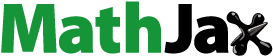
Abstract
Uveal melanoma (UM) is the most frequent primary ocular tumour among adults. Here, we aimed to establish the immune cell-based signature to predict the overall survival (OS) of UM patients. The mRNA profile and matched clinical records of 80 UM patients were downloaded from The Cancer Genome Atlas (TCGA) database. CIBERSORT was used to verify the immune cell types of individuals. The univariate analysis found the CD8+ T cell, monocyte, CD4+ memory T cell (resting) and mast cell (resting) were significantly associated with the OS of UM patients. Subsequently, the LASSO Cox regression test was applied to establish the signature, by which the patients were separated into high- and low-risk subgroups. The Kaplan–Meier analyses found for these patients in the high-risk group had a poor survival rate than those in the low-risk group. The predictive value and stability were confirmed by the receiver operative characteristics curves. Pathway analyses found that the differentially expressed genes between the high- and low-risk subgroups were mainly centralised on immune response-related pathways. Further, the comparison of our signature with clinicopathological records confirmed its superiority and independence. In summary, we established an immune cell-based prognosis-predicting signature for UM patients, which will benefit the individual’s treatment.
Background
Uveal melanoma (UM) demonstrates as a rare subgroup of melanoma, accounting for 3–5% of all melanomas in the USA [Citation1–3]. Although UM is rare, it accounts for 85–95% of all the ocular melanoma cases [Citation4]. About 85% of the tumour cases arise from the choroid, while the remaining cases arise from the iris (3–5%) and ciliary body (5–8%) [Citation4,Citation5]. As studies reported, the median diagnosis age of UM is around 62-year-old, and the peak range is 70–79 years old [Citation4,Citation6,Citation7]. The prevalence rate of males is 30% higher than females [Citation4]. Although the local treatment has been improved for UM patients, the survival rates are rarely improved. In addition, recent advances in genetics promote prognostication and enable to predict these cancer patients with high metastatic potential.
Interestingly, the eye is an immune-privileged region, which is correlated with a set of active immunosuppressive responses. The eye aqueous humour consists of a large number of anti-inflammatory and immunosuppressive cytokines, such as TGF-β and macrophage migration inhibitory factor (MIF) [Citation8–10]. Preclinical studies found that stimulated with interferon-gamma in UM cells increase the expression of PD-L1, which promotes the immune escape by compromising the function of T cells [Citation11]. Meanwhile, studies found that the lymphocytic infiltration is correlated with a poor prognosis of primary UM patients [Citation12,Citation13], while mechanisms of this phenomenon are unknown. Detailly, one study indicated that tumour-related macrophages are also proved to be a poor prognostic marker for primary UM patients [Citation14]. Consistently, Bronkhorst et al. [Citation15] found that a high rate of infiltrated macrophages is associated with chromosome 3 monosomy, which is linked to a worse prognosis. Recent work also indicated that the UM can evade the immune system, and for these evaded patients may be sensitive to immune checkpoint blockade therapy. Nevertheless, although CTLA-4 and PD-1 could succeed in treating cutaneous melanoma, consistent results were not obtained among metastatic UM patients, because most of UM rarely expressed PD-L1[Citation11,Citation16]. Thus, to establish a robust immune signature, which could act as overall survival (OS) predictor for UM patients from the tumour immunology aspect and serve as potential therapeutic targets for immunotherapy is warranted. Here, we utilised the RNA-seq data from The Cancer Genome Atlas (TCGA) database trying to generate and cross-validate the immune cell-based OS predicting signature for UM patients.
Materials and methods
Data acquirement
The 80 UM patients’ mRNA expression data with matched clinical records, such as pathological grade, tumour stage, and overall survival time and status, were downloaded from TCGA databases. For 80 UM patients, 56 were assigned to the training set and 24 patients assigned to the validation set.
