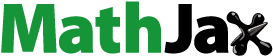
ABSTRACT
The digital transformation is an important driver of long-run productivity growth and, as such, it has the potential to promote a more inclusive and sustainable growth. However, digital capabilities, crucial to develop and govern new digital technologies, are unevenly distributed across European regions, increasing the risk of divergence and polarisation. By taking advantage of a set of original indicators capturing the level of digital skills in the regional workforce, this work analyses the factors shaping the process of digital skill accumulation in the EU over the period 2011–2018. Relying on transition probability matrices and dynamic random effects probit models, we provide evidence of a strong and persistent regional polarisation in the adoption and deployment of digital skills. Further, we investigate whether structural factors and European funds (European Regional Development Fund, Cohesion Funds and European Social Funds) are capable of shaping the digitalisation process and favouring regional convergence.
1. INTRODUCTION
Strengthening Europe's digital capabilities is the key to promote firms’ national and international competitiveness (Crespi et al., Citation2021; EC, Citation2022). In this context, the widespread diffusion of digital skills (DS) is of crucial importance to enable digital transformation on both the demand and supply sides. On the supply side, the development of digital technologies strongly relies on the absorptive capacity of companies, industries and regions, which is determined by the quantity and quality of skills the former are endowed with (D'Este et al., Citation2014; Fusillo et al., Citation2022). DS are also a key component of National and Regional Innovation Systems (NIS and RIS), playing a relevant role in the processes of regional diversification and structural change (Balland & Boschma, Citation2021; Castellacci et al., Citation2020; Santoalha et al., Citation2021). As for the demand side, consumption of digital goods and services often requires the availability of DS without which demand may not materialise.
The actual path of digitalisation across EU economies is highly heterogeneous due to the persistent structural polarisation between (i.e., core–periphery divide) and within (i.e., regional divide) countries (Celi et al., Citation2018; Citation2019; Citation2020). A polarisation that is likely to affect technological and skill capabilities alike, with core regions strengthening their relative position and peripheral ones falling behind. The EC has allocated to digitalisation about 20% of the Next Generation EU budget (150 billion euros) together with specific plans such as the ‘Digital Compass’ and the ‘European Chips Act’ that are to complement the long season of Cohesion Policy that, however, is not proving sufficient to halt the polarisation process (Gräbner et al., Citation2020).
The growing importance of DS in the economic policy domain is mirrored by the flourishing of empirical research focusing on their role in shaping economic performance as well as on factors that may facilitate/hamper their diffusion (Ciarli et al., Citation2021). The available evidence in this field is far from conclusive, though. As measuring digital competences is a complex task and reliable data sources are scarce, literature gaps abound and a number of relevant research questions still need to be addressed. First, DS are often measured relying on proxies that do not allow accounting for their degree of complexity (Castellacci et al., Citation2020). This means that elementary skills that may be enough for an individual to use simple digital devices are not properly distinguished from the more sophisticated ones such as, for example, those needed to design or develop new digital technologies (Santoalha et al., Citation2021). If this is the case, rough assessments of DS endowment are in order and relevant heterogeneities, in terms of digital performance, risk being overlooked. Second, there is a lack of evidence concerning the supply, demand and structural factors that can explain the diffusion of digital competences at the regional level and, no less relevantly, about those that may foster processes of convergence or divergence across regions (on this point, see Balland & Boschma, Citation2021). By the same token, there is no conclusive evidence regarding the role of policies aimed at strengthening regions’ knowledge and technological capabilities. This is particularly important in the European context as a significant share of the EU structural funds is directed at promoting regional convergence by strengthening local capabilities concerning digital knowledge and technologies (Crescenzi & Giua, Citation2020). However, EU funds are of different types – e.g., Cohesion funds, Social funds, Regional Development funds, etc. – and their ability to strengthen regional capabilities in terms of DS may vary according to their aim, size and characteristics. In this respect, fresh empirical evidence may be helpful to understand if and to what extent specific policy tools may be more capable than others to promote EU regions’ digital upgrading.
This work aims at filling some of the abovementioned literature gaps providing a number of empirical and theoretical contributions. First, a novel regional-level indicator providing a fine-grained measure of DS is proposed. This allows assessing the diffusion of DS accounting for their relative complexity, i.e., DS are distinguished between user, practitioner and developer in tune with Castellacci et al. (Citation2020). Second, convergence/divergence patterns are investigated, testing whether DS represent an additional driver of core–periphery polarisation across EU countries and regions (Celi et al., Citation2018). Third, the role of supply and demand determinants is investigated, assessing whether key elements characterising large-scale integration (LSI) – i.e., sectoral specialisation and share of high-tech industries, firm size and innovation intensity, labour market characteristics and share of high skilled workers – may contribute to explaining the diffusion of DS at the regional level (Caravella et al., Citation2021). Fourth, we provide evidence concerning the role of different EU funds (European Cohesion Fund (ECF), European Social Fund (ESF), European Regional Development Fund (ERDF)) in promoting, all other things being equal, the regional diffusion of DS. From a theoretical point of view, we add to two major literature streams. The first one regards the analysis of structural convergence/divergence in Europe. While a number of contributions have provided evidence on both causes and consequences of cross-country polarisation (see, for example, Gräbner et al., Citation2020; Reveiu et al., Citation2022), few studies have concentrated their attention on digital competences as an additional factor shaping such asymmetric dynamics. Nonetheless, DS represent a fundamental component of countries’ and regions’ technological capabilities and their uneven diffusion may further deepen polarisation. The second stream of literature concerns studies assessing the role of EU funds as tools capable of strengthening weaker areas and promoting convergence (see, among others, Di Caro & Fratesi, Citation2022). Thanks to the availability of rich data regarding both DS as well as EU funds, we are able to explore this relationship, accounting for key structural heterogeneities characterising European regions.
The article is organised as follows. Section 2 reviews the relevant literature and spells out the key research questions. Section 3 illustrates the data used for the analysis and reports a detailed mapping of the regional distribution of DS. Section 4 describes the econometric strategy and reports the results while Section 5 concludes, discussing the main policy implications.
