Abstract
According to previous studies, linc-UBC1 is abnormally expressed in various human tumours. Nonetheless, the clinical significance and mechanism of linc-UBC1 in cancer remains unclear. In our present analysis, we wanted to explore the specific role of linc-UBC1 in malignant tumours by integrating all of the relevant literature and subsequently elucidating the relationship between linc-UBC1 expression level and clinical characteristics of cancers. An elaborate database search of PubMed, Embase, Wanfang Data, Web of Science, Ovid, Medline, Cochrane Library and PMC was carried out up to 8 August 2019. We further applied the pooled odds ratio (OR) and hazard ratio (HR) to evaluate OS. After filtering by strict criteria, 11 studies containing 1017 cases were included in this analysis. Our results implied that high expression of linc-UBC1 was obviously related to poor OS in cancer (HR =1.735, 95% 1.348–2.235, p < .001 random effects model). Analogously, the data revealed that high expression of linc-UBC1 was highly correlated with lymph node metastasis (OR = 2.912, 95% CI: 2.056–4.125, p < .001 fix effects model) and high tumour stage (OR = 2.678, 95% CI: 1.859–3.857, p < .001 fix effects model). In summary, linc-UBC1 overexpression is associated with poor OS and advanced tumour stage and could be used as a novel prognostic biomarker in various cancers.
Introduction
With the aging of the population and continuous improvement of medical diagnostic techniques, cancer has become the most dominant public concern in the world and the single barrier to increasing life expectancy worldwide. Based on the estimates of GLOBOCAN 2018, there were approximately 18.1 million new cancer cases and 9.6 million cancer-related deaths worldwide in 2018 [Citation1]. Since 2010, cancer has been the main cause of death in China and other countries [Citation1,Citation2]. For patients with nonmetastatic cancer in the early stage, there are still many treatments, such as radical tumour resection, radiotherapy and chemotherapy, and radiofrequency ablation, to address their disease. However, for patients with advancing tumour stage, the treatments that can be used are still limited [Citation3]. Lymph node metastasis is considered a key predictor of tumour progression and is widely used in clinical prognosis [Citation4]. Additionally, tumour-node-metastasis (TNM) stage has always been identified as a predictive factor of prognosis [Citation5]. Therefore, the identification of predictive factors for the LNM and TNM of tumours are urgent.
At present, traditional diagnostic methods, including imaging detection, needle biopsy and blood testing, can only detect large lesions but are often not sensitive to small lesions, so they prone to false negatives for small lesions [Citation6]. Therefore, it is necessary to find a method that can detect lesions more accurately. With the spread of whole-genome sequencing, the integration of genomics and bioinformatics analysis suggests the potential for potential predictors of tumour prognosis [Citation7,Citation8]. Nonetheless, many markers that have been found in a particular cancer type do not have consistent functions in other cancers [Citation9]. A biomarker that broadly predicts tumour prognosis and metastasis needs to be further applied to clinical diagnosis.
Long noncoding RNA (lncRNA) is a noncoding RNA that plays various biological roles in organisms [Citation10]. In addition, an increasing number of studies explain that lncRNA plays a role in promoting cancer on transcriptional and posttranscriptional levels [Citation9,Citation10]. Long noncoding RNA linc-UBC1, also known as BLACAT1, is a 2,616-kb lncRNA encoded on human chromosome 1q32.1 [Citation11]. It was first found to be expressed at a high level in bladder cancer (BLA) [Citation11,Citation12]. Recent studies have revealed that linc-UBC1 has a pro-oncogenic role in solid tumours [Citation13,Citation14]. In addition to BLA, linc-UBC1 has been found to be involved in the tumorigenesis of many other cancers, such as papillary thyroid carcinoma (PTC) [Citation15], cervical cancer (CC) [Citation16,Citation17], colorectal cancer (CRC) [Citation14,Citation18], gastric cancer (GC) [Citation19], oesophageal squamous cell carcinoma (ESCC) [Citation20], urothelial carcinoma (UC) [Citation21], and lung cancer [Citation13,Citation22,Citation23]. More importantly, the expression of linc-UBC1 is thought to be significantly related to the prognosis and stage of the above cancers. Linc-UBC1 may play a similar pro-oncogenic function in different cancers. However, there is no systematic review to integrate the related data for linc-UBC1. To further uncover and summarize the relationship between linc-UBC1 expression level and relative clinical features, this first meta-analysis of relevant articles was conducted to evaluate whether linc-UBC1 could be used as a biomarker in the prognosis of different tumours.
