Abstract
Atherosclerosis is a systemic disease associated with inflammatory cell infiltration and activation of immune-related pathways. In our study, we aimed to uncover immune-related changes and explore novel immunological features in the development of carotid atherosclerotic plaques. First, we applied integrated bioinformatics methods, including CIBERSORT and gene set enrichment analysis (GSEA). The gene expression matrices GSE28829, GSE41571, and GSE43292 were obtained from the Gene Expression Omnibus (GEO) dataset. After a series of data pre-processing steps, the resulting combined expression matrices were analysed using the CIBERSORT, GSEA, and Cluster Profiler packages. After the comparison and analysis between the carotid atherosclerotic plaques in the early and advanced stages, we discovered that there is a higher percentage of activated memory CD4 T cells and a lower percentage of resting memory CD4 cells in advanced-stage plaques. Moreover, activation of memory CD4 T cells can promote the development of carotid atherosclerotic plaques. Additionally, FOXP3+ Treg cell maturation can also participate in the progression of carotid plaques.
Background
Carotid artery disease, caused by a buildup of atherosclerotic plaque inside the arterial wall, is one of the most important causes of cerebrovascular disease [Citation1]. During carotid plaque formation, early-stage lesions may be asymptomatic, but the intermediate and advanced stages are more severe and comparatively more likely to rupture, leading to thrombus formation in the carotid artery, a frequent cause of ischaemic cerebrovascular events [Citation2]. Many factors, such as oxidative stress and inflammation, have been found to participate in the progression from early to advanced stage plaque [Citation3]. The infiltration of inflammatory cells and activation of immune-related pathways are critical features in plaque progression [Citation4]. Several types of immune cells, such as lymphocytes [Citation5], natural killer T cells [Citation5], and T cells [Citation6,Citation7] are involved in plaque progression. In addition, inflammatory cytokines such as TNF-α, IFN-γ, IL-1β, IL-6, and IL-8 have been reported to play a critical role in the development of plaques [Citation6]. However, the changes in immune cell types from the early to advanced plaque stages are still unclear. In addition, the underlying mechanisms regulating the development of carotid atherosclerotic plaques are still unclear.
Many researchers have attempted to illustrate this progression. For instance, leukocyte cathepsin C was shown to promote atherosclerotic lesion progression by selective tuning of innate and adaptive immune responses [Citation8]. Moreover, PELATON, as a monocyte- and macrophage-specific lncRNA, was also proven to have an important role in plaque progression [Citation9]. The critical role of particular immune cells and immune-related pathways in the progression of carotid atherosclerotic plaques was confirmed by these molecular and cellular experiments, but fewer studies have been conducted to explore the correlation of genes and immune cells or overall landscape in progression-related big data. Hence, an overall description of the immune-related landscape of the early stage and advanced stage should be conducted.
In recent years, advances in gene chip technology have enabled the analysis of changes in mRNA levels between different samples, which has helped identify novel and important genes when studying disease mechanisms. However, traditional microarray analysis is often restricted to differentially expressed genes (DEGs). Since carotid artery disease is closely associated with immune cells and the inflammatory system, a more comprehensive bioinformatics analysis of immune-related pathways should be applied. In this study, two microarray datasets downloaded from the Gene Expression Omnibus (GEO) were used to screen for enrichment in immune-related pathways and differently infiltrated immune cells. Gene Set Enrichment Analysis (GSEA) was used to analyse enriched gene sets, Kyoto Encyclopaedia of Genes and Genomes (KEGG) pathways, and immunological signatures account for all expression data rather than DEGs alone. Finally, CIBERSORT was used to reconstruct the state of immune cell infiltration in carotid atherosclerotic plaques at different stages.
Through an in-depth analysis of expression array datasets taken from plaque samples in the early and advanced stages, our study aimed to explore the immunological mechanism of carotid atherosclerotic plaques comprehensively, and identify new immunological targets for preventing the progression of carotid atherosclerotic plaques.
