries also varies, which affects the economies of agglomeration. Therefore, the economic structure of an area is also expected to have specific effects on its labour income (Ferreira et al., Citation2022; Rinz, Citation2022). Another important factor mentioned in the model is the transport infrastructure. The improvement of infrastructure will help increase connectivity between companies and increase the efficiency of the economies of agglomeration as well as the income of labour (Duvivier et al., Citation2013; Liu et al., Citation2016; Ouwehand et al., Citation2022). Finally, the factors of natural conditions or geographical location also can affect labour income, so they are also controlled in the model.
This study also uses instrumental variables to control for bias due to omitting important variables and potential two-way relationships in the model. It is clear that the income of labour can also affect the spatial structure of an area. When the local income is high, it will attract labour from other areas to migrate, causing a change in its spatial structure. In order to ensure the validity of the analytical results, this study conducts a two-stage least square (TSLS) regression with instrumental variables representing the spatial aspect.
From the factors mentioned above. The general model of this study is proposed as follows:
where Inci is the income of labour in area i; Edu is the variable that reflects the level of education; NonAgr is the variable that represents the local economic structure; Trans is a variable describing transportation infrastructure; X is a set of variables representing dimensions of the spatial structure, including: urban population size (Urb), degree of polycentricity (Poly) and degree of dispersion (Dis); and r is the control variable representing aspects of differences in natural conditions and geographical locations of areas.
3.2. Data
This study analysed all 63 provinces of Vietnam with data from 2019. This is the first level in the hierarchy of local government. Each province has at least a population of 900,000 and an area of 5000 km2. In addition, each province has 11 or more subordinate administrative units, including at least one city. This choice ensures that the areas are large enough to be analysed and consistent with the homogeneity between areas (Brezzi & Veneri, Citation2015). For the main explanatory variable, labour income, the study uses data on the total monthly income of local workers, including monthly wages, overtime pay, bonuses, self-employment income and other income. Other monetary benefits accrue as benefits, for example, benefit meals and benefit clothing. For developed countries, wages play an important role in income. However, this is not true for developing countries where self-employment income is the main source. In Vietnam, the self-employment rate accounted for 54.3% of total employment in 2019,Footnote3 so the analysis of labour income would have broader implications. For data showing education level, this study uses the local proportion of trained workers aged 15 years and over to measure. Regarding the economic structure, non-agricultural activities are often associated with high productivity, providing a higher and more stable source of income than agricultural activities. The economic structure change from agricultural to non-agricultural is often associated with economic development and income improvement (Rodrik, Citation2016). Therefore, the proportion of the non-agricultural sector is selected for analysis, and this value is measured by the ratio of the value-added contribution of the non-agricultural sector to the total value added to the region. The development of local transport infrastructure in this study will be controlled by the proportion of the land area used for transport purposes. All these values are extracted from the Vietnamese statistical yearbook. The descriptive statistics for these variables are presented in .
Table 1. Descriptive statistics of variables in models.
As for the main variables of the model, the spatial aspects of the areas have been described in detail on how to measure them in the research methods section. They consist of urbanization externalities, degree of polycentricity and degree of dispersion. The baseline data for this measurement, including urban population and urban population proportion, are also available in the statistical yearbook. In Vietnam, the spatial structure has differences between localities. Metropolises such as Hanoi, Hai Phong, Da Nang, Can Tho and Ho Chi Minh City will often have a high degree of polycentricity when they include many urban areas, and between these urban areas there is not much difference in size. Meanwhile, provinces with low levels of development often have only one large urban area and a few surrounding small urban areas. The main features of the spatial structure of this country is reflected in . In addition, the dummy variables to control for unobservable heterogeneity in natural conditions, the geographical locations of areas were classified into six regions, including Red River Delta, Northern mountain, Central coastal, Central Highlands, South East and Mekong River Delta.
Figure 3. Main features of the spatial structure of Vietnam: (a) population distribution, (b) degree of polycentric–monocentric dimension and (c) degree of decentralized–centralized dimension.
Source: Author’s calculations.