Methods
Studies collection
Our group search databases by using “linc-UBC1 or (lncRNA upregulated in bladder cancer 1)” and “cancer or tumor” as search keywords in PubMed, Embase, Wanfang Data, Web of Science, Ovid, Medline, Cochrane Library, and PMC databases and selecting potentially related articles available as of 8 August 2019.
Exclusion and inclusion criteria
The inclusion criteria were as follows: (1) analysis of Linc-UBC1 expression group and prognosis in cancer patients; (2) patients divided into high/low expression groups; and (3) available clinical features, including TNM, OS and LNM. The exclusion criteria were as follows: (1) abstracts, comments, case reports, reviews and animal studies; (2) studies without clinical features; and (3) studies unrelated to linc-UBC1.
Data extraction
Two authors (ZXW, XHB) extracted and reviewed relevant information from the eligible articles in line with the inclusion and exclusion criteria. Disagreements were solved by the corresponding author (LYK), and the second author (LWH) assisted. The following information was extracted: author, publication year, multivariate analysis, country of author, type of cancer, cut-off values, total number of included patients, number of high and low linc-UBC1 expression group, number of patients with LNM, number of patients with (high tumour stage) HTS, hazard ratios (HRs) and corresponding 95% CI for OS, detection method of Linc-UBC1 expression levels, follow-up months and OS.
Statistical methods
Most HR values came from the included studies; log HR and standard error (SE) were used to integrate survival results [Citation24]. Some HR values could not be obtained directly from the articles, so we used software (Engauge Digitiser software) to calculate HRs by analysing Kaplan-Meier curves. In addition, to evaluate the heterogeneity of the available data, we apply I2 and p values to assess the pooled HR. [Citation25]. When there was no interstudy heterogeneity in the eligible articles (p > .1 and I2 < 50%), we used the fixed-effects model to assess the analysis. In contrast, the random-effects model is used for the model analysis when there was a large degree of heterogeneity between studies (p < .1 and I2 > 50%). The Egger or Begg test was used to evaluate potential publication bias. The sensitivity analysis was conducted to assess the stability of eligible articles. Stata 12.0 was applied to analyse all the statistical analyses. p values < .05 indicated that a relationship was statistically significant.
Quality assessment
The quality of the included articles was assessed by two investigators (LMJ, LDQ) on the basis of the Newcastle-Ottawa Scale (NOS) [Citation26]. For any divergence, a third investigator (LYK) assessed the quality again. Supplementary Table 1 displays the explicit standard for quality assessment. A higher total score represents a higher article quality.
Results
Identification of articles
A preliminary search of the electronic database provided 97 studies. By excluding duplicate articles, 55 potential related articles were identified. After careful screening of abstracts, irrelevant research was removed, and 23 potentially relevant studies were selected. After evaluating the full texts, 12 studies without survival results and another clinical pathological parameter information were eliminated. In the end, 11 studies were selected for the final meta-analysis. ()
Characteristics of the included articles
In 11 articles, a total of 1,017 patients were included, with an average sample size of N = 92.45 (range 44–160) per article. Among them, 5 studies recruited more than 100 cancer patients. Most of the articles were from China, and one article was from Europe. All patients in our included studies underwent surgery. This review evaluated nine cancers, mainly urinary cancers, indicating that linc-UBC1 is closely associated with urinary cancer. All studies used qPCR to detect the expression of linc-UBC1 in cancer tissues. The reference genes were glyceraldehyde-3-phosphate dehydrogenase (GAPDH), TBP or β-actin. In our study, linc-UBC1 expression was high in almost all cancer samples, including papillary thyroid carcinoma (PTC) samples, and significantly different from that in para-carcinoma tissue. includes the main clinical features from eligible studies. In addition, the quality of the studies we was verified by applying the Newcastle-Ottawa Scale (NOS), and all articles maintained a high quality ().