Materials and methods
Microarray datasets
In this study, the raw gene expression profiles GSE28829, GSE41571, and GSE43292 were downloaded from the GEO database (https://www.ncbi.nlm.nih.gov/geo/). The GSE28829 expression profile consists of 16 advanced and 13 early-stage plaques, detected by the Affymetrix Human Genome U133 Plus 2.0 Array. The GSE41571 expression profile consists of six advanced and five early-stage plaques, detected by the Affymetrix Human Genome U133 Plus 2.0 Array. The GSE43292 dataset consists of 32 advanced and 32 early-stage carotid atherosclerotic plaques detected by the Affymetrix Human Gene 1.0 ST Array. Carotid atherosclerotic plaque in the advanced stage is defined as a plaque with a fibrous cap, whereas carotid atherosclerotic plaque in the early stage is identified by intimal thickening and intimal xanthoma [Citation10].
Data pre-processing
To obtain the critical information from the two gene expression matrices, the merging and pre-processing of raw data were conducted using a Perl script and the sva package [Citation11] from R software (R-project.org). Then, the probe ID for every gene was converted to a gene symbol using a Perl script. If a gene symbol corresponded to multiple probe IDs, the mean expression level of the probes was calculated as the representative expression level of that gene.
CIBERSORT
Immune cell infiltration in carotid plaques was evaluated using the CIBERSORT algorithm [Citation12]. CIBERSORT is an analysis tool that uses gene expression data to represent the cell composition of complex tissues based on pre-processed gene expression profiles. The LM22 gene file of CIBERSORT was used to define 22 immune cell subsets and analyse early-stage plaque tissue and advanced stage plaque tissue data, which were obtained from the CIBERSORT web portal (http://CIBERSORT.stanford.edu/).
As described in a previous study, p-values and root mean squared errors were counted for each expression file in CIBERSORT. This default signature matrix of 100 permutations was used in this algorithm. Only data with a CIBERSORT p value < .05 was filtered and reserved for the following analysis. Thus, the output was directly integrated to generate an entire matrix of immune cell fractions. The visualisation of the results from CIBERSORT was carried out using the R packages, corplot, vioplot, and ggplot2 [Citation13].
Gene set enrichment analysis (GSEA)
After data pre-processing, the enrichment analyses for Gene Ontology (GO) terms, KEGG pathways, and immunologic signature gene sets were analysed with the GSEA software [Citation14], and the merged gene matrices were uploaded to the software. The number of permutations was set to 1000, and the permutation type was set as “phenotype”. In addition, gene set databases used during this analysis were downloaded from the Molecular Signatures Database (http://software.broadinstitute.org/gsea/msigdb/index.jsp) [Citation15].
Detection and functional enrichment analysis of DEGs
The DEGs between advanced stage samples and early stage samples were determined using the limma package in R [Citation16]. The thresholds were log2 (fold change)>1 and adjusted p value < .05. DEG visualisation was conducted using a heatmap using the pheatmap package [Citation17].
The Cluster Profiler R package [Citation18] was used to functionally analyse the enriched pathways of DEGs in GO terms and KEGG pathways. The p value was corrected using the Benjamini method or false discovery rate (FDR) for multiple testing calibrations. The threshold was set at p < .05. The results were visualised using the ggplot2 package [Citation13].
Principal component analysis (PCA) of infiltrated immune cells
PCA is a multivariate regression analysis algorithm, which we used to define the differences in infiltrated immune cells between two different groups [Citation19,Citation20]. The PCA graph was rendered using the ggplot2 package in the R software [Citation13]. The PCA graph could classify infiltrating immune cells as variables and describe the difference between carotid atherosclerotic plaque samples in the early and advanced stages.
Results
Composition of infiltrated immune cells in early and advanced plaque tissues from the GEO expression array dataset
The fraction of infiltrated immune cells was first investigated between plaque tissues in early and advanced stages using GEO expression array data. From a total of 104 samples, 47 early and 53 advanced samples were eligible for CIBERSORT (p < .05). As shown in , the fraction of immune cells varied significantly among the samples and groups. M2 macrophages, M0 macrophages, naive B cells, CD8 T cells, and resting CD4 memory T cells represented the top five highest-infiltrating fractions in carotid atherosclerotic plaques. Inversely, CD4 naive T cells and eosinophils were present in lower quantities. Meanwhile, compared with early plaque tissues, advanced tissues generally contained a higher fraction of CD4 memory-activated T cells and M0 macrophages (p value <.05). However, advanced plaques contained a lower fraction of CD8 T cells, CD4 memory resting T cells, T cell regulatory (Tregs), activated natural killer (NK) cells, monocytes, and resting dendritic cells (p value <.05) ().
