Abstract
High-density lipoprotein (HDL) particles comprising heterogeneous subclasses of different functions exert anti-inflammatory effects by interacting with immune-response cells. However, the relationship of HDL subclasses with immune-response cells in metabolic unhealth/obesity has not been defined clearly. The purpose of this study was to delineate the relational changes of HDL subclasses with immune cells and inflammatory markers in metabolic unhealth/obesity to understand the role of anti-inflammatory HDL subclasses. A total of 316 participants were classified by metabolic health. HDL subclasses were detected by microfluidic chip electrophoresis. White blood cell (WBC) counts and lymphocytes were assessed using automatic haematology analyser. Levels of high-sensitivity C-reactive protein (hs-CRP) and interleukin 6 (IL-6) were measured. In our study, not only the distribution of HDL subclasses, but also HDL-related structural proteins changed with the deterioration of metabolic disease. Moreover, lymphocytes and inflammation factors significantly gradually increased. The level of HDL2b was negatively associated with WBC, lymphocytes and hs-CRP in multivariable linear regression analysis. In multinomial logistic regression analysis, high levels of HDL3 and low levels of HDL2b increased the probability of having an unfavourable metabolic unhealth/obesity status. We supposed that HDL2b particles may play anti-inflammation by negatively regulating lymphocytes activation. HDL2b may be a therapeutic target for future metabolic disease due to the anti-inflammatory effects.
Introduction
Reverse cholesterol transport (RCT) is considered to represent the classical anti-atherosclerotic function of high-density lipoprotein cholesterol (HDL-C). HDL-C transfers excess cholesterol molecules from the macrophages of chronically inflamed vascular walls and peripheral cells to the liver for excretion into bile [Citation1]. In addition to the RCT effect, HDL particles also manifest anti-inflammatory and anti-coagulation effects [Citation2,Citation3]. Hidesuke Kaji has proposed that HDL interact with innate and adaptive immune systems to play an anti-inflammation effect, such as HDL inhibiting T-cell activation and the expression of inflammatory factors in macrophages and dendritic cells [Citation4]. However, HDL subclasses are less known these roles under metabolic unhealth and obesity conditions.
It has been postulated that dyslipidaemia due to a low level of HDL-C is a major independent risk factor for cardiovascular disease (CVD). However, a study involving a cohort of 33,000 patients with end-stage renal disease revealed a U-shaped association between the HDL-C level and CVD mortality [Citation5] and increasing HDL-C concentrations did not reduce the risk of cardiovascular disease [Citation6]. The picture of HDL-C as the “good” cholesterol has eroded. Previous also have indicated that epigenetic changes within the ABCA1 (ATP-binding cassette A1) gene promoter among French-Canadian founder population contribute to the interindividual variability in plasma HDL-C concentrations and are associated with coronary artery disease (CAD) expression with negatively related to HDL-2 phospholipid level and HDL particle size [Citation7], while MicroRNA-144 antagonism in male mice increase circulating HDL cholesterol levels by remodeling the HDL particle, and enhance reverse cholesterol transport through performing an effect with 3′-UTR harbours binding sites for miR-33, miR-758, and miR-26 of Cyp7b1 which have been shown to regulate Abca1 and lipid metabolism [Citation8].
In general, an isolated static measurement of HDL-C does not represent a robust strategy to assess the efficiency of a dynamic transport system for HDL, nor the multiple additional functions of these particles. On the basis of density, HDL particles can be separated into two main subfractions by ultracentrifugation: HDL2 and HDL3 [Citation9]. HDL2 and HDL3 can be sub-fractionated further into five distinct subclasses of decreasing size: HDL2b, HDL2a, HDL3a, HDL3b, and HDL3c. HDL3 occupies the density range d 1.125–1.21 g/ml, and HDL2 occupies the lower density range of the spectrum (d 1.063–1.125 g/ml) [Citation10]. HDL particles are highly complex and carry various proteins (apo molecules, enzymes, lipid-transfer proteins, acute-phase proteins, complement components and other proteins), ∼100 lipid species, and ∼12 microRNAs [Citation6]. Retinol-binding protein (RBP), one of major components of the HDL proteome, delivers retinol from liver to peripheral tissues and is associated with metabolic disease. Apolipoprotein (apo)A-1 is the most abundant HDL-associated protein and involves in the metabolism of HDL subclasses [Citation11], reverses cholesterol transport [Citation12] and anti-inflammation [Citation13]. Paraoxonase-1 (PON-1), also considered to be an anti-oxidative enzyme among HDL-associated proteins, plays a protective effect against atherosclerosis development in humans and in animal models [Citation14–16]. However, the contents of RBP, apoA-1 and PON-1 in HDL subclasses have not been researched thoroughly.
HDL particles have been detected in chronic inflammatory disease, the acute phase of inflammation, cancer, diabetes mellitus (DM), sepsis, systemic lupus erythematosus and rheumatoid arthritis (RA) [Citation17–19]. During chronic inflammation, normal, protective HDL particles are transformed to pro-inflammatory HDL particles. However, in the chronic inflammation seen in metabolic syndrome (MS), the transformation of HDL subclasses and the correlations with inflammatory factors needs further study. Obesity, a state of chronic low-grade inflammation [Citation20], is a major public health problem worldwide, increasing the risk of disability and mortality from chronic diseases such as CVD, T2DM, cerebrovascular disease and cancer. People with similar body-weight or body mass index (BMI) values have substantially different levels of CVD risk [Citation21]. Risk factors implicated causally in atherogenesis include metabolic disorders and obesity, which can lead to hypertension, hypertriglyceridaemia, T2DM and visceral adiposity. Therefore, the sub-phenotypes have been described as “metabolically unhealthy obesity” (MUO), “metabolically healthy obesity” (MHO) and “metabolically unhealthy normal weight” (MUNW) [Citation22–24]. The Nurses’ Health Study including 90257 women has found that cardiovascular disease risk of women with MHO was increased compared with metabolically healthy normal weight (MHNW) (HR 1.39, 95% CI 1.15–1.68), but risk was considerably higher in women with MUNW (2.43, 2.19–2.68), overweight (2.61, 2.36–2.89) and obesity (3.15, 2.83–3.50) [Citation25]. Importantly, Whitehall II Cohort Study of British Government Workers (N = 2,521) has showed that one-half of healthy obese adults transform to unhealthy obese, and only 10% transform to healthy nonobese follow-up 20 years [Citation26]. Nevertheless, it remains unclear how metabolic risk factors change in individuals with metabolic health in different BMI groups in the long term and how the onset of metabolic disorders affects cardiovascular disease risk among healthy individuals. Inflammation can alter the function of the cells of the artery wall in a manner that drives atherosclerosis [Citation27] and metabolic disease [Citation28]. However, few studies have focussed on the transformation of HDL subclasses and their anti-inflammatory effect in different metabolic unhealth/obese states.