Table 1. Characteristics of studies in this meta-analysis.
Table 2. Quality assessment of eligible studies (Newcastle-Ottawa Scale).
Meta-analysis
Association between linc-UBC1 and OS
In total, 10 studies included information related to OS, including a total of 930 patients. The HRs of the seven articles were extracted directly from the data published in corresponding articles. The HR of another three articles was calculated by the method mentioned in the method section. Furthermore, analysis using Stata 12.0 software displayed an obviously significant relationship between high expression of linc-UBC1 and OS (p < .0001, HR = 1.735, 95% CI: 1.348–2.235, random effect model), with moderate heterogeneity (I2 = 42.7%, p = .073) (). To explore the independent function and heterogeneity of linc-UBC1 in OS, we performed a subgroup analysis of the OS group based on type of cancer and sample size. For the set of cancer types, there was a significant negative correlation between linc-UBC1 expression and OS in the following tumours: BLC (HR = 1.963, 95% CI: 1.093–3.526, p = .024), CRC (HR = 1.615, 95% CI: 1.141–2.284, p = .007), and LC (HR = 1.703, 95% CI: 1.054–2.751, p = .03) (Supplementary Figure 1A). All of the above cancers showed little heterogeneity between them (I2 < 50%, p > 1.0). For another type of cancer (HR = 2.082, 95% CI: 0.944–5.799, p = .069), significant heterogeneity was found (I2 = 76%, p = .006) (), which may be due to the large differences between various cancers. Regarding the UC, CC, GC, and ESCC groups, because the above tumours were only reported in one of our studies, subgroup analysis could not be performed. Similarly, for grouping of sample sizes, the association between linc-UBC1 and OS was present in more than 100 (HR = 1.794, 95% CI: 11.107–2.996, p = .001) or less than 100 samples (HR = 1.53, 95% CI: 1.356–1.737, p < .0001) (Supplementary Figure 1B). Taken together, for almost all included tumours, the high linc-UBC1 expression pattern is more likely to be related to poor OS than the low expression group. More importantly, the subgroup analysis suggested that, in terms of OS, linc-UBC1 may be an independent prognostic indicator for different cancers.
Table 3. Results of this meta-analysis.
Correlation between Linc-UBC1 and clinicopathological traits of cancer
Seven studies analysed the association between linc-UBC1 and the clinical pathological characteristics of cancer, including 622 patients with TNM stage data. The tumours studied in the 7 articles included BLC, PTC and CC. Due to the significant heterogeneity between the included studies (I2 = 65.4%, p = .008), we applied the random-effect model to examine the correlation between linc-UBC1 expression and TNM stage. The OR between the high and low expression groups (III/IV vs. I/II) of linc-UBC1 was 2.157 (95% CI: 1.171–3.975, p = .008, random effects model) (Supplementary Figure 2A). To evaluate the reason for the high heterogeneity, we found a significant difference between studies by Liao et al. and other studies (Supplementary Figure 1A). Further sensitivity analysis and publication bias analysis in HTS revealed an obvious bias (p < .1) and an unstable pattern (Supplementary Figure 2B and 2C). Thus, we excluded the study of Liao et al. from the TNM group and reanalysed the association between linc-UBC1 levels and TNM. The final six-article examination showed strong trend between linc-UBC1 and high tumour stage (OR = 2.678, 95% CI: 1.859–3.857, p < .001) (), without obvious heterogeneity (I2 = 45.7%, p = .101). Similarly, 7 studies comprising 622 participants included information on LNM stage and high/low groupings of linc-UBC1 expression. The data displayed a significant positive correlation between high linc-UBC1 expression and LNM occurrence (OR = 2.912, 95% CI: 2.056–4.125, p < .001) (). More importantly, the data showed moderate heterogeneity between the studies (I2 = 43.1%, p: .104), indicating that these data were universal. In conclusion, the relatively high expression of linc-UBC1 in cancer may serve as a prognostic indicator for lymph node metastasis and high tumour stage. The above results are shown in .
