Abstract
Immune system disorder is associated with metastasis of triple-negative breast cancers (TNBCs). A robust, individualized immune-related genes (IRGs)-based classifier was aimed to develop and validate in our study to precisely estimate the axillary lymph node (ALN) status preoperatively in patients with early-stage TNBC. We first analyzed RNA sequencing profiles in TNBC patients from The Cancer Genome Atlas database by using bioinformatics approaches, and screened 23 differentially expressed IRGs. A 9-gene panel was generated with an area under the curve (AUC) of 0.77 [95% confidence interval (95% CI), 0.68–0.87]. We detected the 9 ALN-status-related IRGs in the training set (n = 133) and developed a reduced and optimized five-IRGs signature, which effectively distinguished TNBC patients with ALN metastasis (AUC, 0.80; 95% CI, 0.65–0.86), and was superior to preoperative ultrasound-based ALN status (AUC, 0.73; 95% CI, 0.53–0.93). Predictive efficiency (AUC, 0.77; 95% CI 0.61–0.93) of this five-IRGs signature was validated in the validation set (n = 81). Furthermore, IRGs nomogram incorporated IRGs signature with US-based ALN status showed higher ALN status prediction efficacy than US-based ALN status and five-IRGs signature alone in both training and validation sets. IRGs nomogram may aid in identifying patients who can be exempted from axillary surgery.
Novelty and impact: An immune-related genes (IRGs) nomogram was first developed and externally validated in our study, which incorporated the IRGs signature with ultrasound (US)-based axillary lymph nodes (ALN) status. IRGs nomogram is superior to IRGs signature alone for preoperative estimation of ALN metastasis in patients with triple-negative breast cancer (TNBC). It is a favourable biomarker for preoperatively predicting ALN metastasis risk and may aid in clinical decision-making in early-stage TNBCs.
Introduction
Breast cancer (BC) is one of the most common malignancies worldwide and is the first leading cause of cancer-related mortality among women with an estimated 620,000 deaths every year [Citation1,Citation2]. Four major molecular subtypes of BCs were luminal A and B subtypes, HER-2 amplification subtype, and triple-negative subtype [Citation3,Citation4]. Among these, triple-negative breast cancer (TNBC) accounts for approximately 15% of all BCs, and the patients with TNBCs have worse prognosis than those with other subtypes [Citation5,Citation6]. Currently, the diagnosis and clinical staging of BCs is mainly achieved by physical examination, combined with ultrasonography (US), mammography, computed tomography (CT), and magnetic resonance (MR) [Citation7–10]. However, these aforementioned procedures are inadequate for screening and estimating the axillary lymph node (ALN) status of TNBC patients.
Compared with patients suffering from other breast cancer subtypes, patients with TNBC are more likely to suffer ALN metastasis at the time of initial diagnosis [Citation11]. ALN metastasis is confirmed as the most critical independent risk factor at the time of initial diagnosis of TNBCs [Citation12,Citation13]. Currently, a modified radical mastectomy with ALN dissection is still one of the most effective surgical treatments for patients with local advanced BCs without distant metastasis, especially early-TNBCs [Citation14]. However, many patients acknowledged that after surgery their dissected ALNs were non-metastasis pathologically, and ALN dissection before might be unnecessary and excessive, which made patients suffer from many unnecessary risks of postoperative morbidity, such as lymphoedema, shoulder stiffness, paraesthesia, and so on [Citation15–17]. Therefore, it is necessary to investigate a robust diagnostic marker for predicting ALN metastasis before surgery, and which will make significant improvement in precision treatment for patients and effectively avoid excessive ALN dissection, which often results in severe complications [Citation18,Citation19].
ALN status prediction has been brought to the forefront in clinical practice. Several biomarkers such as biological moleculars, classifiers and models have been identified to predict ALN status, nevertheless, limitations are always inevitable [Citation20,Citation21]. As reported, more and more immune-related moleculars have been proven as key factors in the carcinogenesis and development of cancers, such as breast cancer [Citation22]. Presently, immunotherapy by targeting the immune checkpoints has widely used in the clinical treatment of immunogenic breast cancer [Citation23–25]. Moreover, the prognostic value of the immune-related moleculars in TNBCs has been demonstrated in several studies [Citation26–29]. Therefore, in order to gradually establish and complete ALN status prediction, we supplement and perfect clinicopathological and biological prediction with immune-related genomic prediction, and further construct immune-based prognostic signature for TNBCs.
In this study, a systematic analysis of the immune-related genes (IRGs) characteristics of ALN status was conducted, and an IRGs signature was developed and validated subsequently for the preoperative prediction of ALN metastasis in patients with TNBC. Moreover, an IRGs nomogram, incorporating the IRGs signature and clinical risk factors, was constructed and can provide an individual, non-invasive preoperative assessment of the risk of ALN metastasis in TNBC patients.