2. DIGITAL SKILLS ACROSS EUROPE: PREVIOUS EMPIRICAL EVIDENCE AND NEW RESEARCH QUESTIONS
In what follows, we review the literature analysing diffusion and economic implications of DS focusing on three main streams of contributions on which our research questions (RQs) are built up. The first stream of literature adopts a rather broad perspective focusing on the so-called ‘digital divide’ – i.e., the gap between high and low DS, which can divide citizens, students, workers, rural and urban areas, regions and economies – investigating its causes and discussing the effectiveness of policies aimed at reducing it (Helsper & Van Deursen, Citation2015; Hidalgo et al., Citation2020; Van Dijk, Citation2008; Zillien & Marr, Citation2013). The key argument here is that the opportunities for growth, innovation and development of economies are inversely related to the relative intensity of the digital (territorial) divide affecting them (Thonipara et al., Citation2020). Indeed, the asymmetric distribution of DS among countries and territories may be explained by geo-demographic characteristics such as population density or distance from large cities; socio-economic factors such as gender, age, skill level, income inequalities; and structural elements such as firm size, innovation intensity, quality of infrastructures (OECD, Citation2021). Considering the multidimensional nature of digital divides, the OECD suggests implementing coordinated policies aimed at strengthening, simultaneously, structural capabilities (e.g., broadband connectivity) as well as end-user capabilities. Along similar lines, Lucendo-Monedero et al. (Citation2019) highlight that broadband access plays a crucial role in explaining the digital divide both for individuals and households. However, the availability of digital infrastructure may not be enough to bridge the gap (Crespo Cuaresma & Lutz, Citation2021). Analysing a panel of European regions, Szeles (Citation2018) shows how the digital divide is, to a large extent, explained by differences in terms of economic growth, educational attainment and R&D expenditure. Relatedly, the empirical literature focusing on the diffusion of DS and technologies across regions agrees that, although some forms of convergence in socio-economic factors associated with the access to digital technologies occurred over time, the divide has declined in relative but not in absolute terms (Kathuria & Oh, Citation2018), and this is not expected to disappear in the absence of an adequate policy mix (Crespo Cuaresma & Lutz, Citation2021). In other words, in the presence of persistent asymmetries in terms of socio-economic, technological and productive capabilities, digitalisation may deepen cross-country and territorial divides regardless of the provision of adequate infrastructure, such as high-speed broadband. Among the policies capable of reducing the digital divide, in turn, those related to the implementation of the regional ‘smart specialisation strategies’ appear to be particularly relevant. In this context, Castellacci et al. (Citation2020) identify DS as key enablers for access to advanced external knowledge and information, enabling the emergence and enhancement of sectors closer to ICT production and driving regional diversification processes.
Further evidence on digital polarisation patterns is provided by Capello et al. (Citation2022), who adopt a spatial approach to assess the way in which the ‘digital service economy’ is spreading (heterogeneously) across EU regions. They detect a strong divide between the most industrialised regions, where digital value creation models are more pervasive, mobilising significant amounts of investments and skills, and less developed ones, where digitalisation seems to spread more slowly and without the generation of relevant capabilities. Complementary evidence is provided by Reveiu et al. (Citation2022), showing that EU regions characterised by strong digital endowments are significantly more resilient vis-à-vis external shocks and crises. More generally, the importance of including the geographical dimension has been highlighted by Billon et al. (Citation2008, Citation2009) suggesting the explicit consideration of the role of spatial spillover of digitalisation that leads to identifying high adoption regions in the geographical centre of Europe. Also, Lutz (Citation2019) provides evidence on the existence of a north–south polarisation, with the northern European regions consistently presenting more widespread adoption of digital technologies than southern and eastern ones.
Interestingly enough, previous literature lacks an explicit exploration of the role of DS in shaping LIS and RIS. In this respect, Rodríguez-Pose and Crescenzi (Citation2008) and, more recently, Fernandes et al. (Citation2021) offer some hints by emphasising how the complex interaction between local and external knowledge, on the one hand, with local and external socioeconomic and institutional conditions and, on the other, can shape the innovation capacity of each region (Antonelli et al., Citation2022; Antonelli & Colombelli, Citation2018). In particular, the uneven spatial distribution of innovation and skills is at the centre of the analyses by Crescenzi et al. (Citation2020) and Kemeny et al. (Citation2022). Focusing on agglomeration patterns and inequalities, these authors highlight a generalised process of polarisation according to which innovation opportunities are concentrated in key hubs benefiting from self-reinforcing mechanisms that shield their privileged position. In such a context, skills are polarised too. Key hubs and ‘affluent places’ hosting the major educational and research institutions which, in turn, act as a ‘magnet’ attracting large inflows of high-skilled students and workers. A similar result is found by Bloom et al. (Citation2020) who have explored the geographical development of new technologies in the US. The authors highlight that, initially, technologies are concentrated in local hubs but, over time, their adoption diffuses geographically. However, despite this process of diffusion, the initial hubs retain a disproportionate share of high-skill employment. These technology hubs are more likely to arise in areas with universities and high-skilled labour pools, therefore reinforcing territorial inequalities.
This evidence suggests that given their tacit, local and cumulative nature (Dosi et al., Citation2000; Dosi & Marengo, Citation2015; Fanti et al., Citation2021) and the role of structural and institutional heterogeneities in shaping their diffusion (Castellacci et al., Citation2020), DS not only are likely to be unevenly distributed across regions, but such differences might be persistent. Stronger regions that first accumulate tacit knowledge, digital competences, capabilities and related innovative capacity are likely to increase that advantage vis-a-vis latecomers. Building on these considerations, the empirical analysis will focus on the identification of eventual persistence effects in the regional distribution of DS, thus providing evidence about our first RQ that can be spelled out as follows:
RQ1. Is there a process of structural divergence and polarisation across EU regions as regards the diffusion of DS?
Finally, the role of demand factors and structural peculiarities of sectors in shaping digitalisation dynamics across some key European economies has been recently analysed by Reljic et al. (Citation2021). Testing the impact of digital technologies on European employment and distinguishing between different professional categories they found that higher digitalisation, in terms of digital capital deepening, is associated with job creation in the case of managers, with a reduction in the employment of clerks whose numbers, on the other hand, increase with greater digital intermediate inputs. From this point of view, the role of sectoral heterogeneities in job creation/destruction has been clearly highlighted by Dosi et al. (Citation2021) who have distinguished the alternative effects on employment dynamics of embodied vs disembodied technological change. According to the authors, upstream sectors performing R&D activity are more likely to experience job growth, whereas in the downstream sector, whereby capital-goods bought from the upstream sector are employed for the production, labour-saving effects are more likely to emerge. Therefore, the sectoral structure of regions may shape both employment dynamics as well as the diffusion of skills. The prevalence of science-based and specialised supplier industries (upstream sectors) more likely correlates with higher skills and job creation, whereas regional economies grounded on scale-intensive, information-intensive or supplier dominated industries (downstream sectors) experience job destruction effects specifically in low-skill jobs affected by process technologies.
Hence, the second research question (RQ2) to be addressed in this paper concerns the role of key supply, demand and structural factors that may shape the regional diffusion of DS. In particular, we test whether areas characterised by large shares of high-tech and knowledge-intensive productions, a significant number of big firms or by a relatively larger number of patents per capita, all things being equal, are also more abundant in terms of DS. The overall economic strength of EU regions, in turn, is accounted for by including value added per employee. Here, the theoretical expectation is quite straightforward: structurally stronger regions are expected to display an equally strong DS endowment, in line with the arguments put forth by Crescenzi and Giua (Citation2020) and Caravella et al. (Citation2021). The second set of factors relates to region-specific labour market characteristics: relative shares of young, tertiary educated, permanent and non-EU workers are accounted for. In this case, regions characterised by a young, well-educated and stable labour force are expected to be in a better position to develop and accumulate DS (Kleinknecht, Citation2020). With no clear ex-ante expectation about the relative importance of each factor, RQ2 is thus formulated as follows:
RQ2. What are the structural factors contributing the most to the diffusion of DS across EU regions?