Several methods have been developed to partition HDL into various subclasses: analytical ultracentrifugation, isopycnic equilibrium, vertical auto profile, two-dimensional gel electrophoresis, nuclear magnetic resonance, and ion mobility [Citation9,Citation29]. However, these methods are labour-intensive, expensive, time-consuming, and restricted to research laboratories for measurements of HDL subfractions [Citation29,Citation30]. We utilize microfluidic chip electrophoresis (MCE), the effectiveness has been proven [Citation31], that is rapid, robust, and easy-to-use for HDL subtyping in people suffering from metabolic unhealth or obesity.
In this study, we aim to investigate the transition of HDL subclasses, the alterations to HDL-related proteins, and the correlations between HDL subclasses and immune cells and inflammatory factors in metabolic/obese states.
Materials and methods
Study population
We used the data of Southern China cohort, which was part of the KHDC (Kidney disease, hypertension, diabetes and cardiovascular disease) study. The design and methodology of the KHDC study have been described previously [Citation32]. This study was approved by the ethics committee of the Third Affiliated Hospital of Southern Medical University and supported by Southern Medical University Clinical Research Program.
The present study was conducted in April 2018 in six communities in Wanzhai Town, Zhuhai City, South China. The participants, all Han Chinese, were divided into groups according to the combination of the presence or absence of obesity or overweight and the presence or absence of metabolic unhealth: (i) control (MHNW); (ii) MUNW; (iii) MHO; (iv) MUO. Metabolic unhealth was diagnosed according to the National Cholesterol Education Program Adult Treatment Panel III [Citation33] except waist [Citation34]. Metabolic unhealth was defined as hypertension (systolic blood pressure/diastolic blood pressure ≥ 130/85 mmHg or treatment of previously diagnosed hypertension), a low level of high-density lipoprotein-cholesterol (HDL-C < 1.05 mmol/L in men and < 1.25 mmol/L in women), high triglyceride (TG) level (≥1.7 mmol/L), hyperglycaemia (fasting glucose ≥ 5.6 mmol/L or previously diagnosed T2DM). MHNW was defined as normal weight according to World Health Organisation criteria as BMI of 18.5 kg/m2 to < 25.0 kg/m2 without the indication of metabolic unhealth. MUNW used the definition of having two or more than two criteria of metabolic unhealth with normal weight [Citation23]. The definition of MHO was based on the diagnosis of obesity or overweight (BMI ≥ 25 kg/m2) plus the absence of all of the criteria of metabolic unhealth [Citation34]. MUO used the definition of having two or more than two criteria of metabolic unhealth with obesity or overweight (BMI ≥ 25 kg/m2).
Exclusion criteria were patients: (i) with a malignant tumour, systemic immune disease, lung disease, acute/chronic infectious disease, or alcoholic liver disease; (ii) using lipid-regulating drugs; (iii) younger than 18 years or older than 75 years of age.
Data collection and laboratory measurements
Questionnaires comprised information on demographics (age, sex), lifestyle (cigarette smoking, alcohol consumption, physical exercise), history of disease and anthropometric indices (height, weight, waist circumference and hip circumference). Waist circumference and hip circumference were measured by a trained examiner. They were determined using a measuring tape positioned at the high point of the iliac crest (waist circumference) and at the maximum circumference of the buttocks (hip circumference). The waist-to-hip ratio was calculated as the waist circumference divided by the hip circumference. After resting for 30 min, blood pressure was measured with a calibrated mercury sphygmomanometer.
The level of fasting plasma glucose (FPG) was measured by a standard enzymatic method. The level of fasting insulin was measured using an electrochemiluminescence method. Homoeostatic Model Assessment of Insulin Resistance (HOMA-IR) was calculated as: HOMA-IR = (fasting insulin × fasting glucose)/22.5.
Concentration of RBP were measured by transmission turbidimetry. Concentration of PON1 was determined by using human PON1 ELISA kit (MLBio, Shanghai, China) on Multiskan FC Automatic microplate reader (Thermo Fisher, MA, USA). The level of hs-CRP, apoA-1, and apo B was measured by immunoturbidimetry. The concentration of TG and total cholesterol (TC) was measured by an enzymatic method. The concentration of HDL-C, very low-density lipoprotein-cholesterol (VLDL-C) and low-density lipoprotein–cholesterol (LDL-C) were measured by a colorimetric method. The IL-6 concentration was measured by a chemiluminescence method. WBC counts, lymphocytes, neutrophils counts and monocyte counts were quantified by a Blood Cell Analyser CAL 8000 (mindray, China).
MCE for analyses of HDL subfractions
HDL particles were subtyped based on molecular size and charge using capillary electrophoresis on a microfluidics instrument (MICEP-30; Ardent BioMed, Guangzhou, China). The assay for HDL subtyping was developed for quantification of HDL2b and HDL3 subclasses. The assay kit consists of a microfluidic chip, polymer-gel separation matrix, specific HDL fluorescent dyes, and a microchannel surfactant activator. This kit can fulfil the requirement for a highly separation-efficient, sensitive and repeatable assay. A lipid-specific, proprietary dye was used to stain HDL particles selectively. A fluorescence detector within the instrument detects the dye. Electrophoretic patterns were analysed by a proprietary software program. The percentage of HDL2b can be obtained by calculating the proportion of the peak area of HDL-2b to the whole peak area of HDL using the statistical software within this program. The percentage of HDL3 was calculated in the same way. The total HDL-C concentration can be measured by using an appropriate control.