Materials and methods
Public dataset and RNA-Seq details
To identify a gene-expression signature for detecting ALN metastasis of TNBCs, we first investigated genome-wide gene-expression profiles of primary tumours with or without ALN metastasis in The Cancer Genome Atlas (TCGA) database. RNA-sequencing data of 132 TNBC patients with pathological T1–T2 stage from TCGA was analyzed, among them, 88 patients were ALN metastasis negative and 44 patients were ALN metastasis positive. Clinical characteristics of these 132 patients are shown in Supplementary Table S1.
The normalized TCGA RNA-Seq gene expression data and clinical information for TNBC were downloaded from Firehose Broad GDAC portal (http://gdac.broadinstitute.org/, accessed on 1 June 2018). The TCGA normalized read counts for the RNA-seq data were filtered by assigning all values less than three and log2-transformed to avoid noise. Genes were mapped to the coordinates of human genome using the UCSC Human Genome probe Map.
The Wilcoxon signed-rank test was used to selected differential gene expression between ALN-positive and ALN-negative patients in the TCGA TNBC RNA-Seq dataset. A least absolute shrinkage and selection operator (LASSO) logistic regression model was constructed by using selected gene features. And then, the area under the curve (AUC) values of the receiver operator characteristic (ROC) plots was used to assess the diagnostic performance of each gene panel.
IRGs definition
The immune-related genes (IRGs) were downloaded from the ImmPort database (http://www.immport.org) [Citation30], including 17 immune categories according to their different molecular function, such as T-cell receptor signalling pathway, B-cell receptor signalling pathway, Natural Killer Cell Cytotoxicity, antimicrobials, cytokine, TNF family receptors, and so on. TCGA platform was used to measure 1811 IRGs from the ImmPort database.
Patients
Two independent cohorts with 214 operable TNBC patients who were pathologically diagnosed as T1–T2 were enrolled in this study. Among them, 133 patients and their specimens after surgeries for training set were selected from the Sun Yat-sen University Cancer Centre (SYSUCC), Guangzhou, China, between January 2012 and December 2014. Other 81 TNBC patients and their specimens after surgeries were consecutively enrolled as validation sets from the First Affiliated Hospital of Guangzhou Medical University (GZMUFAH), Guangzhou, China, between January 2010 and August 2016.
The inclusion criteria of patients in our study were as follows: (a) all tumour tissue specimens were histologically confirmed for invasive breast carcinoma; (b) TNBC was diagnosed if immunohistochemical tests showed that oestrogen receptor (ER), progesterone receptor (PR), and human epidermal growth factor receptor 2 (HER2) were all negative; (c) tumours were scored as ER/PR negative if they had less than 1% nuclear staining; (d) available clinicopathological characteristics (age, tumour size, tumour location, histologic grade, Ki67, US-based ALN status) and enough tumour tissues for RNA extraction and quantitative real-time polymerase chain reaction (qRT-PCR) assay; (e) tumour size were pathologically diagnosed as no more than 5 cm; (f) patients should have received sentinel lymph node biopsy or ALN dissection. The exclusion criteria of our study were as follows: (a) patients with distant metastatic disease before operation; (b) patients received chemotherapy or other anticancer treatments before surgery.
In addition, all patients were treated with postoperative systemic treatments according to the clinical practice guidelines. All procedures associated with the study were approved by the Institutional Review Boards of all participating institutions, and ethical approval and written informed consent were obtained for the retrospective analysis.
qRT-PCR analysis of IRGs expression
Total RNAs from 214 formalin-fixed paraffin-embedded (FFPE) TNBC tumour tissues was extracted as described previously [Citation18,Citation31], and the expression of TNBC ALN status-related mRNAs (selected in the discovery stage) was further examined via qRT-PCR. We used PrimeScript™ RT Master Mix (TaKaRa, Dalian, China) to synthesize first-strand cDNA. The qRT-PCR was carried out using SYBR-Green PCR Master Mix (Applied Biosystems, American). Homo sapiens glyceraldehyde 3-phosphate dehydrogenase was used as an internal reference gene to normalize mRNA levels. Expression levels of each mRNA were calculated using the comparative Ct method.
Statistical analysis
Volcano plot analysis was performed based on adjusted t-test p values, false discovery rate (FDR) and absolute fold change (FC). To minimize the risk of over-fitting, we applied a logistic regression model combined with the LASSO (glmnet, version 2.0-5) that is a method of automatic variable selection, which can be used to select predictors X of a target variable Y from a larger set of potential or candidate predictors X. The LASSO punishes the absolute size of the regression coefficient according to the value of the tuning parameter λ. When there are many possible predictors, many of which have an effect on the target variable that is actually zero to very small, then LASSO is particularly useful in variable selection by setting zero for some variables. The penalty parameter was estimated by 10-fold cross-validation (CV) in the training data set at one standard error beyond the minimum partial likelihood deviance. Significant clinicopathologic risk factors were selected by multivariable logistic regression and incorporated into construct an IRGs nomogram. The backward step-wise selection method was used for the likelihood ratio test.