Figure 1. The fraction of 22 subsets of immune cells in carotid atherosclerotic plaque from early stage and advanced stage. (A) Early stage plaques; (B) advanced stage plaques. X axis: each GEO sample; Y axis: percentage of each kind of immune cells.
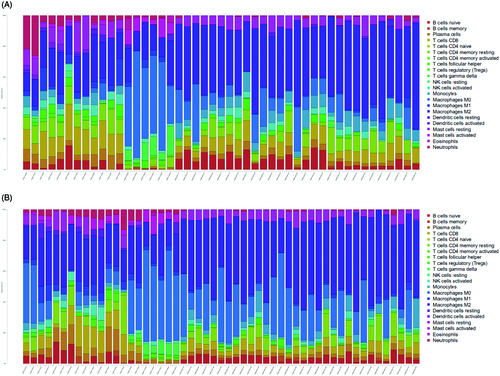
Figure 2. The violin graph shows the difference of immune infiltration between early stage plaques and advanced stage plaques. The early stage plaque group is shown in blue and advanced stage plaque group is shown in red. p-Value < .05.
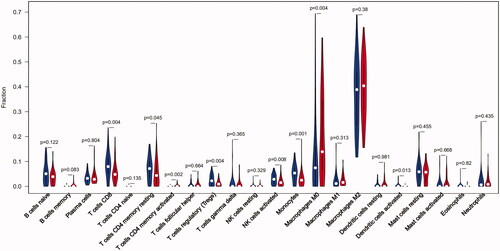
Additionally, we conducted a correlation analysis of infiltrated immune cells in plaques and found multiple pairs of positively and negatively related immune cells (). The score represents the degree of correlation. This result suggests that activated mast cells and follicular helper T cells showed the most synergistic effect. Meanwhile, CD8 T cells and M0 macrophages showed the most competitive effect.
Figure 3. The co-expression patterns among fractions of immune cells. Red: positive correlation; blue: negative correlation.
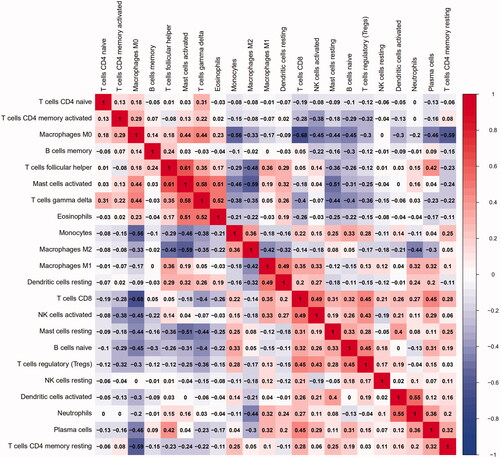
The PCA results showed that the fractions of immune infiltration could help us to distinguish advanced plaques from early plaques ().
Kegg pathway and GO analysis showed high enrichment of the immunological pathway and gene sets
In order to explore the possible pathways and gene sets associated with immune functions, all expression data were divided into plaques in the early and advanced stages and then subjected to GSEA analysis. The exported results showed a high correlation with immune cells and immune-related functions, as expected.
Compared with early-stage plaques, the top 20 KEGG pathways in advanced stage plaques were mainly enriched in primary immune-related pathways such as the Toll-like receptor signalling pathway, antigen processing and presentation, natural killer cell-mediated cytotoxicity, and primary immunodeficiency; pathways associated with immune-related diseases such as autoimmune thyroid disease, asthma, graft versus host disease, and systemic lupus erythematosus; pathways associated with cytokine activation, including the chemokine signalling pathway and cytokine-cytokine receptor interaction (). Ten representative immune-related pathways are visualised in .
Figure 5. Results of GO and KEGG from GSEA. (A and B) 10 representative enriched immune-related GO gene sets; (C and D) 10 representative enriched immune-related KEGG pathways. GSEA: Gene set enrichment analysis; GO: gene ontology; KEGG: Kyoto Encyclopaedia of Genes and Genomes.