Briefly, a linear-polymer solution of poly (N, N-dimethyl acrylamide) (Polysciences, Warrington, PA, USA) was used as the separation matrix. Serum samples, calibration materials and quality-control materials were diluted 1:50 in sample buffer (250 mM TAPS, pH 7.5) in the presence of a lipophilic fluorescent dye (Dyomics, Jena, Germany) and allowed to incubate for 5–15 min before loading onto the wells of the microfluidic chip. Separation was carried out by starting the chip run, which executed a software script that applied currents and voltages in a predefined manner. Fluorescence-stained lipoproteins were detected by laser-induced fluorescence at 680 nm. After completion of the chip run, the chip was discarded and the electrodes were cleaned with a designated cleaning chip. The entire procedure was carried out within one hour.
Statistical analyses
Statistical analyses were carried out using SPSS 23.0 (IBM, Armonk, NY, USA). Significance was set at p < .05. Data are shown as the percentage for categorical variables, the mean ± SD for variables with a normal distribution, or the median (25% quartile, 75% quartile) for categorical variables without a normal distribution. Differences between groups were ascertained with one-way ANOVA for the mean value, non-parametric test for the median value, or chi-square test for percentage distribution. Independence of associations was assessed by Pearson correlation analysis using HDL subclasses as the dependent variable. Stepwise multiple regression analysis was done for levels of TG, apoA1, hs-CRP and other known risk factors of HDL subclasses, including age, sex, smoking status, physical exercise, BMI and HOMA-IR. Multinomial logistic regression was undertaken to assess the association between metabolic/obesity status (dependent) and HDL subclasses (independent) by adjusting for age, sex and moderate (exercise 1–3 h a week) physical exercise.
Results
Characteristics of the study subjects
A total of 316 participants from a cross-sectional cohort study in Southern China formed the study cohort. The median age was 55 years, and 68.8% were women. The characteristics of the cohort at baseline were significantly different (p < .05) except for sex, smoking status, physical exercise, and alcohol consumption (). In comparison with that in other groups, MUO participants had inferior metabolic-health status for blood pressure, fasting glucose, fasting insulin, HOMA-IR, lipid levels, and weight. However, levels of HDL-C were lower in the MUO group. MUNW cases had inferior metabolic-health status than that of MHO patients.
Table 1. Characteristics of the study cohort at baseline.
Distribution of HDL subclasses
HDL subclasses were determined by MCE. showed the clinical utility of HDL 2 b-c and HDL3-c measurements in metabolic disease and healthy controls. MUO patients had an ∼8% lower proportion of HDL2b-c (27%) and ∼11% higher proportion of HDL3-c (31%) than that in controls (35% in HDL2b-c, 20% in HDL3-c), respectively (p < .01) (). There was no significant difference in the distribution of HDL subclasses between controls and MHO (35% in HDL2b and 23% in HDL3). In the MUNW group, the HDL2b-c percentage (32%) was lower and the HDL3-c percentage (26%) was higher than that in controls (p < .01). The serum concentration (in mmol/L) of HDL2b-c in the MUO group (0.37 ± 0.15) was significantly lower than that in controls (0.64 ± 0.18), the MHO group (0.57 ± 0.18) and MUNW group (0.50 ± 0.21). The concentration (in mmol/L) of small HDL3-c particles in the MUO group (0.41 ± 0.09) was higher (p < .01) than that in the controls (0.33 ± 0.08), MHO (0.35 ± 0.13) and MUNW (0.38 ± 0.10) groups. These results revealed that HDL2b-c and HDL3-c were closely related to metabolic/obesity status.
Figure 1. Distribution of HDL subclasses between groups. Level of HDL2b-c (%) (A), HDL3-c (%) (B), HDL2b-c (mmol/L) (C) and HDL3-c (mmol/L) (D) in the four groups. The bar height indicates the proportion of each HDL subclasses expressed as a percentage relative to total HDL-protein as determined by absorbance ± standard error. *p < .05, **p < .01 by one-way ANOVA.
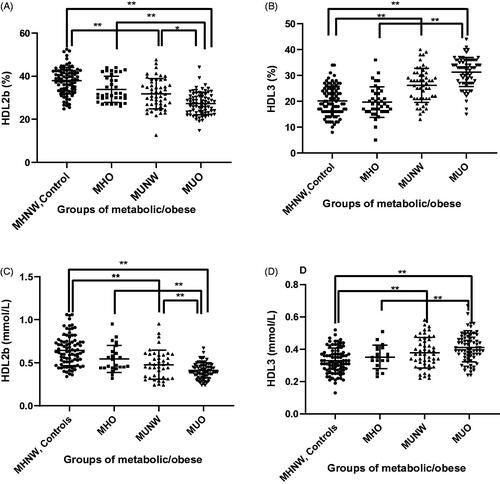
Alterations of HDL-related proteins
The functional properties of HDL subclasses were closely related to structural proteins. Therefore, alterations in structural proteins could affect the functional characteristics of HDL subclasses. Not only the distribution of HDL subclasses, but also HDL-related structural proteins, including apoA-1, RBP and PON-1 changed with the deterioration of metabolic disease. demonstrated the level of HDL-related proteins measurements in metabolic unhealth/obesity. Serum apoA-1 concentration (in mg/dL) were lower in subjects with MUO (1.4 ± 0.05) or MUNW (1.4 ± 0.09) or than in MHO (1.5 ± 0.09) MHNW (1.6 ± 0.04) (). Serum RBP levels (mg/L) were higher in subjects with MUO (65.5 ± 2.0) or MUNW (61.9 ± 3.3) than in MHNW (47.7 ± 1.1) (). PON-1 was also considered to be an anti-oxidative enzyme among HDL-associated proteins, However, there was no significant difference in serum PON-1 concentration among all groups.