All the statistical analysis was performed with the SPSS software (version 22, SPSS Inc., Chicago, IL) and R statistical software (version 3.5.1; http://www.Rproject.org). The packages of R used in this study are as follows: “glmnet,” “rms,” “Hmisc,” “pROC,” and “Dca.R”. The conventional two-sided p value < .05 was considered significant in all analyses.
Results
Clinical characteristics
We developed the model for the preoperative prediction of ALN metastasis in three stages, including the discovery, training and validation stages. The whole workflow for the discovery of IRGs and the development of an IRGs nomogram is presented in . In total, we recruited 214 TNBC patients who underwent axillary surgery without preoperative therapy from two centres with the exception of TCGA data (Supplementary Table S1). We quantitated the expression of the identified mRNAs in the TNBC patients using qRT-PCR. The patients' clinicopathologic characteristics are summarized in . The baseline clinical and pathological characteristics were similar between the training and validation cohorts (all p > .05).
Figure 1. Study design. SYSUCC: Sun Yat-sen University Cancer Center; GZMUFAH: First Affiliated Hospital of Guangzhou Medical University; LASSO: The least absolute shrinkage and selector operation.
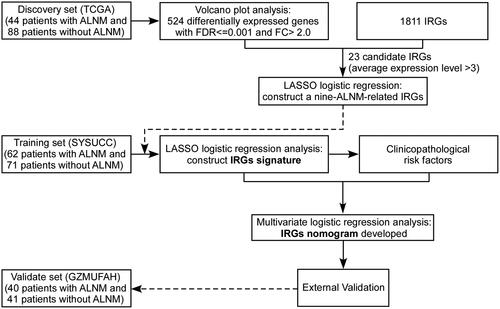
Table 1. Clinicopathological characteristics of all patients from two independent centres.
Selection of candidate IRGs to predict ALN metastasis
In the discovery stage, we identified 524 differentially expressed mRNAs in discovery set by volcano plot analysis (FC > 2. 0, FDR ≤ 0.001 and p < 0.05) (). IRGs in TNBCs which can promote tumour metastasis were downloaded from ImmPort database (http://immport.niaid.nih.gov) and 36 IRGs were found associated with ALN metastasis from the 524 mRNAs. To optimization the genes signature for a clinically viable and practical assay, we then filtered out all candidate genes with low expression levels (average expression level < 3), which subsequently resulted in a panel of 23 IRGs (Supplementary Table S2). To avoid colinearity, we then used a LASSO logistic regression algorithm to screen ALN status-correlated mRNAs and selected nine TNBC-associated IRGs from the above 23 IRGs (); The optimal tuning parameter λ estimation in the LASSO model corresponded to the minimum prediction errors via the 10-fold CV method (). Using risk scores based on the detection of logistic regression, we evaluated the performance of the constructed 9-gene panel [AUC = 0.77, 95% confidence interval (95% CI) = 0.68–0.87] (). Compared with other clinical factors such as age (AUC = 0.54, 95%CI = 0.39–0.69), tumour stage (AUC = 0.51, 95% CI = 0.25–0.77), and the anatomical location of the primary tumour (AUC = 0.55, 95% CI = 0.25–0.85), our model achieved a superior diagnostic power (all p < .01 compared with each factor) (). To obtain biological information of the IRGs signature, we carried out enrichment analysis of the 9 unique component IRGs with DAVID tool (https://david.ncifcrf.gov/). Biological processes of gene ontology (GO) with false discovery rate (FDR) value less than 0.05 were selected and 15 over-represented biological processes in GO were identified (Supplementary Figure 1). Most biological processes were epidermal growth factor receptor signalling pathway, phagocytosis, glucose metabolic process, and leukocyte migration.
Figure 2. Construction of an immune-related genes (IRGs) signature. (a) Volcano plot illustrating a comparison of mRNAs expression in early-stage TNBC with ALN metastasis or not, and finally 524 mRNAs were selected as candidates for the establishment of IRGs signature. (b) Hierarchical clustering shows the collinearity of 36 candidate IRGs among the above 524 mRNAs. (c) LASSO algorithms were used to identify ALN-status-related IRGs and nine optimal IRGs were selected in the discovery cohort. (d) LASSO coefficient profiles of the 23 ALN-status-related IRGs based on the TCGA data.
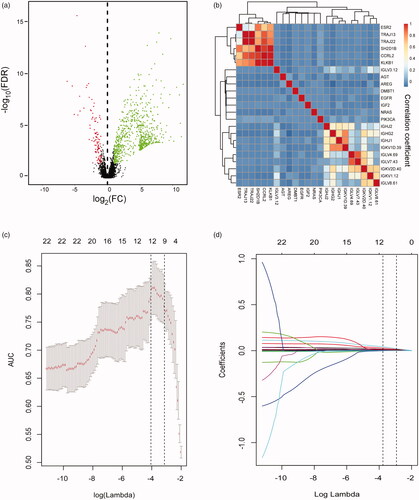
Figure 3. Receiver operating characteristic (ROC) curves comparing the accuracy of the 9-gene panel, age, sex, and tumour location in the TCGA dataset. The ROC curve of the 9-gene panel was based on a 10-fold cross-validation on the TCGA dataset and the average area under the ROC curve (AUC) was 0.77 (95% CI = 0.68–0.87). CI: confidence interval; ALN indicates axillary lymph node.