With a more granular spatial detail (NUTS3), Fratesi and Perucca (Citation2014; Citation2019) emphasise the importance of ‘territorial capital assets’, such as high-value functions in economic activities and human capital, in enabling the growth impact of cohesion policies, pointing out that their effectiveness is greater when investments are focused on assets that are complementary to those already present in the region. For Rodríguez-Pose and Garcilazo (Citation2015), a key mediator of the efficiency of EU spending on regional economic growth is the high quality of local and regional governments, linked to a high degree of accountability and transparency, with well-trained staff and led by trustworthy politicians who are aware of the interests of local communities. In line with previous literature, the recent analysis by Di Caro and Fratesi (Citation2022) shows that the heterogeneous effects of cohesion policies may be related to the presence of a number of national and regional contextual factors, including the level of national development, the quality of regional institutions and the endowment of regional human capital. Analysing the Italian case, Giua (Citation2017) reports a positive employment impact of Cohesion Funds in those southern regions where sectors such as manufacturing, construction, retail trade and tourism play a relevant role. More recently, Crescenzi and Giua (Citation2020) provided new evidence concerning the spatial concentration of the economic and employment effect of EU cohesion policy.
While broadly reporting a positive impact of EU cohesion and regional funds,Footnote1 the empirical literature points to a highly uneven territorial distribution of their effects. Such heterogeneity is related to the uneven distribution of key intervening factors (Brandsma & Kancs, Citation2015) – i.e., structural elements related to productive and technological capabilities, institutional quality, labour supply and skill endowment – and, as recently shown by Dicharry (Citation2023), by the similarly heterogeneous absorptive capacity of EU regions. To the best of our knowledge, the relationship between EU funds and regional performance in terms of digitalisation, including the diffusion of DS, is still poorly investigated. This represents a relevant knowledge gap, as DS may represent a key driver of regions’ technological and structural upgrading. On the other hand, the digital divide is contributing to deepening the divide between and within-country polarisation that has plagued Europe in recent years (Celi et al., Citation2022). Against this background, a better understanding of the relationships between different types of EU funds and DS accumulation at the regional level is an issue of high scientific and policy relevance. Therefore, such a literature gap paves the way to the third research question (RQ3) addressed in this paper. In particular, we distinguish between EU funds that are directed at: i) strengthening EU’s internal cohesion by supporting member states with a gross national income per capita below 90% EU-27 average, i.e., the EUCF; ii) supporting member states’ labour and social policies, including those directly related to the development of skills, i.e., the ESF; iii) correcting imbalances between the development levels of EU regions including two specific programmes aimed at supporting regional policies on research and digitalisation, i.e., the ERDF. An overall positive contribution of the abovementioned EU funds on the ability of regions to accumulate DS is expected.Footnote2 However, relevant heterogeneities are also likely to emerge. As a result, RQ3 is spelled out as follows:
RQ3. Do European structural and regional funds (EUCF, ESF and ERDF) contribute to the diffusion and accumulation of DS across EU regions?
3. DATA AND DESCRIPTIVE EVIDENCE
3.1. Data
The data used in this article stem from different sources: (i) the European Multilingual Classification of Skills, Competences, Qualifications and Occupations (ESCO); (ii) the European Labour Force Survey (EU-LFS); (iii) the European Regional Accounts (EU-REGIO); (iv) the OECD-REGPAT and (v) the historical EU payments database.
ESCO provides a multilingual classification of European skills, competences, qualifications and occupations. It allows the matching of occupational profiles to skills, competences and qualifications at a high level of disaggregation. The EU-LFS is the largest European household sample survey and provides labour market statistics for 35 participating countries over time. Individual data can be aggregated at the NUTS level since EU-LFS is stratified at the geographical level. The EU-REGIO maintained by Eurostat provide a regional breakdown for major aggregates, such as gross value added and household income. The OECD EPO-REGPAT is a comprehensive dataset providing all patent applications to the European Patent Office. We rely on inventors addresses to assign patents to all EU regions. Finally, the historical EU payments database reports regionalised annual EU expenditure data (in current prices then transformed to 2015 prices) for specific EU funds (ECF, ESF, ERDF).
Information from the above-mentioned sources have been merged at the NUTS2 level in order to have an integrated database at the European regional level covering 24 countries divided by 179 regions for 8 years (2011–2018).Footnote3 Table A2 in the Appendix in the supplemental data online shows the composition of the database.
3.2. Digital skills indicator
The DSIs (digital skill indicators) are computed to measure the level of digitalisation of the local workforce. The main source is the v.1.0 release of ESCO. For almost 3000 occupations (or job profiles), a detailed description of about 13,500 representative skills is provided. To measure the digital intensity of occupations, we applied a three step strategy. First, for each skill descriptor we identify the words potentially related to the use of digital technologies following the criterion by Chiarello et al. (Citation2021).Footnote4 We identify almost 1200 ‘digital skills’ (DSKILL). Each DSKILL is then assigned to a category depending on the degree of professional expertise involved:
user level (309 skills): requiring basic knowledge concerning how to use the technology for very specific purposes; no specialist expertise needed;
practitioner level (348 skills): requiring a certain degree of expertise to tailor technology and adapt it to the on use context;
developer level (546 skills): requiring significant expertise to design and modify technology.
As a second step, DSKILL are matched with each 4-digit ISCO occupation (429 individual occupations). Following Castellacci et al. (Citation2018), we then build the DSI for each 3-digit ISCO occupation relying on the following formula:
(1)
(1) where the value of the DSI indicator for each 3-digit ISCO category j is equal to the sum of DS assigned to the related 4-digit ISCO category y –
– weighted by the number of employees belonging to such occupations –
.
As a third step, we rely on (1) to derive a regional DS indicator. The following weighting procedure is carried out:
(2)
(2) where the regional
(2) –
– is equal to the weighted average of (1) using the ISCO 3-digit employment shares –
– as weights. The latter is our key measure to assess diffusion and determinants of DS at the EU regional-level.
3.3. Mapping DS
displays the diffusion of DS in the EU between the years 2011 and 2018. DS are highly heterogeneous across regions. This evidence is coherent with the relationship between digital divide and structural polarisation between European countries and regions documented in the literature and illustrated in Section 2. EU regions are in fact heterogeneous in terms of economic structures, labour market characteristics, education systems and sectoral compositions. DS turn out to be significantly more concentrated in northern EU regions, particularly Scandinavian ones, as compared to southern Europe. Within-country heterogeneity matters too, however. In fact, non-negligible parts of France, Germany and Italy display a remarkable intensity in terms of DS. Moreover, distinguishing between users, practitioners and developers allows the unravelling of additional evidence. Developers are relatively more concentrated in ‘affluent’ high-tech and service-oriented regions. Concerning practitioners, a greater heterogeneity is detected. Overall, regions where capitals and big cities are located tend to outperform inner zones. On the other hand, Germany, Finland, Sweden and the UK show a quite uniform diffusion of DS.
Figure 1. The DSIs across EU regions, annual average (2011–2018).