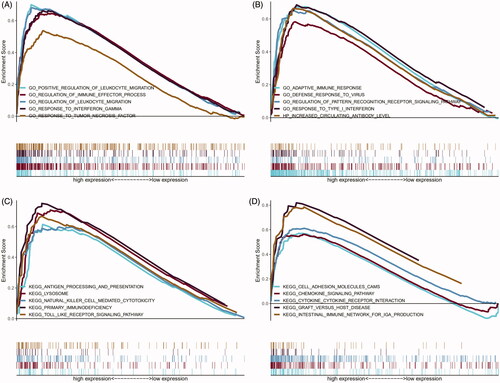
Table 1. Top 20 significant KEGG pathways enriched by DEGs in GSEA, p < .05.
To explore the immune-related gene function in the progression of carotid plaques, GO analysis was conducted using GSEA. The top 20 results of GO analysis showed that variations in gene function between the early and advanced plaques were mainly enriched in interferon-associated gene sets, including response to type I interferon and response to interferon gamma. The functions of inflammatory cells consisted of regulation of leukocyte migration and positive regulation of leukocyte migration as well as other immune response-related gene sets (). Ten representative immune-related GO terms are shown in .
Table 2. Top 20 significant GO terms enriched by DEGs in GSEA, p < .05.
Moreover, the GO and KEGG analyses based on DEGs between plaques in the early and advanced stages showed results similar to those of GSEA ( and ). Gene Ontology was mainly enriched in the regulation of inflammatory response, regulation of immune effector process, regulation of leukocyte degranulation, regulation of myeloid leukocyte-mediated immunity, leukocyte migration, and positive regulation of response to external stimuli. The KEGG pathway was mainly enriched in pertussis, Staphylococcus aureus infection, the PPAR signalling pathway, complement and coagulation cascades, and phagosome and viral protein interaction with cytokines and cytokine receptors.
Figure 6. Heatmap of all DEGs between early stage and advanced stage plaques. Each column represents a carotid atherosclerotic plaque sample, and each row represents a DEG. The gradual colour change from blue to orange indicates the changing process from downregulation to upregulation. DEGs: differentially expressed genes.
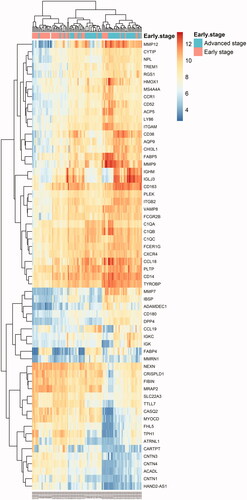
The immunological signature enrichment analysis by GSEA shows that maturation of FOXP3+ treg cells participate in the progress of atherosclerotic plaque
To characterise the in-depth signature of immunological function associated with the progression of atherosclerotic plaque, GSEA was also used to obtain the biological processes enriched in immunologic signature gene sets. All expression data were imported into GSEA. Then, 1886 functional gene sets were enriched (FDR <25%; nominal p value < 1%). The gene sets in CD8 T cells, peripheral blood mononuclear cells (PBMC), FOX3P+ Treg cells, dendritic cells (DCs), and monocytes were mainly enriched. The top 20 enriched gene sets are listed in .
Table 3. Top 20 significant immunological signature enriched by DEGs in GSEA, p < .05.
Besides the gene sets associated with Foxp3+ Treg, different maturity subsets were significantly enriched, including GSE42021 CD24hi vs. CD24low Tconv thymus down; GSE42021 Treg PLN vs. CD24int Treg Thymus down; GSE42021 Treg PLN vs. CD24lo Treg thymus down and GSE42021 Treg PLN vs. CD24lo Treg thymus down, which indicates that the process of the maturation of FOXP3+ Treg cells could promote the deterioration of atherosclerotic plaque ().
Discussion
Carotid atherosclerotic plaques are critical for the progression of cerebrovascular disease. Carotid atherosclerotic plaques at an early stage can become advanced and unstable and provoke a cerebrovascular event within several years [Citation21]. However, the exact mechanism involved in plaque progression is not known, but it involves immune cells and immune-related pathways [Citation22]. Hence, understanding the molecular mechanism of carotid artery atherosclerosis development is critically important for the diagnosis and therapy of cerebrovascular disease.