CIBERSORT analysis, prognostic signature construction, and receiver operating characteristic (ROC) evaluation
We analysed the cell type ratios of each UM patient by online software, CIBERSORT (https://cibersort.stanford.edu/) in both “Relative” and “Absolute” mode [Citation17]. The output file showed the cell compositions of each UM patient. The univariate Cox regression analysis was firstly performed on all candidate cell types in the training set to screen out the OS-related candidates (p < .05). Then, the multivariate Cox regression analysis was performed to make a proportional hazards model using R packages. The prediction model could be described as the following:
Which could also be described as:
According to the risk score, the patients were divided into high- and low- risk subgroups according to the median cut-off of the risk score. The prognostic manifestation was assessed by ROC curves, which could be used to test the predictive accuracy and sensitivity of the newly established signature.
Kaplan–Meier (K–M) and pathway enrichment analyses
The survival differences between the high- and low-risk groups were calculated by the K–M analyses. In addition, LIMMA analysis was performed to find out the differentially expressed genes (DEGs) between the high- and low-risk groups, with the log2 (fold change) > 1. In addition, Kyoto encyclopaedia of genes and genomes (KEGG), gene set enrichment analysis (GSEA) and gene ontology (GO) analyses were employed to perform the gene enrichment analyses. All the analyses were conducted using R software (Version 3.4.0).
Results
CIBERSORT analysis, prognosis signature construction, and risk classification
We used the online software, CIBERSORT to classify the cell types and ratios within each UM patient. The relative proportion of immune cell subtypes are shown in . In previous TCGA UM study, the authors inferred that a CD8+ T cell infiltrate was present in ∼30% of M3-UM (UM with disomy of chromosome 3) while nearly absent in D3-UM (UM with monosomy of chromosome 3), and almost all tumour samples were infiltrated by leukocyte cells. However, our result (“Absolute” mode of CIBERSORT) shows more samples with infiltrated CD8+ T cell but similar with the previous study, more proportion of M3-UM samples infiltrated CD8+ T cell (34/42 vs. 22/38) and the fraction of CD8 T + cells in M3-UM is significantly more than that in D3-UM (Figure S1A). Besides, we found more infiltrated macrophages in the M3-UM than that in D3-UM, which is consistent with a previous study [Citation18] (Figure S1B).
The Univariate analysis was conducted to verify the connection between the cell types and ratios and OS of UM patients. We found that the CD8+ T cell, monocyte, CD4+ memory T cell (resting) and mast cell (resting) were significantly associated with OS (). Under the LASSO Cox regression analysis, we established a risk signature based on the hazard ratio (HR) and co-efficient (co-ef) of each cell type. The risk formula = −1.028523987 × (CD8+ T cell) − 8.340048643 × [CD4+ memory T cell (resting)] − 14.22510687 × monocyte − 7.376415147 × [mast cell (resting)] (). With the help of this signature, the risk score for each patient in both the training and validation cohorts was obtained. We set the median value of risk scores derived from the training cohort as the cut-off value, after that, these UM patients were assigned into high- and low-risk subgroups.
Figure 2. The establishment of prognosis predicting signature for uveal melanoma (UM) patients. (A) The univariate analysis showed the correlation between immune cell types and ratios and the overall survival of UM patients. (B) The hazard ratio (HR) of the enrolled immune cell type derived by LASSO Cox regression analysis.
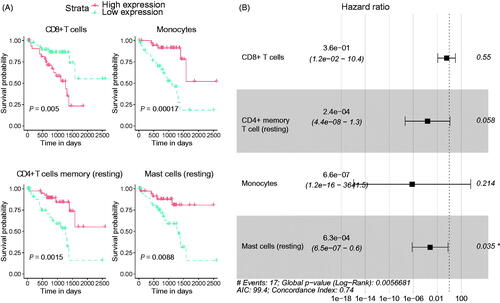
K–M and ROC curve analyses
Based on the risk sub-classification, we used the K–M analysis to verify the survival differences between the high- and low-risk groups. The results confirmed that these patients in the high-risk group mostly had a poor survival rate than those with lower-risk (, p = .00048) in the training set. In addition, the ROC curve proved the stability and sensitivity of the model, with an AUC value of 76.3 (95%CI: 58.3–94.3, (). We further validated the predictive value of this model in the validation cohort, with consistent results obtained, or even better (K–M analysis, p < .001; AUC = 95.4, 95%CI: 85.7–100, ). Taken together, these results confirm the usage and stability of our signature.