Figure 2. Alteration of HDL related proteins between groups. Level of apoA-1 (mg/dL) (A), RBP (mg/L) (B), PON-1 (ng/ml) (C) in the four groups. The bar height indicates the proportion of each protein expressed as a percentage relative to total value as determined by absorbance ± standard error. *p < .05, **p < .01 by one-way ANOVA.
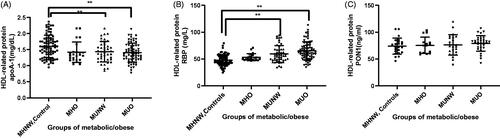
Inflammation levels increase as metabolic unhealth/obesity worsen
Lymphocytes (1.7*109/L, 2.0*109/L, 2.2*109/L and 2.3*109/L in the four groups, separately) (), levels of IL-6 (in pg/ml) (3.4 ± 0.3, 3.6 ± 0.1, 3.9 ± 0.2, 4.1 ± 0.2 in the four groups, separately) () and hs-CRP (mg/L) (1.6 ± 0.5, 1.8 ± 0.2, 3.0 ± 0.7 and 3.5 ± 0.6 in the four groups, separately) () were consistently observed in patients with obesity and abnormal metabolism. No significant difference shown among the distribution of WBC counts, neutrophil counts and monocyte counts (). Both B and T cells released pro-inflammatory cytokines including IL-6 in metabolic-abnormal obesity, bolstering local and systemic inflammation in metabolic-abnormal obesity. Our experimental condition limited the further separation of lymphocyte types.
Figure 3. Inflammatory cells counts and inflammation factors in the four groups. White blood cells count (A), neutrophils count (B), monocyte count (C) and lymphocytes count (D) in controls, MHO, MUNW and MUO. The levels of IL-6 (D) and hs-CRP (E) in controls, MHO, MUNW and MUO. *p < .05, **p < .01 by one-way ANOVA.
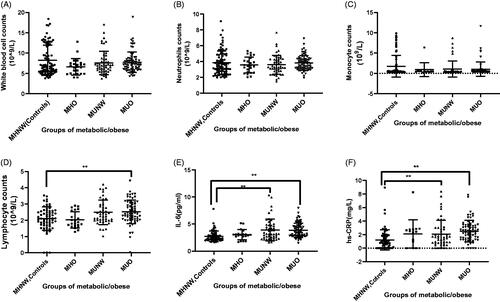
Correlations between HDL subclasses and metabolic variables, inflammatory cytokines and inflammatory cells
showed the correlations between HDL subclasses and metabolic variables (waist, SBP, TG, HDL-C, FPG and IR index), HDL-related proteins (RBP, apoA-1 and PON-1), immune-cells (WBC, lymphocyte and monocyte) and inflammatory factors (IL-6 and hs-CRP). We further carried out multiple regression analysis using a model adjusted by age, sex, current smoking, and physical exercise (). Levels of TG, apoA1, HOMA-IR and hs-CRP were associated directly with the HDL2b-c level (p < .05 for all), while the hs-CRP level was not associated with HDL3-c as shown in .
Table 2. Correlations between HDL subclasses and metabolic factors and inflammatory indexes.
Table 3. Stepwise linear regression models.
After multivariable adjustment, low serum HDL2b (log per 1%; unstandardized coefficient β: −4.80; 95% CI: −8.13 to −1.46; p = .005), and high serum HDL3 (log per 1%; unstandardized coefficient β: 3.80; 95% CI: 1.50 to 6.10; p = .001) were significantly associated with high level of WBC; low serum HDL2b (log per 1%; unstandardized coefficient β: −1.53; 95% CI: −2.54 to −0.41; p = .008), and high serum HDL3 (log per 1%; unstandardized coefficient β: 1.12; 95% CI: 0.36 to 1.89; p = .001) were significantly associated with high levels of lymphocytes; low serum HDL2b (log per 1%; unstandardized coefficient β: −9.67; 95% CI: −14.49 to −4.91; p < .001) was significantly associated with high levels of hs-CRP ().
Table 4. Multivariable linear regression analysis of HDL subclasses and inflammatory indexes.
HDL2b as a protective factor for metabolic unhealth/obesity
Multinomial logistic regression was undertaken to assess the association between metabolic/obesity status and HDL subclasses by adjusting for confounding factors. The HDL2b-c level (%) was a protective factor for MHO (β = −0.193; odds ratio (OR): 0.825; 95% confidence interval (CI): 0.716–0.949; p = .007), MUNW (−0.130; 0.878; 0.795–0.970; 0.010) and MUO (–0.276; 0.758; 0.675–0.852; 0.000) (). HDL3-C was a risk factor for MHO (β = 0.131; OR: 1.140; 95%CI: 1.010–1.286; p = .024), MUNW (0.108; 1.114; 1.019–1.219; 0.000) and MUO (0.269; 1.308; 1.173–1.459; 0.000). A high level of HDL3-c and low level of HDL2b-c increased the probability of having an unfavourable metabolic/obesity status.
Figure 4. The result of multinomial logistic regression analysis. Significant results of multinomial logistic regression analysis to assess the association between HDL subclasses and metabolic syndrome/obesity adjusted by sex, age, moderate physical activity, and quitting smoking. *p < .05. **p < .01. Metabolically healthy normal weight (MHNW) was set as the reference group.