Since the advent of microarrays, they have provided thousands of gene expression datasets. Microarrays have been widely used to predict the underlying targets for the prevention and therapy of carotid atherosclerotic plaques. In this study, infiltrating immune cells and their immunological gene functions and pathways in plaque carotid artery tissues were analysed using the gene matrices GSE28829, GSE41571, and GSE43292 obtained from the GEO dataset. Only inflammation-related atherosclerotic carotid plaque datasets were included. According to the pathological morphological staging method of the American College of Cardiology, the early-stage lesions were characterised by scattered macrophage foam cells, fatty streaks, intimal thickness, and resident stability. However, advanced plaques are characterised by deep ulceration, disruptions of the lesion surface, haematoma or haemorrhage, and thrombotic deposits [Citation23]. The results from CIBERSORT showed that the infiltrated immune cell subsets were similar in early and advanced plaques. M2 macrophages, M0 macrophages, naive B cells, CD8 T cells, and CD4 memory resting T cells represented the top five infiltrating fractions in carotid artery atherosclerosis. Inversely, there were fewer CD4 naive cells and eosinophils. The results of the composition of carotid atherosclerotic plaques from CIBERSORT is consistent with previous studies that used flow cytometry and single cell sequencing [Citation24–27]. Single cell sequencing of human carotid atherosclerotic plaques in a mouse atherosclerosis model showed that T cells and macrophages dominate the immune landscape of atherosclerotic plaques.
Atherosclerosis is an inflammatory disease. Plaque progression is primarily mediated by cells of the monocyte/macrophage lineage. However, the contribution of other immune cells is still unknown. The differences between early and advanced stage plaques could reveal the immune cells participating in the progression of carotid atherosclerotic plaques. The present analysis shows a higher fraction of activated CD4 memory T cells and a decreased fraction of CD8 T cells, resting CD4 memory resting T cells, Tregs, activated NK cells and monocytes and resting dendritic cells are the main immune cell composition changes that occur during the progression of carotid plaques. Multiple studies have shown that the monocyte-macrophage system contributes to atherosclerosis development, thereby uncovering important molecular mechanisms [Citation28,Citation29]. However, previous studies have rarely focussed on the changes in the number and contribution of other immune cells such as memory CD4 T cells and NK cells.
Several experimental studies have shown that T cells play a critical role in the immune response observed during the process of atherogenesis [Citation30,Citation31]. In the artery, cholesterol accumulation followed by vascular inflammation promotes adaptive T cell response [Citation32] and causes the differentiation of naive CD4+ T cells to effector or memory cells of specialised T cell subsets in secondary lymphoid organs and in the chemokine-driven recruitment of specific T cell and monocyte subsets in atherosclerotic plaques [Citation33–35]. During atherogenesis, memory CD4 T cells especially experience expansion and antigen-experienced T cells, which could result from antigen reexposure and further elicit stronger and more sustained immune response [Citation36]. However, their clinical confirmation in humans remains limited. Our study provided clinical evidence of the potential role of memory CD4 T cells activation in the progression of plaques.
Previous studies have shown that immature dendritic cells already exist under the endothelium in healthy arteries. However, the majority of dendritic cells in advanced plaques seem to be activated and rapidly expand during the progression of atherosclerotic lesions. In the sub-endothelial space of the aorta, dendritic cells can efficiently accumulate lipids and therefore contribute to the initiation and further progression of the disease [Citation37,Citation38]. Moreover, activated dendritic cells can produce a number of pro-inflammatory cytokines [Citation39]. As previous studies have shown, CD8 T cells played a similar role in the development of atherogenesis, as they significantly increase as human lesions progress and become vulnerable to rupture but show a decrease in healed plaque ruptures and fibrotic calcified plaques [Citation40]. However, our study showed inconsistent results with previous studies on the percentage of dendritic cells and CD8 T cells, which may be explained by the following reason: a more significant change in other immune cells, such as M0 macrophages, decreased the relative percentage of these kinds of cells.