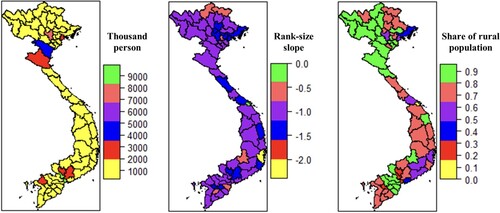
This study relies on previous studies to select suitable variables for instrumental variables, in which the instrumental variables for spatial dimensions can all use the corresponding values in the past (Ciccone, Citation2002; Meijers & Burger, Citation2010; Ouwehand et al., Citation2022). The instrumental variables used in this study will be urban population, degree of polycentricity and degree of dispersion of areas in 1979. This was when Vietnam has just reunified the country and was in the early stages of looking for an appropriate economic management mechanism and policies to develop the economy. It was not until 1986 that Vietnam began to conduct ‘Doi Moi’ with significant changes in socio-economic characteristics. First, transforming from public ownership of the means of production, which took the form of state ownership of industry, and collectivization of the agricultural and handicraft sectors (cooperatives own all means of production, including land, cattle, tools, machines; cooperative members receive products based on their labour contributions), to a multi-sector economy with the existence of many forms of ownership. Second, the transition from central planning by ordinances, associated with the subsidy mechanism, allocating inputs and outputs with prices fixed and regulated to a market economy mechanism with the management of the state at the macro-level ensures the business autonomy of each enterprise. Third, change from a self-sufficient economy to an economy that is open to the world.
4. RESULTS
4.1. Impacts of spatial structure on labour income with ordinary least squares regression (OLS)
shows the results of the analysis of impacts from the spatial dimension on the income of labour in areas. Each model represents cases where different spatial facets are involved, and these models all include control variables. The first column shows the names of the variables incorporated in the model, and the remaining columns show the corresponding regression results of these variables. Models (1) to (3) describe the case with only one spatial dimension; models (4) to (6) describe the case with a combination of two spatial dimensions; and model (7) reflects the case where all three spatial dimensions are present.
Table 2. Results from ordinary least squares regression (OLS) for the effects of spatial structure on labour income.
The results from the models show that the effects of the control variables, including the level of education, the development of transport infrastructure, and the natural characteristics of areas on labour income, are all statistically significant. Meanwhile, the coefficient of the variable representing the economic structure of the locality has a positive sign but is not statistically significant. Regarding spatial structure, single and combined models have shown that these factors have specific effects on labour income. First, urbanization externalities – are represented by the total urban population, whose estimated coefficients are positive and statistically significant at the 1% level when combined into any of the different models. For the polycentric–monocentric dimension, the coefficient of this variable is all negative and only statistically significant in the presence of urbanization externalities. Furthermore, this study also did not detect multicollinearity among the variables included in the model. Therefore, estimates of the impact of the polycentric–monocentric dimension on labour income may be inaccurate when not controlling for urbanization externalities. Finally, the dispersed–centralized dimension shows that the estimated coefficient is negative and is always statistically significant at the 1% level.
4.2. Impacts of interaction between urbanization externalities and spatial structure on labour income
As mentioned above, the urban population affects the impact of the polycentric–monocentric dimension on labour income. It suggests there may be the existence of moderate effects between spatial dimensions. Therefore, examining the impact of interactions between urbanization externalities and the remaining spatial dimensions on labour income is necessary. In , model (8) represents the case of interaction between urbanization externalities, and degree of polycentricity; model (9) represents the case of interaction between urbanization externalities and degree of dispersion; and model (10) reflects the case of both interactions at the same time. The results show that the urbanization externalities and the degree of dispersion are still statistically significant factors in the forecast of labour income when combining all three spatial dimensions in their model. The magnitude of coefficients and standard deviation of these variables are almost unchanged when adding variables describing interactions between spatial aspects. In addition, the interaction between urbanization externalities and the polycentric–monocentric dimension shows a negative effect on labour income at a 1% significance level in both models in which it is present. In contrast, the interaction between urbanization externalities and the dispersed–centralized dimension does not impact labour income because its coefficient is not statistically significant.
Table 3. Results from ordinary least squares regression (OLS) for the effects of interaction between spatial dimensions on labour income.