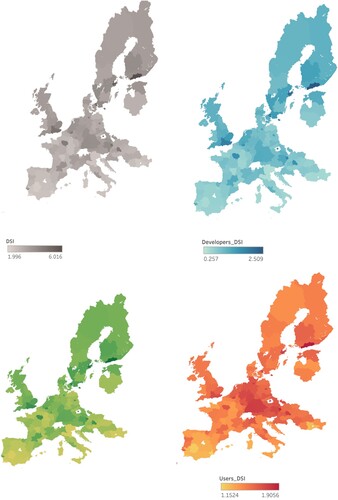
Do digital skills change over time? Can any convergence/divergence dynamics be detected? As a very preliminary answer to these questions, displays the association between DS indexes as measured in 2011 and changes over the 2011–2018 period. The scatter plot shows a slightly positive relationship between the two variables. Despite some ‘digital divergence’ seeming to emerge, no clear-cut pattern can be detected. Most regions are, in fact, located in the middle part of the scatter plot, displaying quite heterogeneous trajectories.
Figure 2. Changes in the DSI across regions and over time.
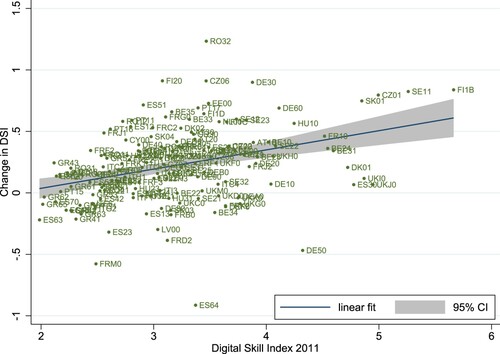
Indeed, the dispersion of the DSI () has increased over time with some regions moving to the right and recording higher concentrations of digital competencies while others seem to be stuck in the initial positions. Overall, although the distribution is moving to the right, suggesting a generalised DS upgrading in 2018 with respect to 2011, dispersion is also increasing, lending support to the polarisation hypothesis.Footnote5
3.4. Structural dynamics
The diffusion of DS is now further investigated, relying on transition probability matrices (TPM). This allows the capturing of the degree of convergence/divergence among regions and, no less relevantly, the relative persistence of their ‘digital status’.
We first briefly introduce the TPM methodology as applied to the DS case. Let’s consider and
as the events of being below and above the median value of DSI. The events could be approximated by a two-state Markov chain with transition probabilities:
(3)
(3) The corresponding AR (1) process for the stochastic variable
is the following:
where:
(4)
(4)
Each term of the TPM will be the conditional probability , or the probability of moving from state
to state
. Based on the estimated transition probabilities different situations are possible, in the case of a two-dimensional matrix:
Transient digitalisation: if the sum of the lead diagonal terms is less than 1 there is no evidence of persistence.
Weak digitalisation persistence: if the sum of the main diagonal terms is more than 1 but some of these terms are lower than 1/n (in this case 0.5).
Strong digitalisation persistence, if the sum of the main diagonal terms is more than 1 and all the main diagonal terms are larger than 1/n (in this case 0.5).
The following table reports the TPMs for both broad DSI as well as developers, practitioners and users. More specifically, the main diagonal informs on the overall rate of persistence, while the second diagonal provides information about the relative importance of barriers to entry and exit. Overall, we find evidence of strong digitalisation persistence as all terms of the main diagonal are larger than 0.5 and their sum is more than 1. This first evidence suggests the presence of intertemporal stability in the accumulation/diffusion of DS ().
Table 1. TPMs across groups of countries.
While digitalisation persistence seems to be widespread, some regions have greater chances to move from a low towards a high digitalisation path. This is the case of the ‘core’ countries: about 20% moved from below to above the DSI median over the considered time span. This percentage grows to 24% when it comes to the more complex developers’ skills.
Regions located in the north and Scandinavian EU countries perform even better. Conversely, only 4% of southern European regions have registered a transition over time: 5% for developers and 6% for users.
replicates the exercise focusing on regions distinguished between those classified by the EC as ‘target regions’ and receiving funds accordingly (during the 2007–2013 period); and those having no access to these funds. Upon such a very preliminary descriptive inspection, therefore, it seems that being a target of EU funds is not, as such, a guarantee of DS upgrading.Footnote6
Table 2. TPMs across groups of target regions (2007–2013).
4. EMPIRICAL STRATEGY AND RESULTS
We now explore the probability of regions to move from backwardness to digital upgrading through a multivariate approach controlling, simultaneously, for both structural factors and EU funds. We rely on a discrete choice panel data model based on the estimator proposed by Wooldridge (Citation2005) and applied, among others, by Peters (Citation2008) and Antonelli et al. (Citation2012). While TPMs provide a useful background picture concerning the relative persistence of regional DS patterns, the following analysis allows the identification of the actual influence of path-dependency, after controlling for relevant structural and policy drivers.
The dependent variable is regressed against its past realisation (t-1), its initial value and a set of relevant controls
including, for each region i, information regarding sectoral composition, average firm size, labour market characteristics and competences, technological capabilities. The dependent variable (DSKILL) is a dummy assuming value one in period t if a region is above the median of the DSI; and 0 otherwise. Our goal is to capture ‘true state dependence’ in terms of regions’ DS accumulation controlling, as much as possible, for endogeneity sources related to observable and unobservable heterogeneity. In particular, endogeneity may arise due to the correlation between regions’ DS endowments – proxied by DSI at
– and unobservable elements which may explain long-term structural differences concerning regions’ economic, technological and skill-related dynamism. To partly solve this problem, we rely on the procedure proposed by Wooldridge (Citation2005), specifying the distribution of the unobserved component
conditional on DSI at
and on the region-specific time average of the controls included in
. In other words, we use the first ‘realisation’ of the DS indicator
and the time-averaged covariates
as predictors of the individual (regional) effect. The estimated model can thus be written as follows:
(5)
(5) where for each region i and year t the dummy
is regressed against its initial value
, its first lag
, the matrix of covariates
and their region-specific time average
. The matrix X includes a first set of structural variables related to the region’s productive structure: (i) the share of employees in high-tech and knowledge intensive services as defined by OECD; (ii) the share of employees in large and small companies. A second set of variables has to do with workforce composition and job characteristics: (iii) the share of employees with tertiary education; (iv) the share of young workers; (v) the share of workers having permanent contracts; (vi) the share of non-EU workers. A third set of variables, in turn, refers to technological and macroeconomic characteristics proxied by: vii) the number of patents per habitant and viii) the value added per employee (in logarithm). Finally, a specific test is run including three variables related to EU funds (see Table 5A for descriptive statistics of variables included in the analysis): ix) EUCF, ESF and ERDF. Notice that all models have been estimated clustering standard errors at the regional level.
4.1. Results
shows the results for different specifications of the model regarding the general DSI (column 1) as well as the specific indicators for developers (column 2), practitioners (column 3) and users (column 4). Results support the thesis of high persistence of digital skills since, even after controlling for all the structural factors included in X, the probability of observing an upgrading in digital skills (that is, a transition from below-the-median to above-the-median DSI) in period t is still positively and significantly affected by its previous realisation. In other words, having high digital capabilities in the previous period is, all things being equal, a strong and significant predictor of the current DS status. Initial conditions matter too.Footnote7 The coefficient associated with the initial value of DSI is in fact positively and significantly correlated to the dependent variable, across all specifications. However, when we run the analysis distinguishing between users, practitioners and developers, interesting differences arise. In particular, while persistence is detected with respect to users and digital developers’ skills, the opposite holds for practitioners. This result is probably influenced by the peculiar distribution of regions in this specific indicator with respect to the other two cases. As shown in Figures 1A, 2A and 3A, the distribution of regions for practitioners’ DS is less dispersed and more concentrated around the mean, implying that state changes are more probable for this category than for the others.