NK cells are a critical part of the innate immune system. NK cells reside in peripheral lymphoid organs and develop independently of the thymus. NK cell activity may be activated through stimulation by lipid antigens presented by the MHC-I-like molecule CD1d [Citation41]. Previous studies have suggested that the number of circulating NK cells in patients is related to serious atherosclerosis [Citation42]. In addition, upregulation of NKG2C+ NK cells in peripheral blood is related to a higher risk of plaque rupture in patients with cytomegalovirus infections [Citation43]. However, studies exploring the accurate function of NK cells in atherosclerosis have been complicated by the lack of reasonable animal models. LDLR-/- mice with defective NK cells generated by transferring bone marrow from transgenic mice overexpressing Ly49A under the control of a granzyme A promoter showed reduced atherosclerosis, showing the morbigenous role of NK cells in atherosclerosis [Citation44]. Through the use of NK cell-depleting antibodies, we found that NK cells could aggravate atherosclerosis. Perforin and granzyme B-mediated by transferring NK cells deficient in perforin or granzyme B into lymphocyte-deficient ApoE-/- mice could be their main cytolytic mechanism [Citation45]. Strangely, NK cell activation was decreased in advanced plaques, which may indicate that NK cells play a different role in advanced atherosclerotic plaques.
In addition, our results () also show the synergistic correlation between activated mast cells and follicular helper T cells as well as a competitive correlation between CD8 T cells and M0 macrophages. However, few studies have reported a possible mechanism for these relationships. We speculate that activated mast cells and follicular helper T cells can mutually enhance their effects in this process. However further investigation into the correlation between these immune cells needs to be conducted.
Functional annotation demonstrated that the DEGs were mainly involved in immune-related functions. The KEGG pathways from all expression array data were mostly enriched in immune and inflammatory response-related pathways, including primary immune-related, with immune disease-related, and cytokine activation-associated pathways. Simultaneously, the enrichment of GO terms showed that variations in gene sets between the early and advanced plaques were mainly enriched in interferon-associated, cellular response to interferon gamma, and functions of inflammatory cells and other immune-related gene sets. Moreover, the functional annotation based on DEGs between the advanced and early-stage plaques showed results similar to those of GSEA. A series of studies have reported that the activation of primary immune cells and the production and release of a mass of cytokines and complements, including interferon gamma, play critical roles in all stages of atherosclerotic plaques. However, other pathways and biological processes, such as pathways associated with immune-related diseases, have not been reported frequently in the progression of plaques. For these reasons, we speculate that these pathways could have an underlying critical role in the development of carotid atherosclerotic plaques.
Unlike CIBERSORT, immunological signature enrichment analysis was used to explore the function rather than the composition of immune cells in the development of carotid atherosclerotic plaques. The immunological signature enrichment analysis conducted by GSEA showed that gene sets in CD8 T cells, PBMCs, Foxp+ Treg cells, DCs, and monocytes were mainly enriched, which may indicate that the functions of these kinds of cells were changed significantly, and may regulate the progression of plaques. More importantly, four gene sets associated with Foxp3+ Treg subsets of different maturity were significantly enriched, which suggests that they could play different roles in the progression of carotid atherosclerotic plaque. The forkhead box transcription factor FOXP3 has been identified as the key lineage marker and master switch in the regulation of Treg development and function [Citation46]. FOXP3 is now accepted as the “gold standard” for defining thymic-derived Tregs [Citation47]. Previous studies have shown that a low percentage of Foxp3+ regulatory T cells was detectable in all progressive stages of human atherosclerotic lesions and proved their protective effects in atherosclerotic lesions. However, the exact Foxp3+ Treg subsets should be researched in greater depth. Our study provides a new perspective on Foxp3+ Treg subsets of different maturity.
Conclusions
To conclude, we described the immune landscape in detail, revealing the underlying immune infiltration patterns of carotid atherosclerotic plaques at different stages. Our work advances the understanding of the immune response and provides valuable insight into the immune mechanism of the progression of carotid atherosclerotic plaque.
Disclosure statement
No potential conflict of interest was reported by the author(s).
Data availability statement
All raw data are available in GEO datasets (GSE28829, GSE41571, and GSE43292).
Correction Statement
This article has been republished with minor changes. These changes do not impact the academic content of the article.
Additional information
Funding
References
- Spence JD. Intensive risk factor control in stroke prevention. F1000Prime Rep. 2013;5:42.
- Narula J, Nakano M, Virmani R, et al. Histopathologic characteristics of atherosclerotic coronary disease and implications of the findings for the invasive and noninvasive detection of vulnerable plaques. J Am Coll Cardiol. 2013;61(10):1041–1051.