4.3. Impacts of spatial structure on labour income with two-stage least squares (TSLS)
In order to ensure the certainty of the assessment of the relationship between the spatial structure and labour income, this study examines the estimated results of the model with the instrumental variable using TSLS. The estimation and testing results of the models are presented in . These models cover three single spatial dimensions: urbanization externalities, polycentric–monocentric and dispersed–centralized dimensions. The only difference among them is the combination of interaction between spatial dimensions. Model (11) is a model that represents the case where there is no interaction between spatial aspects. In contrast, models (12) and (13) respectively reflect the case where there is an interaction between urbanization externalities with the polycentric–monocentric dimension and dispersed–centralized dimension. The test results from support using instrumental variables in the model. The weak instrumental variables test (F-test) helps to check whether the selected instrumental variables are strongly correlated with the endogenous variables. The F-test values for all five endogenous variables are statistically significant at the 1% level and always greater than 10 (consistent with the ‘rule-of-thumb threshold’). Similarly, the Wu–Hausman test shows that the variables considered endogenous are all endogenous at the 5% significance level. In addition, the Sargan test also indicates no statistical evidence for the endogenous phenomena of instrumental variables included in the model.
Table 4. Results from two-stage least squares (TSLS) for the effects of spatial dimensions on labour income.
For the estimation results, all three models in show that the coefficients and significance levels of the predictors are similar to the results estimated by OLS. The variables representing the education level, transport infrastructure development, and the dummy variables representing the geographical areas are statistically significant. In contrast, the variable's coefficient representing the local economic structure is not statistically significant. Regarding spatial structure, dispersed–centralized dimension, and urbanization externalities still strongly predict labour income. Similarly, the coefficients of the variables representing the interaction between spatial aspects also have the same statistical significance compared with the results of OLS estimation. There is a slight difference in the regression results using TSLS and OLS that the coefficient of the variable reflecting the polycentric–monocentric dimension is significant only in models (11) and (13). In contrast, this coefficient is not statistically significant in the model with the interaction between urbanization externalities and the polycentric–monocentric dimension (12).
5. DISCUSSION
From the results presented above, the impact of spatial structure on labour income is undeniable. However, different dimensions of the spatial structure have different effects. First, urbanization externalities are reflected in the urban population, positively affecting labour income. This finding is not surprising when it is consistent with previous research results. A large urban population will benefit through agglomeration economies, thereby improving productivity and labour income (Graham et al., Citation2010; Tabuchi & Yoshida, Citation2000; Thoresson, Citation2021). Second, the dispersed–centralized dimension significantly impacts labour income. Specifically, with a high degree of dispersion, effects from agglomeration economies are limited, and regional linkage patterns such as growth poles or core–periphery are not productive (Bac, Citation2022; Truong Cong, Citation2021), thus leading to a negative impact on labour income. Regarding the polycentric–monocentric dimension, the impact of this aspect on labour income is unclear. In the regression results by OLS, the degree of polycentricity in the spatial structure tends to impact labour income negatively. However, this effect is only significant when controlling the urban population. More specifically, labour income will decrease when the spatial structure has an increased degree of polycentricity. The particular point is that for the regression using TSLS, the coefficient expressing the direct impact of the polycentric–monocentric dimension on labour income becomes insignificant when adding the variable representing the interaction between urbanization externalities and polycentricity. This shows that the impact of the degree of polycentricity in spatial structure on income depends on the urban population. This statement is consistent with the conclusion in the study of Meijers and Burger (Citation2010), and Ouwehand et al. (Citation2022) argue that the impact of the degree of polycentricity becomes less significant when placed in regions with a large urban population. At the same time, this finding is also highly consistent with Brezzi and Veneri (Citation2015). They argue that the degree of polycentricity affects gross domestic product (GDP) per capita at different regional scales. However, the above explanation is only suitable for the morphological polycentric approach because there may be differences with the functional polycentric approach. In a somewhat related study on the impact of spatial structure on labour productivity, Kwon and Seo (Citation2018) showed that morphological polycentricity is positively related to labour productivity, while functional polycentricity is negatively associated with it. This also supports the point of heterogeneity of the two approaches in terms of polycentricity (Chen et al., Citation2021; Veneri & Burgalassi, Citation2012). In addition, the results from the model with TSLS also show that controlling for endogenous phenomena plays a vital role in analysing the impact of spatial dimensions on labour income. Ignoring this issue can lead to inaccurate conclusions.