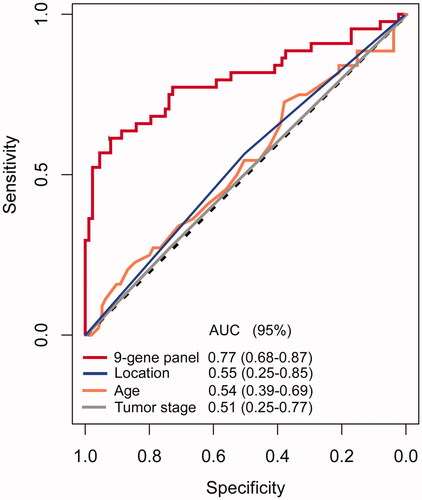
Development and validation of an IRGs signature to predict ALN status of TNBC
In the training stage, a LASSO logistic regression analysis was used to construct a five-IRGs signature for predicting ALN status in the training set (the SYSUCC set, n = 133) based on the findings in the discovery stage (). The qRT-PCR expression values of the five candidate IRGs in ALN positive and negative TNBC patients are shown in Supplementary Figure 2a. According to our trained model, a risk score for the risk of ALN metastasis was calculated for each patient based on their individual expression levels of the final five IRGs: risk score = −1.8535537002 + (0.3872794910*IIGHG2) + (0.0008150497*IIGHJ2) + (0.0359629751*IIGKV1D_39) + (0.1011920809*INRAS) + (0.0927424924*IPIK3CA). In this formula, Ix indicates the log10-scaled expression value of x gene. ROC analysis was performed to investigate the predictive efficiency of the IRGs signature. The resulting AUC was 0.80 (95%CI = 0.65–0.95), which indicated that our five-IRGs-based signature had superior predictive performance than individual IRGs to discriminate individuals with ALN metastasis from all patients in the training set ( and Supplementary Figure 2(b)). Predictive efficiency of the five-IRGs-based signature was validated in the validation set (n = 81), with resulting AUC value of 0.77 (95%CI 0.61–0.93) (). When stratified by US-based ALN status, there was a potential benefit for our IRGs signature to identifying the high-risk population among those who were US-based ALN negative and the low-risk population among those who were US-based ALN positive, thus avoiding understaging or overstaging in clinical practice (Supplementary Figure 3).
Figure 4. Development and evaluation of an immune-related genes (IRGs) signature. (a) Five optimal IRGs were selected based on LASSO algorithms in the training set. (b)LASSO coefficient profiles of the five ALN-status-related IRGs based on the training set data. Plots (c) and (d) show the ROC curves of the IRGs signature in the training and validation cohort, respectively.
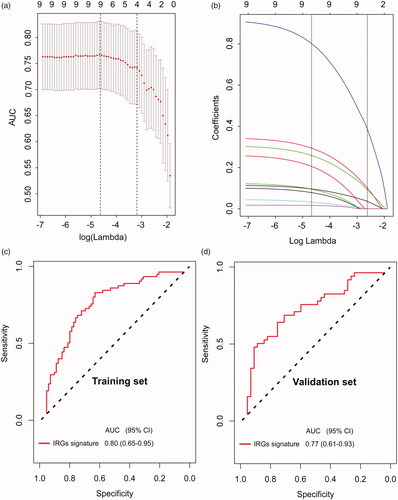
Development and validation of an IRGs nomogram for individualized prediction
To construct a clinical nomogram, we first evaluated the risk of clinicopathological factors with univariate analysis () and selected only US-based ALN status using a multivariable logistic regression model (). By combining the selected US-based ALN status with the IRGs signature, we constructed an IRGs nomogram, which can predict the individual risk of ALN metastasis in TNBC patients preoperatively (). To compare the discrimination ability of the IRGs nomogram with the US-based ALN status, we conducted a ROC analysis of the TNBC patients. The results suggested that the IRGs nomogram had higher prediction efficacy (AUC = 0.87, 95% CI = 0.76–0.95) compared with the model containing US-based ALN status (AUC = 0.73, 95% CI = 0.53–0.93) alone in training set (). The corresponding calibration curve indicated good discrimination for the nomogram, which is shown in . The Hosmer–Lemeshow test suggested that there was no significant departure from excellent fit (p > .05). In the validation stage, the IRGs nomogram was externally validated in the GZMUFAH set (n = 81) with higher prediction efficacy (AUC = 0.82, 95% CI = 0.73–0.90 for IRGs nomogram; AUC = 0.67, 95%CI = 0.50–0.85 for US-based ALN status) (). Calibration curves were also plotted to assess the calibration of the nomogram () and the Hosmer–Lemeshow test also indicated a satisfactory goodness-of-fit in the GZMUFAH set (p > .05).