Figure 3. The performance of the immune-cell based signature. Kaplan–Meier curves of the high- and low-risk uveal melanoma subgroups divided by the overall survival (OS) predicting signature in the training cohort (A), and validation cohort (B); receiver operating characteristic (ROC) curves of the high- and low-risk groups divided by the OS predicting signature in the training cohort (C), and validation cohort (D).
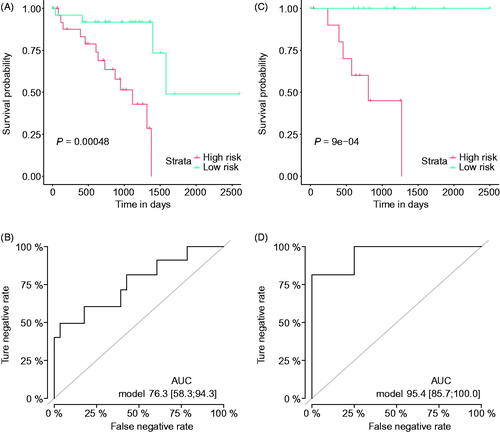
Multivariate and subgroup analyses
To further validate the significance of our signature, we performed the multivariate analysis by pooling the immune-cell based signature with clinicopathological features. The results showed that the immune cell-based signature and new tumour event after initial treatment were still statistically significant (). Besides, the multiline ROC curve showed that our signature had the best predictive value than age, tumour stage, tumour morphology percentage, new tumour event after initial treatment, Chromosome 3 loss, Chromosome 6p gain, Chromosome 8q gain, extrascleral extension, and histological type, with the AUC value of 79.5% (95%CI: 64.9–94.2, ).
Figure 4. Multivariate analyses. (A) The multivariate analyses were performed to test the significance of the signature when pooled with clinicopathological features; (B) Multiline ROC curves showed the superiority of our signature.
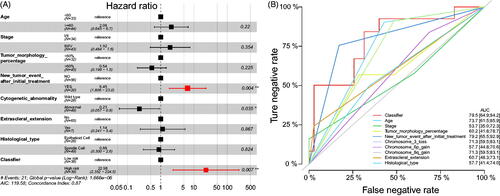
Further, we also analysed whether the signature was suitable for different subgroups, and the results indicated that the signature was able to predict the OS of UM patients with different ages (<60 and > =60), tumour stage (I + II and III + IV), tumour morphology percentage (<60% and > =60%), cytogenetic abnormality (normal and abnormal) subgroups, while it was only significant for new tumour event after initial treatment (No) subgroup and extrascleral extension (No) subgroup ().
Figure 5. Subgroup analyses. The application value of the signature was assessed in different subgroups, including age, new tumour event after initial treatment, tumour stage, tumour morphology percentage, cytogenetic abnormality, and extrascleral extension.