The coefficients of the control variables on education level and the development of transport infrastructure in all models have positive signs and are statistically significant, showing these are important control variables that have an apparent effect on labour income. Accordingly, the development of human capital through the proportion of trained labour will help improve labour income, and this result also reaffirms the same conclusions about the relationship between human capital and labour income (Ferreira et al., Citation2022; Rinz, Citation2022). Similarly, the development of transport infrastructure will also help improve the connectivity of localities, thereby increasing the efficiency of agglomeration economies and spillover effects. Another critical point is that other factors affect labour income besides those mentioned above. These factors are bundled together and reflected through dummy variables for different localities. Differences in the natural characteristics of localities, such as climate, soil, topography, and regional connectivity, can lead to different sources of income from economic activities based on the specific nature of the locality. In addition, differences in price levels and socio-cultural characteristics associated with geographical characteristics and policies of each region may also cause differences in workers’ income. In Vietnam, corresponding to different geographical areas, the government has different policies to promote specific economic activities with the characteristics of each region. Although this research results do not indicate where this difference comes from specifically. However, this result is a testament to the importance of adding other factors to the characteristics of regions in comparing labour income beyond the spatial structure, human capital and transport infrastructure.
6. CONCLUSIONS
This study analyses the impact of spatial structure on labour income, an essential topic in the planning and development of localities, attracting the attention of researchers in recent times. By taking Vietnam as a typical example of combining TSLS analysis with instrumental variables, this study provides strong evidence of the impact of spatial structure on labour income. The urban population size represents the urbanization externalities that have a positive effect. In contrast, spatial dispersion of the population with a non-centralized model will have a negative impact. This suggests that the local design and planning in the direction of centralizing will contribute to improving labour income. In particular, the polycentricity in spatial structure will tend to impact labour income negatively, and the urban population size influences this effect. The results from this study also show that the analysis of the relationship between the spatial structure and labour income is a complex issue because it interweaves the causal relationship. Therefore, ignoring this fact can lead to erroneous judgments.
Although this is the first study on the impact of spatial structure on labour income in developing countries in general and Vietnam in particular, this study still has certain limitations. First, this study only evaluates based on three general dimensions: urbanization externalities, polycentric–monocentric and decentralized–centralized. Meanwhile, spatial structure can also be reflected in many different aspects. Second, this study uses the ‘rank–size’ relationship to analyse the polycentric–monocentric dimension measurement method, so the approach with a different measure can still give a different result. Third, because of data limitations, this study can only use instrumental variables describing the spatial structure in 1979. Although the tests show that these instrumental variables satisfy the conditions to become a good instrumental variable, the use of instrumental variables in the analysis should be chosen by older historical data to make the results more certain. Finally, although controlled for in this research model, several factors representing differences between geographical areas that affect local income have not been separated because of data availability limitations; therefore, further analysis is required to draw more appropriate conclusions. The limitations presented above are also helpful suggestions for further related studies.
DATA AVAILABILITY
Data available on request from the authors.
DISCLOSURE STATEMENT
No potential conflict of interest was reported by the author.
Additional information
Funding
Notes
1 Data are extracted from https://data.worldbank.org/.
2 Data are extracted from https://data.worldbank.org/.
3 Data are extracted from https://data.worldbank.org/.
REFERENCES
- Acosta, P., Cruces, G., Galiani, S., & Gasparini, L. (2019). Educational upgrading and returns to skills in Latin America: Evidence from a supply–demand framework. Latin American Economic Review, 28(1), 1–20. https://doi.org/10.1186/s40503-019-0080-6
- Ahlfeldt, G. M., Redding, S. J., Sturm, D. M., & Wolf, N. (2015). The economics of density: Evidence from the Berlin wall. Econometrica, 83(6), 2127–2189. https://doi.org/10.3982/ECTA10876
- Anas, A., Arnott, R., & Small, K. A. (1998). Urban spatial structure. Journal of Economic Literature, 36(3), 1426–1464.