- Mury P, Chirico EN, Mura M, et al. Oxidative stress and inflammation, key targets of atherosclerotic plaque progression and vulnerability: potential impact of physical activity. Sports Med. 2018;48(12):2725–2741.
- Ammirati E, Moroni F, Norata GD, et al. Markers of inflammation associated with plaque progression and instability in patients with carotid atherosclerosis. Mediators Inflamm. 2015;2015:718329
- van Puijvelde GH, van Wanrooij EJ, Hauer AD, et al. Effect of natural killer T cell activation on the initiation of atherosclerosis. Thromb Haemost. 2009;102(08):223–230.
- Oliveira RT, Silva RM, Teo FH, et al. Detection of TCD4+ subsets in human carotid atheroma. Cytokine. 2013;62(1):131–140.
- Olofsson PS, Soderstrom LA, Wagsater D, et al. CD137 is expressed in human atherosclerosis and promotes development of plaque inflammation in hypercholesterolemic mice. Circulation. 2008;117(10):1292–1301.
- Herias V, Biessen EA, Beckers C, et al. Leukocyte cathepsin C deficiency attenuates atherosclerotic lesion progression by selective tuning of innate and adaptive immune responses. Arterioscler Thromb Vasc Biol. 2015;35(1):79–86.
- Hung J, Scanlon JP, Mahmoud AD, et al. Novel Plaque Enriched Long Noncoding RNA in Atherosclerotic Macrophage Regulation (PELATON). Arterioscler Thromb Vasc Biol. 2020;40(3):697–713.
- Golledge J, Greenhalgh RM, Davies AH. The symptomatic carotid plaque. Stroke. 2000;31(3):774–781.
- Leek JT, Johnson WE, Parker HS, et al. The sva package for removing batch effects and other unwanted variation in high-throughput experiments. Bioinformatics. 2012;28(6):882–883.
- Chen B, Khodadoust MS, Liu CL, et al. Profiling tumor infiltrating immune cells with CIBERSORT. Methods Mol Biol. 2018;1711:243–259.
- Ginestet C. ggplot2: elegant graphics for data analysis. J R Stat Soc A Stat. 2011;174(1):245–246.
- Subramanian A, Tamayo P, Mootha VK, et al. Gene set enrichment analysis: a knowledge-based approach for interpreting genome-wide expression profiles. Proc Natl Acad Sci U S A. 2005;102(43):15545–15550.
- Liberzon A, Subramanian A, Pinchback R, et al. Molecular signatures database (MSigDB) 3.0. Bioinformatics. 2011;27(12):1739–1740.
- Ritchie ME, Phipson B, Wu D, et al. limma powers differential expression analyses for RNA-sequencing and microarray studies. Nucleic Acids Res. 2015;43(7):e47.
- Kolde R. Pheatmap: Pretty Heatmaps. 2015. https://rdrr.io/cran/pheatmap/
- Yu G, Wang L-G, Han Y, et al. He Q: clusterProfiler: an R package for comparing biological themes among gene clusters. OMICS. 2012;16(5):284–287.
- Hild M, Beckmann B, Haas SA, et al. An integrated gene annotation and transcriptional profiling approach towards the full gene content of the Drosophila genome. Genome Biol. 2003;5(1):R3
- Zhao W, Wang D, Zhao J, et al. Bioinformatic analysis of retinal gene function and expression in diabetic rats. Exp Ther Med. 2017;14(3):2485–2492.
- Kamtchum-Tatuene J, Wilman A, Saqqur M, et al. Carotid plaque with high-risk features in embolic stroke of undetermined source: systematic review and meta-analysis. Stroke. 2020;51(1):311–314.
- Wolf D, Ley K. Immunity and inflammation in atherosclerosis. Circ Res. 2019;124(2):315–327.
- Stary HC, Chandler AB, Dinsmore RE, et al. A definition of advanced types of atherosclerotic lesions and a histological classification of atherosclerosis. A report from the Committee on Vascular Lesions of the Council on Arteriosclerosis, American Heart Association. Circulation. 1995;92(5):1355–1374.
- Vorobyova DA, Lebedev AM, Vagida MS, et al. Immunological analysis of human atherosclerotic plaques in ex vivo culture system. Kardiologiia. 2016;11_2016(11):78–85.