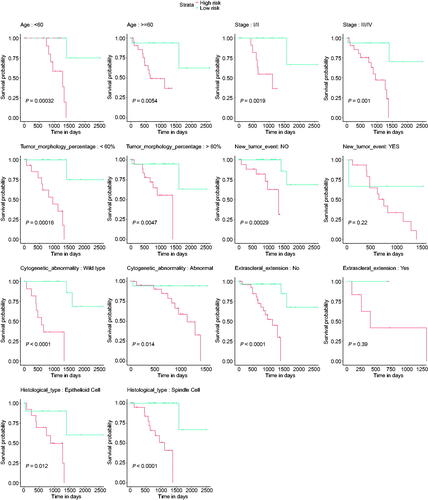
GO, KEGG and GSEA analyses
The principal component analysis (PCA) result was consistent with risk classification (). In addition, we calculated the DEGs between the high- and low-risk groups ( and Tables S1 and S2). We found that 1619 genes were upregulated in the high-risk group compared with the low-risk group, while 736 genes were down-regulated. Based on the DEGs, we did the GO (BP, biological process; CC, cellular component; and MF, molecular function), Reactome and KEGG pathway enrichment analyses (). The GO-BP analysis revealed that the upregulated DEGs were mostly enriched in the regulation of T cell activation, T cell activation, positive regulation of leukocyte activation and so on (). Similar results were uncovered for GO-CC analysis that these genes were mostly enriched in the external side of plasma membrane, immunoglobulin complex, immunoglobulin complex, circulating, MHC class II protein complex and so on (). GO-MF analysis identified that these genes were related to the antigen-binding, cytokine receptor binding, cytokine activity, immunoglobulin receptor binding and so on (). Moreover, results revealed by Reactome and KEGG analyses also confirmed these findings obtained above that these DEGs were significantly enriched in the immune system function related pathways (). Besides, for these downregulated genes, we obtained highly similar results, described in Figure S2. Moreover, the GSEA analysis was employed to assess the differences between high- and low-risk groups, with consistent results obtained (). Taken together, our results suggest that immune-related processes may involve in the tumorigenesis or progression of UM finally influencing individuals’ survival outcomes.
Figure 6. Differentially expressed genes (DEGs) between high- and low-risk uveal melanoma groups. (A) Principal Component Analysis (PCA) successfully separated the high- and low-risk uveal melanoma patients. (B) Volcano plot displayed the DEGs between high- and low-risk groups of uveal melanoma patients.
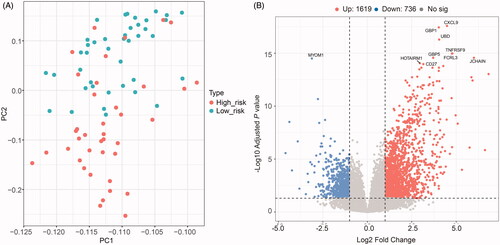
Discussion
In this study, we deconvoluted the relative proportions of infiltrated immune cell types in UM and established an immune cell-based signature for the prognosis predicting of UM patients. The model is validated more effectively than other individual clinicopathological features, as well as genetic predictors and is appropriate for the majority of clinical subgroups. We compared the gene expression profile between the inferred groups with different survival risks and found that cytokine/cytokine receptor activation pathways and other inflammation-related pathways were upregulated in the high-risk group, highlighting their key biological implications in UM progression.
Although surgery or radiotherapy achieves perfect rates of control for these patients with localised diseases, approximately 50% of the UM patients develop metastases 15 years after initial diagnosis [Citation1,Citation19–22]. The liver is the most frequently affected organ, followed by lung, skin/soft tissues and the bone [Citation23–26]. In a recent study, Kuk et al. [Citation24] analysed 286 UM patients, who were diagnosed with distant metastasis between 2000 and 2013, and the median OS was only 13.4 months. In the TCGA cohort we used, 14 patients were diagnosed with metastasis after surgery, of them, 11 patients were assigned into the high-risk group inferred by our immune cell-based OS predicting model. This result supports that the infiltrated immune components, as well as cytokine-related inflammation, influence the metastatic potential of the UM. However, the mechanisms of why/how these inflammatory cells infiltrate to the primary UM and the reason for inconsistent clinical outcomes remain suppositional. Accumulating evidence shows that tumour-microenvironment crosstalk induces malignant cells to mediate inflammatory responses. Notably, it is possible that the primary UM has already metastasised and been exposed to the immune system, as the route of metastasis is purely haematologic (i.e. no exposure to