- Angel, S., & Blei, A. M. (2016). The spatial structure of American cities: The great majority of workplaces Are no longer in CBDs, employment sub-centers, or live–work communities. Cities, 51, 21–35. https://doi.org/10.1016/j.cities.2015.11.031
- Arribas-Bel, D., & Sanz-Gracia, F. (2014). The validity of the monocentric city model in a polycentric age: US metropolitan areas in 1990, 2000 and 2010. Urban Geography, 35(7), 980–997. https://doi.org/10.1080/02723638.2014.940693
- Bac, T. C. (2022). Исследование структурной трансформации сельской экономики с точки зрения региональных [Revisiting rural economic structural transformation from the viewpoint of regional linkages]. Economy of Region, 18(2), 312–323. https://doi.org/10.17059/ekon.reg.2022-2-1
- Barde, S. (2010). Increasing returns and the spatial structure of French wages. Spatial Economic Analysis, 5(1), 73–91. https://doi.org/10.1080/17421770903511338
- Batten, D. F. (1995). Network cities: Creative urban agglomerations for the 21st century. Urban Studies, 32(2), 313–327. https://doi.org/10.1080/00420989550013103
- Batty, M. (2001). Polynucleated urban landscapes. Urban Studies, 38(4), 635–655. https://doi.org/10.1080/00420980120035268
- Berdegué, J. A., Carriazo, F., Jara, B., Modrego, F., & Soloaga, I. (2015). Cities, territories, and inclusive growth: Unraveling urban–rural linkages in Chile, Colombia, and Mexico. World Development, 73, 56–71. https://doi.org/10.1016/j.worlddev.2014.12.013
- Brezzi, M., & Veneri, P. (2015). Assessing polycentric urban systems in the OECD: Country, regional and metropolitan perspectives. European Planning Studies, 23(6), 1128–1145. https://doi.org/10.1080/09654313.2014.905005
- Burger, M., & Meijers, E. (2012). Form follows function? Linking morphological and functional polycentricity. Urban Studies, 49(5), 1127–1149. https://doi.org/10.1177/0042098011407095
- Burger, M. J., Meijers, E. J., & Van Oort, F. G. (2014). Regional spatial structure and retail amenities in The Netherlands. Regional Studies, 48(12), 1972–1992. https://doi.org/10.1080/00343404.2013.783693
- Camagni, R. P., & Salone, C. (1993). Network urban structures in northern Italy: Elements for a theoretical framework. Urban Studies, 30(6), 1053–1064. https://doi.org/10.1080/00420989320080941
- Capello, R., & Camagni, R. (2000). Beyond optimal city size: An evaluation of alternative urban growth patterns. Urban Studies, 37(9), 1479–1496. https://doi.org/10.1080/00420980020080221
- Card, D. (1999). Handbook of labor economics. Handbook of Labor Economics, 3, 1801–1863. https://doi.org/10.1016/S1573-4463(99)03011-4
- Chen, W., Golubchikov, O., & Liu, Z. (2021). Measuring polycentric structures of megaregions in China: Linking morphological and functional dimensions. Environment and Planning B: Urban Analytics and City Science, 48(8), 2272–2288. https://doi.org/10.1177/2399808320974687
- Ciccone, A. (2002). Agglomeration effects in Europe. European Economic Review, 46(2), 213–227. https://doi.org/10.1016/S0014-2921(00)00099-4
- Combes, P. P., Duranton, G., & Gobillon, L. (2008). Spatial wage disparities: Sorting matters!. Journal of Urban Economics, 63(2), 723–742. https://doi.org/10.1016/j.jue.2007.04.004
- Cong, B. T. (2022). The agglomeration of business types at the regional level in Vietnam. Science & Technology Development Journal–Economics–Law and Management, 6(1), 2101–2114. https://doi.org/10.32508/stdjelm.v6i1.826
- Cong, B. T., & Van, T. Q. (2021). The impacts of spatial interaction on agricultural productivity and average income: A case of Vietnam. Journal La Sociale, 2(2), 12–20. https://doi.org/10.37899/journal-la-sociale.v2i2.372
- Cortes, G. M., & Tschopp, J. (2020). Rising concentration and wage inequality (No. 13557). Institute of Labor Economics (IZA).