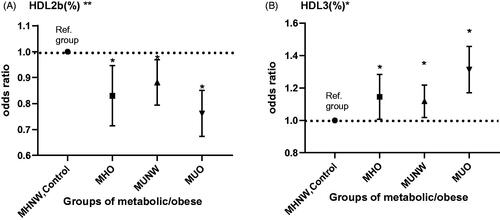
Discussion
The present study demonstrate that metabolic unhealth/obesity are accompanied by modification in HDL subclasses distribution as well as alterations in structural and functionality. Namely, our results have shown dominant presence of HDL subclasses with the deterioration of metabolic status and obesity, rather than deterioration of obesity alone. Metabolic/obese status influence HDL subclasses, which are linked to insulin resistance, high levels of TG, and low levels of apoA-1. In addition to changes in the proportion and concentration of HDL subclasses, the associated structural proteins also change, the concentration of RBP increase, the levels of serum apoA-1 decrease. Importantly, we observe from clinical that HDL2b particles are negatively correlated with WBC counts, lymphocyte counts and the levels of hs-CRP. HDL2b may play an anti-inflammatory effect by negatively regulating lymphocytes activation. A high level of HDL3-c and low level of HDL2b-c increase the probability of having an unfavourable metabolic/obesity status. Our study suggests that HDL2b may be a therapeutic target for future metabolic unhealth/obesity.
1. Abnormal metabolism of HDL subclasses cause a decrease in the HDL2b-c level and an increase in the HDL3-c level in patients suffering from metabolic unhealth/obesity
We find that HDL subclasses distribution is shifted towards smaller HDL3 particles in metabolic unhealth and obesity patients compared to controls. In univariate correlation analysis and stepwise linear regression models, HDL subclasses are closely related to HOMA-IR index, TG and apoA1. We will explain these relationships from the perspective of HDL metabolism.
The ABCA1 transporter transports the efflux of cellular cholesterol to lipid-poor ApoA1 to form nascent pre-β-HDL. The latter can be converted to mature α-HDL (HDL3 and HDL2) through lecithin-cholesterol acyltransferase (LCAT)-based esterification of free cholesterol in nascent pre-β-HDL to cholesterol ester. Mature α-HDL subspecies could interconvert through the action of cholesteryl ester transfer protein (CETP), hepatic lipase (HL), endothelial lipase, and LCAT [Citation35]. Julia T et al. have found LCAT activities increase in obese but otherwise young and healthy women, suggesting a compensatory mechanism, but appear to decrease when in severe comorbidities and pathological conditions [Citation36,Citation37]. LCAT plays an important part in HDL-C metabolism and the conversion from HDL3-c to HDL2b-c [Citation38]. If the serum level of TG increases, LCAT expression could decrease [Citation35]. Oppositely, apoA-1 activates LCAT. Under the condition of insulin insistence (IR), higher levels of TG and lower levels of apoA-1, indispensable enzymes among HDL metabolic pathway were affected, causing abnormal metabolism of HDL subclasses.
In addition, Rye and colleagues have established that with an increase in plasma TG concentration, the activity of CETP and HL is strengthened [Citation39]. The triglyceride-rich HDL can convert to small HDL by HL and endothelial lipase for further participation in transport [Citation35,Citation40]. High levels of TG affect a variety of enzyme activities to lead to abnormal metabolism of HDL subclasses. Whether lowering the levels of TG can improve the abnormal metabolism of HDL in metabolic disease patients still needs further research.
In the state of IR, the lipolytic enzyme lipoprotein lipase (LPL) activity may be reduced, which impacts the maturation of HDL in the circulation [Citation41]. The predominant underlying risk factors for the metabolic disease appear to be abdominal obesity and IR; other associated conditions can be physical inactivity, ageing, and hormonal imbalance [Citation33]. IR interferes with the metabolism of HDL, which may be the underlying pathogenesis of metabolic syndrome. Abnormal metabolism of HDL subclasses causes a decrease in the HDL2b-c level and an increase in the HDL3-c level in patients suffering from metabolic unhealth/obesity.
2. Various correlations of multifunctional factors among various HDL subclasses, indicate HDL subclasses have functional heterogeneity among metabolic unhealth/obesity
Not only the distribution of HDL subclasses, but also HDL-related structural proteins, including apoA1 and RBP change with the deterioration of metabolic unhealth/obesity.
Kahn et al. have reported that high RBP4 levels is associated with components of the MS and inversely correlated with glucose transporter 4 (GLUT4) [Citation42], which is considered to be the principal insulin-stimulated glucose transporter. We find that RBP levels have increased significantly in patients with metabolic disease. A reduction in serum RBP4 levels in response to exercise training showed only in subjects in whom insulin resistance improved [Citation42]. Injection of recombinant RBP4 in normal mice or transgenic overexpression of human RBP4 causes IR. Conversely, genetic deletion of RBP4 enhances insulin sensitivity. Thus, RBP4 may contribute to the pathogenesis of insulin resistance [Citation43]. We also further find that RBP is inversely with HDL2b, but no significant correlation with HDL3. We suspect that RBP content in different HDL subclassses is not consistent. This also indicates that HDL subclasses have functional heterogeneity among metabolic unhealth/obesity.
ApoA-1 mimetic peptide (D-4F) administration in Sprague-Dawley rat is shown to increase anti-oxidant capacity and anti-inflammatory properties, thus conferring robust vascular protection [Citation44]. Free fatty acids activate IKK-k/NF-kB pathway by TLR4, causing overexpression of adhesion molecules and pro-inflammatory factors such as ICAM-1, VCAM-1, IL-6 and TNF-α. HDL or ApoA1, reduced TLR4 migration into lipid rafts, thereby blocked NF-kB activation in endothelial cells to play an anti-inflammation effect [Citation13]. Multivariable linear regression analyses demonstrate that HDL2b is positively with apoA-1, while negatively with inflammatory cells and inflammatory factors, indicating that HDL2b-c may play an anti-inflammation effect.
Previous research has reported PON-1 activity is more closely related to the HDL particle concentration or large HDL particles than to HDL cholesterol in T2DM patients [Citation45], partly consistent with the result of our study. Stepwise linear regression models show a positive correlation between PON1 concentration and percentage of HDL2b-c, while PON1 concentration is excluded of the stepwise linear regression models of HDL3-c percentage.