local lymph nodes). Our findings support that the presence of an inflammatory transcriptome in the primary UM is a “risk factor” for metastases. The tumour likely has already metastasised thus stimulating an immune response and those immune cells circulate back to the primary tumour. Further, since the liver is the most affected organ, studies indicated that the liver expresses a high level of CXCL12, a kind of cytokine, which is the ligand of CXCR4, while the CXCR4 is identified upregulated in human UM cells. The interaction between CXCL12 and CXCR4 increases the coincidence that the circulating tumour cells form metastases in the liver. One functional study showed that UM cells respond chemotactically to the stimulation of soluble factors generated by liver cells and the chemotactic response is suppressed by anti-CXCR4 antibody in vitro [Citation27]. In addition, a recent study suggested that about 60% of the primary UM patients express CXCR4 protein, and the most intense CXCR4 protein expression is found on epithelioid melanoma cells, which is closely correlated with malignancy [Citation28]. Consistently, we found that for these UM patients in high-risk subgroup have elevated expression of cytokine-related receptors, such as CCR1-8, CCRL2, CXCR3, CXCR6, IL1R2, IL21R, IL2RA, etc., compared with low-risk subgroup, supporting the chemotaxis hypothesis. Interestingly, the study also found that the expression of CXCR4 and CCR7 on primary UM cells is reduced after immigrating in the liver [Citation27]. Thus, retention of UM cells in the liver is not only related to chemokine gradient mediated chemotaxis but also due to the loss of chemokine receptors once the UM cells reach in the liver. Here, we draw a cartoon to describe the potential mechanisms of how these immune cells or immune reaction influences on UM cell metastases (Figure S3).
To sum up, our study identifies and validates an immune cell-based OS predicting signature for UM patients, which will benefit the treatment decision. The cytokine-related inflammation is a key biological event during UM progression and metastasis, which holds the potential to be an efficiency target of UM therapy.
Author contribution
Conceived and designed the study: Y.N., Z.Z. and S.Z. Performed the literature search and data extraction: Y.N., Z.Z., G.C. and Y.W. Analysed the data: Z.Z., Y.W. and M.P. Drafted the manuscript: Y.N., Z.Z. and S.Z.
191012-R2-Supplementary_tables.xls
Download MS Excel (395.5 KB)Newly_added-Figure_S3.pdf
Download PDF (1.5 MB)Figure_S2.pdf
Download PDF (429 KB)Figure_S1.pdf
Download PDF (305.5 KB)Disclosure statement
No potential conflict of interest was reported by the author(s).
References
- Aronow ME, Topham AK, Singh AD. Uveal melanoma: 5-year update on incidence, treatment, and survival (SEER 1973-2013). Ocul Oncol Pathol. 2018;4(3):145–151.
- Singh AD, Turell ME, Topham AK. Uveal melanoma: trends in incidence, treatment, and survival. Ophthalmology. 2011;118(9):1881–1885.
- Chang AE, Karnell LH, Menck HR. The National Cancer Data Base report on cutaneous and noncutaneous melanoma: a summary of 84,836 cases from the past decade. The American College of Surgeons Commission on Cancer and the American Cancer Society. Cancer. 1998;83(8):1664–1678.
- McLaughlin CC, Wu XC, Jemal A, et al. Incidence of noncutaneous melanomas in the U.S. Cancer. 2005;103(5):1000–1007.
- Damato B. Progress in the management of patients with uveal melanoma. The 2012 Ashton Lecture. Eye (Lond). 2012;26(9):1157–1172.
- Damato EM, Damato BE. Detection and time to treatment of uveal melanoma in the United Kingdom: an evaluation of 2,384 patients. Ophthalmology. 2012;119(8):1582–1589.
- Andreoli MT, Mieler WF, Leiderman YI. Epidemiological trends in uveal melanoma. Br J Ophthalmol. 2015;99(11):1550–1553.
- Niederkorn JY. Ocular immune privilege and ocular melanoma: parallel universes or immunological plagiarism? Front Immunol. 2012;3:148.
- Apte RS, Niederkorn JY. Isolation and characterization of a unique natural killer cell inhibitory factor present in the anterior chamber of the eye. J Immunol. 1996;156(8):2667–2673.