- Cortinovis, N., & Van Oort, F. (2019). Between spilling over and boiling down: Network-mediated spillovers, local knowledge base and productivity in European regions. Journal of Economic Geography, 19(6), 1233–1260. https://doi.org/10.1093/jeg/lby058
- Dieleman, F. M., & Faludi, A. (1998). Polynucleated metropolitan regions in northwest Europe: Theme of the special issue. European Planning Studies, 6(4), 365–377. https://doi.org/10.1080/09654319808720468
- Dogaru, T., Van Oort, F., Diodato, D., & Thissen, M. (2016). Agglomeration and knowledge in European regional growth. In S. Conventz, B. Derudder, A. Thierstein, & F. Witlox (Eds.), Hub cities in the knowledge economy (pp. 181–203). Routledge.
- Du, H., Chen, A., Li, Y., Ma, L., Xing, Q., & Nie, Y. (2022). Perceived income inequality increases status seeking among low social class individuals. Asian Journal of Social Psychology, 25(1), 52–59. https://doi.org/10.1111/ajsp.12455
- Duvivier, C., Li, S., & Renard, M. F. (2013). Are workers close to cities paid higher nonagricultural wages in rural China? Applied Economics, 45(30), 4308–4322. https://doi.org/10.1080/00036846.2013.778953
- Fallah, B. N., Partridge, M. D., & Olfert, M. R. (2011). New economic geography and US metropolitan wage inequality. Journal of Economic Geography, 11(5), 865–895. https://doi.org/10.1093/jeg/lbq016
- Ferreira, F. H., Firpo, S. P., & Messina, J. (2022). Labor market experience and falling earnings inequality in Brazil: 1995–2012. The World Bank Economic Review, 36(1), 37–67. https://doi.org/10.1093/wber/lhab005
- Ferreira, F. H., & Lanjouw, P. (2001). Rural nonfarm activities and poverty in the Brazilian Northeast. World Development, 29(3), 509–528. https://doi.org/10.1016/S0305-750X(00)00111-X
- Fingleton, B., & Longhi, S. (2013). The effects of agglomeration on wages: Evidence from the micro-level. Journal of Regional Science, 53(3), 443–463. https://doi.org/10.1111/jors.12020
- Fontes, G. G., Simões, R. F., Oliveira, H. C. D., & M, A. (2010). Urban attributes and wage disparities in Brazil: A multilevel hierarchical model. Regional Studies, 44(5), 595–607. https://doi.org/10.1080/00343400902926367
- Glaeser, E. L. (2010). Introduction to the economics of agglomeration. In E. L. Glaeser (Ed.), Agglomeration economics (pp. 1–14). University of Chicago Press.