Figure 3. Forest plot of the correlation between UBC1 expression and clinicopathological characteristics. (A) Forest plot of the correlation between UBC1 expression and HTS in different cancer patients. (B) Forest plot of the correlation between UBC1 expression and LNM in different cancer patients. HTS, high tumor stage; LNM, lymph node metastasis.
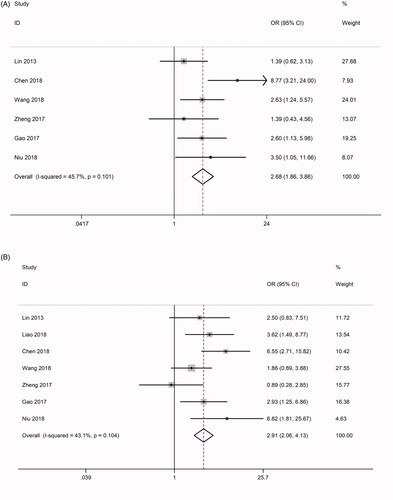
Publication bias and sensitivity analysis between eligible studies
In our study, 10 studies included OS data, so for the OS group, we applied Begg’s test, and the results suggested that there was no significant publication bias in the HR assessment (p = .186 > .10) (). For the TNM and LNM stage groups (7 studies), we used Begg’s test, and the results also found no significant publication bias for TNM and LNM evaluation (PTNM = 1.00 > 0.10, PLNM = 0.872 > 0.10) (). Furthermore, we performed a sensitivity analysis of the included studies applying Stata 12.0 to confirm the stability of the meta-analysis results for linc-UBC1 in the TNM, LNM and OS groups. The results show that the articles involved in all analyses show an overall stable distribution pattern ().
Figure 4. Funnel plot analysis of potential publication bias in the study (Begg’s test): (A) OS group, (B) TNM group, (C) LNM group. OS, overall survival; TNM, tumor node metastasis, LNM, lymph node metastasis.
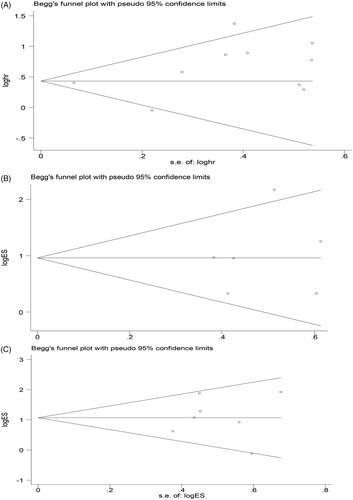
Figure 5. Sensitivity analysis of effect of individual studies: (A) on the pooled HRs for UBC1 and overall survival of patients, (B) on the pooled ORs for UBC1 and TNM stage of patients, (C) on the pooled ORs for UBC1 and LNM of patients. TNM, tumor node metastasis; LNM, lymph node metastasis, HR, hazard ratio.
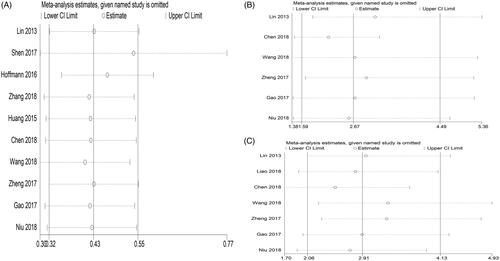
Discussions
Linc-UBC1 is abnormally expressed in various human tumours, promoting tumour metastasis and development. In this current analysis, a new systematic review was performed to reveal the pro-oncogenic role of linc-UBC1 and the association between linc-UBC1 levels and clinical features of different cancers. Our results implied that high expression of Linc-UBC1 was significantly associated with poor OS in cancer groups (p < .001, HR =1.735, 95% 1.348–2.235, random-effects model). Analogously, the data revealed that high expression of Linc-UBC1 was highly correlated with lymph node metastasis (OR = 2.912, 95% CI: 2.056–4.125, p < .001 fixed-effects model) and high tumour stage.