The anti-inflammatory, anti-coagulation, anti-atherosclerotic function and RCT effect of HDL particles are closely with HDL structural protein, such as apoA1. However, certain HDL-related protein promotes the development of disease, for example, high RBP4 levels is associated with components of the MS. Therefore, HDL-related proteins change, HDL particles function and their mechanisms in disease will also change.
3. Univariate correlations of inflammatory cells and factors among various HDL subclasses, indicate HDL subclasses have potential anti-inflammation heterogeneity among metabolic unhealth/obesity
Inflammation levels including IL-6 and hs-CRP, and lymphocytes counts are higher in the metabolically unhealthy participants (). The relationships between HDL particles and immunocytes has been attracted more and more attention, but the studies on HDL subclasses are still lacking.
Westerterp et al. have demonstrated that HDL reversed the inflammatory response by promoting efflux lipids from lipid-loaded macrophages [Citation46]. HDL has been shown to interact with lipid rafts on the surface of lipid-carrying macrophages and to deplete cholesterol from these microdomains in an active through ABCA1 and ABCG1 or a passive/diffusional manner [Citation12,Citation47]. HDL particles prevent foam cell formation by this way, thus preventing atherosclerosis cardiovascular disease [Citation47]. On the contrary, blockade or dysfunctional ABCA1 and ABCG1 receptors leads to cholesterol efflux disorder, which activate of the TLR-related NLRP3 inflammasome pathway in macrophage further activating T cells [Citation46,Citation47]. Activated T cells secrete macrophage-colony stimulating factor (GM–CSF), which interact with GM–CSF receptor to enhance macrophage secretion of interleukins (IL-23, IL-12, and IL-6) as well as further induce T-cell expansion [Citation47]. Gemma Vilahur has proposed that HDL lessening T-cell response impede the development of chronic inflammatory disorders thereby being a novel atherosclerosis target [Citation48]. Appropriate HDL2b-related cholesterol efflux via ABCA1 and ABCG1 [Citation1] impedes intracellular cholesterol loading in macrophages preventing an aberrant inflammatory response [Citation17]. However, we find HDL3 may have potential pro-inflammatory effect. An explanation for the positive correlations of HDL 3 with inflammatory indexes in our study could be the fact that normal, protective HDL could transform to pro-inflammatory HDL [Citation17].
In our study, we observe from clinical that HDL2b particle is negatively correlated with lymphocyte counts, the levels of hs-CRP and the levels of IL-6, while HDL3 is positively with inflammatory indexes, suggesting HDL subclasses have potential anti-inflammation heterogeneity among metabolic unhealth/obesity.
4. HDL2b may be a therapeutic target for future metabolic unhealth/obesity, and MCE could be applied for clinical high-accuracy HDL subtyping
In the long term, the risk of CVD and early death is higher in MUO cases than that in MHO patients [Citation34]. Weight loss and a healthy lifestyle that can aid transition from MUO to MHO, and reduce cardiometabolic risk in individuals with MUO. However, reaching and maintaining a normal weight with lifestyle intervention alone is very difficult [Citation25,Citation34]. Moreover, epidemiologic studies and meta-analyses have demonstrated that people with MHO are at a higher risk of CVD in comparison with that in healthy people [Citation25]. The mechanisms that link these risk factors to atherogenesis are incompletely understood, but these risk factors also participate in activation of inflammatory pathways [Citation49]. Nicholls and colleagues have showed that infusions of recombinant HDL (75 mg/kg of ApoA1 Milano) inhibited expression of vascular cell adhesion molecule-1 and chemokines [Citation50]. Therefore, HDL subclasses could be targets for the treatment of chronic inflammation in metabolic diseases due to their anti-inflammatory effects.
Currently, lipid-modifying drugs such as statins, cholesteryl ester transfer protein inhibitors, fibrates and niacin often increase cholesterol concentrations of specific HDL subclasses [Citation10]. Statin drugs are associated with a modest 5%–7% increase in HDL-C concentration due to complex alterations in lipoprotein AI and lipoprotein AII metabolism, as well as synthesis apo AI. While the major beneficial effect of statins is attributed to its strong capacity to decrease LDL-C levels. In clinical studies fenofibrate administration lead to an increased synthesis of apo AII with a minimal increase in apo AI synthesis [Citation10]. Novel therapies are well anticipated such as selectively raising the levels of functionally superior HDL subclasses, thereby reducing cardiovascular risk in patients. Hypoglycaemic drugs have certain effects on lipid metabolism. Metformin is able to decrease intestine-derived TG-rich lipoproteins measured in plasma such as chylomicrons and activate AMP-activated protein kinase (AMPK) to modulate cholesterol synthesis in T2DM [Citation51]. The thiazolidendiones, peroxisome proliferator-activated receptor (PPAR) g-agonists, predominantly lessen the flux of free fatty acids from adipose tissue to the liver [Citation52]. But, the effects of hypoglycaemic drugs on HDL subclasses remains to further study.
Diets rich in polyunsaturated fats can improve the anti-inflammatory capacity of HDL particles [Citation53], and a low-fat, high-fibre diet in combination with exercise can convert HDL particles from a pro-inflammatory state to an anti-inflammatory state [Citation54]. Exercise without a restricted diet can improve the anti-inflammation capacity of HDL3 in MS patients [Citation55]. Our study suggest that HDL2b may be a therapeutic target for future metabolic disease due to its potential anti-inflammatory effect. However, showing the clinical utility of HDL subclasses using efficient and easy-to-use measurement methods is challenging. MCE offers several advantages over those of existing methods. The accuracy of MCE has been verified. MCE is less time-consuming and requires a lower sample size than that for ultracentrifugation and other methods [Citation31]. MCE has been applied to detect HDL subclasses and could be employed in the clinic. However, MCE still has the weakness as lacking of broad-scale clinical application due to high cost and throughput in the middle level (with 12 samples per chip) among utilization.
Conclusions
In summary, our study investigates the potential anti-inflammatory of HDL sunclass in metabolic unhealth/obesity. We observe from clinical that HDL2b particle is negatively correlated with lymphocyte counts and the levels of hs-CRP, and negatively regulated lymphocytes activation. HDL2b may be a therapeutic target for future metabolic disease due to its potential anti-inflammatory effect. MCE has been applied to detect HDL subclasses and could be employed in the clinic.