Figure 5. The IRGs nomogram for ALN metastasis prediction in patients with TNBC. (a) IRGs nomogram was constructed to preoperatively predict ALN metastasis, with the US-based ALN status and IRGs signature incorporated. Plots (b) and (c) show the ROC curves of the IRGs nomogram in the training and validation cohort, respectively. Calibration curve for clinical-immune signature in (d) training cohort and (e) validation cohort. The 45-degree solid dotted black line represents a perfect prediction, and the red solid line represents the predictive performance of the nomogram, of which a closer fit to the dashed line indicates means a better prediction accuracy for the model.
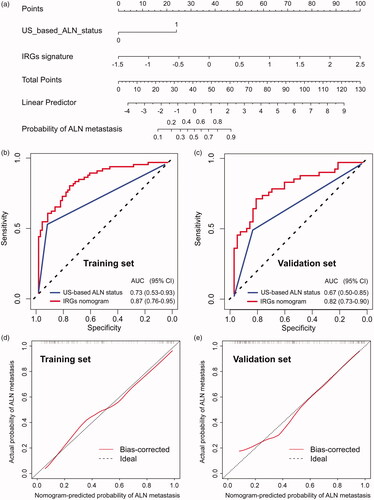
Table 2. Univariate association of IRGs signature and clinicopathological factors with ALN status in TNBC.
Table 3. Multivariate logistic regression analysis for ALN metastasis in TNBC.
Moreover, the DCA results for the nomogram are presented in . The DCA showed that if the threshold probability for patients or clinicians is 14% or more, ALN status-related treatment decision-making based on the IRGs nomogram adds more net benefit than treating either all patients or none scheme in each independent data set in this study. It also showed higher net benefit than IRGs signature alone.
Figure 6. Model comparisons and clinical usefulness of the IRGs nomogram. Decision curve analysis of the prediction nomogram model in (a) training set and (b) validation set. Solid black line: net benefit when all breast cancer patients are considered as not having the ALN metastasis; solid grey line: net benefit when all breast cancer patients are considered as having the event. Solid red or blue line: net benefit when all breast cancer patients are considered according to the developed IRGs signature or nomogram model. In our current study, the decision curves in both the training and validation sets showed that if the threshold probability is 14% or more, then using the IRGs signature or nomogram to predict ALN metastases adds more benefit than treating either all or no patients, while the ideal model is the model with the highest net benefit at any given threshold.
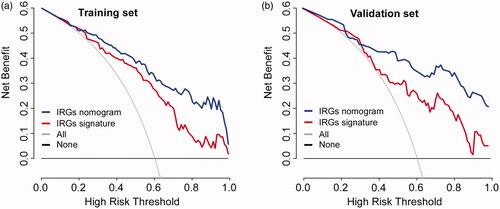
Discussion
In our study, we performed a systematic and comprehensive RNA sequencing-based IRGs expression profiling analysis in specimens of TNBC patients with/without ALN metastasis, and developed a five IRGs signature for identifying ALN metastasis, which showed robust performance signature in both training and validation cohorts. Furthermore, we demonstrated that our five IRGs signature was superior to currently used clinicopathological risk factors in diagnosing ALN metastasis. In addition, the ALN status prediction performance of a combined classifier which incorporated five IRGs signature with US-based ALN status was significantly superior to the IRGs signature or single clinicopathological feature in TNBC patients.
ALN status is vitally important for therapeutic decision-making for early T stage TNBC patients in clinical [Citation32]. Currently, sentinel lymph node biopsy and lymphadenectomy following pathologic diagnosis is the best approach for assessing ALN status of TNBC patients. According to the American Joint Committee on Cancer and the Union for International Cancer Control, N staging for TNBC is classified based on the number of metastatic ALNs. It is obvious that the number of identified metastatic ALNs improve with increase in the total number of surgically dissected ALNs. Thus, inadequate number of dissected ALNs could potentially lead to stage migration and subsequent underestimation of the disease severity. However, sufficient dissection of ALN radical lymphadenectomy may lead to dysfunction, and so on. Therefore, availability of robust biomarkers that facilitate categorization of patients with TNBC before surgery based on the ALN status will permit individualization of the surgical treatments and improve overall mortality and morbidity of TNBC patients by avoiding excessive lymphadenectomy. The subgroup of patients with a very low risk of ALN metastasis identified with the our nomogram might be candidate for observation strategy and no axillary surgery is indeed required. While ALN dissection should be conducted directly and sentinel lymph node biopsy is no longer necessary for the TNBC patients with high risk of ALN metastasis. To our knowledge, our study is the largest multi-molecular markers analysis of primary tumour of early invasive TNBC with known ALN status to date and reveals an association between IRGs expression and ALN status. Although the accuracy of individual genes was relatively modest, the combination of multiple genes revealed remarkable diagnostic accuracy. Collectively, we demonstrated that the potential of a multigene panel for identifying ALN metastasis in TNBC is realistic, and can be used in the clinic in not so distance future.