According to the forest plot, the data displayed a close, poor association between linc-UBC1 level and OS with modest heterogeneity. To elucidate the origin of heterogeneity between studies, a subgroup analysis was conducted according to the sample size and cancer type. The results displayed that linc-UBC1 might be used as an independent indicator for OS in various tumours (Supplementary Figure 1). Additionally, no obvious heterogeneity was found in BLC, CRC and LC compared with other cancer types. Similarly, the results also showed that, according to , the incidence of LNM and the prevalence of advanced stage between the high-low linc-UBC1 expression groups were significantly different. Nonetheless, as for the analysis of LNM and TNM, significant heterogeneity between the articles was observed, which may be attributed to the limited number of studies. All in all, these results support that linc-UBC1 acts as a potential predictive biomarker in various cancers.
The present analysis was first used to assess the association between linc-UBC1 level and the prognosis and clinical features of different types of cancer patients and to propose it as a potential target for the early detection of tumours. A similar lncRNA meta-analysis previously revealed a link between the expression of lncRNAs, including UCA1 [Citation27], HOTAIR [Citation28], CRNDE [Citation29], NEAT1 [Citation30], and MALAT1 [Citation31], and associated cancer prognoses. Related studies have shown that lncRNA can transduce exogenous signals into the cytoplasm through cell membranes, affecting cell phenotype, and this process involves multiple signalling pathways [Citation23,Citation32]. Although the underlying mechanism between linc-UBC1 overexpression and adverse tumour outcomes is still unknown, the relevant studies have gradually uncovered its functional pathways. Linc-UBC1 is a multifunctional lncRNA with regulatory effects on target genes, such as ATG7, EZH2 and circRNA [Citation14,Citation19,Citation33]. Linc-UBC1 can regulate onco-proteins to induce cancer development. For instance, Linc-UBC1 exerts its function by physically regulating the PRC2 complex to regulate the histone modification status of the target gene in BLA [Citation11]. Linc-UBC1 could affect cancer progression through integration with microRNAs, such as miR-144 and miR-361 [Citation13,Citation19]. Additionally, Linc-UBC1 regulates the development of cervical cancer by regulating Wnt/β-catenin signalling pathways [Citation16]. Integrated bioinformatics analysis revealed that Linc-UBC1 had distinct value in discriminating CRC from other noncancer diseases [Citation34]. To provide potential guidance for mechanistic research, we systematically reviewed Linc-UBC1 and related molecular targets, pathways and potential noncoding RNA (microRNAs and circRNAs) in this meta-analysis, which provides a reference for exploring the relationship between Linc-UBC1 and cancer ().
Table 4. Summary of linc-UBC1 with their potential targets, pathways and related microRNAs.
Nonetheless, there were several limitations to the present analysis. First, only seven articles showed clinical characteristics of cancers, so these findings need to be further confirmed in a large cohort. Second, some of the included studies, especially in the subgroup analysis, were loosely relevant, so these findings need more evidence to confirm. Third, all included studies divided cut-off values by inconsistent methods; no subgroup analysis was performed to test whether cut-off values were factors affecting the analysis. Fourth, only reports of OS, TNM and LNM studies were selected, and other clinical indexes, such as tumour size and sex, were not included in the study because some clinical values were limited or not of research value, but these can be further explored in the future. Analysis was performed via a large sample analysis. Fifth, most eligible studies come from China, so it is not clear whether our findings are applicable to other countries. To better determine the clinical application, the scope and validation of the samples need to be further investigated in the future.