Table 3. Marginal effects of dynamic random effects probit model with unobserved heterogeneity.
Overall, these results provide a positive answer to our RQ1. The diffusion of DS across EU regions turns out to be characterised by persistence effects leading to divergence and polarisation mechanisms. A potential explanation may point to the well-known path-dependent nature of digital technologies and competences as well as to those largely documented between and within-country structural polarisation affecting the European economy. A polarisation that indeed emerges also looking at DS types, particularly concerning users and developers’ skills. Regions located at the top and bottom of the conditional DSI distribution are in fact very much likely to keep their status despite the number of economic, technological and labour market heterogeneities that are controlled for.
The inclusion of a large set of controls allows testing of the robustness of the relationships identified between past and current realisation of the digital skill indicators. Turning, therefore, to our RQ2 we can assess that, among most important factors, the share of employees in high-tech manufacturing and knowledge intensive services plays a crucial role, except for digital users, which is not surprising. Regions where most of the high-tech manufacturing and knowledge intensive activities are concentrated are also likely to show a relatively more intense demand for practitioners and developers’ DS. Basic DS, such as those related to the use of common digital artifacts, are in turn more widespread across sectors and regions. This evidence is coherent with the polarisation pattern, documented in Section 4, between core and northern EU regions, where most of the high-tech manufacturing and services are concentrated.
Similar arguments may apply concerning the positive and significant role of firm size. Since Schumpeter, it is common knowledge that large firms are the loci where high skills tend to be accumulated and DS are no exception. Several research papers exploring firm-level data (Calvino et al., Citation2022; Müller et al., Citation2021) have highlighted that large companies have a significantly higher probability to adopt digital technologies due to their relatively stronger absorptive capacity. Our results support this view, highlighting that regions where there is a higher concentration of large companies are also favoured in terms of DS accumulation, particularly when it comes to developers and practitioners’ skills.
Regarding the accumulation of skills, including digital ones, education is of course the most obvious predictor. This is confirmed by our estimations: regions showing a relatively higher share of tertiary educated workers are also better positioned in terms of DS, in particular with regard to developers and practitioners. Indeed, the possibility to fully exploit the potential of digital technologies, for instance in terms of labour productivity, depends among other factors on the availability of specific skills and competences usually associated with tertiary education.
Finally, demand seems to matter too, a higher value added per employee is also a strong predictor of DS accumulation with respect to the broad indicator. When specific digital profiles are distinguished, it turns out that the ‘demand-pull’ effect plays a role only in the case of practitioners while no significance is detected concerning users and developers.
Hence, the empirical evidence so far explored emphasises three main key factors that are likely to shape EU regions’ dynamics in terms of DS: (i) concentration of large and high-tech/knowledge intensive businesses; (ii) presence of a qualified workforce able to virtuously interact with digital technologies; (iii) a sustained aggregate demand. This suggests that the diffusion of DS in European regions follows a process which is both past and path dependent. As previously highlighted, past conditions are relevant and robust and tend to favour regional divergence and polarisation processes. However, contingent factors are able to affect the direction and the dynamics of the digitalisation path followed by regions, suggesting that some form of convergence can be attained when proper actions are put in place over time.
In this respect, it appears to be of interest investigating how European structural and regional funds may shape the diffusion and accumulation of DS (RQ3). In order to provide an answer to this question, we enrich the empirical model specified in (5) by including three variables proxying the amount spent per inhabitant by three specific European funds (EUCF, ESF and ERDF).
It is worth recalling that the EUCF targets only the weakest member states, i.e., those whose gross national income per inhabitant is less than 90% of the EU average. It aims to reduce economic and social disparities and to promote sustainable development. The ESF supports investments to promote employment, human capital and social cohesion. Finally, the ERDF focuses its investments on key priority areas such as innovation and research, the digital agenda; it provides support for small and medium-sized enterprises and the low-carbon economy.
clearly shows that the inclusion of the three funds does not alter the main relationships already sketched, overall confirming the presence of persistence patterns across regions in terms of relative DS intensity. When looking at the broad DSI indicator, results show that only regions benefitting from the EUCF tend to benefit in terms of higher use of digital capabilities. However, both coefficients associated with the ESF and ERDF do not show any statistically significant effect. This result, although it does not represent robust evidence of the existence of a causal nexus between the considered variables, offers interesting insights that can be further scrutinised in more dedicated analyses. It is worth underlining that the EUCF turns out to be the more significant form of support for regions trying to pursue a DS upgrading. While other funds might have a positive effect on the overall process of digitalisation of EU regions, they appear to be not particularly effective in shaping a process of convergence between core and periphery regions in terms of DS accumulation. This is even more clear when the specific DS categories of digital skills are analysed. Results show that the statistical significance of the EUCF variable is mainly driven by its effect on the group of users, which sounds reasonable as it attains to the more basic domain of digital skills, on which the weakest regions can more easily realise a catch-up process. Moreover, while for the group of users, the coefficient relative to the ESF turns out to be (barely) positively significant, the one associated with the ERDF is found to be negative and statistically significant. A possible interpretation of this outcome is, on the one hand, that regions receiving a greater amount per capita of ESF, were able to benefit off dedicated resources on skills and human capital which is a main target of this type of support programme. On the other, the greater concentration (and effective use) of resources on key areas, including innovation and digital transformation, which is required by law for the most developed regions with respect to less advanced ones, might have exerted a divergent rather than a convergent effect. This might explain why those weakest regions receiving a greater level of ERDF per capita increase their relative distance in terms of users of digital skills with respect to the most advanced regions.
Table 4. Marginal effects of dynamic random effects probit model with unobserved heterogeneity.
5. CONCLUSIONS
This study provides new empirical evidence on regional patterns of digitalisation in Europe during the decade 2011–2018. In particular, the analysis focused on the distribution of DS across EU regions, by developing a regional digital skill index starting from the ESCO taxonomy. The DSI is a synthetic measure of the local DS endowment of EU regions and basically reflects the occupational composition of regions based on the degree of digitalisation knitted within the employed workforce. The paper offers a rich set of descriptive evidence based on the regional distribution of the DSI, as well as an econometric analysis which allowed us to appreciate the actual role of structural elements and EU policies in shaping the path of regional digital upgrading while controlling for the past dependency of such phenomenon. Furthermore, we investigated the link between EU policies and the intensity of use of digital skills. In particular, we found a positive correlation between EUCF and superior regional DSI performance. Similarly, the ESF turns out to be an effective lever of the digital upgrade, but statistically weaker if compared to the EUCF. Both, EUCF and ESF, are positively related to the diffusion of ‘digital-friendly’ occupations regarding basic and elementary digital skills. This result is coherent with their objectives to support employment, help people find better jobs and ensure more equal employment opportunities, paying particular attention to the less skilled workforce, such as young people and the long-term unemployed (Colnot and Pellegrin, Citation2019). However, we detect a negative and statistically significant link between the ERDF and the above median DSI-users indicator, suggesting that the greater concentration and effective use of resources on innovation and digitalisation strategies for the most developed regions with respect to less advanced ones, might have exerted a divergent rather than a convergent effect.