With the rapid development of whole genome and transcriptome sequencing in recent years, the molecular characteristics of most cancer types are becoming clearer and clearer, including TNBC [Citation33–37]. Primary tumours may produce the premetastatic niche before subsequent metastasis in secondary organ, including ALN [Citation38]. In patients with TNBC, the immune system and tumour immuno-microenvironment are the key to premetastatic niche formation and are related to the prognosis and survival of patients [18,Citation38–40]. In addition, the continuous stimulation of tumour-derived factors leads to the formation of immunosuppressive microenvironments in lymph nodes, including cytotoxic T-cells. In addition, as the main cell component of lymph nodes, B cells have been shown to be essential for lymphangiogenesis, which indicates that B cells have a certain role in lymph node metastasis. Mechanistically, new roles of B cells in tumour-promoting humoral immunity and resistance to cancer therapy have been found [Citation38]. Although in lymph nodes, T cells are the main component of this environment, there is a significant accumulation of B-cells, rather than T-cells, in lymph nodes shortly after breast cancer cell inoculation. And the IgG production of these B-cells increased significantly in lymph nodes, suggesting the B-cells promote the lymph node premetastatic niche formation through the systemic humoral immune response to tumours [Citation38,Citation41]. From the ImmPort database, we found that more genes were involved in B-cells rather than T-cells receptors processes, which is consistent with the latest research finding [Citation38]. As far as we know, our study is the first to develop an immune system-based multigene expreesion classifier for determination of TNBC ALN status. Among the remaining genes in our IRGs signature, NRAS and PIK3CA have been reported to involve in cancer cell proliferation, invasion, and angiogenesis [Citation42,Citation43]; however, the function of IGHG2, IGHJ2 and IGKV1D_39 in cancer is not completely clear and deserves further study.
Although our current results show that our IRGs signature can distinguish TNBC ALN status preoperatively, there were some inherent limitations to the current study. First, the retrospective nature of our study is the inherent limitation, although we have tried to include different data cohorts for training and validation of our biomarker. Second, the source of tumour samples for gene expression detection is from paraffin tissue samples, not from fresh tumour coarse needle puncture tissues before axillary surgery. Considering that breast punctual biopsy is a common diagnosed practice to collect biopsy samples before the surgery, it would have been ideal to validate our gene signature in punctual biopsy specimens. In addition, we did not evaluate the role of our model in other molecular subtypes and BC patients treated with neoadjuvant chemotherapy because our study was initially conducted based on operable TNBC. Moreover, diverse biological processes that may provide more complete molecular features of the tumours will be integrated in our future studies.
Conclusions
In this study, we developed a novel IRGs signature to predict ALN metastasis of patients with TNBC. IRGs nomogram, which incorporated IRGs signature and US-based ALN status showed clinical usefulness in stratifying patients with or without ALN metastasis in TNBCs and identifying patients who can be exempted from axillary surgery. These results may improve current treatment standards.
Ethics approval and consent to participate
The present study was approved the Institutional Review Board of Sun Yat-sen University Cancer Centre and the First Affiliated Hospital of Guangzhou Medical University.
Consent for publication
We have received consent from individual patients who have participated in this study. The consent forms will be provided upon request.
Authors’ Contributions
Conceptualization: Hailin Tang and Wenbo Zheng; Data curation: Xinhua Xie, Zhongying Huang, Lun Chen and Wei Tang; Formal analysis: Hailin Tang, Xinhua Xie, Zhongying Huang and Weige Tan; Funding acquisition: Xinhua Xie and Weige Tan; Investigation: Lun Chen, Lingxiao Pan and Weige Tan; Methodology: Hailin Tang, Xinhua Xie, Zhongying Huang, Renjie Zhu, Xigang Ye and Jin Gao; Project administration: Lun Chen and Xigang Ye; Resources: Lun Chen, Xiaoshen Zhang, Lingxiao Pan and Jin Gao; Software: Wei Tang, Renjie Zhu, Xiaoshen Zhang and Lingxiao Pan; Supervision: Xinhua Xie and Wenbo Zheng; Validation: Xigang Ye and Wenbo Zheng; Visualization: Wei Tang, Xigang Ye and Wenbo Zheng; Writing_original draft: Hailin Tang, Xinhua Xie and Zhongying Huang; Writing_review and editing: Xinhua Xie, Weige Tan and Wenbo Zheng.