- Glaeser, E. L., & Mare, D. C. (2001). Cities and skills. Journal of Labor Economics, 19(2), 316–342. https://doi.org/10.1086/319563
- Glaeser, E. L., Ponzetto, G. A., & Zou, Y. (2016). Urban networks: Connecting markets, people, and ideas. Papers in Regional Science, 95(1), 17–59. https://doi.org/10.1111/pirs.12216
- Graham, D. J., Melo, P. S., Jiwattanakulpaisarn, P., & Noland, R. B. (2010). Testing for causality between productivity and agglomeration economies. Journal of Regional Science, 50(5), 935–951. https://doi.org/10.1111/j.1467-9787.2010.00676.x
- Green, N. (2007). Functional polycentricity: A formal definition in terms of social network analysis. Urban Studies, 44(11), 2077–2103. https://doi.org/10.1080/00420980701518941
- Heckman, J. J., Lochner, L. J., & Todd, P. E. (2006). Handbook of the economics of education. Handbook of the Economics of Education, 1, 307–458. https://doi.org/10.1016/S1574-0692(06)01007-5
- Johansson, B., & Quigley, J. M. (2003). Agglomeration and networks in spatial economies. Papers in Regional Science, 83(1), 165–176. https://doi.org/10.1007/s10110-003-0181-z
- Kwon, K., & Seo, M. (2018). Does the polycentric urban region contribute to economic performance? The case of Korea. Sustainability, 10(11), 4157. https://doi.org/10.3390/su10114157
- Li, Y., & Du, R. (2022). Polycentric urban structure and innovation: Evidence from a panel of Chinese cities. Regional Studies, 56(1), 113–127. https://doi.org/10.1080/00343404.2021.1886274
- Li, Y., & Liu, X. (2018). How Did urban polycentricity and dispersion affect economic productivity? A case study of 306 Chinese cities. Landscape and Urban Planning, 173, 51–59. https://doi.org/10.1016/j.landurbplan.2018.01.007
- Li, Y., Xiong, W., & Wang, X. (2019). Does polycentric and compact development alleviate urban traffic congestion? A case study of 98 Chinese cities. Cities, 88, 100–111. https://doi.org/10.1016/j.cities.2019.01.017
- Liu, X., Derudder, B., & Wu, K. (2016). Measuring polycentric urban development in China: An intercity transportation network perspective. Regional Studies, 50(8), 1302–1315. https://doi.org/10.1080/00343404.2015.1004535
- Liu, X., & Wang, M. (2016). How polycentric is urban China and why? A case study of 318 cities. Landscape and Urban Planning, 151, 10–20. https://doi.org/10.1016/j.landurbplan.2016.03.007
- Liu, X., & Yin, X. (2010). Spatial externalities and regional income inequality: Evidence from China’s prefecture-level data. Frontiers of Economics in China, 5(2), 325–338. https://doi.org/10.1007/s11459-010-0016-1
- Meijers, E. J., & Burger, M. J. (2010). Spatial structure and productivity in US metropolitan areas. Environment and Planning A: Economy and Space, 42(6), 1383–1402. https://doi.org/10.1068/a42151
- Meijers, E. J., Burger, M. J., & Hoogerbrugge, M. M. (2016). Borrowing size in networks of cities: City size, network connectivity and metropolitan functions in Europe. Papers in Regional Science, 95(1), 181–198. https://doi.org/10.1111/pirs.12181
- Mincer, J. A. (1974). Age and experience profiles of earnings. In J. A. Mincer (Ed.), Schooling, experience, and earnings (pp. 64–82). NBER.
- Nilsson, P. (2019). Spatial spillovers and households’ involvement in the non-farm sector: Evidence from rural Rwanda. Regional Studies, 53(5), 731–740. https://doi.org/10.1080/00343404.2018.1482415
- Ouwehand, W. M., van Oort, F. G., & Cortinovis, N. (2022). Spatial structure and productivity in European regions. Regional Studies, 56(1), 48–62. https://doi.org/10.1080/00343404.2021.1950912
- Pandya, S. S. (2010). Labor markets and the demand for foreign direct investment. International Organization, 64(3), 389–409. https://doi.org/10.1017/S0020818310000160
- Parr, J. (2004). The polycentric urban region: A closer inspection. Regional Studies, 38(3), 231–240. https://doi.org/10.1080/003434042000211114
- Partridge, M. D., Rickman, D. S., Ali, K., & Olfert, M. R. (2009). Agglomeration spillovers and wage and housing cost gradients across the urban hierarchy. Journal of International Economics, 78(1), 126–140. https://doi.org/10.1016/j.jinteco.2009.02.004
- Phelps, N. A., & Ohashi, H. (2020). Edge city denied? The rise and fall of Tokyo’s outer suburban “Business core cities”. Journal of Planning Education and Research, 40(4), 379–392. https://doi.org/10.1177/0739456X18773471
- Ravallion, M. (2014). Income inequality in the developing world. Science, 344(6186), 851–855. https://doi.org/10.1126/science.1251875
- Rinz, K. (2022). Labor market concentration, earnings, and inequality. Journal of Human Resources, 57(S), S251–S283. https://doi.org/10.3368/jhr.monopsony.0219-10025R1
- Roback, J. (1982). Wages, rents, and the quality of life. Journal of Political Economy, 90(6), 1257–1278. https://doi.org/10.1086/261120
- Rodrik, D. (2016). Premature deindustrialization. Journal of Economic Growth, 21(1), 1–33. https://doi.org/10.1007/s10887-015-9122-3
- Roth, C., Kang, S. M., Batty, M., & Barthelemy, M. (2011). Structure of urban movements: Polycentric activity and entangled hierarchical flows. PLoS ONE, 6(1), article e15923. https://doi.org/10.1371/journal.pone.0015923
- Sassen S. (2007). Megaregions: Benefits beyond sharing trains and parking Lots? In K. S. Goldfield (Ed.), The economic geography of mega-regions (pp 59–83). The Policy Research Institute for the Region.