Overall, the analysis suggests that regional convergence, also with respect to digital performance, is still a goal far from being achieved. Undoubtedly, the pandemic has accelerated the digitalisation process across regions but the digital polarisation between and within countries remains. However, the proposed analysis offers some indication on the direction to be pursued in order to reverse the polarisation trend and open up a path of (digital) rebalancing across regions. In particular, policies promoting the higher education sector and the local availability of digital competences, as well as structural changes enabling the local development of knowledge-intensive sectors, favouring the growth of firms’ dimension and improving infrastructural conditions, appear to be relevant (Ciarli et al., Citation2021).
In this direction, the national Recovery and Resilience Plans (RRPs) represent the main policy tool to achieve the digital transition, with almost 26% of the spending allocated, to date, to the digital transition. Based on the EU’s broadband strategy to lead the ‘Gigabit society’ by 2025, many RRPs provide actions to ensure the right environment and conditions for the deployment of advanced digital – very high-capacity – networks (i.e., Gigabit connectivity, 5G coverage, Internet connectivity of at least 100 Mbps) among key adopters (i.e., schools, hospitals, public administrations, terrestrial transport path, enterprises and households) and rural and remote areas. This is a pre-condition to close the digital gaps across Europe, but complementary policy actions have to be launched to effectively achieve this goal. For instance, policy support should positively influence the demand-side of digital adoption, especially regarding firms. In this respect we notice that many industrial plans implemented in the context of RRPs are based on individual fiscal benefit to promote digital investments in the framework of Industry 4.0. Based on this mechanism, the territorial distribution of public resources follows a spontaneous path that basically mirrors the spatial location of the productive base. This means that less developed areas, such as the Italian Mezzogiorno and other peripheral areas of Europe, are not provided with the right conditions, in both quantitative and qualitative terms, to maximise the potential of the digital transformation. This implies that, in these areas, firms are, on average, weaker concerning their economic, technological and organisational profiles. These conditions, taken together, hamper the uptake of digital solutions by the private sector, slowing down the overall digital performance of the region. To remove these barriers, industrial policy measures aimed at enlarging and enhancing the local productive base should complement the actions on broadband infrastructures. With specific regard to the local workforce, less developed or declining areas often give rise to young and, not infrequently, highly skilled workers who move to richer regions or countries to find better job opportunities (Ciarli et al., Citation2021). In this context, increasing the demand for advanced (digital) skills to be employed in local public and private organisations would mitigate the migration process and favour the digital upgrading, with an increase in aggregate competitiveness and an improvement in the quality of both private initiatives and public services. This must go hand in hand with a more systemic and holistic logic, in which LIS (or RIS) can perform as ‘digital hubs’ where advanced industries and knowledge-intensive services interact with higher education institutions and qualified public administrations.
Supplemental Material
Download PDF (339.1 KB)ACKNOWLEDGEMENTS
Francesco Crespi acknowledges the support from the Italian Ministry of University and Research. Scientific Research Program of National Relevance (PRIN) 2017, project ‘Innovation for global challenges in a connected world: the role of local resources and socioeconomic conditions’. Valeria Cirillo acknowledges the support of the Italian National Research Project - PRIN 2017 ‘Regional Policies, Institutions and Cohesion in the South of Italy’ (Project code 2017-4BE543), financed by the Italian Ministry of Education, University and Scientific Research from 2020 to 2023. She also acknowledges the support of the European Union's Horizon Europe Research and Innovation programme under grant agreement No 101069651 (BRIDGES 5.0). Views and opinions expressed are however those of the author only and do not necessarily reflect those of the European Union. Neither the European Union nor HADEA can be held responsible for them.
Earlier working paper version: an earlier version of this manuscript has been published as Sapienza University of Rome - Department of Economics and Law working paper. The permanent link is: https://ideas.repec.org/p/sap/wpaper/wp227.html
DISCLOSURE STATEMENT
No potential conflict of interest was reported by the author(s).
Additional information
Funding
Notes
1 According to Ehrlich and Overman (Citation2020) the economic and employment impact of cohesion and regional funds remains an open empirical question, as the available evidence is characterised by a significant degree of heterogeneity. This is partly due to the heterogenous quality of the adopted data sources as well as to the number of econometric approaches the different contributions rely on (Dicharry et al., Citation2019).
2 A number of studies have focused on the impact of EU funds on regional growth and convergence (among others, Crescenzi, Citation2009; Maynou et al., Citation2016; Psycharis et al., Citation2020). To the best of our knowledge, however, no contribution has yet focused on the relationship between EU funds and regions’ DS endowment.
3 As compared to the index proposed by Castellacci et al. (Citation2018), our DS indicator is based on a broader set of skills (nearly 1200 skills reported in the ESCO database instead of 69). This allows capturing, in a more detailed way, of the level of complexity characterising DS, e.g., DS associated to the implementation of elementary (digital) activities or those related to complex activities like those associated to the development of new technologies.
4 We improve the set of words proposed by Chiarello et al. (Citation2021) relying on synonyms, alternative expressions, roots and acronyms ending up with 75 items (Table 1 in Appendix). Notice that to build our occupation-level indicator we considered the absolute number of digital skills rather than the relative one (i.e., the ratio of DS over the total number of skills describing an occupation). By considering a relative measure, in fact, we would have risked underestimating the actual ‘digital content’ of more complex occupations characterised by a relatively larger number of skills.
5 In the Appendix in the supplemental data online, Figures A1, A2 and A3 show digital distributions for each specific indicator.
6 In the Appendix in the supplemental data online, Tables A3, A4 and A5 show TPMs by terciles, at the top 15% and 25% of the DSI distributions.
7 This evidence is coherent with what Cicerone et al. (Citation2022) found concerning the relationship between AI knowledge and green tech specialisation of regions. The authors show that AI knowledge may help in promoting the green-tech specialisation of regions, provided that they were already green-tech specialised in the past.
REFERENCES
- Antonelli, C., & Colombelli, A. (2018). External and internal knowledge in the knowledge generation function. In C. Antonelli (Ed.), The evolutionary complexity of endogenous innovation (pp. 82–108). Elgar.
- Antonelli, C., Crespi, F., & Quatraro, F. (2022). Knowledge complexity and the mechanisms of knowledge generation and exploitation: The European evidence. Research Policy, 51(8), 104081. https://doi.org/10.1016/j.respol.2020.104081
- Antonelli, C., Crespi, F., & Scellato, G. (2012). Inside innovation persistence: New evidence from Italian micro-data. Structural Change and Economic Dynamics, 23(4), 341–353. https://doi.org/10.1016/j.strueco.2012.03.002
- Balland, P. A., & Boschma, R. (2021). Mapping the potentials of regions in Europe to contribute to new knowledge production in industry 4.0 technologies. Regional Studies, 55(10-11), 1652–1666. https://doi.org/10.1080/00343404.2021.1900557
- Beugelsdijk, M., & Eijffinger, S. C. (2005). The effectiveness of structural policy in the European union: An empirical analysis for the EU-15 in 1995–2001. Journal of Common Market Studies, 43(1), 37–51. https://doi.org/10.1111/j.0021-9886.2005.00545.x
- Billon, M., Ezcurra, R., & Lera-Lopez, F. (2008). The spatial distribution of the internet in the European union: Does geographical proximity matter? European Planning Studies, 16(1), 119–142. https://doi.org/10.1080/09654310701748009
- Billon, M., Ezcurra, R., & Lera-Lopez, F. (2009). Spatial effects in website adoption by firms in European regions. Growth and Change, 40(1), 54–84. https://doi.org/10.1111/j.1468-2257.2008.00461.x
- Bloom, N., Hassan, T. A., Kalyani, A., Lerner, J., & Tahoun, A. (2020). In: The geography of new technologies. Institute for New Economic Thinking, Working Paper No. 126 (downloaded from. https://www.ineteconomics.org/uploads/papers/WP_126-Tahoun-et-al.pdf.