- Apte RS, Mayhew E, Niederkorn JY. Local inhibition of natural killer cell activity promotes the progressive growth of intraocular tumors. Invest Ophthalmol Vis Sci. 1997;38(6):1277–1282.
- Yang W, Chen PW, Li H, et al. PD-L1: PD-1 interaction contributes to the functional suppression of T-cell responses to human uveal melanoma cells in vitro. Invest Ophthalmol Vis Sci. 2008;49(6):2518–2525.
- de la Cruz PO, Jr., Specht CS, McLean IW. Lymphocytic infiltration in uveal malignant melanoma. Cancer. 1990;65(1):112–115.
- Whelchel JC, Farah SE, McLean IW, et al. Immunohistochemistry of infiltrating lymphocytes in uveal malignant melanoma. Invest Ophthalmol Vis Sci. 1993;34(8):2603–2606.
- Makitie T, Summanen P, Tarkkanen A, et al. Tumor-infiltrating macrophages (CD68(+) cells) and prognosis in malignant uveal melanoma. Invest Ophthalmol Vis Sci. 2001;42(7):1414–1421.
- Bronkhorst IH, Ly LV, Jordanova ES, et al. Detection of M2-macrophages in uveal melanoma and relation with survival. Invest Ophthalmol Vis Sci. 2011;52(2):643–650. .
- Kaunitz GJ, Cottrell TR, Lilo M, et al. Melanoma subtypes demonstrate distinct PD-L1 expression profiles. Lab Invest. 2017;97(9):1063–1071.
- Newman AM, Liu CL, Green MR, et al. Robust enumeration of cell subsets from tissue expression profiles. Nat Methods. 2015;12(5):453–457.
- Maat W, Ly LV, Jordanova ES, et al. Monosomy of chromosome 3 and an inflammatory phenotype occur together in uveal melanoma. Invest Ophthalmol Vis Sci. 2008;49(2):505–510.
- Rietschel P, Panageas KS, Hanlon C, et al. Variates of survival in metastatic uveal melanoma. J Clin Oncol. 2005;23(31):8076–8080.
- Souto EB, Zielinska A, Luis M, et al. Uveal melanoma: physiopathology and new in situ-specific therapies. Cancer Chemother Pharmacol. 2019;84(1):15–32.
- Mahendraraj K, Lau CS, Lee I, et al. Trends in incidence, survival, sand management of uveal melanoma: a population-based study of 7,516 patients from the Surveillance, Epidemiology, and End Results database (1973-2012). OPTH. 2016;10:2113–2119.
- Krantz BA, Dave N, Komatsubara KM, et al. Uveal melanoma: epidemiology, etiology, and treatment of primary disease. Clin Ophthalmol. 2017;11:279–289.
- Everett L, Copperman T. Metastatic uveal melanoma. N Engl J Med. 2019;380(19):1853.
- Kuk D, Shoushtari AN, Barker CA, et al. Prognosis of mucosal, uveal, acral, nonacral cutaneous, and unknown primary melanoma from the time of first metastasis. Oncologist. 2016;21(7):848–854.
- Chen DS, Mellman I. Elements of cancer immunity and the cancer-immune set point. Nature. 2017;541(7637):321–330.
- Abdel-Wahab N, Safa H, Abudayyeh A, et al. Checkpoint inhibitor therapy for cancer in solid organ transplantation recipients: an institutional experience and a systematic review of the literature. J Immunother Cancer. 2019;7(1):106.
- Li H, Alizadeh H, Niederkorn JY. Differential expression of chemokine receptors on uveal melanoma cells and their metastases. Invest Ophthalmol Vis Sci. 2008;49(2):636–643.
- Scala S, Ierano C, Ottaiano A, et al. CXC chemokine receptor 4 is expressed in uveal malignant melanoma and correlates with the epithelioid-mixed cell type. Cancer Immunol Immunother. 2007;56(10):1589–1595.