Abbreviations | ||
ALN | = | axillary lymph node |
AUC | = | area under the curve |
BC | = | breast cancer |
95% CI | = | 95% confidence interval |
CT | = | computed tomography |
CV | = | cross-validation |
FC | = | fold change |
FDR | = | false discovery rate |
FFPE | = | formalin-fixed paraffin-embedded |
GZMUFAH | = | the First Affiliated Hospital of Guangzhou Medical University |
IRGs | = | immune-related genes |
LASSO | = | least absolute shrinkage and selection operator |
MR | = | magnetic resonance |
qRT-PCR | = | quantitative real-time polymerase chain reaction |
ROC | = | receiver operator characteristic |
SYSUCC | = | Sun Yat-sen University Cancer Centre |
TCGA | = | The Cancer Genome Atlas |
TNBC | = | triple-negative breast cancer; US: ultrasonography |
ER | = | oestrogen receptor |
PR | = | progesterone receptor |
HER2 | = | human epidermal growth factor receptor 2 |
supplementary_file.2019.9.12.doc
Download MS Word (1.7 MB)Acknowledgments
The authors are thankful to the TCGA Research Network and the involved patients for its contribution.
Disclosure statement
The authors declare that they have no conflicts of interest.
Data availability
The datasets supporting the conclusions of this article are included within the article and its Additional files.
Additional information
Funding
References
- Siegel RL, Miller KD, Jemal A. Cancer statistics, 2018. CA Cancer J Clin. 2018;68(1):7–30.
- DeSantis CE, Ma J, Goding SA, et al. Breast cancer statistics, 2017, racial disparity in mortality by state. CA Cancer J Clin. 2017;67(6):439–448.
- Plevritis SK, Munoz D, Kurian AW, et al. Association of screening and treatment with breast cancer mortality by molecular subtype in US Women, 2000–2012. JAMA. 2018;319(2):154–164.
- Tobin NP, Harrell JC, Lovrot J, et al. Molecular subtype and tumor characteristics of breast cancer metastases as assessed by gene expression significantly influence patient post-relapse survival. Ann Oncol. 2015;26(1):81–88.
- Bianchini G, Balko JM, Mayer IA, et al. Triple-negative breast cancer: challenges and opportunities of a heterogeneous disease. Nat Rev Clin Oncol. 2016;13(11):674–690.
- Keren L, Bosse M, Marquez D, et al. A structured tumor-immune microenvironment in triple negative breast cancer revealed by multiplexed ion beam imaging. Cell. 2018;174(6):1373–1387.
- Cho N, Han W, Han BK, et al. Breast cancer screening with mammography plus ultrasonography or magnetic resonance imaging in women 50 years or younger at diagnosis and treated with breast conservation therapy. JAMA Oncol. 2017;3(11):1495–1502.
- Shieh Y, Eklund M, Madlensky L, et al. Breast cancer screening in the precision medicine era: risk-based screening in a population-based trial. J Natl Cancer Inst. 2017;5:109.
- Lee CH, Dershaw DD, Kopans D, et al. Breast cancer screening with imaging: recommendations from the Society of Breast Imaging and the ACR on the use of mammography, breast MRI, breast ultrasound, and other technologies for the detection of clinically occult breast cancer. J Am Coll Radiol. 2010;7(1):18–27.
- Friedewald SM, Rafferty EA, Rose SL, et al. Breast cancer screening using tomosynthesis in combination with digital mammography. JAMA. 2014;311(24):2499–2507.
- Barry P, Vatsiou A, Spiteri I, et al. The spatiotemporal evolution of lymph node spread in early breast cancer. Clin Cancer Res. 2018;24(19):4763–4770.
- Houvenaeghel G, Sabatier R, Reyal F, et al. Axillary lymph node micrometastases decrease triple-negative early breast cancer survival. Br J Cancer. 2016;115(9):1024–1031.
- Wang L, Hu X, Wang P, et al. Integrative 3' untranslated region-based model to identify patients with low risk of axillary lymph node metastasis in operable triple-negative breast cancer. Oncologist. 2018;24(1):22–30.
- Lyman GH, Somerfield MR, Bosserman LD, et al. Sentinel lymph node biopsy for patients with early-stage breast cancer: American Society of Clinical Oncology Clinical Practice Guideline Update. JCO. 2017;35(5):561–564.
- Giuliano AE, Ballman KV, McCall L, et al. Effect of axillary dissection vs no axillary dissection on 10-year overall survival among women with invasive breast cancer and sentinel node metastasis: the ACOSOG Z0011 (Alliance) randomized clinical trial. JAMA. 2017;318(10):918–926.
- Tadros AB, Yang WT, Krishnamurthy S, et al. Identification of patients with documented pathologic complete response in the breast after neoadjuvant chemotherapy for omission of axillary surgery. JAMA Surg. 2017;152(7):665–670.
- Uno H, Hassett MJ, Wei LJ. Axillary vs sentinel lymph node dissection in women with invasive breast cancer. JAMA. 2018;319(3):306.
- Xie X, Tan W, Chen B, et al. Preoperative prediction nomogram based on primary tumor miRNAs signature and clinical-related features for axillary lymph node metastasis in early-stage invasive breast cancer. Int J Cancer. 2018;142(9):1901–1910.
- Balasubramanian I, Fleming CA, Corrigan MA, et al. Meta-analysis of the diagnostic accuracy of ultrasound-guided fine-needle aspiration and core needle biopsy in diagnosing axillary lymph node metastasis. Br J Surg. 2018;105(10):1244–1253.