- Brandsma, A., & Kancs, D. A. (2015). RHOMOLO: A dynamic general equilibrium modelling approach to the evaluation of the European union's R&D policies. Regional Studies, 49(8), 1340–1359. https://doi.org/10.1080/00343404.2015.1034665
- Calvino, F., De Santis, S., Desnoyers-James, I., Formai, S., Goretti, I., Lombardi, S., Manaresi, F., & Perani, G. (2022). Closing the Italian digital gap: The role of skills, intangibles and policies, OECD Report.
- Capello, R., Lenzi, C., & Panzera, E. (2022). The rise of the digital service economy in European regions. Industry and Innovation, 30(6), 637–663.
- Cappelen, A., Castellacci, F., Fagerberg, J., & Verspagen, B. (2003). The impact of EU regional support on growth and convergence in the European union. Journal of Common Market Studies, 41(4), 621–644. https://doi.org/10.1111/1468-5965.00438
- Caravella, S., Crespi, F., Guarascio, D., & Tubiana, M. (2021). Heterogeneity in the demand-growth relationship at the firm level: The role of demand sources and innovation/knowledge characteristics. Economics of Innovation and New Technology, 30(5), 516–535. https://doi.org/10.1080/10438599.2020.1853013
- Castellacci, F., Consoli, D., & Santoalha, A. (2018). Technological Diversification in European Regions: The Role of E-Skills (No. 20181009). Centre for Technology, Innovation and Culture, University of Oslo.
- Castellacci, F., Consoli, D., & Santoalha, A. (2020). The role of e-skills in technological diversification in European regions. Regional Studies, 54(8), 1123–1135. https://doi.org/10.1080/00343404.2019.1681585
- Celi, G., Ginzburg, A., Guarascio, D., & Simonazzi, A. (2018). Crisis in the European monetary union. Oxon: Routledge.
- Celi, G., Guarascio, D., Reljic, J., Simonazzi, A., & Zezza, F. (2022). The asymmetric impact of war: Resilience, vulnerability and implications for EU policy. Intereconomics, 57(3), 141–147.
- Celi, G., Guarascio, D., & Simonazzi, A. (2019). Unravelling the roots of the EMU crisis. Structural divides, uneven recoveries and possible ways out. Intereconomics, 54(1), 23–30. https://doi.org/10.1007/s10272-019-0786-3
- Celi, G., Guarascio, D., & Simonazzi, A. (2020). A fragile and divided European union meets COVID-19: Further disintegration or ‘Hamiltonian moment’? Journal of Industrial and Business Economics, 47(3), 411–424. https://doi.org/10.1007/s40812-020-00165-8
- Chiarello, F., Fantoni, G., Hogarth, T., Giordano, V., Baltina, L., & Spada, I. (2021). Towards ESCO 4.0–Is the European classification of skills in line with Industry 4.0? A text mining approach. Technological Forecasting and Social Change, 173, 121177.
- Ciarli, T., Kenney, M., Massini, S., & Piscitello, L. (2021). Digital technologies, innovation, and skills: Emerging trajectories and challenges. Research Policy, 50(7), 104289. https://doi.org/10.1016/j.respol.2021.104289
- Cicerone, G., Faggian, A., Montresor, S., & Rentocchini, F. (2022). Regional artificial intelligence and the geography of environmental technologies: Does local AI knowledge help regional green-tech specialization? Regional Studies, 57(2), 330–343.
- Colnot, L., & Pellegrin, J. (2019). The contribution of cohesion policy to digitalisation: An adequate approach? Centre for Industrial Studies (CSIL), Milan. Working Paper, (01).
- Crescenzi, R. (2009). Undermining the principle of concentration? European union regional policy and the socio-economic disadvantage of European regions. Regional Studies, 43(1), 111–133. https://doi.org/10.1080/00343400801932276
- Crescenzi, R., & Giua, M. (2020). One or many cohesion policies of the European union? On the differential economic impacts of cohesion policy across member states. Regional Studies, 54(1), 10–20. https://doi.org/10.1080/00343404.2019.1665174
- Crespi, F., Caravella, S., Menghini, M., & Salvatori, C. (2021). European technological sovereignty: An emerging framework for policy strategy. Intereconomics, 56(6), 348–354. https://doi.org/10.1007/s10272-021-1013-6
- Crespo Cuaresma, J., & Lutz, S. U. (2021). Modelling and projecting digital trends in European regions: An econometric framework. Regional Studies, 55(10-11), 1696–1710. https://doi.org/10.1080/00343404.2021.1976746
- D'Este, P., Rentocchini, F., & Vega Jurado, J. M. (2014). The role of human capital in lowering the barriers to engaging in innovation: Evidence from the spanish innovation survey. Industry and Innovation, 21(1), 1–19. https://doi.org/10.1080/13662716.2014.879252
- Di Caro, P., & Fratesi, U. (2022). One policy, different effects: Estimating the region-specific impacts of EU cohesion policy. Journal of Regional Science, 62(1), 307–330. https://doi.org/10.1111/jors.12566
- Dicharry, B. (2023). Regional growth and absorption speed of EU funds: When time isn’t money. Regional Studies, 57(3), 511–524. https://doi.org/10.1080/00343404.2022.2092611
- Dicharry, B., Nguyen-Van, P., & Pham, T. K. C. (2019). “The winner takes it all” or a story of the optimal allocation of the European cohesion fund. European Journal of Political Economy, 59, 385–399. https://doi.org/10.1016/j.ejpoleco.2019.05.003
- Dosi, G., & Marengo, L. (2015). The dynamics of organizational structures and performances under diverging distributions of knowledge and different power structures. Journal of Institutional Economics, 11(3), 535–559. https://doi.org/10.1017/S1744137414000204
- Dosi, G., Nelson, R. R., Winter, S. G., & Winter, S. G. (Eds.). (2000). The nature and dynamics of organizational capabilities. Oxford university Press.
- Dosi, G., Piva, M., Virgillito, M. E., & Vivarelli, M. (2021). Embodied and disembodied technological change: The sectoral patterns of job-creation and job-destruction. Research Policy, 50(4), 104199. https://doi.org/10.1016/j.respol.2021.104199
- Ehrlich, M. V., & Overman, H. G. (2020). Place-based policies and spatial disparities across European cities. Journal of Economic Perspectives, 34(3), 128–149. https://doi.org/10.1257/jep.34.3.128
- Esposti, R., & Bussoletti, S. (2008). Impact of objective 1 funds on regional growth convergence in the European union: A panel-data approach. Regional Studies, 42(2), 159–173. https://doi.org/10.1080/00343400601142753
- European Commission. (2022). European Commission digital strategy, C(2022) 4388 final, Bruxelles.