- Singh, H., Gill, A. S., & Choudhury, P. K. (2022). Household expenditure on secondary education in Haryana (India): levels, patterns and determinants. Millennial Asia, 09763996211073230. https://doi.org/10.1177/09763996211073230
- Soto, J., & Paredes, D. (2016). Cities, wages, and the urban hierarchy. Journal of Regional Science, 56(4), 596–614. https://doi.org/10.1111/jors.12269
- Sun, T., & Lv, Y. (2020). Employment centers and polycentric spatial development in Chinese cities: A multi-scale analysis. Cities, 99, 102617. https://doi.org/10.1016/j.cities.2020.102617
- Tabuchi, T. (2019). Do the rich and poor colocate in large cities? Journal of Urban Economics, 113, 103186. https://doi.org/10.1016/j.jue.2019.103186
- Tabuchi, T., & Yoshida, A. (2000). Separating urban agglomeration economies in consumption and production. Journal of Urban Economics, 48(1), 70–84. https://doi.org/10.1006/juec.1999.2157
- Taubenböck, H., Standfuß, I., Wurm, M., Krehl, A., & Siedentop, S. (2017). Measuring morphological polycentricity – A comparative analysis of urban mass concentrations using remote sensing data. Computers, Environment and Urban Systems, 64, 42–56. https://doi.org/10.1016/j.compenvurbsys.2017.01.005
- Thoresson, A. (2021). Employer concentration and wages for specialized workers (No. 2021: 6). IFAU – Institute for Evaluation of Labour Market and Education Policy.
- Truong Cong, B. (2021). The impact of metropolises’ characteristics on provincial economic structure transformation: Evidence from Vietnam. Cogent Economics & Finance, 9(1), 1937849. https://doi.org/10.1080/23322039.2021.1937849
- Van Meeteren, M., Poorthuis, A., Derudder, B., & Witlox, F. (2016). Pacifying Babel’s tower: A scientometric analysis of polycentricity in urban research. Urban Studies, 53(6), 1278–1298. https://doi.org/10.1177/0042098015573455
- Veneri, P., & Burgalassi, D. (2012). Questioning polycentric development and Its effects. Issues of definition and measurement for the Italian NUTS-2 regions. European Planning Studies, 20(6), 1017–1037. https://doi.org/10.1080/09654313.2012.673566
- Wang, M., Derudder, B., & Liu, X. (2019). Polycentric urban development and economic productivity in China: A multiscalar analysis. Environment and Planning A: Economy and Space, 51(8), 1622–1643. https://doi.org/10.1177/0308518X19866836
- Wang, Z., & Chen, L. (2019). Destination choices of Chinese rural–urban migrant workers: Jobs, amenities, and local spillovers. Journal of Regional Science, 59(3), 586–609. https://doi.org/10.1111/jors.12444
- Yu, Z., Zhang, H., Tao, Z., & Liang, J. (2019). Amenities, economic opportunities and patterns of migration at the city level in China. Asian and Pacific Migration Journal, 28(1), 3–27. https://doi.org/10.1177/0117196819832054
- Yue, W., Wang, T., Liu, Y., Zhang, Q., & Ye, X. (2019). Mismatch of morphological and functional polycentricity in Chinese cities: An evidence from land development and functional linkage. Land Use Policy, 88, 104176. https://doi.org/10.1016/j.landusepol.2019.104176