- Vieira AF, Dionisio MR, Gomes M, et al. P-cadherin: a useful biomarker for axillary-based breast cancer decisions in the clinical practice. Mod Pathol. 2017;30(5):698–709.
- Dihge L, Bendahl PO, Ryden L. Nomograms for preoperative prediction of axillary nodal status in breast cancer. Br J Surg. 2017;104(11):1494–1505.
- Gentles AJ, Newman AM, Liu CL, et al. The prognostic landscape of genes and infiltrating immune cells across human cancers. Nat Med. 2015;21(8):938–945.
- Topalian SL, Drake CG, Pardoll DM. Immune checkpoint blockade: a common denominator approach to cancer therapy. Cancer Cell. 2015;27(4):450–461.
- Kok M, Winer EP, Loi S. Passion for immune checkpoint blockade in triple negative breast cancer: comment on the IMpassion130 study. Ann Oncol. 2019;30(1):13–16.
- Li CW, Lim SO, Chung EM, et al. Eradication of triple-negative breast cancer cells by targeting glycosylated PD-L1. Cancer Cell. 2018;33(2):187–201.
- Denkert C, von Minckwitz G, Darb-Esfahani S, et al. Tumour-infiltrating lymphocytes and prognosis in different subtypes of breast cancer: a pooled analysis of 3771 patients treated with neoadjuvant therapy. Lancet Oncol. 2018;19(1):40–50.
- Karn T, Jiang T, Hatzis C, et al. Association between genomic metrics and immune infiltration in triple-negative breast cancer. JAMA Oncol. 2017;3(12):1707–1711.
- Denkert C, Liedtke C, Tutt A, et al. Molecular alterations in triple-negative breast cancer-the road to new treatment strategies. Lancet. 2017;389(10087):2430–2442.
- Savas P, Virassamy B, Ye C, et al. Single-cell profiling of breast cancer T cells reveals a tissue-resident memory subset associated with improved prognosis. Nat Med. 2018;24(7):986–993.
- Bhattacharya S, Andorf S, Gomes L, et al. ImmPort: disseminating data to the public for the future of immunology. Immunol Res. 2014;58(2–3):234–239.
- Gong C, Tan W, Chen K, et al. Prognostic value of a BCSC-associated microRNA signature in hormone receptor-positive HER2-negative breast cancer. Ebiomedicine. 2016;11:199–209.
- Morrow M, Jagsi R, McLeod MC, et al. Surgeon attitudes toward the omission of axillary dissection in early breast cancer. JAMA Oncol. 2018;4(11):1511.
- Kwa M, Makris A, Esteva FJ. Clinical utility of gene-expression signatures in early stage breast cancer. Nat Rev Clin Oncol. 2017;14(10):595–610.
- Banerji S, Cibulskis K, Rangel-Escareno C, et al. Sequence analysis of mutations and translocations across breast cancer subtypes. Nature. 2012;486(7403):405–409.
- Lu HM, Li S, Black MH, et al. Association of breast and ovarian cancers with predisposition genes identified by large-scale sequencing. JAMA Oncol. 2018; 5(1):51–57.
- Ma X, Liu Y, Liu Y, et al. Pan-cancer genome and transcriptome analyses of 1,699 paediatric leukaemias and solid tumours. Nature. 2018;555(7696):371–376.
- Qu A, Yang Y, Zhang X, et al. Development of a preoperative prediction nomogram for lymph node metastasis in colorectal cancer based on a novel serum miRNA signature and CT scans. Ebiomedicine. 2018;37:125.
- Gu Y, Liu Y, Fu L, et al. Tumor-educated B cells selectively promote breast cancer lymph node metastasis by HSPA4-targeting IgG. Nat Med. 2019;25(2):312.
- Steenbrugge J, Breyne K, Demeyere K, et al. Anti-inflammatory signaling by mammary tumor cells mediates prometastatic macrophage polarization in an innovative intraductal mouse model for triple-negative breast cancer. J Exp Clin Cancer Res. 2018;37(1):191.
- Takada K, Kashiwagi S, Goto W, et al. Possibility of avoiding axillary lymph node dissection by immune microenvironment monitoring in preoperative chemotherapy for breast cancer. J Transl Med. 2018;16(1):318.
- Olkhanud PB, Damdinsuren B, Bodogai M, et al. Tumor-evoked regulatory B cells promote breast cancer metastasis by converting resting CD4(+) T cells to T-regulatory cells. Cancer Res. 2011;71(10):3505–3515.
- Song YK, Wang Y, Wen YY, et al. MicroRNA-22 suppresses breast cancer cell growth and increases paclitaxel sensitivity by targeting NRAS. Technol Cancer Res Treat. 2018;17:153303381880999.
- Rimawi MF, De Angelis C, Contreras A, et al. Low PTEN levels and PIK3CA mutations predict resistance to neoadjuvant lapatinib and trastuzumab without chemotherapy in patients with HER2 over-expressing breast cancer. Breast Cancer Res Treat. 2018;167(3):731–740.