- Falck, O., Heimisch-Roecker, A., & Wiederhold, S. (2021). Returns to ICT skills. Research Policy, 50(7), 104064. https://doi.org/10.1016/j.respol.2020.104064
- Fanti, L., Guarascio, D., & Tubiana, M. (2021). Skill mismatch and the dynamics of Italian companies’ productivity. Applied Economics, 53(59), 6790–6803. https://doi.org/10.1080/00036846.2021.1948963
- Fernandes, C., Farinha, L., Ferreira, J. J., Asheim, B., & Rutten, R. (2021). Regional innovation systems: What can we learn from 25 years of scientific achievements? Regional Studies, 55(3), 377–389. https://doi.org/10.1080/00343404.2020.1782878
- Fratesi, U., & Perucca, G. (2014). Territorial capital and the effectiveness of cohesion policies: An assessment for CEE regions. Investigaciones Regionales, 29, 165–191.
- Fratesi, U., & Perucca, G. (2019). EU regional development policy and territorial capital: A systemic approach. Papers in Regional Science, 98(1), 265–281. https://doi.org/10.1111/pirs.12360
- Fusillo, F., Consoli, D., & Quatraro, F. (2022). Resilience, skill endowment, and diversity: Evidence from US metropolitan areas. Economic Geography, 98(2), 170–196. https://doi.org/10.1080/00130095.2021.2008797
- Giua, M. (2017). Spatial discontinuity for the impact assessment of the EU regional policy: The case of Italian objective 1 regions. Journal of Regional Science, 57(1), 109–131. https://doi.org/10.1111/jors.12300
- Gräbner, C., Heimberger, P., Kapeller, J., & Schütz, B. (2020). Is the eurozone disintegrating? Macroeconomic divergence, structural polarisation, trade and fragility. Cambridge Journal of Economics, 44(3), 647–669. https://doi.org/10.1093/cje/bez059
- Helsper, E. J., & Van Deursen, A. J. A. M. (2015). Digital skills in Europe: Research and policy. Digital Divides: The new Challenges and Opportunities of e-Inclusion, 195, 125–144.
- Hidalgo, A., Gabaly, S., Morales-Alonso, G., & Urueña, A. (2020). The digital divide in light of sustainable development: An approach through advanced machine learning techniques. Technological Forecasting and Social Change, 150(2019), 119754. https://doi.org/10.1016/j.techfore.2019.119754
- Kathuria, V., & Oh, K. Y. (2018). ICT access: Testing for convergence across countries. The Information Society, 34(3), 166–182. https://doi.org/10.1080/01972243.2018.1438549
- Kemeny, T., Petralia, S., & Storper, M. (2022). Disruptive innovation and spatial inequality. Regional Studies, 1–18.
- Kleinknecht, A. (2020). The (negative) impact of supply-side labour market reforms on productivity: An overview of the evidence. Cambridge Journal of Economics, 44(2), 445–464. https://doi.org/10.1093/cje/bez068
- Lucendo-Monedero, A. L., Ruiz-Rodríguez, F., & González Relaño, R. (2019). Measuring the digital divide at regional level. A spatial analysis of the inequalities in digital development of households and individuals in Europe. Telematics and Informatics, 41, 197–217. https://doi.org/10.1016/j.tele.2019.05.002
- Lutz, S. U. (2019). The European digital single market strategy: Local indicators of spatial association 2011–2016. Telecommunications Policy, 43(5), 393–410. https://doi.org/10.1016/j.telpol.2018.10.003
- Maynou, L., Saez, M., Kyriacou, A., & Bacaria, J. (2016). The impact of structural and cohesion funds on eurozone convergence, 1990–2010. Regional Studies, 50(7), 1127–1139. https://doi.org/10.1080/00343404.2014.965137
- Müller, J. M., Buliga, O., & Voigt, K. I. (2021). The role of absorptive capacity and innovation strategy in the design of industry 4.0 business models-A comparison between SMEs and large enterprises. European Management Journal, 39(3), 333–343. https://doi.org/10.1016/j.emj.2020.01.002
- OECD. (2021). Bridging digital divides in G20 countries. OECD Publishing. https://doi.org/10.1787/35c1d850-en.
- Peters, B. (2008). Innovation and firm performance: An empirical investigation for German firms (Vol. 38). Springer Science & Business Media.
- Psycharis, Y., Tselios, V., & Pantazis, P. (2020). The contribution of cohesion funds and nationally funded public investment to regional growth: Evidence from Greece. Regional Studies, 54(1), 95–105. https://doi.org/10.1080/00343404.2018.1525696
- Reljic, J., Evangelista, R., & Pianta, M. (2021). Digital technologies, employment and skills. Industrial and Corporate Change, Online first. https://doi.org/10.1093/icc/dtab059
- Reveiu, A., Vasilescu, M. D., & Banica, A. (2022). Digital divide across the European union and labour market resilience. Regional Studies, 1–15. https://doi.org/10.1080/00343404.2022.2044465
- Rodríguez-Pose, A., & Crescenzi, R. (2008). Research and development, spillovers, innovation systems, and the genesis of regional growth in Europe. Regional Studies, 42(1), 51–67. https://doi.org/10.1080/00343400701654186
- Rodríguez-Pose, A., & Fratesi, U. (2004). Between development and social policies: The impact of European structural funds in objective 1 regions. Regional Studies, 38(1), 97–113. https://doi.org/10.1080/00343400310001632226
- Rodríguez-Pose, A., & Garcilazo, E. (2015). Quality of government and the returns of investment: Examining the impact of cohesion expenditure in European regions. Regional Studies, 49(8), 1274–1290. https://doi.org/10.1080/00343404.2015.1007933
- Rodríguez-Pose, A., & Novak, K. (2013). Learning processes and economic returns in European cohesion policy. Investigaciones regionales. Journal of Regional Research, (25), 7–26.
- Santoalha, A., Consoli, D., & Castellacci, F. (2021). Digital skills, relatedness and green diversification: A study of European regions. Research Policy, 50(9), 104340. https://doi.org/10.1016/j.respol.2021.104340
- Szeles, M. R. (2018). New insights from a multilevel approach to the regional digital divide in the European union. Telecommunications Policy, 42(6), 452–463. https://doi.org/10.1016/j.telpol.2018.03.007
- Thonipara, A., Sternberg, R. G., Proeger, T., & Haefner, L. (2020). Assessing the Digital Divide and its Regional Determinants: Evidence from a Web-Scraping Analysis (No. 25/2020). Working Paper.
- Van Dijk, J. A. G. M. (2008). The digital divide in Europe. The handbook of Internet politics.
- Wooldridge, J. M. (2005). Simple solutions to the initial conditions problem in dynamic, nonlinear panel data models with unobserved heterogeneity. Journal of Applied Econometrics, 20(1), 39–54.
- Zillien, N., & Marr, M. (2013). The digital divide in Europe. In Massimo Ragnedda & Glenn W. Muschert (Eds.), The digital divide (pp. 75–86). Routledge.