- Lebedeva A, Vorobyeva D, Vagida M, et al. Ex vivo culture of human atherosclerotic plaques: a model to study immune cells in atherogenesis. Atherosclerosis. 2017;267:90–98.
- Fernandez DM, Rahman AH, Fernandez NF, et al. Single-cell immune landscape of human atherosclerotic plaques. Nat Med. 2019;25(10):1576–1588.
- Meeuwsen J, van Duijvenvoorde A, Gohar A, et al. High levels of (un)switched memory B cells are associated with better outcome in patients with advanced atherosclerotic disease. J Am Heart Assoc. 2017;6(9):e005747.
- Tabas I, Lichtman AH. Monocyte-macrophages and T cells in atherosclerosis. Immunity. 2017;47(4):621–634.
- Herrero-Fernandez B, Gomez-Bris R, Somovilla-Crespo B, et al. Immunobiology of atherosclerosis: a complex net of interactions. Int J Mol Sci. 2019;20(21):5293.
- Libby P, Ridker PM, Maseri A. Inflammation and atherosclerosis. Circulation. 2002;105(9):1135–1143.
- Hansson GK, Hermansson A. The immune system in atherosclerosis. Nat Immunol. 2011;12(3):204–212.
- Hansson GK. Inflammation, atherosclerosis, and coronary artery disease. N Engl J Med. 2005;352(16):1685–1695.
- Zernecke A, Weber C. Chemokines in the vascular inflammatory response of atherosclerosis. Cardiovasc Res. 2010;86(2):192–201.
- Stemme S, Faber B, Holm J, et al. T lymphocytes from human atherosclerotic plaques recognize oxidized low density lipoprotein. Proc Natl Acad Sci U S A. 1995;92(9):3893–3897.
- Sallusto F, Geginat J, Lanzavecchia A. Central memory and effector memory T cell subsets: function, generation, and maintenance. Annu Rev Immunol. 2004;22:745–763.
- Ammirati E, Cianflone D, Vecchio V, et al. Effector memory T cells are associated with atherosclerosis in humans and animal models. J Am Heart Assoc. 2012;1(111):27–41.
- Zernecke A. Dendritic cells in atherosclerosis: evidence in mice and humans. Arterioscler Thromb Vasc Biol. 2015;35(4):763–770.
- Koltsova EK, Ley K. How dendritic cells shape atherosclerosis. Trends Immunol. 2011;32(11):540–547.
- Alberts-Grill N, Denning TL, Rezvan A, et al. The role of the vascular dendritic cell network in atherosclerosis. Am J Physiol Cell Physiol. 2013;305(1):C1–C21.
- van Dijk RA, Duinisveld AJ, Schaapherder AF, et al. A change in inflammatory footprint precedes plaque instability: a systematic evaluation of cellular aspects of the adaptive immune response in human atherosclerosis. J Am Heart Assoc. 2015;4(4):e001403.
- Vivier E, Tomasello E, Baratin M, et al. Functions of natural killer cells. Nat Immunol. 2008;9(5):503–510.
- Clerc G, Rouz PM. Lymphocyte subsets in severe atherosclerosis before revascularization. Ann Intern Med. 1997;126(12):1004–1005.
- Martinez-Rodriguez JE, Munne-Collado J, Rasal R, et al. Expansion of the NKG2C + natural killer-cell subset is associated with high-risk carotid atherosclerotic plaques in seropositive patients for human cytomegalovirus. Arterioscler Thromb Vasc Biol. 2013;33(11):2653–2659.
- Whitman SC, Rateri DL, Szilvassy SJ, et al. Depletion of natural killer cell function decreases atherosclerosis in low-density lipoprotein receptor null mice. Arterioscler Thromb Vasc Biol. 2004;24(6):1049–1054.
- Selathurai A, Deswaerte V, Kanellakis P, et al. Natural killer (NK) cells augment atherosclerosis by cytotoxic-dependent mechanisms. Cardiovasc Res. 2014;102(1):128–137.
- Hori S, Nomura T, Sakaguchi S. Control of regulatory T cell development by the transcription factor Foxp3. Science. 2003;299(5609):1057–1061.
- Shevach EM. From vanilla to 28 flavors: multiple varieties of T regulatory cells. Immunity. 2006;25(2):195–201.