In brief, linc-UBC1 overexpression is associated with poor OS and advanced tumour stage and could be used as a novel prognostic biomarker in multiple tumours. Thus, combining Linc-UBC1 expression evaluation with regular clinical examinations can provide a potential way to provide targeted prognostic and therapeutic options for different individuals. However, it is strongly advised that large-cohort, well-designed studies are further conducted to validate the precise role of linc-UBC1 in tumour development.
Significance
LncRNA linc-UBC1 was indicated as an important factor in various tumours. This study applied a meta-analysis to quantitatively display the results of related studies. The results showed that high linc-UBC1 expression was strongly associated with the poor outcome of patients, which means it may serve as an important biomarker for clinical therapy and diagnosis.
Author contributions
ZXW, XHB and LDQ collected detailed information on the included articles. ZXW, LMJ, LWH and MWJ analysed the clinical data. ZXW and XHB drafted the manuscript, and LYK provided clinical expertise. LYK designed and supervised the project and established the workflow of the analysis. All authors have read and agreed to the final version of the manuscript.
supplementary_information.docx
Download MS Word (3.7 MB)Acknowledgments
We appreciate and apologise to the participants whose names are not listed on the author list but who joined in this project.
Disclosure statement
No potential conflict of interest was reported by the author(s).
Additional information
Funding
References
- Bray F, Ferlay J, Soerjomataram I, et al. Global cancer statistics 2018: GLOBOCAN estimates of incidence and mortality worldwide for 36 cancers in 185 countries. CA Cancer J Clin. 2018;68(6):394–424.
- Chen W, Zheng R, Baade PD, et al. Cancer statistics in China, 2015. CA Cancer J Clin. 2016;66(2):115–132.
- Siegel RL, Miller KD, Jemal A. Cancer statistics, 2016. CA Cancer J Clin. 2016;66(1):7–30.
- Gupta GP, Massague J. Cancer metastasis: building a framework. Cell. 2006;127(4):679–695.
- O’Sullivan B, Brierley J, Byrd D, et al. The TNM classification of malignant tumours-towards common understanding and reasonable expectations. Lancet Oncol. 2017;18:849–851.
- Leyland-Jones BR, Ambrosone CB, Bartlett J, et al. Recommendations for collection and handling of specimens from group breast cancer clinical trials. J Clin Oncol. 2008;26(34):5638–5644.
- Vanharanta S, Massague J. Origins of metastatic traits. Cancer Cell. 2013;24(4):410–421.
- Vockley JG, Niederhuber JE. Diagnosis and treatment of cancer using genomics. BMJ. 2015;350:h1832.
- Evans JR, Feng FY, Chinnaiyan AM. The bright side of dark matter: lncRNAs in cancer. J Clin Invest. 2016;126(8):2775–2782.
- Batista PJ, Chang HY. Long noncoding RNAs: cellular address codes in development and disease. Cell. 2013;152(6):1298–1307.
- He W, Cai Q, Sun F, et al. linc-UBC1 physically associates with polycomb repressive complex 2 (PRC2) and acts as a negative prognostic factor for lymph node metastasis and survival in bladder cancer. Biochim Biophys Acta. 2013;1832(10):1528–1537.
- Chen X, Dai M, Zhu H, et al. Evaluation on the diagnostic and prognostic values of long non-coding RNA BLACAT1 in common types of human cancer. Mol Cancer. 2017;16(1):160.
- Ye JR, Liu L, Zheng F. Long noncoding RNA bladder cancer associated transcript 1 promotes the proliferation, migration, and invasion of nonsmall cell lung cancer through sponging miR-144. DNA Cell Biol. 2017;36(10):845–852.
- Su J, Zhang E, Han L, et al. Long noncoding RNA BLACAT1 indicates a poor prognosis of colorectal cancer and affects cell proliferation by epigenetically silencing of p15. Cell Death Dis. 2017;8(3):e2665.
- Liao D, Lv G, Wang T, et al. Prognostic value of long non-coding RNA BLACAT1 in patients with papillary thyroid carcinoma. Cancer Cell Int. 2018;18(1):47.