Acknowledgments
The authors thank to all residents in Wanzhai Town, Zhuhai City. We would like to thank the laboratory of The Third Affiliated Hospital of Southern Medical University for providing biochemical tests. We would like to thank Guangdong Ardent Biomed Co. Ltd for providing the support of microfluidic chips technique.
Disclosure statement
I declare that we have no competing interests.
Additional information
Funding
References
- Tall AR, Yvan-Charvet L, Terasaka N, et al. HDL, ABC transporters, and cholesterol efflux: implications for the treatment of atherosclerosis. Cell Metab. 2008;7(5):365–375.
- Nicholls SJ, Dusting GJ, Cutri B, et al. Reconstituted High-Density lipoproteins inhibit the acute Pro-Oxidant and proinflammatory vascular changes induced by a periarterial collar in normocholesterolemic rabbits. Circulation. 2005;111(12):1543–1550.
- Fotakis P, Kothari V, Thomas DG, et al. Anti-inflammatory effects of HDL (high-density lipoprotein) in macrophages predominate over proinflammatory effects in atherosclerotic plaques. Arterioscler Thromb Vasc Biol. 2019;39(12):e253–e272.
- Kaji H. High-Density lipoproteins and the immune system. J Lipids. 2013;2013:684903–684908.
- Moradi H, Streja E, Kashyap ML, et al. Elevated high-density lipoprotein cholesterol and cardiovascular mortality in maintenance hemodialysis patients. Nephrol Dial Transplant. 2014;29(8):1554–1562.
- F K. High-Density lipoprotein in chronic kidney diseases — the devil is in the detail. J Am Soc Nephrol. 2018;29:1356–1371.
- Guay S-P, Brisson D, Munger J, et al. ABCA1 gene promoter DNA methylation is associated with HDL particle profile and coronary artery disease in familial hypercholesterolemia. Epigenetics. 2012;7(5):464–472.
- Cheng J, Cheng A, Clifford BL, et al. MicroRNA-144 silencing protects against atherosclerosis in male, but not female mice. Arterioscler Thromb Vasc Biol. 2020;40(2):412–425.
- Rosenson RS, Brewer HB, Chapman MJ, et al. HDL measures, particle heterogeneity, proposed nomenclature, and relation to atherosclerotic cardiovascular events. Clin Chem. 2011;57(3):392–410.
- Woudberg NJ, Pedretti S, Lecour S, et al. Pharmacological intervention to modulate HDL: What do We target? Front Pharmacol. 2017;8:989.
- Gebhard C, Rhainds D, He G, et al. Elevated level of lecithin:cholesterol acyltransferase (LCAT) is associated with reduced coronary atheroma burden. Atherosclerosis. 2018;276:131–139.
- Landry YD, Denis M, Nandi S, et al. ATP-binding cassette transporter A1 expression disrupts raft membrane microdomains through its ATPase-related functions. J Biol Chem. 2006;281(47):36091–36101.
- Cheng AM, Handa P, Tateya S, et al. Apolipoprotein A-I attenuates palmitate-mediated NF-κB activation by reducing Toll-like receptor-4 recruitment into lipid rafts. PLoS One. 2012;7(3):e33917.
- Triolo M, Annema W, Dullaart RPF, et al. Assessing the functional properties of high-density lipoproteins: an emerging concept in cardiovascular research. Biomark Med. 2013;7(3):457–472.
- Deakin SP, James RW. Genetic and environmental factors modulating serum concentrations and activities of the antioxidant enzyme paraoxonase-1. Clin Sci (Lond)). 2004;107(5):435–447.
- Graner M, et al. Association of paraoxonase-1 activity and concentration with angiographic severity and extent of coronary artery disease. J Am Coll Cardiol. 2006;47(12):2429–2435.
- Hahn BH, Grossman J, Ansell BJ, et al. Altered lipoprotein metabolism in chronic inflammatory states: proinflammatory high-density lipoprotein and accelerated atherosclerosis in systemic lupus erythematosus and rheumatoid arthritis. Arthritis Res Ther. 2008;10(4):213–213.
- Holzer M, Wolf P, Curcic S, et al. Psoriasis alters HDL composition and cholesterol efflux capacity. J Lipid Res. 2012;53(8):1618–1624.
- Nob Court E, et al. Defective antioxidative activity of small dense HDL3 particles in type 2 diabetes: relationship to elevated oxidative stress and hyperglycaemia. Diabetologia. 2005;48(3):529–538.
- Ying W, Fu W, Lee YS, et al. The role of macrophages in obesity-associated islet inflammation and β-cell abnormalities. Nat Rev Endocrinol. 2020;16(2):81–90.
- Gonzalez-Muniesa P, et al. Obesity. Nat Rev Dis Primers. 2017;3:17034.
- M B. Metabolically healthy obesity. Endocr Rev. 2020;41(3):405–420.
- Stefan N, Schick F, Häring H. Causes, characteristics, and consequences of metabolically unhealthy normal weight in humans. Cell Metab. 2017;26(2):292–300.
- Smith GI, Mittendorfer B, Klein S. Metabolically healthy obesity: facts and fantasies. J Clin Invest. 2019;129(10):3978–3989.
- Eckel N, Li Y, Kuxhaus O, et al. Transition from metabolic healthy to unhealthy phenotypes and association with cardiovascular disease risk across BMI categories in 90 257 women (the nurses' health study): 30 year follow-up from a prospective cohort study. Lancet Diabetes Endocrinol. 2018;6(9):714–724.
- Bell JA, Hamer M, Sabia S, et al. The natural course of healthy obesity over 20 years. J Am Coll Cardiol. 2015;65(1):101–102.
- Kobiyama K, Ley K. Atherosclerosis. Circ Res. 2018;123(10):1118–1120.
- González-Gil EM, C.S. C., J., Inflammation in metabolically healthy and metabolically abnormal adolescents: the HELENA study. Nutr Metab Cardiovasc Dis. 2018;28:77–83.
- Miller WG, Myers GL, Sakurabayashi I, et al. Seven direct methods for measuring HDL and LDL cholesterol compared with ultracentrifugation reference measurement procedures. Clinical Chemistry. 2010;56(6):977–986.
- Asztalos BF, Sloop CH, Wong L, et al. Two-dimensional electrophoresis of plasma lipoproteins: Recognition of new apo A-I-containing subpopulations. Biochim Biophys Acta Lipids Lipid Metab. 1993;1169(3):291–300.
- Mueller O, et al. PROCAM study: risk prediction for myocardial infarction using microfluidic high-density lipoprotein (HDL) subfractionation is independent of HDL cholesterol. Clin Chem Lab Med. 2008;46(4):490–498.
- Ene-Iordache B, Perico N, Bikbov B, et al. Chronic kidney disease and cardiovascular risk in six regions of the world (ISN-KDDC): a cross-sectional study. Lancet Glob Health. 2016;4(5):e307–e319.
- Grundy SM, Cleeman JI, Daniels SR, National Heart, Lung, and Blood Institute, et al. Diagnosis and management of the metabolic syndrome: an American Heart Association/National Heart, Lung, and Blood Institute Scientific Statement. Circulation. 2005;112(17):2735–2752.,
- Stefan N, Häring H, Schulze MB. Metabolically healthy obesity: the low-hanging fruit in obesity treatment? Lancet Diabetes Endocrinol. 2018;6(3):249–258.
- Singh IM, Shishehbor MH, Ansell BJ. High-density lipoprotein as a therapeutic target: a systematic review. JAMA. 2007;298(7):786–798.
- Stadler JT, Lackner S, Mörkl S, et al. Obesity affects HDL metabolism, composition and subclass distribution. Biomedicines. 2021;9(3):242.
- Marsche G, et al. Current understanding of the relationship of HDL composition, structure and function to their cardioprotective properties in chronic kidney disease. Biomolecules. 2020;10(9):1348.
- von Eckardstein A, Nofer JR, Assmann G. High density lipoproteins and arteriosclerosis. Role of cholesterol efflux and reverse cholesterol transport. Arterioscler Thromb Vasc Biol. 2001;21(1):13–27.
- Rye K, Clay MA, Barter PJ. Remodelling of high density lipoproteins by plasma factors. Atherosclerosis. 1999;145(2):227–238.
- Lewis GF, Rader DJ. New insights into the regulation of HDL metabolism and reverse cholesterol transport. Circ Res. 2005;96(12):1221–1232.
- Rashid S, Watanabe T, Sakaue T, et al. Mechanisms of HDL lowering in insulin resistant, hypertriglyceridemic states: the combined effect of HDL triglyceride enrichment and elevated hepatic lipase activity. Clin Biochem. 2003;36(6):421–429.
- Graham TE, Yang Q, Blüher M, et al. Retinol-binding protein 4 and insulin resistance in lean, obese, and diabetic subjects. N Engl J Med. 2006;354(24):2552–2563.
- Yang Q, Graham TE, Mody N, et al. Serum retinol binding protein 4 contributes to insulin resistance in obesity and type 2 diabetes. Nature. 2005;436(7049):356–362.
- Peterson SJ, Husney D, Kruger AL, et al. Long-Term treatment with the apolipoprotein A1 mimetic peptide increases antioxidants and vascular repair in type I diabetic rats. J Pharmacol Exp Ther. 2007;322(2):514–520.
- Dullaart RP, Otvos JD, James RW. Serum paraoxonase-1 activity is more closely related to HDL particle concentration and large HDL particles than to HDL cholesterol in type 2 diabetic and non-diabetic subjects. Clin Biochem. 2014;47(12):1022–1027.
- Westerterp M, Gautier EL, Ganda A, et al. Cholesterol accumulation in dendritic cells links the inflammasome to acquired immunity. Cell Metab. 2017;25(6):1294–1304.e6.
- Vilahur G. High-density lipoprotein benefits beyond the cardiovascular system: a potential key role for modulating acquired immunity through cholesterol efflux. Cardiovasc Res. 2017;113(13):e51–e53.
- Tiniakou I, Drakos E, Sinatkas V, et al. High-density lipoprotein attenuates Th1 and th17 autoimmune responses by modulating dendritic cell maturation and function. J Immunol. 2015;194(10):4676–4687.
- Libby P, Buring JE, Badimon L, et al. Atherosclerosis. Nat Rev Dis Primers. 2019;5(1):56.
- Ibanez B, Giannarelli C, Cimmino G, et al. Recombinant HDLMilano exerts greater anti-inflammatory and plaque stabilizing properties than HDL wild-type. Atherosclerosis. 2012;220(1):72–77.
- van Stee MF, de Graaf AA, Groen AK. Actions of metformin and statins on lipid and glucose metabolism and possible benefit of combination therapy. Cardiovasc Diabetol. 2018;17(1):94
- Phielix E, Szendroedi J, Roden M. The role of metformin and thiazolidinediones in the regulation of hepatic glucose metabolism and its clinical impact. Trends Pharmacol Sci. 2011;32(10):607–616.
- Nicholls SJ, Lundman P, Harmer JA, et al. Consumption of saturated fat impairs the anti-Inflammatory properties of High-Density lipoproteins and endothelial function. J Am Coll Cardiol. 2006;48(4):715–720.
- Roberts CK, Ng C, Hama S, et al. Effect of a short-term diet and exercise intervention on inflammatory/anti-inflammatory properties of HDL in overweight/obese men with cardiovascular risk factors. J Appl Physiol (1985). 2006;101(6):1727–1732.
- Sang H, Yao S, Zhang L, et al. Walk-Run training improves the anti-Inflammation properties of High-Density lipoprotein in patients with metabolic syndrome. J Clin Endocrinol Metab. 2015;100(3):870–879.