- Wang CH, Li YH, Tian HL, et al. Long non-coding RNA BLACAT1 promotes cell proliferation, migration and invasion in cervical cancer through activation of Wnt/β-catenin signaling pathway. Eur Rev Med Pharmacol Sci. 2018;22(10):3002–3009.
- Aalijahan H, Ghorbian S. Long non-coding RNAs and cervical cancer. Exp Mol Pathol. 2019;106:7–16.
- Gao X, Wen J, Gao P, et al. Overexpression of the long non-coding RNA, linc-UBC1, is associated with poor prognosis and facilitates cell proliferation, migration, and invasion in colorectal cancer. Onco Targets Ther. 2017;10:1017–1026.
- Wu X, Zheng Y, Han B, et al. Long noncoding RNA BLACAT1 modulates ABCB1 to promote oxaliplatin resistance of gastric cancer via sponging miR-361. Biomed Pharmacother. 2018;99:832–838.
- Niu G, Zhuang H, Li B, et al. Long noncoding RNA linc-UBC1 promotes tumor invasion and metastasis by regulating EZH2 and repressing E-cadherin in esophageal squamous cell carcinoma. J Buon. 2018;23:157–162.
- Droop J, Szarvas T, Schulz WA, et al. Diagnostic and prognostic value of long noncoding RNAs as biomarkers in urothelial carcinoma. PLoS One. 2017;12(4):e0176287
- Li D, Yang W, Zhang Y, et al. Genomic analyses based on pulmonary adenocarcinoma in situ reveal early lung cancer signature. BMC Med Genomics. 2018;11(Suppl 5):106
- Huang F-X, Chen H-J, Zheng F-X, et al. LncRNA BLACAT1 is involved in chemoresistance of non-small cell lung cancer cells by regulating autophagy. Int J Oncol. 2019;54(1):339–347.
- Tierney JF, Stewart LA, Ghersi D, et al. Practical methods for incorporating summary time-to-event data into meta-analysis. Trials. 2007;8:16.
- Higgins JP, Thompson SG, Deeks JJ, et al. Measuring inconsistency in meta-analyses. BMJ. 2003;327(7414):557–560.
- Zeng X, Zhang Y, Kwong JSW, et al. The methodological quality assessment tools for preclinical and clinical studies, systematic review and meta-analysis, and clinical practice guideline: a systematic review. J Evid Based Med. 2015;8(1):2–10.
- Gao J, Cao R, Mu H. Long non-coding RNA UCA1 may be a novel diagnostic and predictive biomarker in plasma for early gastric cancer. Int J Clin Exp Path. 2015;8:12936–12942.
- Ma G, Wang Q, Lv C, et al. The prognostic significance of HOTAIR for predicting clinical outcome in patients with digestive system tumors. J Cancer Res Clin Oncol. 2015;141(12):2139–2145.
- Xie H, Ma B, Gao Q, et al. Long non-coding RNA CRNDE in cancer prognosis: review and meta-analysis. Clin Chim Acta. 2018;485:262–271.
- Chen Z, Xie H, Zhong Y, et al. Role of nuclear paraspeckle assembly transcript 1 as a common molecular marker for prognosis in various cancers. Minerva Med. 2017;108(5):477–479.
- Song W, Wang K, Zhang R, et al. Long noncoding RNA MALAT1: a potential novel prognostic biomarkers in cancers based on meta-analysis. Zhong Nan Da Xue Xue Bao Yi Xue Ban. 2016;41:1163–1167.
- Kopp F, Mendell JT. Functional classification and experimental dissection of long noncoding RNAs. Cell. 2018;172(3):393–407.
- Ding X, Zhang S, Li X, et al. Profiling expression of coding genes, long noncoding RNA, and circular RNA in lung adenocarcinoma by ribosomal RNA-depleted RNA sequencing. FEBS Open Bio. 2018;8(4):544–555.
- Dai M, Chen X, Mo S, et al. Meta-signature LncRNAs serve as novel biomarkers for colorectal cancer: integrated bioinformatics analysis, experimental validation and diagnostic evaluation. Sci Rep. 2017;7:46572